Economics Question
THE MACROECONOMIC CONSEQUENCES OF TERRORISM S. BROCK BLOMBERG GREGORY D. HESS ATHANASIOS ORPHANIDES CESIFO WORKING PAPER NO. 1151 CATEGORY 5: FISCAL POLICY, MACROECONOMICS AND GROWTH MARCH 2004 An electronic version of the paper may be downloaded • from the SSRN website: http://SSRN.com/abstract=525982 • from the CESifo website: www.CESifo.de CESifo Working Paper No. 1151 THE MACROECONOMIC CONSEQUENCES OF TERRORISM Abstract We perform an empirical investigation of the macroeconomic consequences of international terrorism and interactions with alternative forms of collective violence. Our analysis is based on a rich unbalanced panel data set with annual observations on 177 countries from 1968 to 2000, which brings together information from the Penn World Table dataset, the ITERATE dataset for terrorist events, and datasets of external and internal conflict. We explore these data with cross-sectional and panel growth regression analysis and a structural VAR model. We find that, on average, the incidence of terrorism may have an economically significant negative effect on growth, albeit one that is considerably smaller and less persistent than that associated with either external wars or internal conflict. As well, terrorism is associated with a redirection of economic activity away from investment spending and towards government spending. However, our investigation also suggests important differences both regarding the incidence and the economic consequences of terrorism among different sets of countries. In OECD economies, in particular, terrorist incidents are considerably more frequent than in other nations, but the negative influence of these incidents on growth is smaller. JEL classification: E6, h1, H5, D74, O11. Keywords. growth, conflict, terrorism. S. Brock Blomberg Department of Economics Claremont McKenna College Claremont, CA 91711 U.S.A. [email protected] Gregory D. Hess Department of Economics Claremont McKenna College Claremont, CA 91711 U.S.A. Athanasios Orphanides Board of Governors of the Federal Reserve System 21st and C Streets, NW Washington, DC 20551 U.S.A. This paper was written for the Carnegie-Rochester Conference on Public Policy on the Macroeconomics of Terrorism, Pittsburgh, PA, November 21-22, 2003. We thank Tim Riesen for excellent research assistance and Jong-Wha Lee, Kwanho Shin, Michelle Garfinkel, Ivan Jeliazkov, and participants at presentations at Korea University, Korea Institute for International Economic Policy (KIEP) and the Carnegie-Rochester Conference for very helpful comments. Tim Riesen’s assistance was supported by a Freeman Grant. The opinions expressed are those of the authors and do not necessarily reflect the views of the Board of Governors of the Federal Reserve System. 1 Introduction There has been a long tradition among economists to try to understand the economic consequences of conflict and peace. In the aftermath of World War I and leading up to World War II, for example, several leading economists, among them Keynes (1919), Pigou (1940), Meade (1940) and Robbins (1942), sought to trace through the interactions between war, peace and the economic situation of their day, and applied economic reasoning to offer pertinent policy advice.1 In comparison to external wars and internal conflict, however, terrorism—and, in particular, its economic consequences—has received much less attention in the economic literature. But our attention has changed in the post September 11th era. The heightened awareness of the human cost associated with terrorist events as well as the significant redirection of economic resources, presumably motivated by the perceived risks associated with possible future terrorist incidents, have refocused efforts towards a better understanding of terrorism and its economic consequences. The objective of this study is to make progress towards an examination of the macroeconomic consequences of terrorism. Our analysis is based on a rich unbalanced panel data set with annual observations on 177 countries from 1968 to 2000, which brings together information from the Penn World Table dataset, the ITERATE dataset for terrorist events, and datasets of external and internal conflict. Using these data, and building on earlier work on conflict in political science and economics, we perform an empirical investigation of the macroeconomic consequences of international terrorism and interactions with alternative forms of collective violence. As part of our analysis, we document the pervasive nature 1 More recently, researchers have also taken great strides to understand these interactions between conflict and economic well being. Garfinkel (1990), Grossman (1991), Skaperdas (1992), Hess and Orphanides (1995, 2001a,b), and Alesina and Spolaore (1997) have extended our understanding in the direction of arming, insurrection, appropriation, diversionary conflict, form of governance, and the number and size of nations. 1 of international terrorism since 1968, and juxtapose its frequency and impact on economic activity in comparison to internal and external conflict. Then, we investigate the dynamic interactions between these alternative forms of collective conflict and their consequences on economic growth based on cross country growth regressions, panel data regressions, and structural VARs. Our results suggest that, on average, the incidence of terrorism may have an economically significant negative effect on growth, albeit one that is considerably smaller and less persistent than that associated with either external wars or internal conflict. As well, terrorism is associated with a redirection of economic activity away from investment spending and towards government spending. However, our investigation also suggests important differences both regarding the incidence and the economic consequences of terrorism among different sets of countries. Terrorist incidents appear considerably more frequent in more developed nations than in other nations. On the other hand, the negative influence of terrorist incidents on growth appears most significant in the developing world. To put our analysis into perspective, we note that political scientists have long emphasized that terrorism has been a constant source of worldwide tension through much of the post World War II era, and indeed the very origin of the term points to a long history, dating back to the late 1700s.2 In her seminal contribution on the causes of terrorism, Crenshaw (1981), identifies modernization, ‘social facilitation’ and the spread of revolutionary ideologies as important factors that drive terrorism. This political theory serves as a useful point of departure for empirical investigations of terrorism. Modernization can isolate certain groups while at the same time provide more cost effective ways of equipping these same 2 The word “terrorism” apparently first appeared in the English language in reference to the “Reign of Terror” associated with the rule of France by the Jacobins from 1793-94. 2 groups. This view of modernization suggests that terrorism may be more prevalent in higher income countries (i.e OECD countries) which tend to experience higher rates of technological progress.3 Social facilitation or ‘social habits and historical traditions that sanction the use of violence against the government’ (p. 382) is another way of saying that internal violence begets violence. This points to the importance of controlling for interactions between terrorism and other forms of conflict in order to identify the economic consequences of the former, a consideration we incorporate into our analysis. Crenshaw’s final factor comes in such a variety in the data (e.g. nationalism, religion, etc.) that its exploration from an economic perspective is beyond the scope of this paper. A few economic researchers have also examined theoretical aspects of the incidence of terrorism. Lapan and Sandler (1988, 1993) present game theoretic analyses of terrorism, and Garfinkel (2003) examines the interactions between terrorism and other types of conflict. On the empirical side, Enders, Sandler and Cauley (1990) and Enders and Sandler (1993) assess the effectiveness of terrorist-thwarting policies on reducing the incidence of terrorism, O’Brien (1996) looks at the role of terrorism as a foreign policy tool and work by Enders, Sandler and Parise (1992) and Blomberg, Hess and Weerapana (2003, 2004) examine some of the effect of terrorism on the economy. The economic literature has therefore reinforced the political science literature by stressing terrorism’s important link to institutions and other forms of conflict and pointing to the possible economic consequences of these factors. Drawing on this earlier literature, in our investigation of the economic consequences of terrorism we attempt to control several of these factors and the interaction of of terrorism with other forms of conflict. 3 See also Krueger and Maleckova (2002) for analysis consistent with this view. 3 2 The Data and Empirical Regularities In this section, we describe our data sources and limitations and examine some basic empirical regularities of the resulting dataset. In constructing the data set, our goal is to benchmark terrorism data with the standardized and broadly accepted international economic data source—the Penn World Table data, based on the work by Summers and Heston (1991).4 This has certain implications for the organization of our data. Importantly, since our benchmark is given as a country-year panel, we must convert data on the incidence of terrorism (and other variables) accordingly. To allow for examination of possible strategic complementarity and substitutability across forms of collective violence, we include other standard measures of conflict including: measures of internal conflict such as genocide, ethnic war, revolutions and irregular regime changes; and measures of external conflict that allow for both home and away wars. The addition of these variables also permits us to examine some measurement issues associated with the possible mis-classification of various forms of conflict. Our intent is to examine the interaction between terrorism and the economy, controlling for the possible interactions between these types of conflict. Our data are obtained from four different sources. To measure terrorist activities, we employ the latest update of the “International Terrorism: Attributes of Terrorist Events” (ITERATE) data set from Mickolus et. al. (2002). The economic data are obtained from an update of the Summers and Heston (1991) (Penn World Table) data set. Using this dataset, we can calculate annual per-capita GDP growth rates and related variables in PPP adjusted exchange rates from 1968 to 2000. The internal conflict data are obtained from Gurr et. al. (2003) and the external conflict data are obtained from the most recent update to Brecher, 4 We also considered matching other types of data such as tourism, foreign direct investment, etc. However, the availability of the data limited our ability to investigate the issue on a large scale. 