Please answer the following question in a 500 word word short essa written by yourself and unaided by an AI.? Cathy O’Neil’s Weapons of Math Destruction explores how data-driven decisi
Please answer the following question in a 500 word word short essa written by yourself and unaided by an AI.
Cathy O'Neil's Weapons of Math Destruction explores how data-driven decision making or analysis applications (algorithms, statistical tools, dashboards) tend to disenfranchise the already poor and marginalized. One of the major factors in this is the way existing data or proxies are adopted in the engineering and design process. In a 500 word short essy please identify and explain at least two potential ethical problems in the use of algorithmic applications in criminal justice settings like sentencing, recidivism risk prediction, or predictive policing. Include direct quotations from at least two different course readings, videos, or lecture slides (and cite the source indicating the author and title).
Among the course topics you might put into conversation:
O'Neil's writing on recidivism, sentencing, or predictive policing algorithms
the Loomis case in Wisconsin
Ruha Benjamin's discussion of "the new Jim Code"
Weapons of Math Desctruction
LIS 202: Week 10
Human Decision Making
● Expertise is very expensive. ● It takes a long time to develop and support and is dependent
on individual humans in a given agency. ● It can also be idiosyncratic or even biased. Also subject to
occasional error. ● Slower than computational decision making. ● But mostly is very expensive.
Most algorithmic applications are meant to replace human decision making with computational decision making to cut wages/benefits costs, increase throughput rate, and also claim benefits of data driven standardization (reduce bias, reduce error).
Criminal Sentencing, Probation, and Parole Let’s look at how O’Neil describes “recidivism score” algorithms as WMDs: (pg 27 of PDF)
Criminal Sentencing, Probation, and Parole Let’s look at how O’Neil describes “recidivism score” algorithms as WMDs: (pg 27 of PDF)
Criminal Sentencing, Probation, and Parole Let’s look at how O’Neil describes “recidivism score” algorithms as WMDs: (pg 27 of PDF)
Let’s look at the South Dakota LSI-R training PDF. https://dhs.sd.gov/servicetotheblind/docs/LSI-R%20 introductory%20training%20Participant%20Manual.pdf
Human Decision Making
Who gets to appeal to a human decider? Who gets to have a conversation a human when they need something, or when they are subject to outside opinion/decision?
When have you wanted to speak to a human and been denied?
When have you wanted a human agent to use judgment to override a rigid decision tree, rule, or pathway block?
Human Decision Making
Machine Decision Making – Scoring Model
Let’s say that instead of paying human graders we’re going to reduce the cost of college and make grades more fair and transparent by building a model to assign student scores. We also don’t want to add lots more testing on your so we’re going to mix in some predictive modeling.
Using nothing more than what we have now, what could we use to score your work and to predict your success on the material?
Machine Decision Making – Scoring Model 1. Automated quizzes (we already do this!) 2. Attendance (digital check in at lecture) 3. Automated stylistic / keyword analysis of written work 4. Student profile? Where you went to high school? Your
SAT/ACT score? Where you went to kindergarten? Where your parents went to high school? Your parents SAT score? How students “like you” have done in this class before? Age, sex, gender, ethnicity/nation of origin, wealth, zip code of parents/family, likelihood someone “like you” has cheated on assignments in classes before?
5. Length of time spent per resource on canvas? 6. Biometric data? Eyeball/face tracking in canvas or
classroom?
Wednesday – State of Wisconsin v. Loomis (2016) Defendant challenged the State of Wisconsin’s use of a closed source (blackbox) risk assessment software (COMPAS) in the sentencing him to 6 years in prison.
Algorithmic Due Process: The case alleged that using such software in sentencing violates the defendant's right to due process because it prevents the defendant from challenging the scientific validity and accuracy of such test. Also that COMPAS uses gender as a factor.
O’Neil: “these are the three elements of a WMD: Opacity, Scale, and Damage. All of them will be present, to one degree or another, in the examples we’ll be covering. Yes, there will be room for quibbles. You could argue, for example, that the recidivism scores are not totally opaque, since they spit out scores that prisoners, in some cases, can see. Yet they’re brimming with mystery, since the prisoners cannot see how their answers produce their score. The scoring algorithm is hidden.”
