Estimating the Demand for Air Quality:New Evidence Based on theChicago Housing MarketSudip ChattopadhyayABSTRACT. This paper combines a new, largehousehold-level data set with the two-stage he-donic-estimation technique to derive new esti-mates of willingness to pay (WTP) for reduced airpollution
Use STATA to work this project!!!
Need a 6-page writing (word count at least 1800) and STATA worksheet. STATA worksheet is not count in page.
Requirements: WORD COUNT AT LEAST 1800 | .doc file
Requirements: good
Estimating the Demand for Air Quality:New Evidence Based on theChicago Housing MarketSudip ChattopadhyayABSTRACT. This paper combines a new, largehousehold-level data set with the two-stage he-donic-estimation technique to derive new esti-mates of willingness to pay (WTP) for reduced airpollution. The WTP estimates are found robustagainst functional-form specification. MarginalWTP estimates for a reduction in particulate mat-ter (PM-10) are found to be quite comparablewith some previous estimates. Benefits of non-marginal changes exhibit consistently highermonetary returns in the case of PM-10 than in thecase of SO2, signifying that households dislikeparticulate pollution more than they do sulfur.(JEL Q25)I. INTRODUCTIONMeasuring the welfare impact of environ-mental degradation, particularly air pollu-tion, using hedonic techniques has remainedan important area of empirical research in thepast few decades. The use of hedonic benefitestimates of clean air is no longer limited toaddressing welfare issues but extends to in-clude such important aspects as incorporat-ing monetary values for changes in environ-mental quality into national accounts (Smithand Huang 1995). After the theoretical paperby Rosen (1974) developing the hedonicmodel, there have been numerous empiricalstudies which estimate willingness to pay(WTP) for marginal changes in air quality.Unfortunately, studies that estimate the envi-ronmental benefits of non-marginal changesin air quality are so far, very limited. Suchestimates, which are needed to gauge thebenefits of large changes in air quality, re-quire knowledge of the parameters of theconsumer utility function. Deriving such pa-rameter estimates requires the application ofthe hedonic two-stage estimation techniqueon household-level data. The present papercombines a new, large household-level dataset with the two-stage estimation techniqueto derive new estimates of WTP for reducedair pollution.The study models the Chicago housingmarket to estimate the demand for clean airmeasured in terms of concentration of partic-ulate matter (PM-10) and sulfur dioxide(SO2). There have been a few studies that es-timate WTP for reduced air pollution in theChicago housing market (see, e.g., Atkinsonand Crocker 1987; Bender, Gronberg, andHwang 1980). But these studies have limitedappeal, for two reasons. First, the hedonicdata sets considered in these studies pertainto the 1960s or the early 1970s. Second, theestimates are only for marginal changes in airquality, which are not useful for welfareanalysis (see, e.g., Bartik 1988; Palmquist1988, for discussion of exact measurement ofwelfare). Since Chicago falls in the desig-nated non-attainment region by NationalAmbient Air Quality Standards (NAAQS)(National Air Quality Emissions Trend Re-port 1990), it is worthwhile to carry out he-donic analysis with more recent data toestimate WTP for both marginal and non-marginal changes in air quality. The presentresearch compares the new estimates of mar-ginal benefits with the estimates obtained insome previous studies. Using the estimates ofnon-marginal benefits from the second-stagehedonic regression, the study also analyzesthe size of the monetary returns to reducedThe author is with the Department of Economics,Kansas State University. He wishes to thank JanBrueckner, his thesis adviser, for advice and guidance.Thanks are due to John Braden for his comments on anearlier version of the paper and Robert Shaw at Housingand Urban Development Office, Washington, DC, forproviding the data on housing, and two anonymous ref-erees for their helpful suggestions that improved thepaper.Land Economics • February 1999 • 75 (1): 22-38
75(1)Chattopadhyay: Demand for Air Quality in Chicago23air pollution, the knowledge of which is nec-essary for important policy discussions.Two major econometric issues that mustbe addressed in reliable estimation of the he-donic housing price model are parameteridentification and the endogeneity of housingattributes. Identification is usally achieved ei-ther through functional-form restrictions orby using multiple markets (Bartik 1987; Ep-ple 1987; Palmquist 1984; Zabel and Kiel1997). This paper uses the former approach.Since valuation of housing attributes is sensi-tive to functional-form choices, this paperalso analyzes the sensitivity of the hedonicmodel to the choice of functional form. Theeffect of functional-form restrictions is ex-plored by considering six variants of a gener-alized Box-Cox form for the hedonic housingprice equation, as well as two different formsfor the utility function, namely Diewert andTranslog. The problem of endogeneity,which renders ordinary least squares esti-mates inconsistent, is tackled by applying theinstrumental variable technique (Bartik1987; Diamond and Smith 1985; Epple1987). Here, the endogeneity problem is han-dled by an application of the three-stage-least-squares (3SLS) technique.In short, the goal of the present researchis: (1) to appraise the effect of functional-form specification on hedonic two-stage esti-mation; (2) to use the results to measure theWTP for both marginal and non-marginalchanges in air quality, and analyze the sizeof returns to reduced pollution; and finally(3) to compare the estimates of marginalWTP with those of some of the previousstudies, which are based on the first-stage es-timation.The paper is organized as follows. Thenext section discusses the methodologies andassumptions involved in estimating the pa-rameters of a two-stage hedonic model. Sec-tion 3 discusses the Chicago housing sampleand the pollution status of the metropolis.Section 4 investigates the impact of func-tional-form variation. In Section 5, the esti-mates of WTP and the welfare effects ofsmall and major changes in pollution levelsare analyzed and discussed. Finally, Section6 offers concluding remarks.II. HEDONIC ESTIMATION:BASIC THEORYThe Two-Step ProcedureLet z be a vector of housing attributes iz,Zi, . . . Zn) and x be the composite good,which includes all other goods except hous-ing. Also, suppose that consumers have theutility function [/(x, z). Assuming that x is-the numeraire good and that a consumer buysonly one house, the consumer maximizeshis/her utility subject to the budget constraintX -I- P{z) = Y,[la]where Y is income and P(z) is the price of ahouse with attributes z. The consumer deter-mines his optimal choice by solving theequation[Ib]dUldxwhich says that marginal price of attribute z,equals the marginal willingness to pay(WTP), or the marginal rate of substitutionbetween attribute z, and x. The marginalWTP function on the right-hand side of [lb]is also referred to as the inverse demandfunction for attribute z,.The first stage of the Rosen’s (1974) two-step procedure involves specification of theform of the housing price function P(z) andeconometric estimation of its parameters.Marginal prices for a housing attribute arethen determined by taking the derivative ofthe hedonic price function with respect to theattribute in question. The hedonic slope,which depends on the attribute levels, can beused to measure the WTP for a small in-crease in an attribute. Measuring the exactwelfare change for a non-marginal change inan attribute involves movement along a con-sumer bid curve, away from the hedonic tan-gency. In this case, the second-stage estima-tion, which identifies the inverse demandfunction, must be carried out for exact mea-surement of welfare changes.
