7 pages not including cover page and literature cited page Format: – 12 pt. Times New Roman font, double-spaced, 1? margins with
7 pages not including cover page and literature cited page
Format: – 12 pt. Times New Roman font, double-spaced, 1” margins with proper grammar & spelling
Literature Cited: -Single format for bibliography & in-text citations using correct information with at least 4 references total including the two primary articles chosen
Content
· No quotes or paraphrasing
· Introduction: – Explained importance of topic and sufficient background to tie together articles
· Article 1 Summary: – Briefly explained methods, results, conclusion
· Article 2 Summary: – Briefly explained methods, results, conclusions
· Conclusion: – Tied together articles and suggested future directions for research in the topic
RESEARCH ARTICLE
A resource for improved predictions of
Trypanosoma and Leishmania protein three-
dimensional structure
Richard John WheelerID*
Peter Medawar Building for Pathogen Research, University of Oxford, Oxford, United Kingdom
Abstract
AlphaFold2 and RoseTTAfold represent a transformative advance for predicting protein
structure. They are able to make very high-quality predictions given a high-quality alignment
of the protein sequence with related proteins. These predictions are now readily available
via the AlphaFold database of predicted structures and AlphaFold or RoseTTAfold Cola-
boratory notebooks for custom predictions. However, predictions for some species tend to
be lower confidence than model organisms. Problematic species include Trypanosoma
cruzi and Leishmania infantum: important unicellular eukaryotic human parasites in an
early-branching eukaryotic lineage. The cause appears to be due to poor sampling of this
branch of life (Discoba) in the protein sequences databases used for the AlphaFold data-
base and ColabFold. Here, by comprehensively gathering openly available protein
sequence data for Discoba species, significant improvements to AlphaFold2 protein struc-
ture prediction over the AlphaFold database and ColabFold are demonstrated. This is made
available as an easy-to-use tool for the parasitology community in the form of Colaboratory
notebooks for generating multiple sequence alignments and AlphaFold2 predictions of pro-
tein structure for Trypanosoma, Leishmania and related species.
Introduction
Machine learning approaches to protein structure prediction have crossed a critical success
threshold. While predicting the three-dimensional structure of a protein from sequence alone
is still unsolved problem, a multiple sequence alignment (MSA) of the target protein sequence
with related proteins provides key additional information. Cutting edge approaches using such
MSAs now have the potential to reach very high accuracy. MSAs are the input for AlphaFold2
[1] and RoseTTAfold [2], with AlphaFold2 reaching the highest accuracy prediction at the
most recent Critical Assessment of protein Structure Prediction (CASP) competition (CASP14
[3])–an accuracy comparable to experimental protein structure determination. AlphaFold2-
predicted structures for the near-whole proteome of 21 species [4] has been made publicly
available, and tools like ColabFold [5] and the official AlphaFold Colaboratory notebook [6]
make custom predictions easily accessible.
PLOS ONE
PLOS ONE | https://doi.org/10.1371/journal.pone.0259871 November 11, 2021 1 / 12
a1111111111
a1111111111
a1111111111
a1111111111
a1111111111
OPEN ACCESS
Citation: Wheeler RJ (2021) A resource for
improved predictions of Trypanosoma and
Leishmania protein three-dimensional structure.
PLoS ONE 16(11): e0259871. https://doi.org/
10.1371/journal.pone.0259871
Editor: Vyacheslav Yurchenko, University of
Ostrava, CZECH REPUBLIC
Received: September 9, 2021
Accepted: October 27, 2021
Published: November 11, 2021
Copyright: © 2021 Richard John Wheeler. This is an open access article distributed under the terms
of the Creative Commons Attribution License,
which permits unrestricted use, distribution, and
reproduction in any medium, provided the original
author and source are credited.
Data Availability Statement: The protein sequence
database is available from Zenodo (record
5563074, doi:10.5281/zenodo.5563074).
Funding: RJW 211075/Z/18/Z Wellcome Trust
https://wellcome.org/ The funders had no role in
study design, data collection and analysis, decision
to publish, or preparation of the manuscript.
Competing interests: The authors have declared
that no competing interests exist.