PSI = “pre-sentencing investigation” report
“Defendent’s statements” include questionnaire instruments like LSI-R
COMPAS = Correctional Offender Management Profiling for Alternative Sanctions
“Black box” – use of trade secrets protection to shield proprietary algorithms from public scrutiny
“Black box” – use of trade secrets protection to shield proprietary algorithms from public scrutiny
“The key to our product is the algorithms, and they’re proprietary,” said Jeffrey Harmon, Northpointe’s general manager. “We’ve created them, and we don’t release them because it’s certainly a core piece of our business. It’s not about looking at the algorithms. It’s about looking at the outcomes.”
“In Wisconsin, a Backlash Against Using Data to Foretell Defendants’ Futures” NYT, June 22, 2016
COMPAS = Correctional Offender Management Profiling for Alternative Sanctions
COMPAS = Correctional Offender Management Profiling for Alternative Sanctions
COMPAS = Correctional Offender Management Profiling for Alternative Sanctions
Whether it is immoral for the hungry to steal a loaf of bread to feed themself or their family has been at the center of moral reasoning since the 19th century, and asks of the thinker whether higher priority goes to property rights or human rights. The theft of a loaf of bread is the precipitating event that sets Victor Hugo’s 1862 novel Les Miserables.
“He hired out as a hay-maker, as laborer, as neat-herd on a farm, as a drudge. He did whatever he could. His sister worked also but what could she do with seven little children? It was a sad group enveloped in misery, which was being gradually annihilated. A very hard winter came. Jean had no work. The family had no bread. No bread literally. Seven children! One Sunday evening, Maubert Isabeau, the baker on the Church Square at Faverolles, was preparing to go to bed, when he heard a violent blow on the grated front of his shop. He arrived in time to see an arm passed through a hole made by a blow from a fist, through the grating and the glass. The arm seized a loaf of bread and carried it off.… It was Jean Valjean. This took place in 1795. Jean Valjean was taken before the tribunals of the time for theft and breaking and entering an inhabited house at night. There occur formidable hours in our civilization; there are moments when the penal laws decree a shipwreck. What an ominous minute is that in which society draws back and consummates the irreparable abandonment of a sentient being! Jean Valjean was condemned to five years in the galleys.”
COMPAS = Correctional Offender Management Profiling for Alternative Sanctions
Propublica Investigations into COMPAS:
https://www.propublica.org/article/machine-bias-risk- assessments-in-criminal-sentencing
https://www.propublica.org/article/how-we-analyzed-th e-compas-recidivism-algorithm
“A recent analysis of COMPAS's recidivism scores based upon data from 10,000 criminal defendants in Broward County, Florida, concluded that black defendants “were far more likely than white defendants to be incorrectly judged to be at a higher risk of recidivism.” Likewise, white defendants were more likely than black defendants to be incorrectly flagged as low risk. Although Northpointe disputes this analysis, this study and others raise concerns regarding how a COMPAS assessment's risk factors correlate with race.” (Loomis Decision)
Outcome:
Loomis Appealed To SCOTUS but SCOTUS declined to take up case. Leaving WI decision in place.
Outcome:
Outcome: “Although it cannot be determinative, a sentencing court may use a Correctional Offender Management Profiling for Alternative Sanctions (COMPAS) risk assessment as a relevant factor for such matters as: (1) diverting low-risk prison-bound offenders to a non-prison alternative; (2) assessing whether an offender can be supervised safely and effectively in the community; and (3) imposing terms and conditions of probation, supervision, and responses to violations. A COMPAS risk assessment may be used to enhance a judge's evaluation, weighing, and application of the other sentencing evidence in the formulation of an individualized sentencing program appropriate for each defendant. COMPAS is merely one tool available to a court at the time of sentencing and a court is free to rely on portions of the assessment while rejecting other portions.”
,
More Advance Praise for WEAPONS OF MATH DESTRUCTION
“Weapons of Math Destruction is a fantastic, plainspoken call to arms. It acknowledges that models aren’t going away: As a tool for identifying people in difficulty, they are amazing. But as a tool for punishing and disenfranchising, they’re a nightmare. Cathy O’Neil’s book is important precisely because she believes in data science. It’s a vital crash course in why we must interrogate the systems around us and demand better.”
—Cory Doctorow, author of Little Brother and co-editor of Boing Boing
“Many algorithms are slaves to the inequalities of power and prejudice. If you don’t want these algorithms to become your masters, read Weapons of Math Destruction by Cathy O’Neil to deconstruct the latest growing tyranny of an arrogant establishment.”
—Ralph Nader, author of Unsafe at Any Speed
“Next time you hear someone gushing uncritically about the wonders of Big Data, show them Weapons of Math Destruction. It’ll be salutary.”