4 Wilkenfeld and Moser (1988). In all, the resulting data set covers 177 countries over 33 years providing an unbalanced panel data set of over 4000 observations. Unlike the Penn World Table dataset, the sources of our data on conflict have not been examined extensively in the economic literature, so some additional description is warranted. The ITERATE data set attempts to standardize and quantify characteristics, and activities of transnational terrorist groups. An international terrorist event is defined as: “the use, or threat of use, of anxiety-inducing, extra-normal violence for political purposes by any individual or group, whether acting for or in opposition to established governmental authority, when such action is intended to influence the attitudes and behavior of a target group wider than the immediate victims and when, through the nationality or foreign ties of its perpetrators, its location, the nature of its institutional or human victims, or the mechanics of its resolution, its ramifications transcend national boundaries.” Mickolus et. al., (page 2). In short, a terrorist event is required to be employed for political purposes to influence a wider target group on an international scale. This means that events such as September 11, 2001 are included in this dataset but some other terrorism events, such as the Oklahoma city bombing, are not deemed to meet all the relevant criteria.5 ITERATE provides a rich micro-level data set of 12,164 incidents of terrorism across 179 countries from 1968 to 2001. The raw data is grouped into four broad categories. First, there are incident characteristics which code the timing of each event. Second, the terrorist characteristics yield information about the number, makeup and groups involved in the incidents. Third, victim characteristics describe analogous information on the victims involved in the attacks. Finally, life and property losses attempt to quantify the damage of the attack. Unfortunately, the information across many of these categories is not provided in a consistent manner as the original source material comes from news organization resources 5 As the economic data ends in 2000, September 11th is not actually included in our analysis sample. 5 which may fail to report a particular factor, such as the number of victims. This presents a significant limitation of this dataset. Because of this limitation, we limit our attention to the number of terrorist incidents reported, which is the most consistent measure reported in the ITERATE dataset. Further, since we cannot control for the significance of individual events, for our baseline measure of terrorism incidence we define a dummy variable which takes the value 1 if a terrorist event is recorded for a given country year and 0 otherwise. This measure also has the advantage of defining the incidence of terrorism in a manner comparable to the incidence of other forms of conflict in the data set. As an alternative, we also report results using the number of incidents-per-capita in a given year as a measure of the incidence of terrorism. External conflict is the initiation or escalation of a foreign policy crisis that results in violence. A foreign policy crisis is defined by Brecher, Wilkenfeld and Moser (1988) as: “a specific act, event or situational change which leads decision-makers to perceive a threat to basic values, time pressure for response and heightened probability of involvement in military hostilities. A trigger may be initiated by: an adversary state; a non-state actor; or a group of states (military alliance). It may be an environmental change; or it may be internally generated.” (page 3) A foreign policy crisis with an intensity of a specified magnitude is called a conflict. This particular definition comes from the International Crisis Behavior (ICB) project undertaken by Brecher, Wilkenfeld, and Moser (1988) which includes the initiation or escalation of a conflict that warrants the highest level of severity.6 The Internal war data, obtained from Gurr et al (2003), provides data that originates from four broader categories. First, ethnic conflict is defined as conflict between the government and national ethnic, religious, or other communal minorities seeking changes 6 Such a definition is used in Hess and Orphanides (1995). 6 in their status. In order to be considered a war, more than 1000 individuals had to be mobilized and 100 fatalities must have occurred. Second, genocide is defined to include the execution, and/or consent of sustained policies by governing elites or their agents that result in the deaths of a substantial portion of a communal group (genocide) or a politicized non-communal group (politicide). In contrast to ethnic conflict, victims counted are non-combatants and the percentage of those killed in each group is given more weight than the number of dead. Third, revolutionary conflict is defined as conflict between the government and politically organized groups seeking to overthrow those in power. Groups include political parties, labor organizations, or parts of the regime itself. Once again, in order to be considered a conflict, more than 1000 individuals had to be mobilized and 100 fatalities must have occurred. An example of such a conflict would be the Chinese Tiananmen Square massacre of 1989. Finally, regime change includes state collapse and shifts from democratic and authoritarian rule as defined by a shift of at least 3 points on the Freedom House polity scale.7 This measure does not include nonviolent transitions. 2.1 The Geography of Terrorist Incidents The greatest incidence of terrorism in our sample appears in the Americas and Europe. This might suggest that terrorism is an unfortunate consequence of wealth and freedom. For example, after Lebanon with an average of 25.5 terrorist events per year, the United States experiences the second highest incidence, with an average of about 20.4 terrorist events a year, followed closely by Germany and France at 19.3 and 17.9 incidents per year, respectively. But neighboring countries with similar income and political systems do not 7 Freedom House measures the extent of democratization of a regime using two measures—civil liberties and political rights. Both measures are scaled 1 to 7 with 1 being the most democratic. 7 share such high incidence rates. Countries such as Canada (1.4 incidents per year) and the Nordic countries such as Sweden (1.6) Norway(0.5) and Finland (0.0) do not have such problems. Further, from 1995 to 2000, as incomes generally improved across the globe, and democratization increased to unprecedented levels, the average number of terrorist events per country year actually fell and remained below the long term average of about 2.0 in each year.8 This suggests some difficulties in comparing the incidence of terrorism across different nations and time periods. To understand some of the difficulties associated with interpreting the incidence of terrorism, consider the following fact from two of the high-incidence countries mentioned above—the United States and France. During the 1960s, 1970s and part of the 1980s, the main perpetrator in each country came from a single organization. In the United States, the main culprit was the FALN (Armed Front for National Liberation), a Puerto Rican separatist group. In France, the main instigator was (CNLF) the Corsican National Liberation Front. Yet, in both cases, during the later part of the 1980s and 1990s, both the FALN and CNLF are virtually non-existent. Such anecdotal evidence is suggestive of many issues facing researchers dealing with these data which may complicate interpretation of the results. Some statistics may be unduly influenced by one interest group, region or country and may be quite hard to generalize going forward.9 Moreover, if we considered the prevalence of terrorism on a per capita basis, the re8 Since September 11, 2001 terrorism may have again spiked upward. However, the data is not yet available to consider such an issue. Another complication is that a nation is coded as a “recipient” of terrorism when an incident occurs in its territory, even if another nation appears to be the primary target. For example, in the bombing of the U.S. embassies in Africa on August 7 1998, the events are linked to Kenya and Tanzania, and not to the United States. 9 Indeed, Enders and Sander (2002) catalog the types of terrorism over time to highlight the importance of such idiosyncrasies. From the early 1960s to the late 1980s, terrorism is predominantly motivated by nationalism, separatism, and other more radical considerations. Since the 1990s, however, religion appears to have played a larger role. 8 lationship between governance, income and terrorism is somewhat smaller. While a simple Spearman rank correlation shows that the correlation between the country rankings of average incidence of terrorism and the country rankings of average terrorism per capita is .60, countries with large populations such as the United States drop in world rankings. Indeed, as one might suspect, countries in the Middle East tend to be countries with higher rates of terrorist incidents per capita.10 2.2 The Empirical Regularities of Conflict In this subsection we examine a snapshot of the incidence of terrorism around the world and its relationship to other forms of conflict and to economic growth. Table 1 presents the basic statistics on terrorism (T), internal conflict (I), and external wars (E) [both home (H) and away from home (A)]. The data are parsed in a variety of ways to highlight possible differences across the various measures of conflict. The table includes statistics regarding the mean (MEAN), standard deviation (STD), measures of first-order autoregressive persistence (AR1), and the sum of squares (SSQ). Finally, to highlight the importance of possible time and country differences, we provide a breakdown of the variation brought by each of the individual (INDIV), time (TIME) and random (RANDOM) effects. Each column provides the results from a different parsing. Column 2 provides the results over the entire sample. Column 3 provides the results for non-democracies (NONDEMO). Column 4 provides results for OECD economies, which we consider our sample of high income countries. Columns 5 through 7 provide results for Africa (AFRICA), the Middle East (MIDEAST) and Asia (ASIA). 