Detailed – Comprehensive Summary for THIS article
Please, answer for your INITIAL posting and discuss the following questions:
.
1. Detailed – Comprehensive Summary for THIS article.
.
How to Write a Summary of an Article
https://owlcation.com/academia/How-to-Write-a-SummaryLinks to an external site.
.
Your Detailed – Comprehensive Summary for THIS article post should be no less of 1,200 words.
.
2. Which are the three most CRITICAL ISSUES of THIS article? Please explain why? and analyze, and discuss in great detail …
For EACH Critical Issue please post at least two strong comprehensive paragraphs
.
3. Which are the three most relevant LESSONS LEARNED of THIS article? Please explain why? and analyze, and discuss in great detail …
For EACH Lesson Learned please post at least two strong comprehensive paragraphs
.
4. Which are the three most important BEST PRACTICES of THIS article? Please explain why? and analyze, and discuss in great detail …
For EACH Best Practice please post at least two strong comprehensive paragraphs
.
5. How can you relate THIS article with the TOPICS COVERED in class? Please explain, analyze, and discuss in great detail …
.
6. Do you see any alignment of the concepts described in THIS article with the class concepts reviewed in class? Which are those alignments and misalignments? Why? Please explain, analyze, and discuss in great detail …
.
.
Artwork Chad Hagen Nonsensical Infographic No. 5 2009, digital
Spotlight
64 Harvard Business Review December 2013
SpotligHt on Making YouR CoMpanY Data-FRienDlY
In the new era, big data will power consumer products and services. by Thomas H. Davenport
Analytics 3.0
T hose of us who have spent years study- ing “data smart” companies believe we’ve already lived through two eras in the use of analytics. We might call them BBD and ABD—before big data and after big data. Or, to use a naming con- vention matched to the topic, we might say that Analytics 1.0 was followed by Analytics 2.0. Generally speaking, 2.0 releases don’t just add some bells and
whistles or make minor performance tweaks. In contrast to, say, a 1.1 version, a 2.0 product is a more substantial overhaul based on new priorities and technical possibilities. When large numbers of companies began capitalizing on vast new sources of unstructured, fast-moving information—big data—that was surely the case.
Some of us now perceive another shift, fundamental and far- reaching enough that we can fairly call it Analytics 3.0. Briefly, it is a new resolve to apply powerful data-gathering and analysis
hbr.org
December 2013 harvard business review 65
the first time, data about production processes, sales, customer interactions, and more were recorded, ag- gregated, and analyzed.
New computing technologies were key. Informa- tion systems were at first custom-built by compa- nies whose large scale justified the investment; later, they were commercialized by outside vendors in more-generic forms. This was the era of the enter- prise data warehouse, used to capture information, and of business intelligence software, used to query and report it.
New competencies were required as well, be- ginning with the ability to manage data. Data sets were small enough in volume and static enough in velocity to be segregated in warehouses for analysis. However, readying a data set for inclusion in a ware- house was difficult. Analysts spent much of their time preparing data for analysis and relatively little time on the analysis itself.
More than anything else, it was vital to figure out the right few questions on which to focus, because analysis was painstaking and slow, often taking weeks or months to perform. And reporting pro- cesses—the great majority of business intelligence activity—addressed only what had happened in the past; they offered no explanations or predictions.
Did people see analytics as a source of competi- tive advantage? In broad terms, yes—but no one spoke in today’s terms of “competing on analytics.” The edge came in the form of greater operational efficiency—making better decisions on certain key points to improve performance.
Analytics 2.0—the era of big data. The ba- sic conditions of the Analytics 1.0 period predomi- nated for half a century, until the mid-2000s, when internet- based and social network firms primarily in Silicon Valley—Google, eBay, and so on—began to amass and analyze new kinds of information. Although the term “big data” wasn’t coined im- mediately, the new reality it signified very quickly changed the role of data and analytics in those firms. Big data also came to be distinguished from
methods not just to a company’s operations but also to its offerings—to embed data smartness into the products and services customers buy.
