Does La Petit Bakery generate more than $500 per day from cookies, on average?
Requirements: 3 questions
Use the “La Petit Bakery.xlsx” data set, which contains the daily sales of five bakery product categories. Go Data sets “La Petit Bakery.xlsx”
Does La Petit Bakery generate more than $500 per day from cookies, on average?
1) Which would be the most appropriate test? WHY? Which variables will be included in your analysis?
2) What are the skewness and kurtosis of the “Cookies” variable? Does the “Cookies” variable have a normal distribution? WHY?
3) Does La Petit Bakery generate more than $500 per day from cookies, on average? WHY? Did you use a p-value to reach the conclusion? WHY?
NOTE: When reporting your findings, please use the relevant template in “Lecture 2: Comparing Means (templates).”
1 TESTS FOR COMPARING MEANS ONE-SAMPLE Z/T TEST WHEN TO USE? You may perform a one-sample z/t (n < 30) test to compare: (1) a sample to a population (which includes the sample) and (2) a sample to a reference value. Please note that the dependent (outcome) variable should be evaluated on an interval or ratio scale, and, because of underlying assumptions, it is best if it has a normal distribution. HOW TO PERFORM THE ANALYSIS & INTERPRET OUR FINDINGS? Let’s find out if friends and family are an important source of information about movies playing in movie theaters (Q7e). First we estimate the skewness and kurtosis of Q7e (friends & family) to check if this variable has an approximately normal distribution. Q7e has skewness of -0.658 and kurtosis of -0.076. As such, we can conclude that this variable has an approximately normal distribution; we may perform a one-sample t test with confidence in the validity of the results. We perform a one-sample t test comparing the mean of Q7e to the middle point of the scale (2.5). If we find that the Q7e mean is significantly higher than the middle point of the scale, it will mean that friends and family are an important source of information about movies playing in theaters. If the Q7e mean is significantly lower than the middle point of the scale, it will mean that this is not an important source of information. Finally, if the Q7e mean is not significantly different from the middle point of the scale, it will mean that this source of information is of average importance. StatisticQ7e (friends & family)Nbr. of observation500Minimum1.000Maximum4.0001st Quartile3.000Median3.0003rd Quartile4.000Mean2.924Variance (n-1)0.755Standard deviation 0.869Skewness (Fisher)-0.658Kurtosis (Fisher)-0.076
2 How to perform a one-sample z/t test? Test a hypothesis -> Parametric tests -> One-sample t-test and z-test General -> Select the Student’s t test, if sample size <30; otherwise, you may select the Z test. Options -> Theoretical mean = 2.5 Outputs -> Make sure all possible outputs are selected. Charts -> Make sure Distributions and Charts, Box Plots are selected. First we examine the descriptive statistics. We see that 445 students responded to Q7e, with a minimum value of 1.00 and a maximum value of 4.00. The 445 survey respondents rated the importance of friends and family as a source of information about movies playing in movie theaters as 2.924, on average, which exceeds 2.50. What about all students, not just our survey respondents? The probability that students rate friends and family as an important or unimportant source of movie information is close to 100%. Because our sample mean of 2.924 > 2.50, we conclude that students rate friends and family as an important source of information about movies playing in movie theaters. VariableObservationsMinimumMaximumMeanStd. deviationQ7e (friends & family)4451.0004.0002.9240.869Difference0.424t (Observed value)10.281|t| (Critical value)1.965DF444p-value (Two-tailed)< 0.0001alpha0.05
