In the past several weeks, you have been introduced to a range of statistical data analysis tools. Consider what you have learned in the context of progression of data, information, a
In the past several weeks, you have been introduced to a range of statistical data analysis tools. Consider what you have learned in the context of progression of data, information, and knowledge.
- What are the specific techniques you would consider most helpful in transforming information into knowledge (as opposed to just translating data into information)? Support your discussion with relevant examples, research, and rationale.
The final paragraph (three or four sentences) of your initial post should summarize the one or two key points that you are making in your initial response.
Submission Details
- Your posting should be the equivalent of 1 to 2 single-spaced pages (500–1000 words) in length.
- Since you are engaging in research, be sure to cite in the body of the post and add a reference list in APA format. The excessive use of quotes will directly impact performance since this indicates a lack of comprehension and shows that you May not have mastered the concepts.
In the past several weeks, you have been introduced to a range of statistical data analysis tools. Consider what you have learned in the context of progression of data, information, and knowledge.
· What are the specific techniques you would consider most helpful in transforming information into knowledge (as opposed to just translating data into information)? Support your discussion with relevant examples, research, and rationale.
Converting Information
I have learned that there are many ways to obtain data and there are many ways to utilize the data. The most important thing is making the right decision on how you will use the information and what you will be using it for. You need to understand from a marketing perspective what information works best for your organization. All data is not good data, for example, if I am determining marketing strategies for selling cars, I would not need to know the average cost of travel expenses for people taking the bus on a daily basis. But on the flip side, I could market to the bus riders, but they may think that having a monthly bus pass is way cheaper than having to make a car payment, maintenance, gas, and insurance. Although helpful to know how many people are taking the bus versus driving can help, but the information is not needed. However, these people would not be my target audience. I would target people that have already purchased a car with my dealership or people looking to purchase new cars. Providing them with incentives and loyalty promotions will help to increase sells and possibly obtain new customers through my current customers.
Knowing how to convert data into the knowledge can be very helpful in your decision making when it comes to marketing strategies. One way to do this by making comparisons from one instance to another. For example, State Farm wanted to compare one agent’s sales to average sells of another agent, they could review the results and determine performance. Trending sales and expenses over a period of time could also help with translating data into information. Expositors (2021) states information is transformed into knowledge when the mind assesses it as valuable enough to use later (Expositors, 2021, para.4). The insight gained when obtaining the correct data will reap substantial benefits for your business. A few ways to turn information into knowledge are:
· Define the purpose of your goal
· Understand what you need to know
· Produce a data map
· Properly store and utilize the data
· Translate, visualize, and report the information received
· Transform what you know into action
When these actions are performed, you have a higher chance of increased efficiency and effectiveness. It allows you to conduct a deeper analysis and conclude faster. There are tools and resources available to help with understanding your data.
Therefore, knowing your consumer and the strategies to attract them is key to maintaining a successful business as well as excluded and/or eliminating irrelevant data. When data is transformed into knowledge, it can allow businesses to better decisions that will help to improve company costs. Effective trending and accurate forecasting along with a productive marketing strategy can lay the foundation to longevity and growth. Many think that having knowledge and knowing information are the same, however, if you do not now how to use the information obtained in the correct way, it means nothing. The key is advancing raw numbers into knowledge to perceive actionable enough insight on what you have gathered.
Kashisha C.
Expositors. (2021). Turning Information Into Knowledge. https://expositors.org/turning-information-into-knowledge/
,
Linear Regression.html
Linear Regression
Regression is an extension of the correlation analysis discussed in the previous video. It is one of the most frequently used statistical techniques (in business) because it enables analysts to explain factors that drive the outcomes of interest such as the causality addressed in the previous lectures. While correlation analysis can be used to estimate the degree of association between pairs of variables, regression analysis can be used as a way of assessing causal interdependencies.