Trypanosomatids pose a challenge because of their large evolutionary distance from com-
mon model eukaryotes [7, 8]. This order includes important unicellular human, animal and
plant parasites, including the human infective Trypanosoma cruzi, Trypanosoma brucei and many human-infective Leishmania species. T. cruzi and Leishmania infantum are the most deadly of these species and were included in the initial 21 AlphaFold whole proteome predic-
tions [1, 4]. Trypanosomatids are members of an early-branching eukaryote linage (Discoba)
which also includes the less common, but still deadly, pathogen Naegleria fowleri. Other spe- ciose Discoba lineages are Euglena and Diplonema, unicellular aquatic organisms and impor-
tant and abundant auto- and heterotrophic plankton respectively. An initial inspection of the
AlphaFold database (alphafold.ebi.ac.uk) suggested protein structure prediction accuracy for
T. cruzi and L. infantum is often low–particularly for kinetoplastid specific proteins–based on self-reported prediction quality scores. Many of these proteins are vital, like the unconven-
tional kinetochore proteins [9].
Discoba diversity is less well sampled by genomes and transcriptomes than lineages like
plants or metazoa, making construction of deep MSAs more difficult. This is important as
MSAs encode additional structural information beyond the primary protein sequence alone:
They capture evidence for co-evolution of different regions of the primary sequence which
may correspond to proximity or interaction in the three-dimensional structure. AlphaFold2
and RoseTTAFold prediction of protein structure is greatly improved by high MSA quality
and depth, with high MSA coverage critical for high confidence predictions [1, 2]. While new
approaches [10] are trying to move beyond multiple sequence alignments, MSAs will remain a
powerful source of information.
Currently, the input databases for the AlphaFold database and the ColabFold notebook are
of UniRef [11] plus environmental sample sequence databases (BFD, Uniclust and MGNify
[12–14]). However, these databases have relatively poor coverage of Discoba. It appears that a
significant quantity of genomic and transcriptomic data available in the community genome
resource TriTrypDB [15, 16], the NCBI genome [17], transcriptome shotgun assembly (TSA)
[18] and sequencing read archive (SRA) [19] databases are not incorporated. It seemed likely
that an improved database is a simple opportunity to improve protein MSAs for protein struc-
ture predictions for Trypanosoma, Leishmania and other Discoba species. Here, protein sequence data was gathered into a comprehensive Discoba database and the
ColabFold MMSeqs2-based pipeline [5, 20] was modified to also include the result of a
HMMER search of Discoba. Using a test set of 30 L. infantum proteins, MSA coverage was always improved, leading to increased AlphaFold2 prediction accuracy in 2/3 of cases.
Improvements were greatest for kinetoplastids-specific proteins, with dramatic improvements
often possible. The necessary tools to make similar protein structure predictions have been
made openly available: The Discoba protein sequence database (for custom searches and MSA
generation), Colaboratory notebooks for generating MSAs by HMMER or MMSeqs2 (for use
in AlphaFold2 or RoseTTAFold implementations), and a standalone Colaboratory notebook
for AlphaFold2 structure predictions based on ColabFold incorporating a search of the Dis-
coba database. These tools are available at github.com/zephyris/discoba_alphafold.
Methods
Discoba sequence data
Predicted protein sequences were gathered from 243 Discoba transcriptomes or genomes (S1
Table): 160 transcriptomes and 83 genomes. 152 from cultured populations (almost all axenic)
and 91 from single cell samples. 238 were assembled giving a good number of predicted
PLOS ONE Improving trypanosomatid protein structure predictions
PLOS ONE | https://doi.org/10.1371/journal.pone.0259871 November 11, 2021 2 / 12
protein sequences (>500). The full set of sequences have been deposited as a Zenodo dataset
(version 1.0.0) [21].
Predicted proteins from genome sequencing were gathered from two sources: TriTrypDB:
All 53 trypanosomatid species available in TriTrypDB [15,16] release 53, using the provided
predicted protein sequences on TriTrypDB where available. For the 17 without predicted pro-
tein sequences the translation of all predicted open reading frames (ORFs) over 100 amino
acids were used, as kinetoplastids typically have compact genomes with short intergenic
sequences and extremely low occurrence of introns [22]. NCBI Genomes: 32 genomes for Dis-
coba species. For the 14 with predicted protein sequences on NCBI the existing prediction was
used. For the 18 without predictions, the translation of all ORFs over 100 amino acids were
used.
Sequencing read archive (SRA): 17 whole genome sequencing (WG-seq) datasets from axe-
nic cultures of Discoba species and 32 single cell WG-seq datasets. For each, assembly was car-
ried out using Velvet [23, 24] (see Genome assembly) and all predicted ORFs over 100 amino
acids were used.