24Land EconomicsEebruary 1999Econometric estimation in the secondstage, however, must face the problem ofidentification of the inverse demand functionon the one hand, and the endogeneity prob-lem on the other. The problem of identifica-tion is associated with the absence of any ad-ditional information in the second stage,which may lead the second-stage estimates tobe a mere repetition of the first stage (Brownand Rosen 1982). The present study imposesfunctional-form restrictions to achieve pa-rameter identification. Under this approachthe researcher must specify both the form ofthe hedonic-price function and a (different)form for the consumer bid functions. The lat-ter choice is equivalent to specifying the util-ity function. Since valuation of housing attri-butes is sensitive to functional-form choices,the impact of functional-form variation onhedonic estimates is an issue that needs in-vestigation in order to obtain reliable esti-mates of WTP. The present research, there-fore, also analyzes the sensitivity of thehedonic model to the choice of functionalform. The effect of functional-form restric-tions is explored by considering six variantsof a generalized Box-Cox form for the he-donic-housing price equation, as well as twodifferent forms for the utility function,namely Diewert and Translog.The problem of endogeneity is associatedwith simultaneous determination of the mar-ginal price and the level of attributes (Bartik1987; Epple 1987; Mendelsohn 1984). Thus,the levels of the attributes are correlatedwith the error term in the second-stage in-verse demand equation, and as a result,ordinary least squares leads to inconsistentestimates. To overcome the endogeneityproblem, the second-stage estimation is car-ried out using 3SLS. Suitable instrumentsmust be truly exogenous and correlated withthe level of attributes, but at the same timeuncorrelated with the error term. Householdattributes such as income, square of income,number of dependent children and its square,race, and marital status are used as instru-ments in the 3SLS estimation. These vari-ables are generally considered to be corre-lated with the levels of consumption ofattributes.The Elexible Eunctional-Eorm ApproachThe most general functional form used sofar in the hedonic literature is the quadraticBox-Cox (Halvorsen and PoUakowski 1981),which has the form[2]where P(z)<«’ = [iPii)f^ – l)]/e and z*”’ =(z’^* — l)/>^. With varying choices of the trip-let {9, X, y}, several functional formsemerge. The following are a few commonlyused forms to be considered in the presentstudy:L 9 = 1, ^ = 1, Y,j = 0: linear2. 9 = 0, A, = 1, Y,j = 0: semi-log3. 9 = 0, ^ = 0, Y,j = 0: log-log4. 9 == 1, X, = 1: quadratic5. jij = 0: Box-Cox linear6. {9, X, y] unrestricted: Box-Cox qua-draticTwo forms of the utility function are con-sidered. The first is the Diewert function:Uix, z) = Vx + > (a, + b,C + 1,-I- -[3]and the second is the Translog function:, z) = log X -I- ^ (a, + 8,C + T,/?)log z,[4]P,7 log Z, log Zj,where C is the number of children in thehousehold, and R is the race of the house-holder. The coefficients a,, 8,, T,, and P.y arethe utility parameters, and k is the number ofhousing attributes. Note that because the de-
75(1)Chattopadhyay: Demand for Air Quality in Chicago25mographics C and R appear as interactionterms in [4], they affect marginal utility ofhousing attributes.With six different Box-Cox functionalforms for the housing price equation and twodifferent forms of utility function, the sec-ond-stage estimating equation has a numberof different specifications. For instance, im-posing the Box-Cox linear housing priceinunction for the left-hand side and the Diew-ert utility function for the right-hand side of[lb], the second-stage 3SLS estimating equa-tion becomes:8,C[5a]for / = 1,2, . . . , k. The error term e, in [5a]is associated with the unobserved tastes ofhouseholds and/or errors in measuring mar-ginal attribute bids. Similarly, with Box-Coxlinear housing price function and withtranslog utility function, the second-stage3SLS estimating equation has the followingform:Zi[5b]Other versions of [5] are generated by con-sidering the other forms of the hedonic-pricefunction discussed above.’ Due to nonlinearspecification of the form [5], the problem ofidentification posed in Brown and Rosen(1982) is solved. For details, readers may seeAppendix 1. To avoid the problem of errorheteroscedasticity, both sides of the equation[5a] are multipled by the term V^/x and thoseof [5b] by z,/x.Cropper, Deck, and McConnell (1988), intheir simulation experiment with a sample of200 house sales in Baltimore, apply similarfunctional-form restrictions to study the per-formance of these forms in correctly estimat-ing the true parameters. In the present study,the purpose of introducing various functionalforms of the hedonic-price function and twodifferent utility functions is, essentially, tostudy sensitivity of WTP estimates acrossspecifications.Once the inverse demand parameters areestimated, the estimates of the utility parame-ters become available, as is apparent fromequations [5a] and [5b]. Given the form ofthe utility function, the estimates of non-mar-ginal WTP can be calculated by integratingthe inverse demand function for attributes.The non-marginal WTP for an increase in Ziform z° to z] for a household h is given by^^iii^Jz,. [6]III. BACKGROUND ANDDATA SOURCESThe Chicago Housing MarketWe consider the Chicago Primary Metro-politan Statistical Area (PMSA) as the singleresidential market for the study.^ The Chi-cago PMSA includes the counties of Cook,DuPage, McHenry, and Will. In this studyonly the counties of Cook and DuPage havebeen included, primarily due to availabilityof sufficient data on air pollution in thesecounties and the proximity of these countiesto the center of the PMSA.Dwelling and household attributes. Data onactual house sales for the years 1989 and1990 were obtained from the U. S. Depart-ment of Housing and Urban Development.These housing data come from Federal’ The other versions of the system of second-stageestimating equations using linear, semilog, log-log,quadratic, and Box-Cox quadratic are not reported dueto lack of space. However, the exact expressions usingthese forms are available from the author on request.^ Since the data set in the study is for individualhouse sales, there is no need to consider the supply sideof the market in the estimation if one assumes that indi-vidual agents do not have any market power (Diamondand Smith 1985; Palmquist 1991). Thus, the supply sideof the market is ignored.