Regression is not a singular technique but a group of techniques that are built around a common core. A dependent variable (typically an outcome of interest, such as redemption response rate, sales level) is explained with the help of one or more independent (also known as predictor) variables. There are multiple ways of classifying regression techniques: simple vs. multiple, linear vs. nonlinear and continuous (dependent variable) vs. discrete. As this is the introductory course, you will only have an overview of these broadly defined categories.
The previous section briefly explored the different types of regression models, such as simple versus multiple, linear versus nonlinear, and continuous (dependent variable) versus discrete. The categorization is driven by the following:
- Independent variable considerations, as in simple versus multiple
- Dependent variable characteristics, as in continuous versus discrete
- Independent variable interactions, as in linear versus nonlinear
All these categorizations have important business applications in which they capture the unique nature of particular problems. In this introductory course, you will learn about the differences between simple and multiple regressions.
The fundamental distinction between simple and multiple regression is the number of independent variables. The former uses a single independent variable, while the latter uses multiple independent variables.
There are relatively easy-to-use statistical applications, such as Statistical Analysis Software (SAS) and Statistical Package for the Social Sciences (SPSS) and, most importantly, spreadsheets like Excel that can be used to conduct regression analysis. The advent of these tools, coupled with the virtual explosion of the quantity and variety of transactional data, has played a part in the proliferation of regression applications seen in the last couple of decades.
,
Correlation.html
Correlation
Correlation analysis is a tool for an objective assessment of pairwise associations of individual variables. As discussed earlier, variables are measured on four different scales, with each scale exhibiting different computational properties. The computation of a measure of association depends on the underlying measurement qualities of the variables of interest. There are ten different correlation coefficients used in computation depending on the measurement properties of individual variables (keeping in mind that each variable can have different measurement properties).
The goal of correlation analysis is to estimate the nature of the relationship that might exist between pairs of variables. Therefore, the association between two variables can be expressed in terms of shape and direction. In terms of shape, variables can be related linearly or nonlinearly. In terms of direction, variables can be related directly or inversely.
Linear relationships progress at a constant rate (illustrated by a straight line graph) whereas nonlinear relationships progress at a changing rate (illustrated by a variety of curves). Consider the relationship between promotional spending and sales. If the relationship between the two was linear, then each time promotional spending increases by, let's say, 10 percent, sales would also rise by 10 percent. This fixed progression would continue indefinitely. If the relationship was nonlinear, the 10 percent increase in promotional spending could initially produce a 10 percent increase in sales, but an additional 10 percent increase in promotional spending could have a very different impact on the increase in sales, whether only 1 percent, 20 percent, or any other value.
While calculating a correlation coefficient may be relatively easy, its interpretation often is not. The use of graphical tools, like those discussed in the beginning of the course. Please see the Supplemental media entitled “Correlation vs. Causation” to see a discussion of whether or not one thing causes the other, or is simply correlated with the other.
Additional Materials
media/transcripts/SUO_MBA5008 W4 L2 Correlation vs. Causation.pdf
Correlation vs. Causation Did This Cause That?
As illustrated in the discussion of the t-test lectures, bivariate analysis is a method of statistically comparing two quantities. The second descriptor of bivariate association is direction. As mentioned earlier, variables can be either directly or inversely correlated. The variables are said to be directly correlated when a rise in the magnitude of one will be accompanied by a rise in the other. It is important to note that these changes do not have to be proportional (i.e., if one increases by 50 percent, the other variable does not need to increase by the same amount). They only need to be in the same direction. You see this type of a relationship between near-term sales and sales incentives. If an increase in one variable is associated with a decrease in another, the variables are said to be inversely correlated. For example, gross domestic product and unemployment rate are inversely correlated, as a rise in the former is associated with a decrease in the latter (and vice versa).
"Correlation is not causation" is one of the often-repeated precepts of the scientific method. You should not confuse cause and effect with associative variation (i.e., changes in variable A being systematically associated with changes in variable B). Just because two factors move together does not mean that one causes the other.