I’ll develop this argument in what follows, mak- ing the case that just as the early applications of big data marked a major break from the 1.0 past, the cur- rent innovations of a few industry leaders are evi- dence that a new era is dawning. When a new way of thinking about and applying a strength begins to take hold, managers are challenged to respond in many ways. Change comes fast to every part of a business’s world. New players emerge, competitive positions shift, novel technologies must be mas- tered, and talent gravitates toward the most exciting new work.
Managers will see all these things in the coming months and years. The ones who respond most ef- fectively will be those who have connected the dots and recognized that competing on analytics is being rethought on a large scale. Indeed, the first compa- nies to perceive the general direction of change— those with a sneak peek at Analytics 3.0—will be best positioned to drive that change.
The Evolution of Analytics My purpose here is not to make abstract observa- tions about the unfolding history of analytics. Still, it is useful to look back at the last big shift and the context in which it occurred. The use of data to make decisions is, of course, not a new idea; it is as old as decision making itself. But the field of busi- ness analytics was born in the mid-1950s, with the advent of tools that could produce and capture a larger quantity of information and discern patterns in it far more quickly than the unassisted human mind ever could.
Analytics 1.0—the era of “business intelli- gence.” What we are here calling Analytics 1.0 was a time of real progress in gaining an objective, deep understanding of important business phenomena and giving managers the fact-based comprehension to go beyond intuition when making decisions. For
Today it isn’t just online and information firms that can create products and services from analyses of data. It’s every firm in every industry.
66 Harvard Business Review December 2013
SpoTlighT on Making YouR CoMpanY Data-FRienDlY
small data because it was not generated purely by a firm’s internal transaction systems. It was externally sourced as well, coming from the internet, sensors of various types, public data initiatives such as the human genome project, and captures of audio and video recordings.
As analytics entered the 2.0 phase, the need for powerful new tools—and the opportunity to profit by providing them—quickly became apparent. Com- panies rushed to build new capabilities and acquire customers. The broad recognition of the advantage a first mover could gain led to an impressive level of hype but also prompted an unprecedented accelera- tion of new offerings. LinkedIn, for example, has cre- ated numerous data products, including People You May Know, Jobs You May Be Interested In, Groups You May Like, Companies You May Want to Follow, Network Updates, and Skills and Expertise. To do so, it built a strong infrastructure and hired smart, productive data scientists. Its highly successful Year in Review, which summarizes the job changes of people in a member’s network, was developed in just a month. And LinkedIn is not the only company focused on speed. One CEO of a big data start-up told me, “We tried agile [development methodology], but it was too slow.”
Innovative technologies of many kinds had to be created, acquired, and mastered. Big data couldn’t fit or be analyzed fast enough on a single server, so it was processed with Hadoop, an open source soft- ware framework for fast batch data processing across parallel servers. To deal with relatively unstructured data, companies turned to a new class of databases known as NoSQL. Much information was stored and analyzed in public or private cloud-computing envi- ronments. Other technologies introduced during this period include “in memory” and “in database” ana- lytics for fast number crunching. Machine- learning methods (semiautomated model development and
testing) were used to rapidly generate models from the fast-moving data. Black-and-white reports gave way to colorful, complex visuals.
Thus, the competencies required for Analytics 2.0 were quite different from those needed for 1.0. The next-generation quantitative analysts were called data scientists, and they possessed both com- putational and analytical skills. Soon the data scien- tists were not content to remain in the back office; they wanted to work on new product offerings and help shape the business.
Analytics 3.0—the era of data-enriched offerings. During 2.0, a sharp-eyed observer could have seen the beginnings of analytics’ next big era. The pioneering big data firms in Silicon Valley began investing in analytics to support customer-facing products, services, and features. They attracted viewers to their websites through better search al- gorithms, recommendations from friends and col- leagues, suggestions for products to buy, and highly targeted ads, all driven by analytics rooted in enor- mous amounts of data.
Analytics 3.0 marks the point when other large or- ganizations started to follow suit. Today it’s not just information firms and online companies that can create products and services from analyses of data. It’s every firm in every industry. If your company makes things, moves things, consumes things, or works with customers, you have increasing amounts of data on those activities. Every device, shipment, and consumer leaves a trail. You have the ability to analyze those sets of data for the benefit of custom- ers and markets. You also have the ability to embed analytics and optimization into every business deci- sion made at the front lines of your operations.