26Land EconomicsFebruary 1999Housing Administration (FHA) records onmortgages insured by FHA. The data set con-stitutes a 50% sample of all FHA-insuredtransactions by new owners in the categoryof single-family detached housing. The FHAdata set in the present study has informationon 3,044 house sales, including selling price,a wide variety of characteristics of the dwell-ing, income, family size, race, marital statusof the purchasing households, as well as thecensus tract in which the home is located.The sample consists of homes from 659 cen-sus tracts located in 103 city districts in thePMSA. A comparison of the sample house-holds with the 1990 census figures reveal thatthe median household income in the sampleis $39,780 while the corresponding censusfigure is $41,745; the median family size inthe sample is 2.80 whereas the same in thecensus is 2.55. Thus, the FHA sample in thecurrent study can be regarded as a fair repre-sentation of the population of households inthe metropolis.Air pollution variables. Air quality datawere obtained from Illinois Annual Air Qual-ity Report. These data are the ambient levelsof particulate (PM-10) and sulfur dioxide(SOj) for the years 1989 and 1990. Duringthe period of reference, Chicago had, in all,16 monitoring stations for PM-10 and 11monitoring stations for sulfur, located in dif-ferent parts of the metropolis. In 1987, EPApromulgated a new National Ambient AirQuality Standards (NAAQS) for PM-10 andsulfur, including other aspects of air quality(National Air Quality Emissions Trend Re-port 1991). The new standards for PM-10 arean expected annual arithmetic mean not toexceed 50 |J.g/m and an expected number ofcases of daily concentrations greater than150 |Xg/m^ per year in a metropolitan regionnot to exceed one. In Chicago, during 1989and 1990, the annual arithmetic mean con-centration ranged from 28 |J.g/m^ to 48 |J,g/m’ (monitoring station located at McCook,Cook County). These values were within theNAAQS limit of 50 |J.g/m However, themetropolis did not meet the NAAQs standardbased on the second criterion mentionedabove during 1990, since three of its moni-toring stations showed a 24-hour concentra-tion higher than 150 ^The new NAAQS for sulfur is a maximumof 0.03 ppm. (parts per million) annual meanconcentration. Chicago met the standard forsulfur during the reference period as thehighest annual mean was observed to be0.011 ppm. (monitoring station located atBedford Park, Cook County) during the pe-riod, but there are instances when it failed toreach the standard. For instance, in 1991, dueto localized impact of an industrial source,the sulfur level went well beyond the accept-able standard, which required immediate com-pliance action (National Air Quality EmissionsTrend Report 1991, Table 4.5).In Table 1, the statistics of annual anddaily mean concentration of PM-10 and SO2are reported for some selected non-attain-ment metropolitan statistical areas for theyear 1990.^ The annual and daily mean val-ues of these pollutants for different monitor-ing stations in Chicago are also reported inTable 2. We observe from Table 1 that ex-cept for St. Louis (82 (ig/m^), the annualmean concentration of PM-10 across the met-ropolitan areas ranged from 27 to 55 |J.g/mwhile from Table 2 the same ranged from 28to 45 M-g/m’ during 1990. The annual meanvalues within the Chicago metropolitan areaduring the period of study, that is, during1989 and 1990, however, ranged from 28 to48 |a.g/m^ The values of daily means (secondmaximum) ranged from 49 to 164 across me-tropolises, while the same ranged from 69 to149 within Chicago during 1990.The annual values of sulfur across themetropolitan areas . reported in Table 1ranged from 0.001 to 0.018 ppm. during1990, whereas the same ranged from 0.004 to0.010 within the Chicago metropolis during1990, as reported in Table 2. The annualmean values of sulfur during the period ofstudy, that is, 1989 and 1990, however,ranged from 0.003 to 0.011. The values ofdaily means (second maximum) ranged from0.003 to 0.101 across metropolises, while thesame ranged from 0.017 to 0.052 within Chi-cago during 1990. In short, variation in airquality within the Chicago metropolis is’ The original list is long and so the selection is donekeeping in mind that the majority of the non-attainmentstates are represented.