10 As detailed later on, in our empirical analysis we examined alternative ways for controlling for population differences but did not find major qualitative differences relative to our baseline results that do not control for country size. 9 Table 1: Basic Statistics STAT MEAN STD AR1 SSQ INDIV TIME RANDOM STAT MEAN STD AR1 SSQ INDIV TIME RANDOM STAT MEAN STD AR1 SSQ INDIV TIME RANDOM ALL 0.148 0.355 0.869 757.6 0.461 0.006 0.536 INTERNAL CONFLICT (I) NONDEMO OECD AFRICA MIDEAST 0.189 0.022 0.151 0.221 0.392 0.148 0.358 0.416 0.860 0.805 0.829 0.892 648.8 21.5 204.6 99.7 0.466 0.478 0.404 0.397 0.006 −0.006 0.019 −0.008 0.521 0.544 0.586 0.633 ASIA 0.291 0.455 0.900 224.7 0.483 −0.004 0.535 ALL 0.008 0.090 0.079 48.6 0.035 0.005 0.960 EXTERNAL HOME WARS (H) NONDEMO OECD AFRICA MIDEAST 0.010 − 0.006 0.040 0.100 − 0.075 0.196 0.064 − 0.106 0.064 42.563 − 8.949 22.086 0.028 − 0.009 0.039 0.005 − 0.025 0.089 0.967 − 0.966 0.876 ASIA 0.007 0.085 −0.008 7.941 0.025 0.019 0.957 ALL 0.009 0.094 0.344 53.5 0.042 0.006 0.952 EXTERNAL AWAY WARS (A) NONDEMO OECD AFRICA MIDEAST 0.009 0.006 0.011 0.024 0.093 0.078 0.103 0.154 0.342 0.497 0.328 0.194 36.7 5.9 16.8 13.6 0.027 0.068 0.034 0.049 0.004 −0.003 0.009 0.092 0.969 0.937 0.958 0.865 ASIA 0.005 0.068 0.397 4.9 0.021 −0.003 0.983 TERRORISM (T) OECD AFRICA 0.425 0.153 0.495 0.360 0.315 0.371 241.1 207.4 0.216 0.232 0.052 0.024 0.741 0.749 2904 2079 ASIA 0.274 0.446 0.424 216.4 0.292 0.015 0.703 561 STAT ALL NONDEMO MEAN 0.269 0.233 STD 0.443 0.423 AR1 0.391 0.361 SSQ 1182.5 755.9 INDIV 0.283 0.257 TIME 0.020 0.022 RANDOM 0.698 0.718 N OBS 5840 1741 MIDEAST 0.382 0.486 0.366 136.6 0.210 0.043 0.760 1551 Notes: MEAN, STD, AR1 refer to the data’s mean, standard deviation and first-order autoregressive parameter, respectively. SSQ is the data’s sum of squares, with INDIV, TIME and RANDOM as the fraction of the data’s variance that can be accounted for individual, time and random effects, respectively. NOBS is the number of observations in the sample. 10 The top panel examines internal conflict. This occurs in about 1 out of every 7 country-years and is much more likely to occur in non-democracies and low income regions. It is twice as likely to occur in the Middle East as in Africa, the latter of which makes up the majority of countries in our sample. A possible suggested interpretation is that many non-democratic and/or low-income countries are inundated with internal strife and that conflict may explain, in large part, why certain countries fail to advance. Interestingly, when considering the variance decomposition of the data, the individual effect seems to be as important as the random effect, with very little variation from the time effect. Moving to panels two and three, we see something different in the external war data. First, external wars are a much less frequent event as even the sum of home and away wars occur at a rate of one tenth the size of internal conflict. Second, while there continues to be a “Middle East” effect in external wars, the decomposition of the variation is almost entirely driven by the random component which suggests that controlling for individual effects will be less important for external war data. Third, home and away wars behave in a much different manner. Indeed, for OECD economies, there are no recorded Home wars in our data sample. Finally, we turn our attention to the incidence of terrorism. As reported in our dataset, terrorism occurs more frequently than other forms of conflict. On average, the frequency is twice that of internal conflict. Its occurrence in Africa and the Middle East is practically identical to internal conflict, highlighting its close relationship. This is also born out in the variance decomposition. As can be seen, a significant portion of the variance in the data is driven by individual effects. Interestingly, terrorism incidents are reported more frequently in democracies and high income (i.e. OECD) countries. This is opposite to what 11 was found in the other forms of conflict. This suggests that terrorism and internal conflict are fundamentally different and provides some assurance that the terrorism data do not primarily capture internal conflict episodes, which would have implied a severe identification issue for our analysis. Table 2 provides further evidence on the relationship between terrorism and other data. Terrorism is indeed correlated with internal conflict—though the relationship is almost entirely seen through country fixed effects. Table 2 also shows that there is a strong positive correlation between terrorism and income—though once again entirely demonstrated through country fixed effects. Following Table 1, Table 2 parses the data across different samples to examine the correlation of terrorism and other forms of conflict with and without fixed effects.11 In addition, this table examines the correlation of terrorism and variables such as GDP per capita (y), growth (∆y), (exports+imports)/GDP (OPEN), and Gini coefficient (GINI).12 The first panel of Table 2, shows that terrorism is not highly correlated with either home or away wars but is significantly positively correlated with internal conflict. It is also striking to see that the correlation coefficient between income and terrorism is significantly positive at about .20. This strong positive relationship might at first glance seem counterintuitive. The unexpected relationship may in fact have more to do with an alternative third factor that cannot be controlled for in such simple analysis as correlations. Some support for this conjecture is suggested when we examine the correlation in democracies (0.06) and for high income countries (−0.03), which demonstrates that for higher income countries which are often democracies, the correlation disappears. We pursue this reasoning in section 3. 11 The middle panel removes both time and fixed effects, though the results are nearly identical if time effects are not removed. 12 The data on Gini coefficients was obtained from Deininger and Squire (1996). 12 Table 2: Correlations with Terrorism Across Sub-Samples Correlation with Terrorism (T) NONDEMO OECD AFRICA MIDEAST 0.045 0.000 0.031 0.084 0.089 0.062 0.127 0.055 0.077 0.078 0.225 0.117 0.060 −0.076 0.113 0.325 −0.043 −0.043 −0.021 0.055 −0.269 −0.207 −0.154 0.220 0.056 0.187 −0.079 −0.178 H A I y ∆y OP EN GIN I ALL 0.037 0.056 0.151 0.197 0.000 −0.164 −0.018 ASIA 0.050 0.084 0.194 0.021 −0.124 −0.177 0.090 H A I y ∆y OP EN GIN I Correlation with Terrorism: Time and Fixed Effects Removed ALL NONDEMO OECD AFRICA MIDEAST ASIA −0.001 −0.005 −0.075 −0.026 0.030 −0.003 0.024 0.058 0.033 0.027 −0.011 0.044 0.062 −0.014 −0.022 0.029 0.068 0.063 −0.039 −0.079 −0.054 −0.045 0.070 0.021 −0.020 −0.014 −0.037 −0.013 0.024 −0.058 −0.024 −0.038 −0.055 0.056 0.000 0.001 0.014 0.051 0.030 −0.010 −0.089 −0.010 Correlation with Terrorism: Fixed Effects ALL NONDEMO OECD AFRICA MIDEAST ASIA H 0.299 0.288 0.000 0.474 0.755 0.481 A 0.203 0.208 0.244 0.666 0.620 0.479 I 0.321 0.213 0.294 0.659 0.353 0.547 y 0.325 0.335 −0.032 0.223 0.562 0.036 ∆y 0.140 0.025 0.228 −0.006 0.524 −0.299 OP EN −0.386 −0.494 −0.456 −0.458 0.531 −0.477 GIN I 0.082 0.154 0.581 −0.142 0.001 0.309 Notes: See Table 1. Correlation coefficients between Terrorism and Home wars (H), Away wars (A), Internal conflict (I), GDP per capita (y), growth (∆y), ln((exports+imports)/GDP) (OPEN), gini coefficient (GINI). Panel 1 represents the gross correlation. Panel 2 represents the correlation after removing time and fixed effects. Panel 3 represents the correlation of the country fixed effect. 13 Upon further inspection, the strong positive relationship between terrorism and internal conflict and terrorism and income is driven by a few sub-samples. In particular, the correlation between terrorism and internal conflict is twice as high in Africa than in other regions. Furthermore, the correlation between terrorism and income is three times higher in the Middle East than other regions. All of these results when taken together provide support for reconsidering these same correlations without time and country effects. The second panel is devoted to this exercise. Panel two shows that removing these effects significantly decreases the correlation between terrorism and conflict, even though terrorism continues to be more correlated with internal conflict (0.06) than home (−0.001) and away (0.02) wars. This panel also shows that removing these effects causes the correlation between terrorism and income and growth to turn negative. In fact, the correlation between income and terrorism turns from positive to negative and statistically significant for democracies. Panel three further highlights these points by comparing the correlation the same variables with only country effects included. The correlations between all measures of conflict are all positive and statistically significant. The correlations between income and terrorism are similarly high. To summarize, there appears to be a strong and positive relationship between terrorism and internal conflict and terrorism and income or growth. It also appears that much of this relationship is driven by country fixed effects—a key element we will control for below for the following reasons. In the following section we attempt to sort out these long-run and short-run effects of terrorism on the economy as well as to understand some of the short-run feedback between terrorism and other types of organized conflict. 