Like the first two eras of analytics, this one brings new challenges and opportunities, both for the com- panies that want to compete on analytics and for the vendors that supply the data and tools with which
Idea in Brief A New erA Firms dealing with analytics saw everything change when big data came along. Now another major shift is under way, as the emphasis turns to building analytical power into customer products and services.
why It MAtters Data analysis used to add the most value by enabling manag- ers to make better internal de- cisions. The new strategic focus on delivering value to custom- ers has profound implications for where analytics functions sit in organizations and what they must do to succeed.
whAt to Do In the era of Analytics 3.0, managers must drive efforts on at least 10 fronts, from creatively combining data management approaches to shaping new analytics-focused roles to setting guidelines for responding to “digital smoke signals.”
hbr.org
December 2013 harvard business review 67
AnAlytIcs 3.0
About the Spotlight Artist Each month we illustrate our Spotlight package with a series of works from an accomplished artist. The lively and cerebral creations of these photographers, painters, and installation artists are meant to infuse our pages with additional energy and intelligence to amplify what are often complex and abstract concepts.
This month we showcase the illustrations of chad Hagen, a Minnesota-based artist and designer whose work has all the visual cues of a richly detailed info- graphic—without the data. As he once put it, “Welcome to the world of fictional visual information.” View his work at chadhagen.com.
to do so. How to capitalize on the shift is a subject we will turn to shortly. First, however, let’s consider what Analytics 3.0 looks like in some well-known firms—all of which were decidedly offline busi- nesses for most of their many decades in operation.
The Next Big Thing, in Beta The Bosch Group, based in Germany, is 127 years old, but it’s hardly last-century in its application of analytics. The company has embarked on a series of initiatives across business units that make use of data and analytics to provide so-called intelligent customer offerings. These include intelligent fleet management, intelligent vehicle-charging infra- structures, intelligent energy management, intel- ligent security video analysis, and many more. To identify and develop these innovative services, Bosch created a Software Innovations group that focuses heavily on big data, analytics, and the “In- ternet of Things.”
Schneider Electric, a 170-year-old company based in France, originally manufactured iron, steel, and armaments. Today it focuses primarily on energy management, including energy optimization, smart- grid management, and building automation. It has acquired or developed a variety of software and data ventures in Silicon Valley, Boston, and France. Its Advanced Distribution Management System, for ex- ample, handles energy distribution in utility compa- nies. ADMS monitors and controls network devices, manages service outages, and dispatches crews. It gives utilities the ability to integrate millions of data points on network performance and lets engineers use visual analytics to understand the state of the network.
One of the most dramatic conversions to data and analytics offerings is taking place at General Elec- tric, a company that’s more than 120 years old. GE’s manufacturing businesses are increasingly becom- ing providers of asset and operations optimization services. With sensors streaming data from turbines, locomotives, jet engines, and medical-imaging de- vices, GE can determine the most efficient and effec- tive service intervals for those machines. To assem- ble and develop the skilled employees needed for this work, the company invested more than $2 bil- lion in a new software and analytics center in the San Francisco Bay area. It is now selling technology to other industrial companies for use in managing big data and analytics, and it has created new technol- ogy offerings based on big data concepts, including
Predix (a platform for building “industrial internet” applications) and Predictivity (a series of 24 asset or operations optimization applications that run on the Predix platform across industries).
UPS, a mere 107 years old, is perhaps the best ex- ample of an organization that has pushed analytics out to frontline processes—in its case, to delivery routing. The company is no stranger to big data, hav- ing begun tracking package movements and trans- actions in the 1980s. It captures information on the 16.3 million packages, on average, that it delivers daily, and it receives 39.5 million tracking requests a day. The most recent source of big data at UPS is the telematics sensors in more than 46,000 com- pany trucks, which track metrics including speed, direction, braking, and drivetrain performance. The waves of incoming data not only show daily perfor- mance but also are informing a major redesign of drivers’ routes. That initiative, called ORION (On- Road Integrated Optimization and Navigation), is arguably the world’s largest operations research project. It relies heavily on online map data and op- timization algorithms and will eventually be able to reconfigure a driver’s pickups and deliveries in real time. In 2011 it cut 85 million miles out of drivers’ routes, thereby saving more than 8.4 million gal- lons of fuel.
