Read the following attached articles: Bending the Cost Curve: Time Se
Read the following attached articles:
Bending the Cost Curve: Time Series Analysis of a Value Transformation Programme at an Academic Medical Centre.
Geographic Variation in the Delivery of High-Value Inpatient Care.
A New Instrument to Measure High Value, Cost-Conscious Care Attitudes Among Healthcare Stakeholders: Development of the MHAQ.
Reducing Healthcare Costs Through Patient Targeting: Risk Adjustment Modeling to Predict Patients Remaining High-Cost.
Watch the following video:
What If All US Health Care Costs Were Transparent? | Jeanne Pinder (https://www.youtube.com/watch?v=ZjeZ8r7yWOk)
Researchers have documented systematic variation in Medicare spending and health services utilization across various geographic regions for decades. The findings also evidence that high Medicare spending is unrelated to health outcomes. Policymakers have changed Medicare payment systems to promote high-value care. As key stakeholders in the health care delivery system, health care organizations and providers play an important role in health care spending.
You are taking on the role of a new CEO of a hospital that was identified as having high health care cost and utilization. In the first meeting with the board, you are tasked to reduce health care spending and utilization in your hospital. Your board provides the main factor influencing health care spending and utilization while maintaining quality of care.
*Diversity within patient populations, patients’ current state of health, access to care, insurance coverage, and patients’ preferences for their care.
As the new CEO, in 300 to 400 words present the strategic plan you would implement to the board on this critical determinant. Be sure to also briefly explain why Physicians’ decisions on what care to give and the availability of reliable medical evidence to guide those decisions is also another important factor in influencing health care spending and utilization while maintaining quality of care.
Support your response with a minimum of two scholarly sources that were published in the last 5 years. Be sure to cite your references using proper APA Style
-
Bendingthecostcurve_timeseriesanalysisofavaluetransformationprogrammeatanacademicmedicalcentre.pdf
-
Geographicvariationinthedeliveryofhigh-valueinpatientcare.pdf
-
ANewInstrumenttoMeasureHighValue.pdf
-
ReducingHealthcareCostsThroughPatientTargeting_RiskAdjustmentModelingtoPredictPatientsRemainingHigh-Cost.pdf
Chatfield SC, et al. BMJ Qual Saf 2019;28:449–458. doi:10.1136/bmjqs-2018-009068 449
Original research
► Additional material is published online only. To view please visit the journal online (http:// dx. doi. org/ 10. 1136/ bmjqs- 2018- 009068).
For numbered affiliations see end of article.
Correspondence to Dr Leora I Horwitz, Department of Population Health, NYU School of Medicine, New York, NY 10016, USA; leora. [email protected] nyulangone. org
SCC and FMV contributed equally.
Received 6 November 2018 Revised 1 February 2019 Accepted 6 February 2019 Published Online First 15 March 2019
To cite: Chatfield SC, Volpicelli FM, Adler NM, et al. BMJ Qual Saf 2019;28:449–458.
► http:// dx. doi. org/ 10. 1136/ bmjqs- 2019- 009427
Bending the cost curve: time series analysis of a value transformation programme at an academic medical centre
steven c chatfield,1 Frank M Volpicelli,2 nicole M adler,2 Kunhee lucy Kim,3,4 simon a Jones,3,4 Fritz Francois,1,5 Paresh c shah,1,6 robert a Press,1,7 leora i horwitz 2,3,4
© Author(s) (or their employer(s)) 2019. Re-use permitted under CC BY-NC. No commercial re-use. See rights and permissions. Published by BMJ.