14 3 Econometric Evidence The purpose of this section is fourfold. First, we want to examine the economic impact of terrorism within the context of the long-standing cross national growth regression literature. Second, we wish to re-examine these results in panel regressions controlling for specific country and year effects and accounting for for possible endogeneity bias using instrumental variables estimation. Third, we wish to establish the extent to which conflict and terrorism reallocate economic activity across investment and government spending. Finally, using these basic findings, we construct a structural VAR to examine the impact of terrorism on GDP per capita and the interrelationship between terrorism and other forms of violence.13 3.1 Cross Country Growth Regressions We begin these exercises by constructing our baseline model by appealing to the literature on economic growth. The workhorse model employed in the literature is cross country regressions (see e.g. Barro, 1997) subjected to robustness checks. As is well documented, the majority of variables in such cross-sectional growth regressions are not found to be as statistically or economically significant as standard growth theory might suggest (see e.g. Levine and Renelt, 1992). In recent years, a series of papers has sought to attack this difficulty by exploiting the panel dimension of the data and by introducing a better set of instruments for geography, policy or institutions with some promising results. For example, Judson and Orphanides (1999) show that the adverse role of high and volatile inflation on growth becomes clearer in panel growth regressions than appears to be in cross-sectional growth regressions. Frankel and Romer (1999) use a gravity model as an instrument for trade 13 Hess (2004) and Blomberg and Hess (2002) explore the empirical inter-relationships between business cycles and internal and external conflict. 15 to demonstrate the importance of policy on growth. Acemoglu, Johnson and Robinson (2001) use historical data on settler mortality to demonstrate the importance of institutions on growth. However, Dollar and Kraay (2003) have recently documented that such instruments may be weak in the sense that the results based on IV/GMM methods are not robust. With this in mind in our analysis we report results based on cross-section and panel growth regressions using OLS as well as estimates based on an IV/GMM approach. Our baseline cross-country regression model includes investment as a share of GDP (I/Y) and the log of initial GDP (lny0i ) to control for transitional dynamics. We also include a dummy for Africa (AFRICA) which researchers have consistently identified as important, both in economic and statistical terms. Starting from this baseline model, and in line with earlier work in this literature, we examined other policy, regional, or institutional variables that might be important in explaining economic growth. Our findings were broadly consistent with the early research—most institutional, geographical or policy variables tended to be fragile in their ability to statistically influence economic growth. Hence, we only include one more control variable (COM)—a dummy variable for non-oil commodity exporters—found to be robust by Easterly and Kraay (2000) when using data that includes many small countries, such as our own.14 ∆yi = β0 + β1 COMi + β2 AFRICAi + β3 lny0i + β4 I/Yi + β5 Ti + β6 Ii + β7 Ei + εi . (1) where ∆yi and (I/Y )i are country i’s average per-capita growth rate and investment rate over the full sample. For the IV/GMM counterparts to our baseline models, we followed the suggestion in De La Croix and Doepke (2003) and controlled for the possible endogeneity 14 We found the trade measure COM was more robust than either a measure of openness or a dummy variable for oil. The negative coefficient associated with COM may indicate that the variable is a proxy for countries that are susceptible to trade shocks but do not have the revenue associated with oil. This is consistent with what was found in Easterly and Kraay (2000). 16 bias by employing initial values of the transitional variables as instruments.15 Table 3 reports the results from the cross-sectional growth regressions. Column 1 is the base case following the early 1990s growth literature. Columns 2 and 3 sequentially include terrorism (T) and internal conflict (I). Columns 4 and 5 include external conflict separate and then together with other forms of conflict to demonstrate how the different types of conflict influence growth. Columns 6 through 10 repeat the same regressions with one change—IV/GMM is employed to instrument for the endogeneity of investment. Column 1 yields the standard results for growth—investment has a positive impact and initial income, Africa and commodity exporters have a negative impact—each statistically significant at all conventional levels. In fact, the sign of these effects are all quite similar to what others have shown using different techniques and data samples. The only impact that appears to be slightly different is the coefficient on initial GDP, which is smaller primarily because we employ GDP in 1967—the year before the terrorism data is available—and not 1960 which is typically used. Column 2 provides our first direct estimate on the impact of terrorism. The impact is negative and statistically significant, implying that if a country were to experience a terrorist event in each year in the sample, per capita growth would drop by about 1.5 percent. To estimate the impact of one conflict for a given year, we must divide the coefficient by 33. While the impact may seem small, it is actually somewhat higher than the impact of internal conflict on growth which is given in Column 3. Column 4 provides more evidence on conflict’s impact on growth. In this case, we see that external conflict appears to have a large and negative impact. Yet, we see that the 15 Due to data limitations, using a common year for the initial values reduces the number of observations to 115. 17 18 0.142∗∗∗ [0.022] 0.147∗∗∗ [0.022] I/Y 115 0.56 115 0.55 −0.663∗∗ [0.279] 0.135∗∗∗ [0.022] −0.782∗∗∗ [0.174] −1.109∗∗∗ [0.366] 3 &I OLS −1.186∗∗∗ [0.325] 115 0.53 −2.837 [3.326] 0.146∗∗∗ [0.022] −0.712∗∗∗ [0.171] −0.987∗∗∗ [0.373] 4 &E OLS −1.123∗∗∗ [0.325] 115 0.57 −0.356 [0.331] 0.696 [3.009] −1.291∗ [0.683] 0.137∗∗∗ [0.022] −0.692∗∗∗ [0.179] −1.357∗∗∗ [0.397] 5 & T,I,E OLS −1.220∗∗∗ [0.335] 113 0.50 0.094∗∗∗ [0.026] −0.498∗∗∗ [0.167] −1.234∗∗∗ [0.378] 6 Base IV −1.155∗∗∗ [0.339] 113 0.54 −1.774∗∗∗ [0.579] 0.094∗∗∗ [0.025] −0.440∗∗∗ [0.166] −1.650∗∗∗ [0.408] 7 &T IV −1.248∗∗∗ [0.340] 113 0.52 −0.877∗∗∗ [0.266] 0.083∗∗∗ [0.026] −0.606∗∗∗ [0.171] −1.404∗∗∗ [0.374] 8 &I IV −1.246∗∗∗ [0.334] 113 0.50 −3.838 [3.230] 0.092∗∗∗ [0.025] −0.492∗∗∗ [0.167] −1.268∗∗∗ [0.383] 9 &E IV −1.165∗∗∗ [0.337] 113 0.54 −0.565∗ [0.329] 0.243 [2.888] −1.257∗ [0.708] 0.087∗∗∗ [0.026] −0.526∗∗∗ [0.174] −1.636∗∗∗ [0.405] 10 & T,I,E IV −1.278∗∗∗ [0.339] Notes: Robust standard errors are presented in square brackets. ∗, ∗∗ and ∗∗∗ represent statistical significance at the .10, .05 and .01 levels, respectively. Models (1) through (10) are different specifications of cross country growth regressions. Models (1) through (5) are the basic OLS model adding separately the different forms of conflict, i.e. terrorism (T), internal conflict (I), home (H) and away (A) wars and their sum, external wars (E). Models (6) through (10) repeat the exercises but estimate the model as IV/GMM with initial investment as a percent of GDP (I/Y) as the instrument. Included in each regression is a dummy for non-oil exporting commodity countries (com), Africa (afr), initial GDP per capita (lny0) and average investment as a percent of GDP (I/Y). The R-squared measure excludes the contribution from the individual fixed effects. Obs R-squared E I 115 0.53 −0.641∗∗∗ [0.166] −0.715∗∗∗ [0.170] lny0 −1.587∗∗∗ [0.569] −1.358∗∗∗ [0.400] −0.963∗∗∗ [0.366] AFRICA T 2 &T OLS −1.200∗∗∗ [0.334] 1 Base OLS −1.116∗∗∗ [0.326] Model Specification Estimation COM Table 3: Cross Sectional Regression: Terrorism and Growth impact of external war is not statistically significant even when considered with other forms of conflict. It is possible that these effects appear small because of endogeneity bias. To address this issue, columns 6 through 10 show the impacts on growth using the IV/GMM specification. We see the general results carry through suggesting the presence of a robust, though economically small, impact of both terrorism and internal wars on growth. More reassuring is the fact that formal tests of the exogeneity of our instruments demonstrate that the model is not rejected at the 5 percent level. However, while the magnitude of the coefficients are higher on the conflict variables, the magnitude is actually smaller on I/Y implying our instruments may not be as strong as one would like.16 There are several possible interpretations of the strong statistical (though small in an economic sense) impact of terrorism in Table 3. First, since the data in Table 3 is purely cross-sectional, there may be important fixed effects that are dominating the information in the data. One way to control for this is to include dummies such as COM and AFRICA, but this is crude at best. Moreover, given our findings in Tables 1 and 2, it might be surprising that the coefficient on terrorism and growth or consumption is negative, given the strong positive correlation in the fixed effect terms. Second, since the data is cross-sectional, the regression may be capturing only the long run effect of terrorism on growth or consumption. It may be of greater interest to examine separately the short- and long-run impact of terrorism given that much of the concern about the economics of terrorism may be related to its impact on financial markets which may have 16 We repeated the same exercise using consumption growth as the dependent variable instead of economic growth. The rationale for this regression is that standard economic theory suggests that utility is a function of consumption, not output. The results (not shown) for consumption growth are very similar to those presented for output. 19 a more transitory relationship to growth. Finally, we may estimate a small economic impact because the impact is in fact small. The main point here is that although results based on cross-sectional regression, such as presented in Table 3, are suggestive, there are significant challenges in interpreting them. Since we are unable to differentiate between long and short run and fixed effects bias, we turn our attention to panel regressions and later also examine the data from the viewpoint of a structural VAR. In Table 4, we reexamine the evidence presented in Table 3 with panel regressions and also examine various country sub-samples (NONDEMO, OECD, AFRICA, MIDEAST, ASIA). In these regressions we control for time (year) and fixed (country) effects as well as for the openness of a country. The resulting specification is: ∆yit = γ0 + γ1 lnopit−1 + γ3 lnyit−1 + γ4 I/Yit−1 + γ5 Tit + γ6 Iit + γ7 Eit + φZ + εit (2) where Z is a set of time and country fixed effects and φ is a vector of corresponding coefficients.17 Column 1 once again shows that I/Y and lagged GDP per capita are statistically significant and have the theoretically predicted sign. Openness, lnop, is also statistically significant and positively signed—consistent with theory. In columns 1 through 4 we are able to estimate the impact of different types of conflict on growth, controlling for time and fixed effects. We find that the coefficient on terrorism continues to be negative and statistically significant. Moreover, the magnitude of the coefficient is much larger than in 17 As a robustness check, we have also examined numerous alternatives to the baseline shown in the table. The results are broadly similar if we consider consumption growth as the dependent variable, if we include various institutional and policy variables, if we use instruments for investment and openness, if we estimate the models using a random effects estimator rather than a fixed effects estimator, or if we modify the models’ lag structures. 