Predicted proteins from transcriptome sequencing were gathered from three sources: Tran-
scriptome shotgun assembly (TSA) database: 11 transcriptomes for Discoba species, using pro-
tein sequence predicted by TransDecoder [25]. Marine Microbial Eukaryotic Transcriptome
Sequencing Project (MMETSP): 2 transcriptomes for Discoba species, using the provided pro-
tein sequences which were predicted using TransDecoder. NCBI SRA: 19 mRNA-seq datasets
from axenic cultures of Discoba species, 2 mRNA-seq datasets from mixed cultures including
a Discoba species and 59 single cell mRNA-seq datasets. For each, transcriptome assembly was
carried out using Trinity [26–28] followed by protein sequence prediction with TransDecoder
[25] (see Transcriptome assembly).
Transcriptome assembly
Transcriptome assembly from RNA-seq data used a standardised pipeline, with the same
approach used for axenic culture, mixed culture and single cell transcriptomic data. Reads
were first error corrected using Rcorrector v1.0.4 [29, 30] (using Jellyfish v2.3.0 [31, 32]) and
corrected reads tidied using TranscriptomeAssemblyTools [33]. Any remaining adaptor
sequences were trimmed using TrimGalore v0.6.0 [34] (using Cutadapt v2.8 [35, 36]) then an
assembly was generated using Trinity v2.12.0 [26–28]. Many of these species use polycistronic
transcription with a single spliced leader sequence trans-spliced onto the start of all mRNAs.
As such common sequences may affect assembly, a two-step approach was used. First, a trial
assembly using 1,000,000 reads (or all reads if fewer were available) was generated and the
common spliced leader sequence identified using a custom script. Cutadapt was then used to
trim reads to remove the spliced leader, then a final assembly was generated using 40,000,000
reads (or all reads if fewer were available). Very similar transcript sequences were removed
using cd-hit-est v4.8.1 (part of CD-HIT [37, 38]) then remaining sequences translated to pre-
dicted proteins using TransDecoder v5.5.0 [25] LongOrfs.
Genome assembly
Genome assembly from WG-seq data also used a standardised pipeline. For single cell geno-
mic data, reads were first error corrected using Rcorrector v1.0.4 [29, 30] (using Jellyfish v2.3.0
[31, 32]) and TranscriptomeAssemblyTools [33]. For all assemblies, any remaining adaptor
sequences were trimmed using TrimGalore v0.6.0 [34] (using Cutadapt v2.8 [35, 36]) then an
assembly was generated using Velvet v1.2.10 [23, 24] using all available reads. As expected cov-
erage and insert size are not necessarily known, a refinement step was used. Reads were aligned
PLOS ONE Improving trypanosomatid protein structure predictions
PLOS ONE | https://doi.org/10.1371/journal.pone.0259871 November 11, 2021 3 / 12
to the assembly using bwa mem v0.7.17 [39, 40] and insert size and mean coverage determined
using samtools v1.10 [41, 42], then a final assembly was generated using Velvet including these
parameters and a minimum coverage threshold of 0.25 the mean trial assembly coverage. All
open reading frames �300 bp (all three frames, both strands) were identified using a custom
script.
Orthology
Protein orthogroups were identified using OrthoFinder v2.5.4 [43–45] (using diamond
v2.0.5.143 [46, 47] and FastME 2.1.4 [48]). Reciprocal best protein sequence search hits were
carried out using diamond v2.0.5.143 [46, 47] with no e-value cut-off. OrthoFinder and recip-
rocal best sequence search hit analysis were carried out on a diverse set of 77 UniProt reference
eukaryote proteomes [49]: UP000001450, UP000002729, UP000007800, UP000012073,
UP000054560, UP000000437, UP000001542, UP000005203, UP000008144, UP000013827,
UP000059680, UP000000539, UP000001548, UP000005226, UP000008153, UP000014760,
UP000179807, UP000000559, UP000001593, UP000005640, UP000008493, UP000018208,
UP000186817, UP000000560, UP000001926, UP000006548, UP000008524, UP000023152,
UP000218209, UP000000561, UP000001940, UP000006671, UP000008743, UP000027080,
UP000247409, UP000000589, UP000001950, UP000006727, UP000008827, UP000030693,
UP000265515, UP000000600, UP000002195, UP000006729, UP000009022, UP000030746,
UP000265618, UP000000759, UP000002296, UP000006906, UP000009138, UP000036983,
UP000316726, UP000000803, UP000002311, UP000007110, UP000009168, UP000037460,
UP000323011, UP000000819, UP000002485, UP000007241, UP000009170, UP000051952,
UP000324585, UP000001357, UP000002494, UP000007305, UP000009377, UP000054408,
UP000444721, UP000001449, UP000002640, UP000007799, UP000011083, UP000054558.