3 T TEST FOR INDEPENDENT SAMPLES WHEN TO USE? T tests for independent samples compare two groups comprising different people. Note that the independent (grouping) variable should be evaluated on a nominal or ordinal scale, and the dependent (outcome) variable should be evaluated on an interval or ratio scale. Because of underlying assumptions, it is best if the dependent variable has a normal distribution, separately for each group. HOW TO PERFORM THE ANALYSIS & INTERPRET OUR FINDINGS? Let’s find out if Undergraduate or Graduate students are more likely to describe themselves as physically and socially active. First we estimate the skewness and kurtosis of Q9 (physically active) and Q10 (socially active), for each group, to check if these variables have approximately normal distributions. We see that all values of the skewness and kurtosis statistics fall within the +2/-2 range suggesting that both survey questions have close to normal distributions, for both groups; as such, we may perform a t test for independent samples with confidence in the validity of the results. StatisticQ9 (physically) | Academic Level-UndergraduateQ9 (physically) | Academic Level-GraduateQ10 (socially) | Academic Level-UndergraduateQ10 (socially) | Academic Level-GraduateNbr. of observations4386243862Minimum1.0001.0001.0001.000Maximum4.0004.0004.0004.000Median3.0003.0003.0003.000Mean3.2223.1803.2863.065Standard deviation (n-1)0.7360.6190.7410.787Skewness (Fisher)-0.759-0.566-0.819-0.533Kurtosis (Fisher)0.4371.6100.285-0.082
4 How to perform a t test for independent samples? Test a hypothesis -> Parametric tests -> Two-sample t-test and z-test General -> One column per variable General -> Sample identifiers = grouping variable Options -> Population variances for the t-test -> Use an F-test √; Cochran-Cox √ Outputs -> Make sure all possible outputs are selected. Charts -> Make sure Distributions and Comparison plots (Box plots) are selected. We examine the Summary statistics table, which shows the number of responses by group. Please note that we use different formulas for the t test when the two groups we are comparing have an equal variance vs. when their variances are unequal. We therefore select “Use an F-test” and the Cochran-Cox approximation in the Options menu. Fisher’s F test evaluates the equality of the variances. If this F test is not significant we can’t reject that null hypothesis that both groups have an equal variance; in this case, XLSTAT automatically estimates the Student’s t test. However, if the F test is significant, we reject the null hypothesis, assuming groups’ variances are unequal. In this case, XLSTAT will utilize the Cochran-Cox approximation. VariableObservationsObs. with missing dataObs. without missing dataMinimumMaximumMeanStd. deviationQ9 (physically) | academic status-Graduate621611.0004.0003.1800.619Q9 (physically) | academic status-Undergradu43824361.0004.0003.2220.736Q10 (socially) | academic status-Graduate620621.0004.0003.0650.787Q10 (socially) | academic status-Undergradua43814371.0004.0003.2860.741
5 Fisher’s F test is not significant for either dependent variable suggesting that the two Academic Level groups have equal variances on both dependent variables, and therefore, the Student’s t test should be utilized both for the “physically active’ and “socially active” survey questions. Our undergraduate survey respondents are more likely to describe themselves as physically and socially active, as evidenced by the mean differences (physically active: 3.222 > 3.180; socially active: 3.286 > 3.065). What about all students, not just our survey respondents? The probability that undergraduate students are more likely to describe themselves as physically active is 33% (1 – 0.670) suggesting that the two student groups are equally like to describe themselves as physically active. The probability that undergraduate students are more likely to describe themselves as socially active is close to 97.1% (1-.029). We therefore conclude that this student group are more likely to describe themselves as socially active. Difference-0.042t (Observed value)-0.427|t| (Critical value)1.965DF495p-value (Two-tailed)0.670alpha0.050Difference-0.222t (Observed value)-2.187|t| (Critical value)1.965DF497p-value (Two-tailed)0.029alpha0.050Ratio0.709F (Observed value)0.709|F| (Critical value)1.428DF160DF2435p-value (Two-tailed0.101alpha0.05Ratio1.128F (Observed value)1.128|F| (Critical value)1.425DF161DF2436p-value (Two-tailed0.498alpha0.05
6