The common thread in these examples is the resolve by a company’s management to compete on analytics not only in the traditional sense (by improving internal business decisions) but also by creating more-valuable products and services. This is the essence of Analytics 3.0.
Some readers will recognize the coming era as the realization of a prediction made long ago. In their 1991 book 2020 Vision, Stan Davis and Bill Davidson argued that companies should “informationalize” their businesses—that is, develop products and ser- vices on the basis of information. They observed that companies emit “information exhaust” that could be captured and used to “turbocharge” their offerings. At the time, their ideas gained traction only among companies already in the information business, such as Quotron (stock data) and the Official Airline Guide (flight data). But today banks, industrial manufactur- ers, health care providers, retailers—any company, in any industry, that is willing to exploit the possi- bilities—can develop valuable products and services from their aggregated data.
Davis and Davidson wrote at a time when supply- ing information was enough. But these days we are
68 Harvard Business Review December 2013
SpoTlighT on Making YouR CoMpanY Data-FRienDlY
inundated with information and have little time to turn it into insight. Companies that were informa- tion providers must become insight providers, us- ing analytics to digest information and tell us what to do with it. Online businesses, with vast amounts of clickstream data at their disposal, have pioneered this approach: Google, LinkedIn, Facebook, Amazon, and others have prospered not by giving customers information but by giving them shortcuts to deci- sions and actions. Companies in the conventional in- formation industry are now well along this path too.
Ten Requirements for Capitalizing on Analytics 3.0 This strategic change in focus means a new role for analytics within organizations. Companies will need to recognize a host of related challenges and respond with new capabilities, positions, and priorities.
Multiple types of data, often combined. Or- ganizations will need to integrate large and small vol- umes of data from internal and external sources and in structured and unstructured formats to yield new insights in predictive and prescriptive models—ones that tell frontline workers how best to perform their jobs. The trucking company Schneider National, for example, is adding data from new sensors to its logis- tical optimization algorithms, allowing it to monitor key indicators such as fuel levels, container location and capacity, and driver behavior. It aims to steadily improve the efficiency of its route networks, lower its fuel costs, and decrease the risk of accidents.
A new set of data management options. In the 1.0 era, firms used data warehouses as the basis for analysis. In the 2.0 era, they focused on Hadoop clusters and NoSQL databases. Today the technol- ogy answer is “all of the above”: data warehouses, database and big data appliances, environments that combine traditional data query approaches with Ha- doop (these are sometimes called Hadoop 2.0), verti- cal and graph databases, and more. The number and complexity of choices IT architects must make about data management have expanded considerably, and
almost every organization will end up with a hybrid data environment. The old formats haven’t gone away, but new processes are needed to move data and analysis across staging, evaluation, exploration, and production applications.
Faster technologies and methods of analysis. Big data technologies from the 2.0 period are con- siderably faster than previous generations of tech- nology for data management and analysis were. To complement them, new “agile” analytical methods and machine-learning techniques are being used to produce insights at a much faster rate. Like agile sys- tems development, these methods involve frequent delivery of partial outputs to the project stakehold- ers; as with the best data scientists’ work, they have an ongoing sense of urgency. The challenge in the 3.0 era is to adapt operational, product development, and decision processes to take advantage of what the new technologies and methods can bring forth.
Embedded analytics. Consistent with the increased speed of data processing and analysis, models in Analytics 3.0 are often embedded into operational and decision processes, dramatically increasing their speed and impact. For example, Procter & Gamble is integrating analytics in day-to- day management decision making through more than 50 “business sphere” decision rooms and more than 50,000 “decision cockpits” on employee computers.
Some firms are embedding analytics into fully automated systems through scoring algorithms and analytics- based rules. Some are building analyt- ics into consumer-oriented products and features. Whatever the scenario, integrating analytics into systems and processes not only means greater speed but also makes it harder for decision makers to avoid using analytics—which is usually a good thing.