—Felix Salmon, Fusion
“From getting a job to finding a spouse, predictive algorithms are silently shaping and controlling our destinies. Cathy O’Neil takes us on a journey of outrage and wonder, with prose that makes you feel like it’s just a conversation. But it’s an important one. We need to reckon with technology.”
—Linda Tirado, author of Hand to Mouth: Living in Bootstrap America
Copyright © 2016 by Cathy O’Neil
All rights reserved.
Published in the United States by Crown, an imprint of the Crown Publishing Group, a division of Penguin Random House LLC, New York.
crownpublishing.com
CROWN is a registered trademark and the Crown colophon is a trademark of Penguin Random House LLC.
Library of Congress Cataloging-in-Publication Data
Name: O’Neil, Cathy, author.
Title: Weapons of math destruction: how big data increases inequality and threatens democracy / Cathy O’Neil
Description: First edition. | New York: Crown Publishers [2016]
Identifiers: LCCN 2016003900 (print) | LCCN 2016016487 (ebook) | ISBN 9780553418811 (hardcover) | ISBN 9780553418835 (pbk.) | ISBN 9780553418828 (ebook)
Subjects: LCSH: Big data—Social aspects—United States. | Big data—Political aspects—United States. | Social indicators—Mathematical models—Moral and ethical aspects. | Democracy— United States. | United States—Social conditions—21st century.
Classification: LCC QA76.9.B45 064 2016 (print) | LCC QA76.9.B45 (ebook) | DDC 005.7— dc23
LC record available at https://lccn.loc.gov/2016003900
ISBN 9780553418811
Ebook ISBN 9780553418828
International Edition ISBN 9780451497338
Cover design by Elena Giavaldi
v4.1
a
THIS BOOK IS DEDICATED TO ALL THE UNDERDOGS
ACKNOWLEDGMENTS
Thanks to my husband and kids for their incredible support. Thanks also to John Johnson, Steve Waldman, Maki Inada, Becky Jaffe, Aaron Abrams, Julie Steele, Karen Burnes, Matt LaMantia, Martha Poon, Lisa Radcliffe, Luis Daniel, and Melissa Bilski. Finally, thanks to the people without whom this book would not exist: Laura Strausfeld, Amanda Cook, Emma Berry, Jordan Ellenberg, Stephen Baker, Jay Mandel, Sam Kanson-Benanav, and Ernie Davis.
CONTENTS
INTRODUCTION
CHAPTER 1 BOMB PARTS: What Is a Model?
CHAPTER 2 SHELL SHOCKED: My Journey of Disillusionment
CHAPTER 3 ARMS RACE: Going to College
CHAPTER 4 PROPAGANDA MACHINE: Online Advertising
CHAPTER 5 CIVILIAN CASUALTIES: Justice in the Age of Big Data
CHAPTER 6 INELIGIBLE TO SERVE: Getting a Job
CHAPTER 7 SWEATING BULLETS: On the Job
CHAPTER 8 COLLATERAL DAMAGE: Landing Credit
CHAPTER 9 NO SAFE ZONE: Getting Insurance
CHAPTER 10 THE TARGETED CITIZEN: Civic Life
CONCLUSION Notes About the Author
When I was a little girl, I used to gaze at the traffic out the car window and study the numbers on license plates. I would reduce each one to its basic elements—the prime numbers that made it up. 45 = 3 x 3 x 5. That’s called factoring, and it was my favorite investigative pastime. As a budding math nerd, I was especially intrigued by the primes.
My love for math eventually became a passion. I went to math camp when I was fourteen and came home clutching a Rubik’s Cube to my chest. Math provided a neat refuge from the messiness of the real world. It marched forward, its field of knowledge expanding relentlessly, proof by proof. And I could add to it. I majored in math in college and went on to get my PhD. My thesis was on algebraic number theory, a field with roots in all that factoring I did as a child. Eventually, I became a tenure-track professor at Barnard, which had a combined math department with Columbia University.
And then I made a big change. I quit my job and went to work as a quant for D. E. Shaw, a leading hedge fund. In leaving academia for finance, I carried mathematics from abstract theory into practice. The operations we performed on numbers translated into trillions of dollars sloshing from one account to another. At first I was excited and amazed by working in this new laboratory, the global economy. But in the autumn of 2008, after I’d been there for a bit more than a year, it came crashing down.