7 T TEST FOR DEPENDENT SAMPLES WHEN TO USE? Paired samples t tests (t tests for dependent samples) are often used for comparing the same observations (e.g., people, units, etc.) at two times or across two situations. Generally, this test is needed when we are testing one group of observations that has been evaluated twice or comparing two groups comprising pairs of similar observations. Note that both dependent (outcome) variables should be evaluated on interval or ratio scales, and, because of underlying assumptions, it is best if both variables come from normal distributions. HOW TO PERFORM THE ANALYSIS & INTERPRET OUR FINDINGS? Let’s find out if the number of screens at a movie theater (Question 5h) or concessions (Question 5b) are more important to movie viewers. First we estimate the skewness and kurtosis of Q5b (food & drink) and Question 5h (no. screens) to check if these variables have approximately normal distributions. Q5b (food & drink) has skewness of -0.065 and kurtosis of -1.134. The skewness and kurtosis of Q5h (no. screens) are -0.506 and -0.358, respectively. We see that both survey questions have close to normal distributions; as such, we may perform a t test for dependent samples with confidence in the validity of the results. StatisticQ5b (drinks & food)Q5h (no. screens)Nbr. of observations500500Minimum1.0001.000Maximum4.0004.0001st Quartile1.0002.000Median3.0003.0003rd Quartile3.0003.000Mean2.3752.821Variance (n-1)0.9920.780Standard deviation 0.9960.883Skewness (Fisher)-0.065-0.506Kurtosis (Fisher)-1.134-0.358
8 How to perform a t test for dependent samples? Parametric tests -> Two-sample t-test and z-test General -> Paired samples Outputs -> Make sure all possible outputs are selected. Charts -> Make sure Distributions and Comparison plots (Box plots) are selected. We examine the descriptive statistics table, which shows the number of responses by group. The number of screens at a movie theater are more important to our survey respondents as evidenced by comparing the two means (2.827 > 2.372). What about all students? The probability that the number of screens and food & drink differ in importance, for all students, is close to 100%. Because the mean for the number of screens is higher than that for food & drink (2.827 > 2.372), we conclude that the number of screens is more important than food & drink. VariableObservationsObs. with m Obs. witho MinimumMaximumMeanStd. deviationQ5b (drinks & food)44404441.0004.0002.3720.994Q5h (no. screens)44404441.0004.0002.8270.881Difference-0.455t (Observed value)-8.064|t| (Critical value)1.965DF443p-value (Two-tailed)< 0.0001alpha0.05
9 ANALYSIS OF VARIANCE (ANOVA) WHEN TO USE? We use ANOVA to examine differences between more than two groups. Note that the independent (grouping) variable(s) should be evaluated on a nominal or ordinal scale, whereas the dependent (outcome) variable should be evaluated on an interval or ratio scale. The ANOVA assumptions require that: (1) residuals (observations – their respective group mean) come from a normal distribution OR the dependent variable has a normal distribution, by group (normality assumption) and (2) the dependent variable has an equal variance across groups (assumption for equality of variances). HOW TO PERFORM THE ANALYSIS & INTERPRET OUR FINDINGS? We want to find out if the importance of going to a movie theater to see a movie varies across Q13 (Year). We also wish to find out whether any such differences would be the same for students residing within a mile or farther than one mile from campus. How to perform an analysis of variance? Modeling data -> ANOVA General -> Select the independent and dependent variables Options -> Interactions/Level: 2 Options -> Model selection should be deselected. Outputs -> General -> Deselect standardized coefficients (√ if testing assumptions). Outputs -> General -> Deselect Predictions and Residuals (√ if testing assumptions). Outputs -> Means -> Multiple comparisons: Check all (apply to all, CIs, sorting up) Outputs -> Means -> Pairwise comparisons: Tukey (HSD) Outputs -> Test assumptions -> Normality test √; Levene’s test, Mean √ (median smaller n) Charts -> Select “Means charts” and “Summary charts, Filter Ys”; deselect all else
10 We examine the descriptive statistics for the independent variable in the output. Variable Observations Minimum Maximum Mean Std. deviation Q2 (importance) 448 1.000 4.000 2.252 0.803 Variable Categories Frequencies % Q12 (residence) Within a mile 249 55.580 More than a mile 199 44.420 Q13 (status) Freshman 71 15.848 Sophomore 82 18.304 Junior 113 25.223 Senior 135 30.134 Graduate student 47 10.491 First we evaluate the normality assumption. If the Shapiro-Wilk test is not significant we conclude that the data satisfies the normality assumption, and we don’t examine the skewness and kurtosis values. Because the Shapiro-Wilk test is significant (W= .957, p < .05) in