Data discovery. To develop products and ser- vices on the basis of data, companies need a capable discovery platform for data exploration along with the requisite skills and processes. Although enter- prise data warehouses were initially intended to
Google, Amazon, and others have prospered not by giving customers information but by giving them shortcuts to decisions and actions.
hbr.org
December 2013 harvard business review 69
AnAlyTiCs 3.0
facilitate exploration and analysis, they have be- come production data repositories for many organi- zations, and, as previously noted, getting data into them is time-consuming. Data discovery environ- ments make it possible to determine the essential features of a data set without a lot of preparation.
Cross-disciplinary data teams. In online firms and big data start-ups, data scientists are often able to run the whole show (or at least to have a lot of independence). In larger and more conventional firms, however, they must collaborate with a variety of other players to ensure that big data is matched by big analytics. In many cases the “data scientists” in such firms are actually conventional quantitative an- alysts who are forced to spend a bit more time than they’d like on data management activities (hardly a new phenomenon). Companies now employ data hackers, who excel at extracting and structuring in- formation, to work with analysts, who excel at mod- eling it.
Both groups have to work with IT, which supplies the big data and the analytical infrastructure, provi- sions the “sandboxes” in which the groups explore the data, and turns exploratory analysis into produc- tion capabilities. The combined team takes on what- ever is needed to get the analytical job done, with frequent overlap among roles.
Chief analytics officers. When analytics are this important, they need senior management over- sight. Companies are beginning to create “chief ana- lytics officer” roles to superintend the building and use of analytical capabilities. Organizations with C-level analytics leaders include AIG, FICO, USAA, the University of Pittsburgh Medical Center, the Obama reelection campaign, Wells Fargo, and Bank of America. The list will undoubtedly grow.
Prescriptive analytics. There have always been three types of analytics: descriptive, which reports on the past; predictive, which uses models based on past data to predict the future; and pre- scriptive, which uses models to specify optimal be- haviors and actions. Although Analytics 3.0 includes
all three types, it emphasizes the last. Prescriptive models involve large-scale testing and optimiza- tion and are a means of embedding analytics into key processes and employee behaviors. They pro- vide a high level of operational benefits but require high-quality planning and execution in return. For example, if the UPS ORION system gives incorrect routing information to drivers, it won’t be around for long. UPS executives say they have spent much more time on change management issues than on algorithm and systems development.
Analytics on an industrial scale. For compa- nies that use analytics mainly for internal decision processes, Analytics 3.0 provides an opportunity to scale those processes to industrial strength. Creat- ing many more models through machine learning can let an organization become much more granular and precise in its predictions. IBM, for instance, for- merly used 150 models in its annual “demand gen- eration” process, which assesses which customer accounts are worth greater investments of salesper- son time and energy. Working with a small company, Modern Analytics, and using a “model factory” and
“data assembly line” approach, IBM now creates and maintains 5,000 such models a year—and needs just four people to do so. Its new systems can build 95% of its models without any human intervention, and another 3% require only minimal tuning from an analyst. And the new models address highly specific products, customer segments, and geographies. A test conducted in one large Asian market showed that such models doubled customer response rates compared with nonstatistical segmentation approaches.
New ways of deciding and managing. In order for analytics to power the data economy in your company, you’ll need new approaches to de- cision making and management. Many will give you greater certainty before taking action. Manag- ers need to become comfortable with data-driven experimentation. They should demand that any important initiative be preceded by small-scale but
Imagine if Ron Johnson’s tenure at J.C. Penney had involved small-scale, data-driven experiments rather than wholesale changes.
70 Harvard Business Review December 2013
HBR.oRgSpotlight on Making YouR CoMpanY Data-FRienDlY
systematic experimentation of this sort, with rigor- ous controls to permit the determination of cause and effect. Imagine, for example, if Ron Johnson’s tenure as CEO of J.C. Penney had involved limited experiments rather than wholesale changes, most of which turned out badly.