75(1)Chattopadhyay: Demand for Air Quality in Chicago27TABLE 1PEAK STATISTICS OF POLLUTION IN SELECTED METROPOLITAN AREASDURING 1990MetropolitanStatistical AreaAnaheim, CAAtlanta, GABaltimore, MDBoston, MABuffalo, NYChicago, ILCleveland, OHDetroit, MIHouston, TXIndianapolis, INKnoxville, TNLos Angeles, CAMiami, FLMinneapolis, MNNew York, NYPhiladelphia, PASt. Louis, MO-ILTucson, AZWashington, DCPM-10 (|xg/cubic meter)Annual Mean’48513338274548353038385530343738824634Daily Mean”108110117361149122114828573132491401038916411683SO2 (partsAnnual Mean”0.0030.0090.0090.0120.0110.0100.0170.0180.0090.0130.0110.0040.0010.0110.0180.0150.0150.0020.015per million)Daily Mean*”0.0100.0430.0320.0570.0810.0520.0800.0700.0390.0470.0440.0140.0030.1010.0630.0620.0640.0070.036Source: National Air Quality and Emissions Trend Report 1991. Table 4.3.” The annual mean concentration is the highest reading across monitoring stations in a metropolitanarea.”•The daily mean concentration is the second highest daily reading in 1990 across monitoring sta-tions in a metropolitan area.TABLE 2STATISTICS OE POLLUTION AT MONITORING STATIONS IN CHICAGO DURING 1990StationBlue IslandChicago-CarverChicago-CAPSChicago-FarrChicago-MayfairChicago-St. FrancisChicago-WashingtonCiceroLyonsMcCookSouth HollandSummitBensenvilleNapervilleJolietRockdalePM-10 (ng/cubic meter)Annual Mean37374039404537373845313930282933Daily Mean”9682931039514914386134121838669969480StationBedford ParkCalumet CityChicago-EdgewaterChicago-NWUChicago-SE PoliceChicago-WashingtonCiceroDes PlainesLemontLisleJolietSO2 (partsAnnual Mean0.0100.0080.0070.0070.0040.0040.0070.0040.0070.0040.004per million)Daily Mean”0.0520.0270.0210.0270.0180.0170.0330.0250.0210.0200.022′ Daily means are the second highest daily readings at different monitoring stations.
28Land EconomicsEebruary 1999comparable with national variation and thus,justifies a study of its differential impact onhouse prices.”The readings of SO2 and PM-10 in thepresent study are annual arithmetic means,obtained at different monitoring stations lo-cated around the metropolis.^ Each home hasbeen assigned the pollution readings of themonitoring station closest to it, as was donein many previous studies. Since the housesales data pertain to two years 1989 and1990, 32 pollution readings for PM-10 areavailable from 16 monitoring stations where-as 22 pollution readings of SO2 are availablefrom il monitoring stations.Neighborhood attributes. Neighborhoodcharacteristics such as median income andpercentage of white population are compiledfrom the 1990 Bureau of Census recordsavailable for each of the 659 census tracts inthe PMSA. These variables vary across cen-sus tracts and are commonly used as proxiesfor standard of living, and racial effects re-spectively, which govern a consumer’s choiceof residential location (see, e.g., Atkinsonand Crocker 1987).Public good (fiscal) attributes. Chicago isknown to have widely varying property taxrates at the level of city districts. Since fiscalattributes like the property tax rate are knownto have considerable influence on the price ofa house (Gyourko and Tracy 1991), this widevariation in property tax rates makes a goodcase for its inclusion in the hedonic priceequation.* The data on aggregate property taxrates of 103 city districts included in the sam-ple are obtained from Illinois State Depart-ment of Revenue publications.’Besides the property tax rate, Tiebout(1956) recognizes the fact that a consumershops around and selects the community thatoffers the tax-expenditure program ideal forhis/her needs. Thus, fiscal attributes such asmunicipal spending and spending by schooldistricts can also be rightfully included in thehedonic regression along with neighborhoodattributes. Oates (1969) uses these two fiscalvariables and property tax rate as explanatoryvariables in determining property values. Thevariable “spending per capita by school dis-trict” also serves as a proxy for neighbor-hood school quality in a similiar way as the”teacher-pupil ratio” used by several au-thors in the past (see, e.g., Harrison and Ru-binfeld 1978). The data on per-capita munici-pal spending were obtained for each of 103city districts from the Census of Governmentpublication on Finances of Individual Gov-ernments.*’ The data on per-capita spendingby school districts were obtained from Illi-nois State Board of Education’s Annual Sta-tistical Report.'”•* Concern has often been expressed that in Chicagolow air pollution may be associated with closeness toLake Michigan. The best way to test this is to includea locational variable such as the distance from LakeMichigan, infonnation on which is not available in thepresent study. However, the data on air pollution in thepresent study reveal that, in the case of both PM-10 aswell as SO2, some of the monitoring stations close toLake Michigan have higher annual mean reading thansome of the monitoring stations farther from it. This re-duces the chances of having air quality variables proxyfor closeness to the lake.’ Note that Chicago failed occasionally to meetshort-term standards for PM-10 and sulfur while it metthe long-term standards during the period of study. Thereason for considering annual mean concentration in thecurrent research is to compare the estimates with thoseof some earlier studies which are based on annual meanconcentration.’ In an earlier study by Atkinson and Crocker (1987)of the Chicago housing market, the actual property taxpaid by individual households was introduced as an ex-planatory variable. But such inclusion is unwarrantedsince this gives rise to simultaneity problem in estima-tion of the first-stage housing price equation, as boththe house price and the property tax paid are determinedsimultaneously.’ This aggregate rate includes rates for county, city,township, elementary school district, high school dis-trict, junior college district, fire protection district, parkdistrict, sanitary district, forest preservation district, andthe category “others.”*The major functions that are included under thegeneral head of “municipal spending” are public wel-fare, hospitals, highways, police protection, fire protec-tion, parks and recreation, housing and community de-velopment, and sewerage and sanitation.’ There are observations on property taxes and per-capita municipal spending for 103 city districts in thesample. Each census tract is first assigned the value ofthe property tax rate and the per-capita municipalspending depending on the city in which it belongs, andthen each dwelling unit is assigned a value of each ofthese variables depending on the census tract in whichit is located.'” In Chicago, city districts and the school districtsare not coterminous. There are 134 school districts inthe sample. A dwelling unit is assigned a value of per-capita spending by school district in a similar way asdiscussed in note 9.