The crash made it all too clear that mathematics, once my refuge, was not only deeply entangled in the world’s problems but also fueling
many of them. The housing crisis, the collapse of major financial institutions, the rise of unemployment—all had been aided and abetted by mathematicians wielding magic formulas. What’s more, thanks to the extraordinary powers that I loved so much, math was able to combine with technology to multiply the chaos and misfortune, adding efficiency and scale to systems that I now recognized as flawed.
If we had been clear-headed, we all would have taken a step back at this point to figure out how math had been misused and how we could prevent a similar catastrophe in the future. But instead, in the wake of the crisis, new mathematical techniques were hotter than ever, and expanding into still more domains. They churned 24/7 through petabytes of information, much of it scraped from social media or e- commerce websites. And increasingly they focused not on the movements of global financial markets but on human beings, on us. Mathematicians and statisticians were studying our desires, movements, and spending power. They were predicting our trustworthiness and calculating our potential as students, workers, lovers, criminals.
This was the Big Data economy, and it promised spectacular gains. A computer program could speed through thousands of résumés or loan applications in a second or two and sort them into neat lists, with the most promising candidates on top. This not only saved time but also was marketed as fair and objective. After all, it didn’t involve prejudiced humans digging through reams of paper, just machines processing cold numbers. By 2010 or so, mathematics was asserting itself as never before in human affairs, and the public largely welcomed it.
Yet I saw trouble. The math-powered applications powering the data economy were based on choices made by fallible human beings. Some of these choices were no doubt made with the best intentions. Nevertheless, many of these models encoded human prejudice, misunderstanding, and bias into the software systems that increasingly managed our lives. Like gods, these mathematical models were opaque, their workings invisible to all but the highest priests in their domain: mathematicians and computer scientists. Their verdicts, even when wrong or harmful, were beyond dispute or appeal. And they tended to punish the poor and the oppressed in our society, while making the rich richer.
I came up with a name for these harmful kinds of models: Weapons of Math Destruction, or WMDs for short. I’ll walk you through an example, pointing out its destructive characteristics along the way.
As often happens, this case started with a laudable goal. In 2007, Washington, D.C.’s new mayor, Adrian Fenty, was determined to turn around the city’s underperforming schools. He had his work cut out for him: at the time, barely one out of every two high school students was surviving to graduation after ninth grade, and only 8 percent of eighth graders were performing at grade level in math. Fenty hired an education reformer named Michelle Rhee to fill a powerful new post, chancellor of Washington’s schools.
The going theory was that the students weren’t learning enough because their teachers weren’t doing a good job. So in 2009, Rhee implemented a plan to weed out the low-performing teachers. This is the trend in troubled school districts around the country, and from a systems engineering perspective the thinking makes perfect sense: Evaluate the teachers. Get rid of the worst ones, and place the best ones where they can do the most good. In the language of data scientists, this “optimizes” the school system, presumably ensuring better results for the kids. Except for “bad” teachers, who could argue with that? Rhee developed a teacher assessment tool called IMPACT, and at the end of the 2009–10 school year the district fired all the teachers whose scores put them in the bottom 2 percent. At the end of the following year, another 5 percent, or 206 teachers, were booted out.
Sarah Wysocki, a fifth-grade teacher, didn’t seem to have any reason to worry. She had been at MacFarland Middle School for only two years but was already getting excellent reviews from her principal and her students’ parents. One evaluation praised her attentiveness to the children; another called her “one of the best teachers I’ve ever come into contact with.”
Yet at the end of the 2010–11 school year, Wysocki received a miserable score on her IMPACT evaluation. Her problem was a new scoring system known as value-added modeling, which purported to measure her effectiveness in teaching math and language skills. That score, generated by an algorithm, represented half of her overall evaluation, and it outweighed the positive reviews from school administrators and the community. This left the district with no choice
but to fire her, along with 205 other teachers who had IMPACT scores below the minimal threshold.
This didn’t seem to be a witch hunt or a settling of scores. Indeed, there’s a logic to the school district’s approach. Admin istrators, after all, could be friends with terrible teachers. They could admire their style or their apparent dedication. Bad teachers can seem good. So Washington, like many other school systems, would minimize this human bias and pay more attention to scores based on hard results: achievement scores in math and reading. The numbers would speak clearly, district officials promised. They would be more fair.
Wysocki, of course, felt the numbers were horribly unfair, and she wanted to know where they came from. “I don’t think anyone understood them,” she later told me. How could a good teacher get such dismal scores? What was the value-added model measuring?