20 21 0.113∗∗∗ [0.021] 0.114∗∗∗ [0.021] −0.567∗∗ [0.248] I/Yit−1 T −3.940∗∗∗ [0.842] 0.115∗∗∗ [0.021] −5.307∗∗∗ [0.462] 3 &E FULL 3.350∗∗∗ [0.445] −3.745∗∗∗ [0.840] −1.270∗∗∗ [0.243] −0.438∗ [0.247] 0.111∗∗∗ [0.021] −5.545∗∗∗ [0.462] 4 & T,I, E FULL 3.226∗∗∗ [0.444] −5.804∗∗∗ [1.230] −1.447∗∗∗ [0.306] −0.537 [0.380] 0.087∗∗∗ [0.030] −6.629∗∗∗ [0.657] 5 & T,I, E NONDEMO 3.600∗∗∗ [0.589] 0.751 [0.773] −0.945 [0.696] −0.184 [0.200] 0.344∗∗∗ [0.029] −8.018∗∗∗ [0.863] 6 & T,I, E OECD 0.142 [0.643] −0.247 [2.038] −2.156∗∗∗ [0.516] −1.194∗ [0.723] 0.075∗ [0.041] −7.042∗∗∗ [0.989] 7 & T,I, E AFRICA 3.240∗∗∗ [0.923] −0.462 [1.725] 1.591 [1.034] 0.064 [0.831] 0.282∗∗ [0.114] −15.990∗∗∗ [3.095] 8 & T,I, E MIDEAST 5.938∗∗∗ [1.952] −5.762∗∗∗ [1.507] −0.687∗∗ [0.306] −0.320 [0.400] 0.243∗∗∗ [0.048] −5.607∗∗∗ [0.920] 9 & T,I, E ASIA 1.240∗ [0.672] Obs 4172 4172 4172 4172 2540 896 1308 262 619 R-squared 0.09 0.09 0.09 0.10 0.10 0.40 0.12 0.38 0.22 Notes: robust standard errors are presented in parentheses. ∗, ∗∗ and ∗ ∗ ∗ represent statistical significance at the .10, .05 and .01 levels, respectively. All specifications include time and individual fixed effects. Models (1) through (9) are different specifications of panel growth regressions. Models (1) through (4) are the basic OLS model adding separately the different forms of conflict, i.e. terrorism (T), internal conflict (I), and external wars (E). Models (6) through (9) repeat the exercises but estimate the model over sub-samples: Non-democracies (NONDEMO), OECD countries, AFRICA, Middle East (MIDEAST) and (ASIA). Included in each regression is ln((exports+imports)/GDP) (lnopit−1 ), lagged GDP per capita (lnyit−1 ) and average investment as a percent of GDP (I/Y)it−1 . E −1.354∗∗∗ [0.242] −5.527∗∗∗ [0.463] −5.354∗∗∗ [0.463] lnyit−1 I 2 &I FULL 3.287∗∗∗ [0.445] 1 &T FULL 3.446∗∗∗ [0.446] Model Specification Sample lnopit−1 Table 4: Panel Regression: Terrorism and Growth the cross-section implying a terrorist attack reduces growth by about 0.5 percent in a given year. Analogously, internal conflict and external conflict also have much higher impacts than in the cross-sectional case. In fact, they each have a much larger impact on growth than does terrorism. Columns 5 through 10 provide the results for other sub-samples—OECD, AFRICA, ASIA, MIDEAST and NONDEMO countries. The results are broadly consistent for the Africa, Asia and non-democratic columns. However, the impact of terrorism is only statistically significant in Africa. As a robustness check regarding the measurement of the incidence of terrorism, in Table 5 we consider the results using an alternative definition, the number of incidents per capita. To this end, for every country/year, we redefine T as the number of terrorist incidents in a country during that year per 100,000 persons. Table 5 suggests that this alternative indicator yields results that are broadly consistent with those reported for our baseline indicator in Table 4. To aid the interpretation of the estimated impact of terrorism in Table 5, note that the mean value of terrorist incidents per-million is 0.2 per year, with a standard deviation is 1.0. Hence, according to the estimated coefficient on terrorism per-capita for the full sample of approximately −0.25, a one standard deviation increase in the incidence of terrorist activity (e.g. raising the number of incidents from 0.2 to 1.2 incidents per-million of population per year) is associated with a reduction in per-capita real gdp growth of about one-quarter of a percentage point. In summary, the results from our cross-country and panel regression analysis point to a common finding. Terrorism appears to have a statistically strong though economically small negative impact on growth. This remains true even when controlling for other types 22 23 0.113∗∗∗ [0.021] 0.116∗∗∗ [0.021] −0.296∗∗∗ [0.108] I/Yit−1 T −3.940∗∗∗ [0.842] 0.115∗∗∗ [0.021] −5.307∗∗∗ [0.462] 3 &E FULL 3.350∗∗∗ [0.445] −3.628∗∗∗ [0.841] −1.288∗∗∗ [0.242] −0.240∗∗ [0.108] 0.113∗∗∗ [0.021] −5.542∗∗∗ [0.462] 4 & T,I, E FULL 3.223∗∗∗ [0.444] −5.592∗∗∗ [1.230] −1.434∗∗∗ [0.304] −0.600∗∗∗ [0.210] 0.088∗∗∗ [0.030] −6.617∗∗∗ [0.656] 5 & T,I, E NONDEMO 3.581∗∗∗ [0.587] 0.811 [0.771] -0.977 [0.694] −0.513∗∗ [0.211] 0.341∗∗∗ [0.029] −7.959∗∗∗ [0.860] 6 & T,I, E OECD 0.184 [0.641] −0.141 [2.030] −2.091∗∗∗ [0.511] −3.856∗∗∗ [1.112] 0.071∗ [0.041] −6.953∗∗∗ [0.984] 7 & T,I, E AFRICA 3.326∗∗∗ [0.920] −0.519 [1.798] 1.567 [1.063] 0.036 [0.305] 0.281∗∗ [0.114] −15.977∗∗∗ [3.095] 8 & T,I, E MIDEAST 5.900∗∗∗ [1.987] −5.803∗∗∗ [1.505] −0.718∗∗ [0.305] 1.166 [0.796] 0.244∗∗∗ [0.048] −5.707∗∗∗ [0.916] 9 & T,I, E ASIA 1.235∗ [0.671] Obs 4172 4172 4172 4172 2540 896 1308 262 619 R-squared 0.09 0.09 0.09 0.10 0.11 0.40 0.12 0.38 0.22 Notes: robust standard errors are presented in parentheses. ∗, ∗∗ and ∗ ∗ ∗ represent statistical significance at the .10, .05 and .01 levels, respectively. All specifications include time and individual fixed effects. Models (1) through (9) are different specifications of panel growth regressions. Models (1) through (4) are the basic OLS model adding separately the different forms of conflict, i.e. annual terrorist incident per 1,000,000 persons (T), internal conflict (I), and external wars (E). Models (6) through (9) repeat the exercises but estimate the model over sub-samples: Non-democracies (NONDEMO), OECD countries, AFRICA, Middle East (MIDEAST) and (ASIA). Included in each regression is ln((exports+imports)/GDP) (lnopit−1 ), lagged GDP per capita (lnyit−1 ) and average investment as a percent of GDP (I/Yit−1 ). E −1.354∗∗∗ [0.242] −5.527∗∗∗ [0.463] −5.345∗∗∗ [0.463] lnyit−1 I 2 &I FULL 3.287∗∗∗ [0.445] 1 &T FULL 3.440∗∗∗ [0.446] Model Specification Sample lnopit−1 Table 5: Panel Regression: Terrorist Incident Per Capita and Growth of conflict and endogeneity concerns. However, terrorism’s drag on growth appears smaller than that of internal conflict or external war. In short, the incidence of terrorism is associated with a small, though significant, negative effect on growth. 3.2 Compositional Effects The central finding from the results reported above is that economic activity appears to be impaired by conflict. The economic mechanisms which generate the slowdown in economic activity are, however, difficult to untangle using aggregate macroeconomic data. From a theoretical standpoint, though, there are a number of possible candidates for why conflict may have an impact on economic activity. First, conflict and terrorism can have an immediately negative effect on output to the extent that it destroys production inputs. Second, conflict and terrorism can severely interrupt economic activity by disrupting household and business spending plans, which can also quickly translate into reduced output. Third, conflict and terrorism may lead to a reallocation of economic activity within a country away from more productive types of spending to spending that is intended to improve the nation’s security. Unfortunately, given the relatively poor quality of cross country data, we cannot sort through these hypotheses beyond acknowledging that they may all have harmful effects on economic activity We can, however, examine the extent to which a country’s investment rate (I/Y )—that is the ratio of investment to GDP—and government spending rate (G/Y )— that is the ratio of government spending to GDP—are affected by conflict and terrorism. A decline in the investment rate and a rise in the government spending rate would be consistent with conflict and terrorism leading to a reallocation of resources away from the accumulation of productive inputs through reduced investment spending, towards increased spending on security (and presumably less productive government activities). We present results from 24 25 −0.407∗∗ [0.184] T −0.225 [0.181] 0.521 [0.345] 2 I I/Y 3.306∗∗∗ [0.328] −0.395 [0.628] 0.558 [0.344] 3 E I/Y 3.323∗∗∗ [0.328] −0.185 [0.181] −0.357 [0.628] −0.389∗∗ [0.185] 0.496 [0.345] 4 TIE I/Y 3.307∗∗∗ [0.328] 0.848∗∗∗ [0.232] −2.141∗∗∗ [0.443] −2.238∗∗∗ [0.443] 0.490∗∗ [0.237] 6 I G/Y 3.027∗∗∗ [0.422] 5 T G/Y 2.925∗∗∗ [0.421] 1.305 [0.808] −2.278∗∗∗ [0.442] 7 E G/Y 2.960∗∗∗ [0.422] 0.796∗∗∗ [0.233] 1.178 [0.807] 0.412∗ [0.238] −2.117∗∗∗ [0.444] 8 TIE G/Y 3.043∗∗∗ [0.422] Obs 4172 4172 4172 4172 4172 4172 4172 4172 R-squared 0.12 0.12 0.12 0.12 0.10 0.10 0.10 0.10 Notes: robust standard errors are presented in parentheses. ∗, ∗∗ and ∗ ∗ ∗ represent statistical significance at the .10, .05 and .01 levels, respectively. All specifications include time and individual fixed effects. Models (1) through (8) are the basic OLS model adding separately the different forms of conflict, i.e. terrorism (T), internal conflict (I), and external wars (E). Models (1) through (4) estimate the model with I/Y as a dependent variable. Models (5) through (8) estimate the model with G/Y as a dependent variable. Included in each regression is ln((exports+imports)/GDP) (lnopit−1 ) and lagged GDP per capita (lnyit−1 ). The R-squared measure does not include excludes the contribution from the individual fixed effects. E I 0.524 [0.344] 1 T I/Y 3.337∗∗∗ [0.327] lnyit−1 Model Specification Dep Var lnopit−1 Table 6: Panel Regression: Terrorism and I/Y and G/Y this examination in Table 6. The models employed in the table are very similar to those in equation (2), except that we place the investment and government spending rates as the dependent variables, and remove the investment rate as an explanatory variable. The results in Table 6 provide some informative