75(1)Chattopadhyay: Demand for Air Quality in Chicago29Access attributes. House prices also dependon the access features, namely distance tomajor employment centers and transport timefrom the neighborhood where the house is lo-cated to the business district. We consider thedistance of the house from the Loop area ofdowntown Chicago as an access attribute.The other access attribute is the distance ofthe house from the nearest highway intersec-tion.Other variables. Apart from the major busi-ness district near the Loop area of downtownChicago, the O’Hare airport location hasbeen emerging as the second major employ-ment center in recent times. To account forthe effect of this second business district, adummy variable OHARE has been intro-duced to capture the effect of proximity tothe employment center near O’Hare. Also toaccount for different tax assessment practicesacross counties, another dummy variableCOOK has been introduced with the value of1 if the housing unit is in Cook County.The variable x, the annual nonhousing ex-penditure of a household, deserves specialmention. As indicated in Section 2, x(= Y —P(z)) is household expenditure on nonhous-ing consumption. Knowledge of this variableis necessary in order to carry out the second-stage estimation of the parameters of the in-verse demand functions, as can be seen fromequation [5]. Since annual nonhousing ex-penditure data are not readily available, wefirst collected FHA mortgage interest ratesprevailing during the period under study.”Since FHA mortgages are mostly 30-yearloans with zero down payments, the mort-gage payment can be calculated in a straight-forward way using the formulaP = RA(1 + R)”((1 + R)’ -where P = monthly payment, R = annualinterest/12, A = amount borrowed, A^ = 360.The monthly payment is then multiplied by12 to arrive at the annual payment for eachhousehold, which is then subtracted from thepurchaser’s annual income to arrive at thefinal figure of annual nonhousing expendi-ture X.In all, there are 22 housing, neighborhood,household, and environmental attributes tobe considered in the hedonic analysis. Thedependent variable in the hedonic-priceequation is the selling price of the house. Thedetails of all the variables, their sources,means, standard deviations, and the expectedsigns of their coefficients in the hedonic priceregression are given in Table 3.IV. EFFECT OF FUNCTIONAL-FORMVARIATIONHedonic CoefficientsThe estimates of slope coefficients underthe six Box-Cox functional forms are not re-ported due to lack of space and since they arenot comparable across specifications. How-ever, significance of coefficients and the R^are reported in Table 4, along with the meanmarginal WTP values.’^ We note here that forthe linear Box-Cox and the quadratic Box-Cox forms, different transformations are ap-plied for the dependent variables and the setof independent variable. However, the sametransformation was applied to each indepen-dent variable.’^ Variables which assume zerovalues have not been transformed. We maynote here that in the cases of linear, semi-log,log-log, and Box-Cox linear forms, all thevariables are of expected signs except forNBATH in the semi-log regression. Table 4reveals that in these four forms, except forthe variables DFNI, NBATH, and SSPEND,” The data on FHA interest rates, available on amonthly basis, are obtained for the period under studyfrom the monthly “News Release” published by theU. S. Department of Housing and Urban Development.Different monthly rates are obtained for the study pe-riod January 1989 to December 1990, and a rate is as-signed to each house depending on the month of pur-chase.’^ In the quadratic and the Box-Cox quadratic mod-els, significance of the linear terms are only reported.In general, the coefficients of the quadratic terms (y,,)are significant at acceptable levels. However, the coef-ficient (Y,J) of the cross-products terms are dropped fromthe regression since they are not significant, either indi-vidually or collectively, at any acceptable level.’^ The values of the transformation parameters in theBox-Cox linear model are 6 = 0.47, and X = 0.05,while those in the Box-Cox quadratic model are 9 =0.48, and X = 0.64.
30Land EconomicsFebruary 1999TABLE 3VARIABLE DEFINITIONS AND DATA SOURCESVariablesExpected Signs inHedonic-PriceRegression, Mean,& Std. Dev.Definitions and SourcesDependentSPRICECovariatesNROOMSLVAREAHAGEEFFLSIZEAIRCONNBATHGARAGEPTAXESPCTWHTMEDINCDFCLDFNIPARTICLEmean: 76,445std. dev.: 24,910positive mean: 6.7std. dev.: 2.7positive mean: 1,431std. dev.: 627negative mean: 28.1std. dev.: 16.4positive mean: 5,765std. dev.: 5,014positive mean: 0.89std. dev.: 0.96positive mean: 1.4std. dev.: 0.7positive mean: 1.5std. dev.: 1.3negative mean: 10.2std. dev.: 1.7positive mean: 47.3std. dev.: 28.5positive mean: 34,991std. dev.: 10,542negative mean: 17.7std. dev.: 8.9undecided mean: 1.9std. dev.: 1.7negative mean: 36.4std. dev.: 4.8Contract sales price of the house in dollar. Does not in-clude any closing cost normally chargeable to the pur-chaser.Source: FHA.Total number of habitable room enclosures.Source: FHA.Total living area in square feet.Source: FHA.Age of the house.Source: FHA.Total area of the lot in square feet.Source: FHA.If the housing has central air-conditioning = 2, if vi’indowor wall air-conditioning = 1, if no air-conditioning = 0.Source: FHA.Number of rooms in the dwelling unit having lavoratory orsink, water closet or toilet, and/or a tub or shower orboth.Source: FHA.If built-in garage = 4, if carport = 3, if not built-in garage= 2, if on-site parking = 1, if none = 0.Source: FHA.Property tax rate in the township district during the year ofpurchase.Source: Illinois Department of Revenue.Percentage of white population in 1990 in the census tract.Source: U.S. Bureau of Census. (1990)Median 1990 income of the census tract.Source: U.S. Bureau of Census (1990).Distance in tenths of a mile from the Loop area in down-town Chicago.Source: Contemporary city maps.Distance in tenths of a mile to the nearest expresswayentrance.Source: Contemporary city maps.Readings obtained for the years 1989 and 1990 from differ-ent monitoring stations in the Chicago metropolis.Source: Illinois Annual Air Quality Report.