This includes 6 kinetoplastid species (Bodo saltans, Leishmania infantum, Leishmania mexi- cana, Perkinsela sp., Trypanosoma brucei brucei and Trypanosoma cruzi), which were used as the basis for identifying kinetoplastid specific proteins.
Intrinsically disordered domains
Intrinsically disordered domains were predicted using IUPred2A [50] using a score threshold
of 0.5 for classification of a residue as disordered.
AlphaFold2 predictions
Existing AlphaFold predictions of protein structures for Leishmania infantum (UP000008153) Trypanosoma cruzi (UP000002296) and Mus musculus (UP000000589) were taken from alphafold.ebi.ac.uk [1], last updated using AlphaFold v2.0 2021-07-01. Per residue and global
predicted local distance difference test score (pLDDT) was taken from the mmCIF file, pre-
dicted average error (pAE) from the error json file.
ColabFold predictions were made using an unmodified version of ColabFold [5, 20], with
the default MSA pipeline, a MMseqs2 [51] search of UniRef [11] and environmental sample
sequence databases [12–14]. Predictions were done using AlphaFold2 parameters from 2021-
07-14, not using Amber [52] relaxation and not using PDB [53] templates. Due to GPU mem-
ory availability in Google Colaboratory, predictions were restricted to proteins with �800
amino acids.
AlphaFold2 predictions incorporating the new Discoba protein sequence database were
carried out using a modified version of ColabFold [5, 20]. The MMseqs2 [51] search of UniRef
[11] and environmental sample sequence databases [12–14], was supplemented with a
HMMER (part of HH-suite) [54] search of the Discoba protein database described here. A
PLOS ONE Improving trypanosomatid protein structure predictions
PLOS ONE | https://doi.org/10.1371/journal.pone.0259871 November 11, 2021 4 / 12
MMSeqs2 search of the Discoba protein database was also trialled but use of HMMER for Dis-
coba searches typically gave slightly higher pLDDT, presumably as AlphaFold v2.0 was trained
using HMMER MSAs. Predictions were again done using AlphaFold2 parameters from 2021-
07-14, not using Amber relaxation and not using PDB templates.
The test set of L. infantum proteins were selected, using a random number generator, from the proteins meeting the criteria for each group, see Results for selection criteria. Randomly
selected conserved genes: A4HU53, A4I2E1, A4HUD2, A4IA46, A4I444, A4IC57, A4IAB2,
A4I7M6, A4I0C5, A4HTD2. Randomly selected not conserved genes: A4I944, A4HYM2,
A4I1S6, A4I5D0, A4IAG0, A4I787, A4I9X8, A4IB72, A4I5C1, A4HS18. Randomly selected
not conserved ‘promising’ (see Results) genes: E9AGZ8, A4HW74, A4HZS9, A4I0P7, A4I2Z9,
A4IDS7, A4HRK9, A4IBK2, A4I4D7, E9AGB8.
Results
AlphaFold2 self-reports confidence in predictions through two measures: predicted local dis-
tance difference test score (pLDDT) [55], a per residue 0 to 100 score with high values showing
higher confidence, and predicted average error (pAE), a per residue pair distance score with
low values showing lower error. Many AlphaFold2-predicted protein structures for Leish- mania infantum and Trypanosoma cruzi (from the AlphaFold database alphafold.ebi.ac.uk [1, 4]) had high pLDDT and low pAE. This is an impressive achievement, however using the
Alphafold database pLDDT proteome-wide performance of AlphaFold2 can be evaluated
quantitatively. For comparison mouse (Mus musculus) was selected as, unlike human proteins, predictions were carried out without special treatment. Overall, L. infantum and T. cruzi pro- tein structure predictions are skewed to lower pLDDT (Fig 1A).
Lower pLDDT could be explained by more disordered protein domains, as predictions for
these regions correlate with low pLDDT [1]. L. infantum and T. cruzi proteins do not have a markedly different predicted degree of disorder to M. musculus (Fig 1B) although, as expected [1], pLDDT had a negative correlation with disorder score in all three species (Fig 1C). Alter-
natively, it may be a limitation due to the depth of the input protein MSAs. pLDDT correlated
with number of orthologs detected using OrthoFinder [43–45] on a set of 77 reference proteins
of diverse eukaryotes (Fig 1D), indicating that MSA depth is a likely explanation.