our analysis, we examine the values of the skewness and kurtosis statistics, by group. We find that Q2 (importance) has skewness and kurtosis values between -2 and +2 for all 10 groups. ANOVA groupSkewness (Fisher)Kurtosis (Fisher)Q2 (importance) | Q12 (residence)|Q13 (Year)-Within a mile|Freshman-0.250-0.768Q2 (importance) | Q12 (residence)|Q13 (Year)-Within a mile|Sophomore-0.128-0.740Q2 (importance) | Q12 (residence)|Q13 (Year)-Within a mile|Junior-0.072-0.816Q2 (importance) | Q12 (residence)|Q13 (Year)-Within a mile|Senior-0.004-0.533Q2 (importance) | Q12 (residence)|Q13 (Year)-Within a mile|Graduate student0.000-1.301Q2 (importance) | Q12 (residence)|Q13 (Year)-More than a mile|Freshman-0.1800.134Q2 (importance) | Q12 (residence)|Q13 (Year)-More than a mile|Sophomore-0.123-0.595Q2 (importance) | Q12 (residence)|Q13 (Year)-More than a mile|Junior-0.070-0.382Q2 (importance) | Q12 (residence)|Q13 (Year)-More than a mile|Senior0.7220.544Q2 (importance) | Q12 (residence)|Q13 (Year)-More than a mile|Graduate student0.5850.665W0.957p-value (Two-tailed)<0.0001alpha0.05
11 We next test for equality of group variances of the dependent variable. Levene’s test evaluates variance equality for groups formed by all independent variables and their interactions. We find equal variances of the dependent variable for all groups (p > .05). If inequality of group variances had been identified, we would have implemented heteroscedasticity (= inequality of variances) consistent standard errors known as Eicker–Huber–White standard errors. To do so, please select Options -> Covariances -> Heteroskedasticity √. You may use any of the heteroscedasticity-consistent standard error formulas (HC); note that HC3 is recommended for sample sizes ≤ 250. Because we didn’t find any violations of the normality assumption or the assumption for equality of variances, we may perform an analysis of variance with confidence in the validity of the results. The Analysis of Variance table shows that there is 98.9% probability the importance of movie theater attendance varies among groups. Source DF Sum of squares Mean squares F Pr > F Model 9 13.611 1.512 2.410 0.011 Error 438 274.886 0.628 Corrected Total 447 288.498 Factorp-valueQ12 (residence)0.072Q13 (Year)0.144Q12 (residence)*Q13 (Year)0.140
12 We next examine the Tukey (HSD) table for the Residence variable. Contrast Difference Standardized difference Critical value Pr > Diff Significant Within vs. More Than 1 Mile -0.090 -1.118 1.965 0.264 No Tukey’s d critical value Category LS means Groups Within a mile 2.214 A More than a mile 2.305 A There is 73.6% probability that students residing within a mile vs. farther than one mile from campus differ in the importance of movie theater attendance. This absence of significant difference is illustrated in the next table where both groups are designated by the same letter “A”. The Tukey (HSD) test shows that freshman students are more likely to see movies at movie theaters than senior students and students pursuing graduate degrees. Contrast Difference Standardized difference Critical value Pr > Diff Significant Freshman vs Graduate student 0.474 3.153 2.739 0.015 Yes Freshman vs Senior 0.368 3.116 2.739 0.017 Yes Tukey’s d critical value Category LS means Groups Freshman 2.496 A Sophomore 2.386 A B Junior 2.266 A B Senior 2.128 B Graduate student 2.022 B
13 Note that the “Freshman” group is designated by the letter “A” only, whereas the Seniors and Graduate Students are designated by “B” only. The Tukey (HSD) test for the interaction shows that: Compared to seniors farther than a mile from campus and students pursuing graduate degrees, freshmen who reside farther than a mile from campus are likely to rate viewing a movie at a movie theater as more important. Contrast Difference Standardized difference Critical value Pr > Diff Significant Freshman*More vs Senior*More 0.612 3.388 3.180 0.026 Yes Freshman*More vs Graduate*More 0.599 3.320 3.180 0.033 Yes Freshman*More vs Graduate*Within 0.643 3.561 3.180 0.015 Yes Tukey’s d critical value: What is an interaction? We observe an interaction when the effect of one variable differs across the levels of another variable. We find evidence of the presence of a significant interactive effect in the Tukey table. The difference between senior and freshman students is not the same for those who reside within a mile vs. farther than a mile