AbstrAct Background Reducing costs while increasing or maintaining quality is crucial to delivering high value care. Objective To assess the impact of a hospital value- based management programme on cost and quality. Design Time series analysis of non-psychiatric, non- rehabilitation, non-newborn patients discharged between 1 September 2011 and 31 December 2017 from a US urban, academic medical centre. Intervention NYU Langone Health instituted an institution-wide programme in April 2014 to increase value of healthcare, defined as health outcomes achieved per dollar spent. Key features included joint clinical and operational leadership; granular and transparent cost accounting; dedicated project support staff; information technology support; and a departmental shared savings programme. Measurements Change in variable direct costs; secondary outcomes included changes in length of stay, readmission and in-hospital mortality. Results The programme chartered 74 projects targeting opportunities in supply chain management (eg, surgical trays), operational efficiency (eg, discharge optimisation), care of outlier patients (eg, those at end of life) and resource utilisation (eg, blood management). The study cohort included 160 434 hospitalisations. Adjusted variable costs decreased 7.7% over the study period. Admissions with medical diagnosis related groups (DRG) declined an average 0.20% per month relative to baseline. Admissions with surgical DRGs had an early increase in costs of 2.7% followed by 0.37% decrease in costs per month. Mean expense per hospitalisation improved from 13% above median for teaching hospitals to 2% above median. Length of stay decreased by 0.25% per month relative to prior trends (95% CI −0.34 to 0.17): approximately half a day by the end of the study period. There were no significant changes in 30-day same-hospital readmission or in-hospital mortality. Estimated institutional savings after intervention costs were approximately $53.9 million. Limitations Observational analysis. Conclusion A systematic programme to increase healthcare value by lowering the cost of care without compromising quality is achievable and sustainable over several years.
IntroductIon Healthcare spending in the USA has increased from 4.4% of the gross domestic product in 1950 to nearly 18% in 2016, reaching $3.3 trillion.1 Per-capita health- care spending is higher than any other industrialised nation,2 but healthcare quality ranks last.3
In response, focus on value in the US healthcare system, defined as health outcomes achieved per dollar spent, has been intensifying.4 In 2015, the Centers for Medicare & Medicaid Services set a goal to have 90% of healthcare reim- bursement be value based by 2018, and commercial payers are following suit.5 6 Health systems are beginning to respond by publishing their approaches to value- based care.7–9
By the beginning of 2014 it had become apparent that our own health system, NYU Langone Health (NYULH), had substantial opportunity to improve value. From 2010 to 2013, our institutional losses on Medicare patients had more than doubled. In 2013, we had been ranked number 1 in quality and accountability by the University Healthcare Consortium (now Vizient)10; however, the American Association of Medical Colleges-Council of Teaching Hospitals (COTH) quarterly survey of hospital operations and finan- cial performance showed we were nearly at the 75th percentile for expense per discharge even after standardising for case mix index (CMI) and Wage Index.11 Analytics from Cleverly and Associates, an external consultant, indicated that our CMI-adjusted Medicare loss per case was the highest among all academic medical centres.12 It was in this environment that
450 Chatfield SC, et al. BMJ Qual Saf 2019;28:449–458. doi:10.1136/bmjqs-2018-009068
Original research
the Dean and Chief Executive Officer (CEO), a single role at NYULH, formed a Value-Based Management (VBM) Task Force to ensure that NYULH not only provided the highest quality but did so at an afford- able cost.
This paper describes the formation and evolution of the VBM programme and its impact on total variable direct cost per case and quality.
Methods study setting NYULH is an academic health system in New York, NY that included two inpatient hospitals (Tisch Hospital, 844 beds; NYU Langone Hospital – Brooklyn, 225 beds) when the VBM project began. Because Tisch Hospita- laccounted for 85% of total admissions and 80% of total inpatient variable direct costs, VBM projects in the first years focused on this hospital. Accordingly, this analysis is restricted to Tisch discharges.