75(1)Chattopadhyay: Demand for Air Quality in Chicago31TABLE 3VARIABLE DEFINITIONS AND DATA SOURCES (continued)VariablesExpected Signs inHedonic-PriceRegression, Mean,& Std. Dev.Definitions and SourcesSULFURSSPENDMSPENDCOOKOHAREHousehold AttributesPURINCRMARSTATNonhousing Expenditurenegative mean: 0.0065std. dev.: 0.0022positive mean: 4,776std. dev.: 353positive mean: 353std. dev.: 142positivepositivemean: 41,996std. dev.: 16,817mean: 1.1std. dev.: 1.3mean: 33,918std. dev.: 15,667Readings obtained for the years 1989 and 1990 from differ-ent monitoring stations in the Chicago metropolis.Source: Illinois Annual Air Quality Report.Operating expenses per pupil in the school district.Source: Annual Statistical Reports 1989 and 1990 of Illi-nois State Board of Education.Expenditure per capita by the municipal government during1987.Source: Census of Government 1987Dummy variable = 1 if the unit is in Cook county, if inDupage County = 0.Dummy variable = 1 if the housing unit is within 5-mileradius of O’Hare business district, and = 0 otherwise.Total annual income of the purchaser in dollars.Source: EHA.Race of the purchaser = 1 if white, and = 0 otherwise.Source: FHA.Number of dependent children in the household.Source: FHA.Marital status of the borrower.Source: FHA.Household’s annual non-housing expenditure in dollars. Ob-tained indirectly from house price and mortgage interestrate.Source for FHA mortgage rates: FHA “News Release.”all Other variables are highly significant.In particular, it is interesting to note thatthe air pollution variables PARTICLE andSULFUR are highly significant under all thefour functional forms mentioned above. In thecase of the fiscal attribute MSPEND (per-capita municipal spending), we note that theslope coefficient is consistently negative andhighly significant, contrary to expectations. Itappears that this variable may be picking upsome unmeasured neighborhood effect likecrime, which could lead to higher policespending.”’The reason for the insignificant impact ofthe access variable DFNI on the housingprice may be that DFNI measures both ac-”’ A major portion of municipal spending pertains topolice protection. There is a correlation of 0.81 betweentotal per capita municipal spending and per capitaspending on police protection. Since more police pro-tection may be associated with more crime, the case ofa negative slope may not be a mere coincidence.
32Land EconomicsEebruary 1999TABLE 4MEAN MARGINAL WTP FOR ATTRIBUTES UNDER THE SIX BOX-COX FORMSVariableNROOMSLVAREAHAGEEFFLSIZEAIRCONNBATHGARAGEPTAXESPCTWHTMEDINCDFCLDFNIPARTICLESULFUR*SSPENDMSPENDCOOKOHARELinearR^ = 0.491,325’14”-205″.382″1,885’661″1,488″-3,154″258″.653″-841″-200=-328″-883″1.271′-16’3,678’4,171 =Semi-logR^ = 0.481,401’14’-226′.377″2,366″-370’1,618″-3,290″282′.783″-990′-174=-363′-878’1.096″-16’5,369’3,681’Log-logR’ = 0.531,411’18”-414″1.841″2,580″1,828=965″-3,577″245″1.003″-1,486″-296=-283″-1,036″.867=-12’7,053″5,342″Quadratic’/?^ = 0.551,465’16’-317’1.266’2,116’601 =1,179′-2,792=226′.877′-1,235′-510=-21″-547=2.399=-25’1,077=2,638=Box-CoxLinearR^ = 0.541,474″16′-372’1.785″2,282″2,306″931′-3,404’230′.900′-1,340′-275=-268″-997′.963″-12″6,107=5,190″Box-Cox’QuadraticR^ = 0.561,519″16″-382’1.778’2,430’236=1,034′-2,571 =228″.866″-1,398′-524=-116″-550=2.628=-16″1,141 =2,103=* The WTP values are for an increase of 0.001 unit.’ significant at .1% level; ”significant at 1% level; ”significant at 5% level; ”significant at 10% level; ‘not signifi-cant at any acceptable level.’ only significance of the linear terms are indicated in the table.cess (which is positively related to the houseprice) and noise pollution due to highwaytraffic (which is negatively related to thehouse price). In short, the access-noise com-bination may be making the effect of thisvariable insignificant. Although its coeffi-cient is significant at the 10% level in mostof the models, the fact that the variableSSPEND is not highly significant may alsobe attributable to the fact that it is not an ex-act nature of school quality but rather a proxyfor the same. The insignificant impact of thevariable NBATH may primarily be due to thefact that this is highly correlated with thevariable NROOMS and LVAREA.’^In the quadratic and the Box-Cox qua-dratic models, we observe that the additionof square terms has rendered the linear coef-ficient of the variable PTAXES insignifi-cant.”” Moreover, some of the variableswhose coefficients were significant at lessthan 0.001 significance level are less signifi-cant now. In the quadratic and the Box-Coxquadratic hedonic we calculate the total ef-fect at the mean level by considering both thelinear and the quadratic terms and find thatthe signs of the total effects are all as ex-pected, except in the case of MSPEND,where it turned out negative. It may be notedthat we have the same finding in this regardin the case of linear, semi-log, log-log, andthe Box-Cox linear functional forms.Marginal WTPLet us now look at the effect of the func-tional form on mean marginal willingness topay reported in Table 4. Mean marginal WTPfor an attribute is obtained by first taking thederivative of the hedonic-price function withrespect to the attribute in question and thenaveraging the derivative values (marginalprices) over all the households in the sam-“The correlation coefficient between NROOMSand NBATH is 0.65 and that between LVAREA andNBATH is 0.82. Six Box-Cox regression were runwithout NROOMS. Little variation was noticed in theWTP estimates when NROOMS was dropped.” Please refer to note 12.