findings. First, the data indicate that terrorism has a strong and negative impact of about half of a percentage point on the investment to GDP ratio. Indeed, as the results in columns 1 to 4 indicate, terrorism has a negative and statistically significant effect on the investment ratio, though the other types of conflict do not. This suggests that even though terrorism has been shown to have the smallest effect on GDP growth, investment spending tends to adjust more negatively to terrorism than do other spending components of GDP. The results in columns 5 through 8, replace the investment share of GDP as the dependent variable with the government spending share of GDP. The results indicate that both terrorism and internal conflict tend to make the government spending rate rise in relation to overall economic activity, with the effect of internal conflict being approximately twice as large as that for terrorism. Notice in particular, that for terrorism the rise in the government spending ratio, approximately 0.4 percentage points, tends to offset the decline in the investment spending ratio, approximately −0.4 percentage points. Taken together, the results indicate that while overall economic activity falls in the face of terrorism, government spending seems to get crowded in while investment spending tends to get crowded out, though the effect is modest.18 18 Examination of country sub-samples (not shown) suggests that these results are strongest in AFRICA and NONDEMO countries. The results are broadly similar if we use the alternative definition of terrorism employed in Table 5. 26 3.3 A Structural VAR Model While the results in the earlier sections analyzed the impact of conflict on growth and the re-allocation of economic activity, there is a sense in which some of these influences may be confounded or mis-attributed due to the dynamic interactions between types of conflict. Indeed, given the interactions between types of conflict documented in Tables 1 and 2, parsing out the separate effects of internal conflict from terrorism on economic activity may be particularly challenging. Moreover, given the likely possibility that alternative forms of organized conflict may be strategic complements are substitutes for one another, a fuller accounting for the effects of terrorism on economic activity. Our approach is to analyze the issues within a structural vector autoregression (VAR). The benefits from this are that while we do not have direct restrictions to impose from a theoretical model, we do have a plausible identifying structure that we can test using overidentifying restrictions. Consider the following four variable VAR that includes, in order, the log-level of real GDP per-capita, as well as dummy variables for internal conflict, external conflict and terrorism.19 Such a VAR allows for economic activity to affect the probability of conflict, for economic activity to feedback onto conflict and it also allows for each type of conflict to dynamically interact with other types of conflict.20 The lag length for the VAR estimates is set at two and, as explained above, we also include fixed effects and time effects in the VAR. We label the reduced form innovations, eYt , eTt , eEt , eIt from the VAR where the residuals 19 The VAR also includes lagged investment and openness as exogenous right hand side variables. They are included to provide continuity with our panel regressions presented above. We also combine home and away external conflicts to keep the analysis more parsimonious. 20 The conflict equations in the VAR are simply dynamic linear probability equations. The coefficient estimates from these linear conflict equations are similar to those that one obtains from the marginal effects from a dynamic Probit specifications. 27 are from the output, terrorism, external conflict and internal conflict equations, respectively. It is straightforward to show that this four variable VAR can identify 6 off-diagonal elements of the variance-covariance matrix, and that by estimating fewer than 6 elements we can test the system’s over-identifying restrictions. As such, the estimation scheme will also provide a mapping from the reduced from errors, e, to the structural innovations, . Our approach to identification is two-fold. First, based on economic intuition, broad historical evidence, and the evidence presented above, conflict can clearly have an effect on economic activity within period. We incorporate this into our model through equation (3) which states that output responds to shocks in the other endogenous variables within a year. eYt = α1 · Tt + α2 · Et + α3 · It + yt (3) eTt = α4 · It + Tt (4) eEt = α5 · It + Et (5) eIt = It (6) The second identification restriction is that internal conflict is a driving force for other types of conflict, as seen in equations (4) and (5) where terrorism and external conflict respond to shocks from internal conflict. Finally, equation (6) indicates that internal conflict is the most exogenous variable in the system that depends only on itself within period. The justifications for our identification restriction are three-fold: First, there is a statistical basis for the selection. Since, we have no strong priors on an identification strategy, our approach was to examine the broadest possible set that were eligible (see Table 7). From this set, the above model not only seemed quite plausible but more importantly was the model for which the over-identifying restrictions were not rejected. Second, political theory suggests 28 that internal conflict impacts terrorism. As first described in Crenshaw (1981), terrorism may be determined by internal conflict. Third, internal conflict is quickly becoming one of the primary culprits named in the escalation of violence today. Of the 25 conflicts listed by Stockholm International Peace Institute in 2000, all but 2 were internally motivated. And policy-makers have been quick to respond. The World Bank has recently developed a research group called “The Conflict Prevention and Reconstruction Unit” to examine such issues with resources devoted from a “Post-Conflict Fund.”21 Table 7 provides the estimates of the four variable structural VAR described in expressions (3) − (6). Each column provides the estimated coefficients a1 − a5 , and standard errors for a different sample: the full sample, non-democracies, OECD, Africa, the Middle East and Asia, respectively. As well, the bottom part of the table provides the p-values from a number of tests of over-identifying restrictions: p-value 1 is the p-value from the test of the restrictions embodied in equations (3) to (6).22 The remaining p-values are for alternative specifications that allow for all shocks to conflict to have a contemporaneous effect on output, but varying combination of interactions between the conflict variables.23 There are three main findings presented in the Table 7 consistent with our earlier results. First, each form of conflict harms growth with terrorism providing the least eco21 Some of the resulting research was compiled in a special 2002 issue of Journal of Conflict Resolution entitled “Understanding Civil War.” 22 This p-value is derived from a χ2 test with 1 degree of freedom. 23 More specifically, p-value 2 is the p-value from a structural VAR model where α4 = α5 = 0 so that internal conflict does not have a contemporaneous effect on terrorism and external conflict. P-value’s 3 (4) are from structural VAR’s where conflict has a contemporaneous effect on output but only terrorism (external) conflict has a contemporaneous effect on internal and external (terrorism and internal) conflict. P-value 5 is from a structural VAR where conflict has a contemporaneous effect on output but only external and internal conflict has a contemporaneous effect on terrorism. P-value 6 is a restricted version of the model in equations (3) − (6) where a5 = 0: in other words, only innovations to internal conflict have a contemporaneous effect on innovations to terrorism. There is one degree of freedom for p-value tests 3 through 5, and two degrees of freedom for p-value tests 2 and 6. 29 Table 7: Structural VAR Evidence Sample a1 Full −0.475∗ [0.247] NONDEMO −0.765∗∗ [0.379] OECD −0.169 [0.219] AFRICA −1.524∗∗ [0.723] MIDEAST 0.029 [0.754] ASIA −0.316 [0.372] a2 − 4.307∗∗∗ [0.895] − 6.094∗∗∗ [1.282] 1.243 [1.430] −1.966 [2.327] −2.130 [1.800] − 4.615∗∗∗ [1.365] a3 −1.267∗∗ [0.525] −1.531∗∗ [0.659] − 3.232∗∗∗ [1.170] − 2.933∗∗∗ [1.130] 0.889 [1.617] −0.567 [0.694] a4 7.735∗∗ [3.344] 1.928∗∗∗ [3.518] −14.303 [18.505] 19.646∗∗ [9.045] 23.817∗ [13.447] −2.896 [7.714] a5 4.188∗∗∗ [0.923] 5.033∗∗∗ [1.035] −5.760∗∗ [2.827] 12.163∗∗ [5.765] 5.409 [5.627] 2.682 [2.085] p-value 1 p-value 2 p-value 3 p-value 4 p-value 5 p-value 6 N OBS 0.242 0.000 0.000 0.026 0.000 0.035 4019 0.376 0.000 0.000 0.001 0.000 0.005 2438 0.388 0.139 0.044 0.477 0.042 0.511 835 0.567 0.023 0.038 0.033 0.035 0.080 1267 0.569 0.222 0.308 0.071 0.336 0.177 252 0.542 0.539 0.195 0.684 0.199 0.774 592 Notes: See Table 4. The model is from a Structural VAR presented in the text, equations (3) − (6). eYt eTt eEt eIt = = = = α1 · Tt + α2 · Et + α3 · It + yt α4 · It + Tt α5 · It + Et It The e’s are the reduced form residuals for output, terrorism, external conflict and internal conflict, and are the structural shocks. P-value 1 is the p-value from the χ2 (1) test of the restrictions embodied in equations (3) to (6). P-value 2 is the p-value from a χ2 (3) test of the structural VAR model where α4 = α5 = 0. Pvalue 3 (4) is from a χ2 (1) test of a structural VAR’s where conflict has a contemporaneous effect on output but only terrorism (external) conflict has a contemporaneous effect on internal and external (terrorism and internal) conflict. P-value 5 is a χ2 (1) test from a structural VAR where conflict has a contemporaneous effect on output but only external and internal conflict has a contemporaneous effect on terrorism. P-value 6 is the χ2 (2) test from is a restricted version of the structural VAR model where a5 = 0. 