VbM task Force The Dean of the NYU School of Medicine serves also as CEO of NYULH, enabling the institution to align academic, clinical and operational priorities. In November 2013, the Dean convened hospital leaders to recommend a road map to value improvement. The programme formally launched in April 2014 with the kick-off of the inaugural VBM Task Force. The Task Force was led by the chief medical officer (RAP), a senior clinician with over 30 years of clinical experience at NYULH. Directly reporting to the VBM Chair were a project lead with a Master's of Business Administration (SCC), and a clinical lead who was a practising hospitalist (FMV), who took joint respon- sibility for prioritisation and oversight of projects, and for initial meetings with stakeholders for new projects. Other members included all hospital-based vice deans and senior vice presidents. The Task Force was supported by 10 project managers, each of whom was assigned responsibility for two to three projects, in addition to their hospital-based responsibilities. VBM project managers had 3–5 years of experience at the institution, a master’s degree either in hospital administration or business administration, and worked within hospital operations, strategy, planning, business development or finance.
cost accounting The VBM team used variable direct cost data from- NYULH’s customised activity-based costing system (EPSi, Allscripts), which was implemented in September 2011, for cost accounting (see online supplementary appendix A for variable direct cost components). This customised system includes several unique features that facilitated identification of opportunities for improved value. First, it is based on actual costs (ie, acquisition costs of drugs and supplies) rather than the ratio of cost to charge, thus eliminating influence of charges
and insurance contracts. Second, it incorporates both inpatient physician and hospital billing so that collec- tive impact of clinical care could be assessed: for instance, the Task Force could assess whether certain types of consults or care by certain types of physi- cians affected outcomes. Third, it includes patient- level quality data such as expected mortality, readmis- sions and length of stay (LOS); and hospital-acquired conditions, facilitating a focus on value. For instance, the Task Force used embedded quality data to calcu- late additional costs of hospital-acquired conditions in considering value opportunities. The expected quality outcomes were obtained from the Vizient Clin- ical Data Base/Resource Manager (CDB/RM), which creates risk-adjustment models based on admissions from hundreds of academic medical centres. Fourth, it includes ‘expense groupings’ that match clinical intu- ition rather than administrative categories like payor or service line: blood, laboratory, pharmacy, radiology, implants and supplies, intensive care and operating room. Finally, additional fields were added as needed to support individual projects—for instance, a field for comanagement was added to track value of hospitalist comanagement of surgical patients.
opportunity identification The VBM Task Force focused on six main areas of potential opportunity: operational efficiency (as meas- ured by labour efficiency and patient throughput metrics); resource utilisation (as measured by vari- able direct cost/case); supply chain management (as measured by wasted materials, price and utilisation); revenue cycle (as measured by appropriate coding and documentation); outliers (as identified by highest cost patients); and corporate services (measured by admin- istrative and overhead costs). Over time, the Task Force identified areas of opportunity within each of these categories through analysis of those metrics, in-person feedback from clinicians, a web-based idea submission form and comparison to performance of seven hospi- tals identified by Vizient as being high quality and low cost (table 1).
Each area of opportunity was assessed by the project and clinical leads to prioritise high-potential proj- ects. For the first round of value projects, a sign-off from the Dean was required. High-potential projects were selected based on projected impact and ease of implementation.
Interventions Using a standardised process improvement model, a dedicated, time-limited project committee was created for each new project. Each committee was co-led by a clinical lead and a project manager. A formal project charter was developed in the first two meet- ings of each project and approved by the Task Force. The Task Force met monthly to review overall VBM metrics and hear reports of individual projects. Once
451Chatfield SC, et al. BMJ Qual Saf 2019;28:449–458. doi:10.1136/bmjqs-2018-009068
Original research
Table 1 Taxonomy of selected VBM projects
Domain Opportunity Project(s) Status
Supply chain management
Non-standardised OR supplies Preference card standardisation, rationalisation In progress Inconsistent naming taxonomy for surgical procedures Preference cards listed items as ‘open for procedure’ that were rarely used
Operational efficiency Delayed discharge because of waits for postdischarge beds
Revision of inpatient rehabilitation assessments; increased use of long-term acute care
In progress
Delays for weekend discharges Weekend discharge coordination staffing increase; warm handoff Friday rounds; weekend rounding initiatives
In progress
Intrainstitutional transfers delayed care Right place, right time bed management; ICU admission criteria; ICU transfer project
Complete