Paradoxically, some of the changes prompted by the widespread availability of big data will not yield much certainty. Big data flows continuously—con- sider the analysis of brand sentiment derived from social media sources—and so metrics will inevita- bly rise and fall over time. Such “digital smoke sig- nals,” as they have been called, can serve as an early warning system for budding problems. But they are indicative, not confirmatory. Managers will have to establish guidelines for when early warnings should cue decisions and action.
Additional uncertainty arises from the nature of big data relationships. Unless they are derived from formal testing, the results from big data generally in- volve correlation, not causation, and sometimes they occur by chance (although having greater amounts of data increases the likelihood that weak results will be statistically significant). Some managers may be frustrated by these facts. If the issue under consider- ation is highly important, further investigation may be warranted before a decision is made.
The use of prescriptive analytics often requires changes in the way frontline workers are managed. Companies will gain unprecedented visibility into the activities of truck drivers, airline pilots, ware- house workers, and any other employees wearing or carrying sensors (perhaps this means all employees, if smartphone sensors are included). Workers will undoubtedly be sensitive to this monitoring. Just as analytics that are intensely revealing of customer be- havior have a certain “creepiness” factor, overly de- tailed reports of employee activity can cause discom- fort. In the world of Analytics 3.0, there are times we need to look away.
Creating Value in the Data Economy Does Analytics 3.0 represent the ultimate form of competing on analytics? Perhaps not. But it seems safe to say that it will be viewed as the point in time when participation in the data economy went mainstream.
The online companies that unleashed big data on the world were built around it from the begin- ning. They didn’t need to reconcile or integrate big data with traditional sources of information and
the analytics performed on it, because for the most part, they didn’t have those traditional sources. They didn’t need to merge big data technologies with tra- ditional IT infrastructures; in their companies, those infrastructures didn’t exist. Big data could stand alone, big data analytics could be the only analytics, and big data technology architectures could be the only IT architectures. But each of these companies now has its own version of Analytics 3.0.
One thing is clear: The new capabilities required of both long-established and start-up firms can’t be developed using old models for how analytics sup- ported the business. The big data model was a huge step forward, but it will not provide advantage for much longer. Companies that want to prosper in the new data economy must once again fundamentally rethink how the analysis of data can create value for themselves and their customers. Analytics 3.0 is the direction of change and the new model for compet- ing on analytics. HBR Reprint R1312C
Thomas H. Davenport is the President’s Distinguished Professor of IT and Management at Babson College,
a fellow of the MIT Center for Digital Business, a senior adviser to Deloitte Analytics, and a cofounder of the Inter national Institute for Analytics (for which the ideas in this article were generated). He is a coauthor of Keeping Up with the Quants (Harvard Business Review Press, 2013) and the author of Big Data at Work (forthcoming from Harvard Business Review Press).
CA RT
o o
n : K
en K
RI M
sT eI
n
“Printergatherer.”
72 Harvard Business Review December 2013
HBR.oRgSpoTligHT on MAKIng YouR CoMPAnY DATA-FRIenDlY
Harvard Business Review Notice of Use Restrictions, May 2009
Harvard Business Review and Harvard Business Publishing Newsletter content on EBSCOhost is licensed for the private individual use of authorized EBSCOhost users. It is no
Collepals.com Plagiarism Free Papers
Are you looking for custom essay writing service or even dissertation writing services? Just request for our write my paper service, and we'll match you with the best essay writer in your subject! With an exceptional team of professional academic experts in a wide range of subjects, we can guarantee you an unrivaled quality of custom-written papers.
Get ZERO PLAGIARISM, HUMAN WRITTEN ESSAYS
Why Hire Collepals.com writers to do your paper?
Quality- We are experienced and have access to ample research materials.
We write plagiarism Free Content
Confidential- We never share or sell your personal information to third parties.
Support-Chat with us today! We are always waiting to answer all your questions.
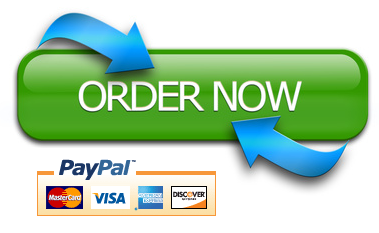