The distinction between correlation and causation is important because a good part of business decision making uses drivers of outcomes of interest in a particular context. Consider the promotional planning process. While designing effective product promotions, you need to pay attention to several aspects such as the offer, the creative presentation, and the means by which the product is distributed. These and many other factors are important because they drive the focal outcomes of the promotions such as trial, purchase, and repurchase. In statistical analysis, such purchase and other outcomes are the effects (dependent variables) of promotional activities, which are causes (independent variables). You will learn more about the context of regression in the next two lectures.
Consider a promotion that uses a coupon or other price incentive. You may estimate the association between, or the correlation of, the promotion redemption rate and the value of the offer. Suppose you compute Pearson's product–moment correlation coefficient as 0.6 by capturing the association of the two variables. On the basis of this value, you conclude that there is a reasonably strong, direct correlation between the promotion redemption rate and the value of the offer. However, you cannot conclude that the offer is a driver of redemption. The reason you could not reach that conclusion is methodological—the correlation coefficient is a measure of association, not causation. In other words, you can say that redemption rate and offer value move up and down together, but you cannot argue that increasing the value of the offer will increase the promotion redemption rate.
© 2017 South University
,
Multivariate Regression.html
Multivariate Regression
The fundamental distinction between simple and multiple regression is the number of independent variables—the former uses a single independent variable, while the latter uses multiple independent variables. Multiple regression can effectively become single regression if only a single predictor turns out to be statistically significant.
The differences among individual regression techniques are attributed primarily to the measurement scale of the dependent variable and the nature of the relationships between the assumed dependent and independent variables (for example, linear vs. nonlinear). Based on measurement, there are three different types of outcomes of regression analysis:
- Continuous (such as sales or response rates)
- Discrete-binary (yes vs. no, as in a certain event materializing or not)
- Discrete-multichomous (such as a choice among several brands)
In terms of the type of relationship (between the dependent variable and the independent variables as a group), linear regression assumes that the impact of independent variables is constant across the values of dependent variables while nonlinear formulation assumes that the relationship varies with the levels of dependent variables.
Simple regression is the most direct extension of the correlation analysis discussed earlier—both focus only on two variables. In correlation, both variables are treated in the same manner. On the other hand, in regression, one variable is used to explain the other. Note that the choice of which variable is being explained, or which is the dependent variable, is driven by business need—the same variable, such as the promotional response rate, can be a dependent variable in one situation, as when the goal is to explain its variability, and an independent variable in another situation, as when the goal is to explain variability in sales. Adding another predictor to simple regression will effectively transform it into multiple regression.
There are few real-life situations where single regression is the preferred model because business outcomes are generally influenced by multiple factors. Thus, a certain degree of familiarity with multiple regression techniques is extremely useful for those who will work in a business environment.
Interestingly, some companies are quick to take advantage of the emergent informational opportunities, while others continue to struggle with regression applications. The different outcomes have frequently been due to the degree to which managers are familiar with regression analysis. The better managers run regressions, the better their decisions, and the better the outcomes for the company.
Collepals.com Plagiarism Free Papers
Are you looking for custom essay writing service or even dissertation writing services? Just request for our write my paper service, and we'll match you with the best essay writer in your subject! With an exceptional team of professional academic experts in a wide range of subjects, we can guarantee you an unrivaled quality of custom-written papers.
Get ZERO PLAGIARISM, HUMAN WRITTEN ESSAYS
Why Hire Collepals.com writers to do your paper?
Quality- We are experienced and have access to ample research materials.
We write plagiarism Free Content
Confidential- We never share or sell your personal information to third parties.
Support-Chat with us today! We are always waiting to answer all your questions.
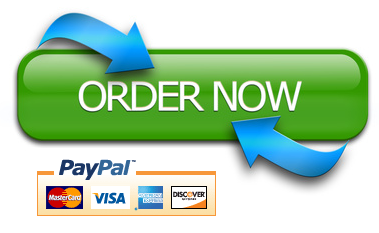