Prolonged dwell time in ICU, step-down and PACU
Prioritise transfers out of those units to facilitate high-cost bed availability
Complete
Wide variability in individual physician practice
Value opportunity tool: analytics to identify specific areas of cost/quality that are outliers per physician
In progress
Lack of postdischarge coordination Dedicated personnel assigned to medicine units to make postdischarge follow-up appointments consistent with patient preferences
Complete
Outliers Non-standardised care promoted development of outlier cases
Epic-based clinical pathways for heart failure, pneumonia and colorectal surgery, GI bleed, paper-based lap sleeve pathway
Complete
End-of-life patients account for disproportionate share of quality, cost, readmission and outliers
Supportive care initiative aimed at improving value of care delivered at end of life both in the outpatient and inpatient setting
In progress
Surgical patients with medical comorbidities had disproportionately length of stay outliers
Hospitalist comanagement programme on acute care surgery, general surgery, neurosurgery services
In progress
Resource utilisation Excessive duplication of studies that did not generate new findings
EHR best practice alert to reduce ordering of repeat echocardiograms within 2 months of last exam
Complete
Above benchmark use of intravenous medications
Pharmacists review dashboard of adult inpatients with enteral access receiving one of 18 target intravenous medications with equivalent oral bioavailability who met criteria for oral conversation
Complete
Corporate services cost disproportionately high
Corporate services challenge to generate ideas for cost reduction
Ongoing
Multidose medication vials were being wasted
Open drugs only as needed rather than in advance in cardiac catheterisation lab
Complete
Excessive use of reference lab testing Alert created in EHR to display turnaround time and cost for 42 reference labs that accounted for 80% of cost. Required attestation when ordering lab that the test was required for inpatient management. Created just-in-time learning for six reference labs to improve ordering practices. Created mechanisms for monthly reporting and real-time feedback.
Complete
Excessively high rate of blood transfusion benchmarked to peer institutions17
Packed red blood cell and platelet transfusion order set revised, clinical decision support applied in the EHR, hospital-wide educational campaign deployed
Complete
Excessive testing of serial troponins in postoperative patients
Created guidelines for obtaining postoperative troponin levels
Complete
EHR, electronic health record; GI, gastrointestinal; ICU, intensive care unit; PACU, postanaesthesia care unit; VBM, value-based management; delete, please delete this footnote .
initial interventions were developed, implemented and refined, continuing oversight was turned over to existing institutional committees.
In addition to implementing specific projects, the VBM Task Force made data widely available throughout the institution. In October 2014, an inter- active dashboard containing up-to-date and historical
data on inpatient Medicare activity (visible by depart- ment, attending physician, diagnosis related group (DRG) family and expense grouping) was rolled out to the Task Force and 1 month later to clinical chairs and their designees (online supplementary appendix B). In January 2015, CMI-adjusted variable direct cost per case was added to the dashboard (visible
452 Chatfield SC, et al. BMJ Qual Saf 2019;28:449–458. doi:10.1136/bmjqs-2018-009068
Original research
by department, physician and expense grouping), and in February 2015, data on the top quartile of Vizient performers were added (online supplementary appendix C). Filters could be applied in real time to any data display, with drill-down to individual patient records.
In the second year of the VBM initiative, the Task Force created a departmental incentive programme. On a semiannual basis, actual versus prior year costs were calculated for each clinical department, adjusted for CMI and healthcare inflation. Departments were given cost targets and quality thresholds, and chairs were held accountable to their goals in semiannual meetings with the Dean, during which VBM performance was specifically reviewed and requests for improvements made if necessary. Targets were adjusted dynami- cally as institutional priorities and objectives evolved. Departments with costs below expected received a fraction of the savings (between 10% and 40%, aver- aging 25%) depending on operational performance metrics such as observed/expected LOS and on quality metrics such as healthcare-associated conditions or patient experience. These funds could be used at chair discretion but were expected to be used to support departmental-specific value improvement initiatives, including supporting physician salary time for proj- ects. Costs for patients spanning multiple departments (such as surgical patients comanaged by hospitalists) were calculated separately and any savings versus expected costs were divided between departments to encourage cross-departmental collaboration (75% to the primary department and 25% to the secondary department). Patients were attributed to department based on discharging physician. Departments were encouraged to use shared savings funds to identify and implement new value-focused initiatives. Departments could view their expected costs and savings to date in real time on the VBM dashboard. The VBM Task Force tracked these projects and provided analytic and logistical support.