from campus: This difference is greater for those who reside farther than a mile from campus. We can conclude that going to a movie theater to see a movie is more important to freshman than to senior students, both farther than a mile from campus (2.643 > 2.031), graduate students residing farther than a mile (2.643 > 2.043), and graduate students residing within a mile from campus (2.643 > 2.000). Category LS means Groups Freshman * More than a mile 2.643 A Sophomore * More than a mile 2.538 A B Freshman * Within a mile 2.349 A B Junior * More than a mile 2.267 A B Junior * Within a mile 2.265 A B Sophomore * Within a mile 2.233 A B Senior * Within a mile 2.225 A B Graduate student * More 2.043 B Senior * More than a mile 2.031 B Graduate student * Within 2.000 B
14 MULTIVARIATE ANALYSIS OF VARIANCE WHEN TO USE? We use multivariate analysis of variance (MANOVA) for comparing means across two or more dependent variables. The multivariate test is typically followed by significance tests on the individual dependent variables. Note that all dependent (outcome) variables should be evaluated on interval or ratio scales, and, because of underlying assumptions, it is best if all dependent variables follow normal distributions. HOW TO PERFORM THE ANALYSIS & INTERPRET OUR FINDINGS? Let’s find out if concessions (Question 5b) and auditorium seating (Question 5e) are equally important for students of varying ages who reside within a mile or farther than a mile from campus. First we estimate the skewness and kurtosis of Q5b (food & drink) and Question 5e (auditorium) to check if these variables have approximately normal distributions. Q5b (food & drink) has skewness of -0.065 and kurtosis of -1.135. The skewness and kurtosis of Q5e (auditorium) are -1.222 and 1.082, respectively. We see that both survey questions have close to normal distributions; as such, we may perform a MANOVA with confidence in the validity of the findings. StatisticQ5b (drinks & food)Q5e (auditorium)Nbr. of observations500500Minimum1.0001.000Maximum4.0004.0001st Quartile1.0003.000Median3.0004.0003rd Quartile3.0004.000Mean2.3753.362Variance (n-1)0.9920.630Standard deviation (n-10.9960.793Skewness (Fisher)-0.065-1.226Kurtosis (Fisher)-1.1341.108
15 How to perform a multivariate analysis of variance? Go to: Modeling data => MANOVA. Options => Interactions/Level: 2 We first examine the descriptive statistics tables. Summary statistics (Quantitative data):VariableObservationsObs. with missing dataObs. without missing dataMinimumMaximumMeanStd. deviationQ5b (drinks & food)44504451.0004.0002.3750.996Q5e (auditorium)44504451.0004.0003.3620.795Summary statistics (Qualitative data):VariableCategoriesCountsFrequencies%Q12 (residence)More than a mile19819844.494Within a mile24724755.506Q14 (age)0 to 18505011.23619-2010610623.82021-2319219243.14624-2638388.539Over 26595913.258Means by factor level:Level VariableQ5b (drinks & food)Q5e (auditorium)Q12 (residence)-Within a mile2.3333.414Q12 (residence)-More than a mile2.4093.320Q14 (age)-21-232.4203.400Q14 (age)-Over 262.1793.349Q14 (age)-24-262.3703.370Q14 (age)-19-202.7373.395Q14 (age)-0 to 182.4753.305
16 Then, we examine the p values. We find that there are no significant differences among any age, residence, or residence x age groups on the two dependent variables as a whole (all p > .22). We may now perform this analysis on each dependent variable separately. Wilks’ test (Rao’s approximation):Q12 (residence)Q14 (age)Q12 (residence)*Q14 (age)Lambda0.9940.9760.986F Observed values1.2901.3350.782DF1288DF2434868868F Critical value3.0171.9491.949p-value0.2760.2220.619
Collepals.com Plagiarism Free Papers
Are you looking for custom essay writing service or even dissertation writing services? Just request for our write my paper service, and we'll match you with the best essay writer in your subject! With an exceptional team of professional academic experts in a wide range of subjects, we can guarantee you an unrivaled quality of custom-written papers.
Get ZERO PLAGIARISM, HUMAN WRITTEN ESSAYS
Why Hire Collepals.com writers to do your paper?
Quality- We are experienced and have access to ample research materials.
We write plagiarism Free Content
Confidential- We never share or sell your personal information to third parties.
Support-Chat with us today! We are always waiting to answer all your questions.
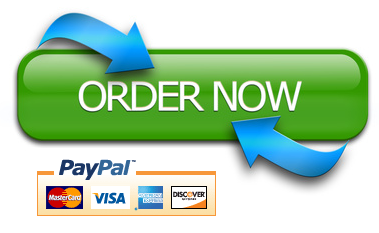