Unlike M. musculus, the distribution of number of orthologs for L. infantum and T. cruzi was strongly bimodal with many having fewer than 10. These proteins had, on average,
markedly lower pLDDTs (Fig 1D). Analysis using more stringent measures of protein specific-
ity to the kinetoplastids showed a similar pattern: Kinetoplastids-specific proteins were identi-
fied as those with only reciprocal best sequence search hits among the kinetoplastids (Fig 1E)
or those with only orthogroup members among the kinetoplastids (Fig 1F), and both sets had
low pLDDT (Fig 1E, 1F). Overall, this confirms that MSA quality is likely the limiting factor
for many T. cruzi and L. infantum protein structure predictions. As much protein sequence data as possible was therefore gathered for Discoba species,
drawing upon both TriTrypDB [15, 16] (well known to the Trypanosoma and Leishmania community), and lesser known, unpublished or very recent data available via nucleotide sequencing databases, gathered using the NCBI taxonomy browser (see Methods, S1 Table).
Many of these proteomes are in UniParc, but seemingly not used to build UniRef100 which is
one of the key databases used by the AlphaFold database and ColabFold. Unlike many applica-
tions, precise knowledge about sample or species identity, high sample purity and high tran-
scriptome/genome coverage are not critical–therefore the gather was as inclusive as possible.
This database ultimately included 238 predicted proteomes, representing 1.45 billion amino
acids across 4.3 million protein sequences.
PLOS ONE Improving trypanosomatid protein structure predictions
PLOS ONE | https://doi.org/10.1371/journal.pone.0259871 November 11, 2021 5 / 12
To benchmark any improvements over the AlphaFold database predictions a set of 30 L. infantum proteins were selected: 10 random proteins which have orthologs in many diverse eukaryotes, 10 random proteins which appear unique to the kinetoplastid lineage (no
orthogroup members outside the kinetoplastids) and 10 random proteins which appeared
‘promising’ and likely to have globular domains but with a low pLDDT in the AlphaFold
Fig 1. Proteome-wide quality of protein structure predictions of kinetoplastid proteins in comparison to mouse
proteins in the AlphaFold database. A) Distribution of per-protein average pLDDT for all L. infantum (7924), T. cruzi (19024) and, for comparison, M. musculus (21588) proteins, from the AlphaFold database [1, 4]. Scores for very low, low, confident and very high confidence categories are the same as used on the website. B) Distribution of per-
protein average IUPred score for the same three species. C) Correlation of per-protein average pLDDT with IUPred
score for the same three species. D) Correlation of per-protein average pLDDT with number of orthologs (total
number of orthogroup members determined from a diverse set of eukaryotes, see Methods). A random number
between 0 and 1 was added to each ortholog count to better represent point density at low ortholog numbers. E,F)
Distribution of per-protein average pLDDT for all L. infantum and T. cruzi proteins lacking an ortholog outside of the kinetoplastids, as determined by either E) orthogroup members only in kinetoplastid species (1509 and 7181 proteins
respectively) or F) reciprocal best protein sequence search hits only in kinetoplastids species (2361 and 11723 proteins
respectively).
https://doi.org/10.1371/journal.pone.0259871.g001
PLOS ONE Improving trypanosomatid protein structure predictions
PLOS ONE | https://doi.org/10.1371/journal.pone.0259871 November 11, 2021 6 / 12
database. The latter were selected based on size (avoiding small proteins, ≳300 amino acids), lack of low complexity or repetitive regions (≲30% unstructured and manually avoiding repeats), orthologs in few species (≲10), without numerous paralogs, and low average pLDDT (≲60).
To carry out AlphaFold2 protein structure predictions ColabFold was selected as a fast but
high accuracy and accessible AlphaFold2 implementation [5, 20]. As expected, unmodified
ColabFold gave per-protein mean pLDDTs comparable to, but on average slightly lower than,
the AlphaFold database for the test proteins (Fig 2A). Lower pLDDTs may be through Colab-
Fold’s use of MMSeqs2 rather than HMMER, on which AlphaFold2 was originally trained, for
MSAs. ColabFold was then modified to generate a HMMER-generated MSA from the Discoba
database and append this to the default MSA, before carrying out the AlphaFold2 prediction.