study cohort and data sources We defined our baseline period as the start of our new fiscal accounting system (September 2011) through launch of the VBM initiative (April 2014). However, Tisch Hospital was closed from 29 October 2012 to 27 December 2012 because of catastrophic damage to its emergency department, some radiology equip- ment and mechanical systems from Superstorm Sandy and underwent a phased reopening over the next few months, with most services including urgent care reopening 14 January 2013. The emergency depart- ment reopened in April 2014 at three times the predis- aster size. Since this disaster skewed cost data during fiscal year 2012 (September 2012 to August 2013), we excluded that period from analysis. Of note, the base- line period for readmission began in January 2012, because prior Vizient data were unavailable.
The postintervention period was May 2014 to December 2017.
Our cohort was all inpatient admissions to Tisch Hospital. We excluded admissions for rehabilitation and inpatient psychiatric treatment, which are not paid on a DRG basis, and excluded normal newborns as is standard in American Hospital Association and Centers for Medicare & Medicaid Services cost accounting. We also excluded cases with no DRG weight assigned or assigned to Medicare Severity Diagnosis Related Group (MS-DRG) 998 (invalid discharge diagnosis) or 999 (ungroupable diagnosis). We divided cases into medicine and surgery cohorts based on the CMS DRG groupings.
All data for statistical analysis were obtained from the cost accounting system, which incorporated the Vizient CDB/RM data on readmission. Data from COTH and from Cleverly and Associates were obtained from reports provided to the institution. Data for publicly reported Medicare measures were obtained from Hospital Compare.
outcomes Rather than attempt to individually quantify and sum the effect of each individual intervention, which may either double-count savings or undercount spillover effects, we assessed the global impact of the programme on total variable direct cost per case (online supple- mentary appendix C). A secondary outcome was LOS, as it was targeted by several of the interventions. We assessed two counterbalancing measures to identify potential unintended consequences: 30-day same-hos- pital readmission rate and in-hospital mortality rate. We also tracked summary statistics on cost, read- missions and mortality from COTH; Cleverly and Associates; and Medicare (via Hospital Compare) to qualitatively determine performance versus peers. Project-specific value metrics were designated for each chartered project but are not reported here.
Independent variables The primary exposure variable was intervention period. To account for potential changes in patient mix over time we included as covariates: age (as indi- cators for 5 years age bin up to 90 and an indicator for 91 and above); sex; race/ethnicity (white, black, other and unknown); insurance (Medicare fee-for-ser- vice (FFS), Medicare Advantage, Medicaid FFS, managed Medicaid, self-pay, commercial or other insurance types); surgical patient indicator, grouped using MS-DRG type; DRG weight (in deciles); Elix- hauser comorbidities; and seasonal indicators.13 Average DRG weight for all hospitalisations represents the CMI. We adjusted the cost outcomes using the seasonally adjusted consumer price index (CPI) for all urban consumers for medical care, and denominated to September 2011 dollars.
453Chatfield SC, et al. BMJ Qual Saf 2019;28:449–458. doi:10.1136/bmjqs-2018-009068
Original research
statistical analysis We used descriptive statistics to characterise preinter- vention and postintervention cohort demographics. Categorical variables were summarised as frequencies and proportions, and continuous variables as means and SDs. We examined differences in preintervention and postintervention cohorts using χ2 tests or t-tests, as appropriate. For illustrative purposes, we graphed percentage change from baseline in both unadjusted and adjusted costs (in September 2011 dollars), indi- cating when key interventions occurred.