30 nomically significant impact. As indicated by the estimated coefficients for α1 through α3 the estimate contemporaneous effect of terrorism, external conflict and internal conflict on output is approximately −0.5, −4.3 and −1.3 percentage points, respectively. Second, there are strong complementarities between the various forms of conflict. As indicated by the estimated coefficients for α4 and α5 , shocks to internal and external conflict lead to a significant increased probability of terrorism. This indicates that internal conflict tends to crowd in other types of conflict, including terrorism. Finally, as indicated by the p-value 1, the overidentifying restriction cannot be rejected at or below the 0.1 level of statistical significance. In sum, these findings indicate that our earlier findings are robust to allowing for dynamic effects of conflict on output as well for dynamic interactions among the conflict variables. Figure 1 provides a plot of the impulse response function for the full sample estimate of the structural VAR. The figure is arranged so that each column demonstrates the dynamic response of the row variables to a one standard deviation shock to the variable denoted at the top of the column. For example, starting from the upper most left corner, we depict a one standard deviation shock to output on output, terrorism, external conflict and internal conflict, respectively. Columns 2 through 4 continue the exercise with the shocks from terrorism, external and internal conflict, respectively. Along with the dynamic response, the 90 percent confidence interval is also plotted. These bands are computed using the technique pioneered by Sims and Zha (1999). The impulse responses in Figure 1 demonstrate a number of key findings. First, the direct negative impact from external and internal shocks to GDP is much larger and longer-lived than from terrorism. So, while the effect of terrorism on economic activity is contemporaneously negative and significant, its effects quickly dissipate even after one 31 Figure 1: Impulse Responses and 90% Error Bands (Full Sample) SHOCK LNGDP TERRORISM 6.3 EXTERNAL INTERNAL 2.4 2.4 2.4 1.6 1.6 1.6 5.4 4.5 0.8 0.8 0.8 -0.0 -0.0 -0.0 -0.8 -0.8 -0.8 -1.6 -1.6 -1.6 3.6 LNGDP 2.7 1.8 0.9 0.0 -0.9 -2.4 0 1 2 3 4 5 6 7 8 -2.4 9 2.4 0 1 2 3 4 5 6 7 8 -2.4 9 40 0 1 2 3 4 5 6 7 8 9 2.4 2.4 1.6 1.6 0.8 0.8 -0.0 -0.0 -0.8 -0.8 -1.6 -1.6 0 1 2 3 4 5 6 7 8 9 0 1 2 3 4 5 6 7 8 9 0 1 2 3 4 5 6 7 8 9 0 1 2 3 4 5 6 7 8 9 35 1.6 30 0.8 25 20 RESPONSE TERRORISM -0.0 15 -0.8 10 5 -1.6 0 -2.4 -5 0 1 2 3 4 5 6 7 8 9 -2.4 0 2.4 2.4 1.6 1.6 1 2 3 4 5 6 7 8 -2.4 9 0 1 2 3 4 5 6 7 8 9 12 2.4 10 1.6 8 0.8 0.8 0.8 6 EXTERNAL -0.0 -0.0 -0.8 -0.8 -0.0 4 -0.8 2 -1.6 -1.6 -2.4 -2.4 0 1 2 3 4 5 6 7 8 9 -1.6 0 -2 0 1 2 3 4 5 6 7 8 9 -2.4 0 2.4 2.4 2.4 1.6 1.6 1.6 0.8 0.8 0.8 -0.0 -0.0 -0.0 -0.8 -0.8 -0.8 -1.6 -1.6 -1.6 -2.4 -2.4 -2.4 1 2 3 4 5 6 7 8 9 20.0 17.5 15.0 12.5 10.0 INTERNAL 7.5 5.0 2.5 0.0 0 1 2 3 4 5 6 LNGDP 7 8 9 0 1 2 3 4 5 6 7 TERRORISM 8 9 -2.5 0 1 2 3 4 5 6 7 EXTERNAL 8 9 INTERNAL year. In contrast, the effect of external war are significant and negative for up to 3 years, while internal conflict has a negative, significant and worsening effect on output for the first few years, which continues to be significant after 6 years. Second, there are strong contemporaneous complementarities between internal conflict and terrorism but there is no feedback with external conflict. This may mean that terrorism and internal conflict provide triggers to one another providing an additional indirect influence on growth. Figure 1 shows that not only do innovations to internal conflict tend to drive up terrorism, but innovations to terrorism itself drives up internal conflict. However, shocks to external conflict do not appear to drive up other types of conflict; rather, they appear to significantly lessen the likelihood of internal conflict in the short run.24 The sub-sample estimates of the structural VAR in Table 7 provide a number of additional findings regarding the significance of non-democratic and developing countries. First, the contemporaneous impact of terrorism on output is significant only for non-democracies and Africa. In particular, the effect for Africa is extremely large. Africa is dramatically affected economically by terrorism and internal conflict, and internal conflict has a particularly strong and synergistic effect on external conflict and terrorism. This suggests that efforts directed towards quelling internal conflict may be especially valuable in Africa as a means towards improving prospects for economic growth. Second, for OECD economies, internal shocks have a much larger impact and negative contemporaneous affect on output, than does terrorism. Moreover, shocks to internal conflict tend to lessen external conflict in OECD economies, rather than increase them as in the full sample. Finally, the overidentifying restriction for the structural VAR, p-value 1, is not rejected at or below the 24 As an aside, it also appears that internal conflict falls in response to positive shocks to output—perhaps a useful lesson for those who believe that poor economic activity can trap countries into continuing levels of violence—see Blomberg, Hess and Thacker (2002). 32 10 percent level for any of the sub-samples providing strong support for our identification strategy.25 This provides indirect evidence consistent with the theory originally put forth in Crenshaw (1981). 4 Conclusion Using a unique data set that provides information on the incidence of international terrorism around the world over four decades, we have endeavored to examine some basic facts regarding the consequences of terrorism on economic activity. We have found that on average, the incidence of terrorism may have an economically significant negative effect on growth, albeit one that is considerably smaller and less persistent than that associated with either external wars or internal conflict. Further, the incidence of terrorism appears to be associated with a diversion of spending from investment towards government expenditures. We also identify differences across geographic areas and political governance both regarding the incidence as well as the likely consequences of terrorism. For advanced economies, as captured by our OECD sample, the evidence of a negative association between the incidence of terrorism and economic growth appears to be smaller and is not statistically significant. These first results, however, should be interpreted with caution. In particular, the relatively poor quality of data regarding individual terrorism incidents forces us to concentrate on measures of the incidence of terrorism that may be much more noisy than our data on the incidence of other forms of conflict, complicating comparisons. Further, to the extent the nature of terrorism has evolved in ways we cannot detect with our data, we may not be able to rely on the historical evidence with much confidence as a guide of the consequences of terrorism in the 25 While the p-values do reveal that more parsimonious and some alternative identifying schemes also pass this test, they do not do so for all sub-samples. 33 future. Nonetheless, our results suggest that the macroeconomic consequences of terrorism are potentially quite significant, confirming the need for a redoubling of public policy efforts towards examining how to best mitigate the associated risk. 34 References Alesina, Alberto and Spolaore, Enrico, 1997. “On the Number and Size of Nations,” Quarterly Journal of Economics, 112(4) 1027-1056. Acemoglu, Daron, Simon Johnson and James A. Robinson, 2001. “The Colonial Origins of Comparative Development: An Empirical Investigation.” American Economic Review, December, pp. 1369-1401. Barro, Robert, 1997. The Determinants of Economic Growth, Lionel Robbins Lectures, Cambridge and London: MIT Press. Brecher, Michael; Wilkenfeld, Jonathan; and Moser, Sheila, 1988. “Crises in the Twentieth Century,” In Handbook of International Crises, Volume I, Oxford, UK: Pergamon Books. Blomberg, S. Brock, Gregory D. Hess, and Akila Weerapana, 2004 “An Economic Model of Terrorism,” Conflict Management and Peace Science , forthcoming. Blomberg, S. Brock, Gregory D. Hess, and Akila Weerapana, 2003 “Economic Conditions and Terrorism,” European Journal of Political Economy , forthcoming. Blomberg, S. Brock, and Gregory D. Hess, 2002 “The Temporal Links Between Conflict and Economic Activity,” Journal of Conflict Resolution, 46(1), 74-90. Blomberg, S. Brock; Hess, Gregory D.; and Siddharth Thacker, 2002. “Is There a ConflictPoverty Trap?,” mimeo. Crenshaw, Martha, 1981 “The Causes of Terrorism,” Comparative Politics 13(4), 379-399. Deininger, Klaus and Lyn Squire, 1996. “A New Data Set Measuring Income Inequality.” World Bank Economic Review, November, 1996, 65-91. De La Croix, David and Matthis Doepke, 2003. “Inequality and Growth: Why Differential Fertility Matters.” American Economic Review, September, 1091-1113. 35 Dollar, David and Aart Kraay, 2003. “Institutions, Trade and Growth: Revisiting the Evidence”, World Bank Policy Research Working Papers, 3004. Easterly, William and Aart Kraay, 2000. “Small States, Small Problems? Income, Growth and Volatility in Small States”, World Development, November, 28(11): 2013-2027. Enders, Walter and Todd Sandler, 1993. “The Effectiveness of Anti-Terrorism Policies: Vector Autoregression Intervention Analysis,” American Political Science Review, 87(4), 839-44. Enders, Walter and Todd Sandler, 2002. “What do we Know About the Substitution Effect of Transnational Terrorism?” mimeo. Enders, Walter , Sandler, Todd, and Jon Cauley, 1990. “Assessing the Impact of TerroristThwarting Policies: An Intervention Time Series Approach,” Defence Economics, 2(1), 1-18. Enders, Walter, Sandler, Todd and Gerald Parise, 1992. “An Econometric Analysis of the Impact of Terrorism and Tourism,” Kyklos, 45:4, 531-54. Frankel, Jeffrey A. and David Romer, 1999. “Does Trade Cause Growth?” American Economic Review, (June) 379-399. Garfinkel, Michelle, 2003. “Global Threats and the Domestic Struggle for Power,” European Journal of Political Economy, forthcoming. Garfinkel, Michelle, 1990. “Arming as a Strategic Investment in a Cooperative Equilibrium,” American Economic Review 80(1) (March), 50-68. Grossman, Herschel I., 1991. “A General Equilibrium Model of Insurrections,” The American Economic Review 81(4) 912-921. Gurr, T.R., K. Jaggers, and W. Moore, 2003. Polity Handbook IV, 2003, University of 36 Colorado Press, Boulder. Hess, Gregory D, 2004. “The Welfare Cost of Conflict: An Empirical Assessment,” mimeo. Hess, Gregory D. and Athanasios Orphanides, 1995. “War Politics: An Economic, RationalVoter Framework,” American Economic Review, 85(4), 828-846. Hess, Gregory D. and Athanasios Orphanides, 2001a. “Economic Conditions, Elections, and the Magnitude of Foreign Conflicts,” Journal of Public Economics, 80(1), 121-140. Hess, Gregory D. and Athanasios Orphanides, 2001b. “War and Democracy,” The Journal of Political Economy, 