This extended MSA improved mean pLDDT for a large majority of protein structure predic-
tions, whether compared to the AlphaFold database or unmodified ColabFold, with less confi-
dent predictions seeing the largest improvement (Fig 2B and 2C). pLDDT increase occurred at
all confidence levels within a protein. Using the confidence thresholds in the AlphaFold data-
base, the proportion of residues over the threshold for a low confidence (>50), confident
(>70, high confidence in backbone structure) and high confidence (>90, likely correct side-
chain rotamers) prediction almost all increased for a large majority of proteins (Fig 2D).
Improvement was most marked among the test proteins not conserved outside of the kine-
toplastids, especially the ones selected as ‘promising’ (Fig 2A). Inspection of these predictions
showed a range of improvements, best interpreted from plots of pAE which show a pairwise
measure of predicted error in residue-residue spacing. Improvements included overall large
decreases in pAE (Fig 3A), the first high confidence prediction of any folds (Fig 3B) and the
prediction of a single high confidence domain (one contiguous block of low pAE) rather than
two subdomains (Fig 3C).
Fig 2. Improved AlphaFold2 predictions using ColabFold and a wider set of Discoba sequences for MSAs. A-C) Comparison of per-protein average pLDDT for 30
test proteins, 10 random widely conserved proteins, 10 random kinetoplastids-specific proteins and 10 ‘promising’ kinetoplastid-specific proteins which appeared
likely to improve with additional MSA sequences. A) Unmodified ColabFold in comparison to the AlphaFold database plotted as: Top, raw pLDDTs. Points to the top
left of the diagonal represent improved (higher pLDDT) predictions. Bottom, change in pLDDT. Points above the horizontal axis represent improvement. B) This
study (ColabFold with HMMER search of additional Discoba sequences) in comparison to unmodified ColabFold. C) This study in comparison to the AlphaFold
database. D) The same comparison as C) but plotting the proportion of residues over different threshold pLDDT values instead of mean pLDDT.
https://doi.org/10.1371/journal.pone.0259871.g002
PLOS ONE Improving trypanosomatid protein structure predictions
PLOS ONE | https://doi.org/10.1371/journal.pone.0259871 November 11, 2021 7 / 12
Discussion
This work shows that significant improvement in the pLDDT and pAE of AlphaFold2 struc-
ture predictions is possible for Trypanosoma and Leishmania proteins, relative to the publicly available AlphaFold database at alphafold.ebi.ac.uk [1, 4] and the open tool ColabFold [5, 20]
(Fig 2). This was simply by designing a protein sequence database for MSA generation more
appropriate for the Discoba branch of eukaryotic life. Easy-to-use tools for MSA generation
and AlphaFold2 structure prediction exploiting this Discoba protein sequence database have
been made available at github.com/zephyris/discoba_alphafold. Even in these early-branching
eukaryotes, the huge advance AlphaFold (and RoseTTAfold) represent can therefore, to a
great extent, translate protein structure determination into a genome and transcriptome
sequencing problem. Although, experimental protein structure determination will continue to
be vital to confirm predictions, explore dynamics and complexes, etc.
Structure prediction improvement was most marked for proteins specific to the kinetoplas-
tids. A large proportion of trypanosomatid parasites’ genomes falls into this group–several
hundred to thousands depending on definition (Fig 1E and 1F). Many of these proteins lack
any domains detectable by primary sequence (sometimes called the ‘dark proteome’) making a
structure prediction a first insight into potential function. However, improvement in protein
Fig 3. Example L. infantum proteins showing significant improvement in
Collepals.com Plagiarism Free Papers
Are you looking for custom essay writing service or even dissertation writing services? Just request for our write my paper service, and we'll match you with the best essay writer in your subject! With an exceptional team of professional academic experts in a wide range of subjects, we can guarantee you an unrivaled quality of custom-written papers.
Get ZERO PLAGIARISM, HUMAN WRITTEN ESSAYS
Why Hire Collepals.com writers to do your paper?
Quality- We are experienced and have access to ample research materials.
We write plagiarism Free Content
Confidential- We never share or sell your personal information to third parties.
Support-Chat with us today! We are always waiting to answer all your questions.
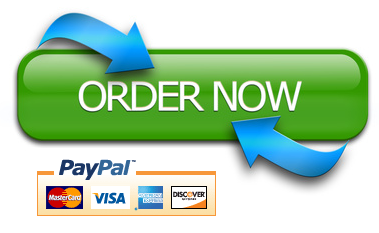