For each cost outcome, we conducted two comple- mentary analyses to determine whether postinterven- tion costs differed from preintervention: statistical process control (SPC) charts and interrupted time series (ITS) analyses. First, we plotted the fully adjusted mean total variable direct costs per case for each month for all patients and for medical and surgical DRGs sepa- rately on SPC charts. We explored whether there was ‘special-cause variation’ in the postintervention period in DRG-weight adjusted costs: that is, whether any mean monthly costs were above or below the 3 SD (99.7%) control lines, or whether there was a run of at least eight consecutive observations above or below the mean.14 To maintain institutional confidentiality of internal cost structure, results are presented as monthly percentage changes from the baseline mean.
We then conducted ITS analyses for the main outcome and all secondary outcomes, setting the inter- ruption at 1 May 2014. This approach is superior to a simple pre-post analysis because it accounts for under- lying secular trends in the preintervention period. We conducted sequential ITS analyses, first without adjustment, then adjusted for all covariates but DRG weight (which could potentially be influenced by changes in coding over time), then fully adjusted. In addition to the covariates specified above, each ITS model included a linear monthly trend term for the preintervention period (‘Time’), an indicator variable for post-VBM months (‘Intervention’), which captures any level change in the outcome postintervention, and a linear monthly trend term only for the post-VBM months (‘Time After Intervention’), which captures any change in slope of the outcome postintervention relative to the preintervention trend.15 16 These three terms allowed us to investigate whether the level and slope of the outcome changed after intervention. We estimated generalised linear models (GLM) with the gamma family and log link for continuous outcomes; and GLMs with the binomial family and logit link for binary outcomes. Since this patient-level analysis includes a cross section of patients plausibly inde- pendently distributed across months, autocorrelation was not assumed.
To determine whether the programme had differen- tial effects by patient population, we then stratified the ITS total variable direct cost analysis by medical versus surgical DRGs.
We calculated estimated savings by subtracting the observed cost from predicted cost for each case and summing the difference across cases in the interven- tion period. We generated predicted costs by esti- mating a fully adjusted model including ITS model parameters for just the preintervention period and then fitting intervention period observations to the model. We calculated estimated costs of the interven- tion by summing the project management personnel costs, including fringe.
Data management and analysis were performed using R 2017 (R Core Team, Vienna, Austria) and Stata V.13 (StataCorp, College Station, TX). The project used only non-identified data and was considered by the NYU School of Medicine Institutional Review Board not to be human subjects research; therefore informed consent for data access was not required.
results During the study period, the VBM Task Force char- tered 62 projects (selected projects are shown in table 1): 36 projects were completed and are now in maintenance phase; another 26 remain in progress. An additional 12 department-led initiatives were conducted. Figure 1 shows the start dates of some of the larger projects.
The total study cohort included 160 434 hospi- talisations (47 404 preintervention and 113 030 postintervention). We excluded 11 716 rehabilita- tion admissions, 4511 psychiatric admissions, 21 451 normal newborn admissions and 4043 admissions that had MS-DRG of missing, 998 or 999. Characteristics of the preintervention and postintervention cohorts are shown in table 2. In the postintervention period, mean DRG weight was higher (1.86 post vs 1.72 pre, p<0.001), more patients were on the surgery service (11.0% vs 10.2%, p<0.001), fewer patients were on the medicine service (29.7% vs 32.4%, p<0.001) and substantially more patients had Medicaid insurance (15% vs 10.5%, p<0.001).
The SPC chart shows 29 consecutive months in the postintervention period below the preintervention mean, and 21 months that were below the lower 3 SD control line, strongly signalling the presence of special cause variation (figure 2A). This pattern was retained for both medicine patients (34 consecutive months below mean; 19 months below lower control line; figure 2B) and surgery patients (21 consecutive months below mean; 3 below lower control line; figure 2C). In addition, the surgery cohort had 12 consecutive months above the preintervention mean in the early intervention period (3 outside the upper control line).
In the ITS analysis, fully adjusted total variable direct costs per case declined an average of 0.22% per month relative to baseline trends (95% CI −0.31 to −0.13), which was partially offset by a non-significant early increase of 1.95% (95% CI −0.12 to 4.06) in costs.