75(1)Chattopadhyay: Demand for Air Quality in Chicago33TABLE 5ESTIMATES OF UTILITY PARAMETERS UNDER THE BOX-COX LINEARHEDONIC PRICE AND DIEWERT UTILITY FUNCTION AND THEIR SIGNIFICANCEVariableNROOMSLVAREAHAGEEFFLSIZEPTAXESPCTWHTMEDINCDFCLDFNIPARTICLESULFURSSPENDMSPENDa30.21’5.36′-16.42’1.19′-184.03’0.66’1.74′-70.14′-0.78′-27.23″-747.59″-0.57″-4.02″Utility FunctionP-3.75″-0.06’1.70″-0.01″38.37″0.00001’0.0044’10.99″-0.0005’2.98″4,053.7’0.014’0.15″Parameters5-0.12′-0.01’0.03′-0.003’0.15′-0.002′-0.002’0.23’0.002′-0.004’1.03′-0.002′-0.05″T0.51″0.04’0.03’0.001’3.38″-0.064′-0.19’0.23’0.11’0.58′-9.63’0.02’0.27’Note: standard errors are not shown to reduce space.• significant at .1% level; ‘significant at 1% level; ‘significant at 5% level; ”significant at 10% level;’not significant at any acceptable level.pie.” It is encouraging to note that the meanmarginal WTP values are similar across themodels. Except for a few occasions, the mar-ginal WTP values for attributes are within 10to 20% of one another across the models. Itis encouraging to note that the marginal WTPvalues of the air pollution variables PARTI-CLE and SULFUR are very close to one an-other, except in the cases of the quadratic andBox-Cox quadratic models. We also noticethat WTP variations across the models forthe attributes NBATH, DFNI, and SSPENDare considerably more than the variations forother attributes. The result on the marginalWTP for change in PTAXES clearly contrib-utes to Gyourko and Tracy’s (1991) work oncross-city tax-rate capitalization.Utility Function Parameter Estimates andNon-Marginal WTPThe estimates of the utility parameters areobtained from the system of 18 second-stageestimating equations (as in [5]) using the3SLS technique. Due to scarcity of space, wepresent in Table 5 the utility parameter esti-mates only for the case of the Box-Cox linearhedonic-price equation and Diewert utility.The utility parameters of the discrete vari-ables AIRCON, NATH, GARAGE, COOK,and OH ARE are not reported in Table 5. Weobserve however, that while the estimatedcoefficients of the linear (a) and quadratic(P) terms of the utility function are, in gen-eral, highly significant, the coefficients ofthe interaction terms with children (8) andrace (x) are significant only in the casesof MSPEND (with C), and NROOMS,PTAXES, and PCTWHT (with R), implyingthe form of the household utility function isnot significantly affected by race and familysize.With the estimates of the utility parame-ters known, the estimates for non-marginalWTP can be calculated in a straightforwardway from the utility function using the right-hand side of equation [5].’* Using the Die-wert utility function with no cross-productterms, the non-marginal WTP for a 25%” In the case of linear Box-Cox hedonic-price func-tion marginal price = J]JP””Z}’ as given in the left-hand side of equation [5]. This function is also the mar-ginal WTP based on the first-order condition. Value ofthis function is calculated for each household in thesample. Mean marginal WTP is then calculated by aver-aging these values over all the households in the sam-ple. A similar approach is adopted for other forms ofthe hedonic price function.’* Equation [5] is for Box-Cox linear specification.For other specifications please refer to note 1.