Well, she learned, it was complicated. The district had hired a consultancy, Princeton-based Mathematica Policy Research, to come up with the evaluation system. Mathematica’s challenge was to measure the educational progress of the students in the district and then to calculate how much of their advance or decline could be attributed to their teachers. This wasn’t easy, of course. The researchers knew that many variables, from students’ socioeconomic backgrounds to the effects of learning disabilities, could affect student outcomes. The algorithms had to make allowances for such differences, which was one reason they were so complex.
Indeed, attempting to reduce human behavior, performance, and potential to algorithms is no easy job. To understand what Mathematica was up against, picture a ten-year-old girl living in a poor neighborhood in southeastern Washington, D.C. At the end of one school year, she takes her fifth-grade standardized test. Then life goes on. She may have family issues or money problems. Maybe she’s moving from one house to another or worried about an older brother who’s in trouble with the law. Maybe she’s unhappy about her weight or frightened by a bully at school. In any case, the following year she takes another standardized test, this one designed for sixth graders.
If you compare the results of the tests, the scores should stay stable, or hopefully, jump up. But if her results sink, it’s easy to calculate the gap between her performance and that of the successful students.
But how much of that gap is due to her teacher? It’s hard to know, and Mathematica’s models have only a few numbers to compare. At Big Data companies like Google, by contrast, researchers run constant tests and monitor thousands of variables. They can change the font on a single advertisement from blue to red, serve each version to ten million people, and keep track of which one gets more clicks. They use this feedback to hone their algorithms and fine-tune their operation. While I have plenty of issues with Google, which we’ll get to, this type of testing is an effective use of statistics.
Attempting to calculate the impact that one person may have on another over the course of a school year is much more complex. “There are so many factors that go into learning and teaching that it would be very difficult to measure them all,” Wysocki says. What’s more, attempting to score a teacher’s effectiveness by analyzing the test results of only twenty-five or thirty students is statistically unsound, even laughable. The numbers are far too small given all the things that could go wrong. Indeed, if we were to analyze teachers with the statistical rigor of a search engine, we’d have to test them on thousands or even millions of randomly selected students. Statisticians count on large numbers to balance out exceptions and anomalies. (And WMDs, as we’ll see, often punish individuals who happen to be the exception.)
Equally important, statistical systems require feedback—something to tell them when they’re off track. Statisticians use errors to train their models and make them smarter. If Amazon.com, through a faulty correlation, started recommending lawn care books to teenage girls, the clicks would plummet, and the algorithm would be tweaked until it got it right. Without feedback, however, a statistical engine can continue spinning out faulty and damaging analysis while never learning from its mistakes.
Many of the WMDs I’ll be discussing in this book, including the Washington school district’s value-added model, behave like that. They define their own reality and use it to justify their results. This type of model is self-perpetuating, highly destructive—and very common.
When Mathematica’s scoring system tags Sarah Wysocki and 205 other teachers as failures, the district fires them. But how does it ever learn if it was right? It doesn’t. The system itself has determined that they were failures, and that is how they are viewed. Two hundred and six “bad” teachers are gone. That fact alone appears to demonstrate
how effective the value-added model is. It is cleansing the district of underperforming teachers. Instead of searching for the truth, the score comes to embody it.
This is one example of a WMD feedback loop. We’ll see many of them throughout this book. Employers, for example, are increasingly using credit scores to evaluate potential hires. Those who pay their bills promptly, the thinking goes
Collepals.com Plagiarism Free Papers
Are you looking for custom essay writing service or even dissertation writing services? Just request for our write my paper service, and we'll match you with the best essay writer in your subject! With an exceptional team of professional academic experts in a wide range of subjects, we can guarantee you an unrivaled quality of custom-written papers.
Get ZERO PLAGIARISM, HUMAN WRITTEN ESSAYS
Why Hire Collepals.com writers to do your paper?
Quality- We are experienced and have access to ample research materials.
We write plagiarism Free Content
Confidential- We never share or sell your personal information to third parties.
Support-Chat with us today! We are always waiting to answer all your questions.
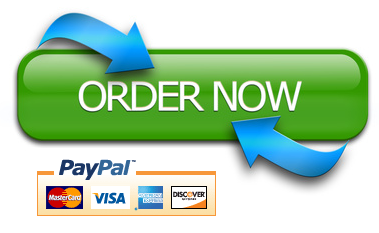