454 Chatfield SC, et al. BMJ Qual Saf 2019;28:449–458. doi:10.1136/bmjqs-2018-009068
Original research
Figure 1 Percentage change in total variable direct costs relative to the preintervention period mean. Costs adjusted for DRG weight; all costs are denominated in September 2011 dollars.
Table 2 Characteristics of study cohort
Characteristic Preintervention n (%)
Postintervention n (%) P value
Overall 47 404 (100.0) 113 030 (100.0) Male 19 586 (41.3) 47 154 (41.7) 0.14 Age, mean (SD) 47 (27) 46 (27) <0.001 Race/ethnicity White 31 393 (66.2) 73 382 (64.9) <0.001 Black 4345 (9.2) 11 013 (9.7) <0.001 Other race 10 275 (21.7) 25 323 (22.4) 0.001 Unknown 1391 (2.9) 3312 (2.9) 0.96 DRG weight, mean (SD) 1.72 (1.7) 1.86 (1.8) <0.001 Elixhauser variables, mean (SD) 0.07 (0.25) 0.07 (0.25) 0.79 Service line Medicine (general and specialty) 15 360 (32.4) 33 610 (29.7) <0.001 Surgery (general and specialty) 4818 (10.2) 12 488 (11.0) <0.001 Neurology 3661 (7.7) 8132 (7.2) <0.001 Obstetrics/gynaecology 9464 (20.0) 23 131 (20.5) 0.02 Paediatrics (general and specialty) 5201 (11.0) 14 850 (13.1) <0.001 Other service 8900 (18.8) 20 819 (18.4) 0.10 Payer Medicare 16 240 (34.3) 36 834 (32.6) <0.001 Medicaid 4968 (10.5) 16 990 (15.0) <0.001 Self-pay 395 (0.8) 997 (0.9) 0.33 Commercial/worker’s compensation/other 25 801 (54.4) 56 437 (49.9) <0.001 DRG, diagnosis related group; FFS, fee-for-service.
This resulted in a net overall reduction in costs of 7.7% by the end of the study period relative to the expected cost without intervention (table 3). The medical cohort declined an average 0.20% per month (95% CI −0.33 to −0.08), while the surgical cohort declined 0.37% per month (95% CI −0.48 to −0.26). In dollar terms,
these savings were equivalent to approximately $59.3 million overall ($10.3 million for medicine and $49.0 million for surgery patients). Intervention costs were approximately $1.5 million per year ($5.375 million in the study period), making net savings approximately $53.9 million.
455Chatfield SC, et al. BMJ Qual Saf 2019;28:449–458. doi:10.1136/bmjqs-2018-009068
Original research
Figure 2 Statistical process control charts of adjusted total direct variable cost per discharge. (A) *Percentage change in mean adjusted total direct variable cost per discharge relative to the preintervention mean, all patients. (B) *Percentage change in mean adjusted total direct variable cost per discharge relative to the preintervention mean, medical DRGs. (C) *Percentage change in mean adjusted total direct variable cost per discharge relative to the preintervention mean, surgical DRGs. The upper and lower bounds denote the 3 SDs from t
Collepals.com Plagiarism Free Papers
Are you looking for custom essay writing service or even dissertation writing services? Just request for our write my paper service, and we'll match you with the best essay writer in your subject! With an exceptional team of professional academic experts in a wide range of subjects, we can guarantee you an unrivaled quality of custom-written papers.
Get ZERO PLAGIARISM, HUMAN WRITTEN ESSAYS
Why Hire Collepals.com writers to do your paper?
Quality- We are experienced and have access to ample research materials.
We write plagiarism Free Content
Confidential- We never share or sell your personal information to third parties.
Support-Chat with us today! We are always waiting to answer all your questions.
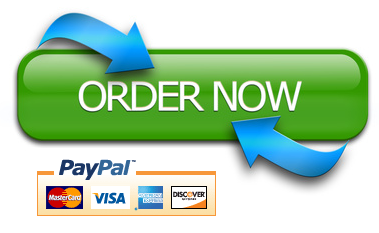