109(4), 776-810. Judson, Ruth and Athanasios Orphanides, 1999. “Inflation, Volatility, and Growth,” International Finance, 2(1), 117-38. Keynes, John Maynard., The Economic Consequences of the Peace. London, UK: Macmillan and Co., 1919. Lapan, Harvey and Todd Sandler, 1988. “To Bargain or Not to Bargain: That is the Question,” American Economic Review Papers and Proceedings, 78:2, 16-21. Lapan, Harvey and Todd Sandler, 1993. “Terrorism and Signaling,” European Journal of Political Economy, 9:3, 383-97. Levine, R. and D. Renelt, 1992. A Sensitivity Analysis of Cross-Country Growth Regressions, American Economic Review, 82(4), 942-963. Krueger, Alan and Jitka Maleckova, 2002. “Education, Poverty, Political Violence and Terrorism: Is There a Causal Connection?”NBER Working Paper 9072, July 2002. Meade, James E., The Economic Basis of a Durable Peace. New York, NY: Oxford University Press, 1940. Mickolus, Edward; Sandler, Todd; Murdock, Jean; and Peter Flemming, 2002. “International 37 Terrorism: Attributes of Terrorist Events (ITERATE),” Vinyard Software, codebook. O’Brien, Sean P., 1996. “Foreign Policy Crises and the Resort to Terrorism: A Time Series Analysis of Conflict Linkages,” The Journal of Conflict Resolution, Vol. 40(2), 1996. Pigou, A. C., The Political Economy of War. London, UK: MacMillan and Co., 1940. Robbins, Lionel, The Economic Causes of War. London, UK: Jonathan Cape, 1942. Sims, Christopher and Zha, Tao, 1999. “Error Bands for Impulse Responses,” Econometrica, 67, 1113-1156. Skaperdas, Stergios, 1992. “Cooperation, Conflict, and Power in the Absence of Property Rights,” American Economic Review, 82(4), 720-739. Summers, Robert and Alan Heston, 1991. “The Penn World Table (Mark 5): An Expanded Set of International Comparisons,” Quarterly Journal of Economics, 106(2), 327-368. 38 CESifo Working Paper Series (for full list see www.cesifo.de) ________________________________________________________________________ 1084 Helge Berger, Jakob de Haan and Robert Inklaar, Restructuring the ECB, November 2003 1085 Lorenzo Forni and Raffaela Giordano, Employment in the Public Sector, November 2003 1086 Ann-Sofie Kolm and Birthe Larsen, Wages, Unemployment, and the Underground Economy, November 2003 1087 Lars P. Feld, Gebhard Kirchgässner, and Christoph A. Schaltegger, Decentralized Taxation and the Size of Government: Evidence from Swiss State and Local Governments, November 2003 1088 Arno Riedl and Frans van Winden, Input Versus Output Taxation in an Experimental International Economy, November 2003 1089 Nikolas Müller-Plantenberg, Japan’s Imbalance of Payments, November 2003 1090 Jan K. Brueckner, Transport Subsidies, System Choice, and Urban Sprawl, November 2003 1091 Herwig Immervoll and Cathal O’Donoghue, Employment Transitions in 13 European Countries. Levels, Distributions and Determining Factors of Net Replacement Rates, November 2003 1092 Nabil I. Al-Najjar, Luca Anderlini & Leonardo Felli, Undescribable Events, November 2003 1093 Jakob de Haan, Helge Berger and David-Jan Jansen, The End of the Stability and Growth Pact?, December 2003 1094 Christian Keuschnigg and Soren Bo Nielsen, Taxes and Venture Capital Support, December 2003 1095 Josse Delfgaauw and Robert Dur, From Public Monopsony to Competitive Market. More Efficiency but Higher Prices, December 2003 1096 Clemens Fuest and Thomas Hemmelgarn, Corporate Tax Policy, Foreign Firm Ownership and Thin Capitalization, December 2003 1097 Laszlo Goerke, Tax Progressivity and Tax Evasion, December 2003 1098 Luis H. B. Braido, Insurance and Incentives in Sharecropping, December 2003 1099 Josse Delfgaauw and Robert Dur, Signaling and Screening of Workers’ Motivation, December 2003 1100 Ilko Naaborg,, Bert Scholtens, Jakob de Haan, Hanneke Bol and Ralph de Haas, How Important are Foreign Banks in the Financial Development of European Transition Countries?, December 2003 1101 Lawrence M. Kahn, Sports League Expansion and Economic Efficiency: Monopoly Can Enhance Consumer Welfare, December 2003 1102 Laszlo Goerke and Wolfgang Eggert, Fiscal Policy, Economic Integration and Unemployment, December 2003 1103 Nzinga Broussard, Ralph Chami and Gregory D. Hess, (Why) Do Self-Employed Parents Have More Children?, December 2003 1104 Christian Schultz, Information, Polarization and Delegation in Democracy, December 2003 1105 Daniel Haile, Abdolkarim Sadrieh and Harrie A. A. Verbon, Self-Serving Dictators and Economic Growth, December 2003 1106 Panu Poutvaara and Tuomas Takalo, Candidate Quality, December 2003 1107 Peter Friedrich, Joanna Gwiazda and Chang Woon Nam, Development of Local Public Finance in Europe, December 2003 1108 Silke Uebelmesser, Harmonisation of Old-Age Security Within the European Union, December 2003 1109 Stephen Nickell, Employment and Taxes, December 2003 1110 Stephan Sauer and Jan-Egbert Sturm, Using Taylor Rules to Understand ECB Monetary Policy, December 2003 1111 Sascha O. Becker and Mathias Hoffmann, Intra-and International Risk-Sharing in the Short Run and the Long Run, December 2003 1112 George W. Evans and Seppo Honkapohja, The E-Correspondence Principle, January 2004 1113 Volker Nitsch, Have a Break, Have a … National Currency: When Do Monetary Unions Fall Apart?, January 2004 1114 Panu Poutvaara, Educating Europe, January 2004 1115 Torsten Persson, Gerard Roland, and Guido Tabellini, How Do Electoral Rules Shape Party Structures, Government Coalitions, and Economic Policies? January 2004 1116 Florian Baumann, Volker Meier, and Martin Werding, Transferable Ageing Provisions in Individual Health Insurance Contracts, January 2004 1117 Gianmarco I.P. Ottaviano and Giovanni Peri, The Economic Value of Cultural Diversity: Evidence from US Cities, January 2004 1118 Thorvaldur Gylfason, Monetary and Fiscal Management, Finance, and Growth, January 2004 1119 Hans Degryse and Steven Ongena, The Impact of Competition on Bank Orientation and Specialization, January 2004 1120 Piotr Wdowinski, Determinants of Country Beta Risk in Poland, January 2004 1121 Margarita Katsimi and Thomas Moutos, Inequality and Redistribution via the Public Provision of Private Goods, January 2004 1122 Martin Peitz and Patrick Waelbroeck, The Effect of Internet Piracy on CD Sales: CrossSection Evidence, January 2004 1123 Ansgar Belke and Friedrich Schneider, Privatization in Austria: Some Theoretical Reasons and First Results About the Privatization Proceeds, January 2004 1124 Chang Woon Nam and Doina Maria Radulescu, Does Debt Maturity Matter for Investment Decisions?, February 2004 1125 Tomer Blumkin and Efraim Sadka, Minimum Wage with Optimal Income Taxation, February 2004 1126 David Parker, The UK’s Privatisation Experiment: The Passage of Time Permits a Sober Assessment, February 2004 1127 Henrik Christoffersen and Martin Paldam, Privatization in Denmark, 1980-2002, February 2004 1128 Gregory S. Amacher, Erkki Koskela and Markku Ollikainen, Deforestation, Production Intensity and Land Use under Insecure Property Rights, February 2004 1129 Yin-Wong Cheung, Javier Gardeazabal, and Jesús Vázquez, Exchange Rate Dynamics: Where is the Saddle Path?, February 2004 1130 Alberto Alesina and Guido Tabellini, Bureaucrats or Politicians?, February 2004 1131 Gregory S. Amacher, Erkki Koskela, and Markku Ollikainen, Socially Optimal Royalty Design and Illegal Logging under Alternative Penalty Schemes, February 2004 1132 David M. Newbery, Privatising Network Industries, February 2004 1133 Charles Yuji Horioka, The Stagnation of Household Consumption in Japan, February 2004 1134 Eiji Fujii, Exchange Rate Pass-Through in the Deflationary Japan: How Effective is the Yen’s Depreciation for Fighting Deflation?, February 2004 1135 Mark M. Spiegel and Nobuyoshi Yamori, Determinants of Voluntary Bank Disclosure: Evidence from Japanese Shinkin Banks, Febrary 2004 1136 Robert Dekle and Kenneth Kletzer, Deposit Insurance, Regulatory Forbearance and Economic Growth: Implications for the Japanese Banking Crisis, February 2004 1137 Takatoshi Ito and Kimie Harada, Bank Fragility in Japan, 1995-2003, February 2004 1138 Kunio Okina and Shigenori Shiratsuka, Policy Duration Effect under Zero Interest Rates: An Application of Wavelet Analysis, February 2004 1139 Francine D. Blau and Lawrence M. Kahn, Do Cognitive Test Scores Explain Higher U.S. Wage Inequality?, February 2004 1140 Michael Rauscher, Economic Growth and Tax-Competing Leviathans, February 2004 1141 Ernst Fehr and Jean-Robert Tyran, Money Illusion and Coordination Failure, February 2004 1142 Ingo Vogelsang, Network Utilities in the U.S. – Sector Reforms without Privatization, March 2004 1143 Marc-Andreas Muendler, Estimating Production Functions When Productivity Change is Endogenous, March 2004 1144 Sascha O. Becker, Samuel Bentolila, Ana Fernandes, and Andrea Ichino, Job Insecurity and Children’s Emancipation, March 2004 1145 Pascalis Raimondos-Møller and Alan D. Woodland, Non-Preferential Trading Clubs, March 2004 1146 Robert Fenge and Matthias Wrede, EU Regional Policy: Vertical Fiscal Externalities and Matching Grants, March 2004 1147 Chi-Yung Ng and John Whalley, Geographical Extension of Free Trade Zones as Trade Liberalization: A Numerical Simulation Approach, March 2004 1148 Marc-Andreas Muendler, Trade, Technology, and Productivity: A Study of Brazilian Manufacturers, 1986-1998, March 2004 1149 Eugene Beaulieu, Vivek H. Dehejia, and Hazrat-Omar Zakhilwal, International Trade, Labour Turnover, and the Wage Premium: Testing the Bhagwati-Dehejia Hypothesis for Canada, March 2004 1150 Giorgio Brunello and Francesca Gambarotto, Agglomeration Effects on EmployerProvided Training: Evidence from the UK, March 2004 1151 S. Brock Blomberg, Gregory D. Hess, and Athanasios Orphanides, The Macroeconomic Consequences of Terrorism, March 2004
Collepals.com Plagiarism Free Papers
Are you looking for custom essay writing service or even dissertation writing services? Just request for our write my paper service, and we'll match you with the best essay writer in your subject! With an exceptional team of professional academic experts in a wide range of subjects, we can guarantee you an unrivaled quality of custom-written papers.
Get ZERO PLAGIARISM, HUMAN WRITTEN ESSAYS
Why Hire Collepals.com writers to do your paper?
Quality- We are experienced and have access to ample research materials.
We write plagiarism Free Content
Confidential- We never share or sell your personal information to third parties.
Support-Chat with us today! We are always waiting to answer all your questions.
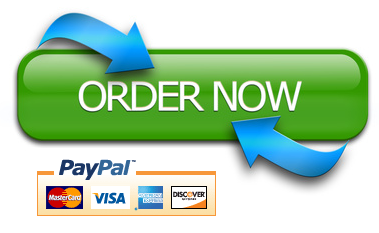