34Land EconomicsEebruary 1999change in attribute n can he derived as fol-lows (for complete derivation please see Ap-pendix 2):dUJdx1.25a^dz,’- (a,- 4- 8,C-I- t,/? + ^,:^i)dZi = [2(a, + 8,C -I- T,^) X(V 1.25a – ^/a) -I- P,i(0.25)a]>/^ [7]where a implies the current attribute level. Ina similar manner, we derive the nonmarginalWTP with a Translog utility function as^dzi = [(a,- -I- 5,C + x,/dU.ldx+ 0.5p,,(log(1.25)+ 2 log(a))] log(1.25)x. [8]Average non-marginal WTP for a 25% in-crease in the attribute levels are calculated byaveraging the values obtained from equations[7] or [8] over all the households in the sam-ple, depending on whether the utility func-tion is Diewert or Translog. These resultsshow almost the same patterns as in the caseof marginal WTP, and they are highly com-parable across the models.” However, in thecase of PARTICLE, the WTP values turnedout positive (implying positive WTP for in-creased air pollution) under the quadratic andBox-Cox quadratic models, in disagreementwith the other models. In these two modelsin about 65% of the cases the WTP valuesare positive for PM-10 and in 35% of thecases the same are positive for sulfur. Wetherefore consider the other Box-Cox func-tional forms, namely the linear, semi-log,log-log, and the linear Box-Cox forms, foranalyzing the WTP estimates for marginaland non-marginal benefits of air quality im-provement. Non-marginal WTP for a 25%change in the air pollution variables are re-ported in Table 6 in the cases of linear, semi-log, log-log, and the linear Box-Cox formsunder the assumptions of Diewert andTranslog utility functions.^”TABLE 6ESTIMATES OF MARGINAL AND NON-MARGINALWTP FOR ENVIRONMENTAL AMENITIES UNDERTHE FOUR BOX-COX FUNCTIONAL FORMSFunctionalFormPM-10LinearSemi-logLog-logBox-Cox linearSO2*LinearSemi-logLog-logBox-Cox linearMarginalWTP-328-363-283-268-883-878-1,036-997Non-MarginalWTP(Diewert)-3,350-3,312-2,282-2,037-1,925-1,427-1,377-1,353Non-MarginalWTP(Translog)-4,329-4,651-2,794-2,729-2,084-1,873-1,633-1,649* The marginal WTP is for an increase of 0.001 unit.V. WILLINGNESS TO PAY FORENVIRONMENTAL AMENITIESA Comparison with Estimates ofPrevious StudiesEstimates of marginal as well as non-mar-ginal WTP for the air pollution variablesPM-10 and SO2 are presented in Table 6. Inthe case of PM-10 (PARTICLE), the mar-ginal impact results from an increase of onemicrogram per cubic meter (the sample lev-els range from 28 to 48 micrograms per cubicmeter). A comparison of these estimates withthose from the previous studies on particulatepollution is worthwhile. Smith and Huang(1995) present in Table 1 of their paper a listof marginal WTP estimates of 37 hedonicstudies on particulate pollution conductedbetween 1967 and 1988. Ranges of some ofthese estimates are $159 to $234 (Chicagostudy by Bender, Gronberg, and Hwang” Since the coefficients of the interaction terms ofattributes with race and children are, in general, insig-nificant, the second-stage estimation is also carried outwithout the interaction terms. It is observed that thenon-marginal WTP values with or without the interac-tion terms are quite similar.^° Due to lack of space non-marginal WTP values ofother housing attributes are not reported. These esti-mates are available from the author on request.
75(])Chattopadhyay: Demand for Air Quality in Chicago351980), $17 to $33 (St. Louis study by Ander-son and Crocker 1971), $116 to $138 (Chi-cago study by Smith 1978), $0.4 to $174(study on multiple cities by Palmquist 1984),$366 (Chicago study by Atkinson andCrocker 1982), $159 to $191 (New Yorkstudy by Appel 1980), $141 to $191 (LosAngeles study by Brucato, Murdoch, andThayer 1990). These estimates are based on1982-84 dollars. In summary, thus, the mar-ginal WTP estimates for particulate pollutionbased on these studies range from $0 to $366in 1982-84 dollars.In the present study, the marginal WTPestimates based on four Box-Cox regressionsin the case of PM-10 range from $268 to$363 in 1989-90 dollars, signifying that theaverage willingness to pay for a one unit re-duction in the pollution level of PM-10 iswithin the range of $268 and $363 in 1989-90 dollars. This goes to show that our esti-mates of marginal WTP are similar to othersin the literature. Moreover, unlike the studiesreported in Smith and Huang (1995), our es-timates are found to be robust against func-tional form variation and specificationchanges.None of the studies referred to in Smithand Huang (1995), however, extends to theestimation of non-marginal WTP for airquality improvement, which is necessary forexact measurement of welfare changes. Theaverage willingness-to-pay estimates for a25% reduction from the current level of PM-10 pollution are also reported in Table 6. Therange of estimates obtained in the four Box-Cox specifications with the Diewert utilityfunction is from $2,037 to $3,350 in 1989-90 dollars. This means that, other things be-ing the same, in 1989-90 a household wouldhave paid in the range of $2,037 to $3,350more, on average, for a house in a regionwith 25% less pollution than the regionwhere the house is actually located. Thus, ifthe government wanted to bring down PM-10 pollution in the entire region by 25%, thebenefit that would accrue to an averagehousehold would have been in the range of$2,037 to $3,350 in 1989-90 dollars.In the case of sulfur dioxide (SO2), themarginal impact results from an increase of0.001 parts per million (the sample levelsrange from 0.003 to 0.011 parts per million).From Table 6, the mean marginal willingnessto pay ranges from $878 to $1,036. The onlypublished Chicago study that estimates mar-ginal WTP for reduced sulfur pollution is byAtkinson and Crocker (1987). However, theycome up with a negative value (—$60) ofWTP based on household level data fromChicago for the period 1964-1968. Their re-sults are not comparable with the results ofour study.No existing studies carry out measurementof non-marginal benefits of reduced sulfurpollution. In our study, the average willing-ness to pay for a 25% reduction from the cur-rent level of sulfur pollution ranges from$1,353 to $1,925 in 1989-90 dollars. The es-timates are based on the four Box-Cox speci-fications and Diewert utility function. Theimplication is that, if the government wantedto bring down the sulfur dioxide pollutionlevel in the entire metropolis by 25%, the av-erage benefit that would accrue to a house-hold would have been in the range of $1,353and $1,925 in 1989-90 dollars. With theTranslog specification, the benefit measuresare slightly higher.From the benefit estimates of non-mar-ginal changes it turns out that Chicagohouseholds are, on average, willing to paymore for a reduction in PM-10 than in SO2.While both pollutants are the result of highenergy use, the former increases respiratoryillness and mortality in humans and the lattercontributes to eco-system acidification. Sincethe harmful effects of PM-10 are more directto humans, it is natural that households aremore willing to pay for its reduction. How-ever, households, in general, can hardly beexpected to know the health effects of vari-ous air pollutants, and thus it is difficult toexplain lower WTP value for sulfur. Oneplausible reason for lower WTP for sulfurcould be that particulate pollution makes theair dirtier than sulfur does, which makes theparticulate pollution more visible than sulfurpollution.VI. CONCLUSIONThe present paper applies a structural esti-mation approach involving two-stage he-donic estimation to a large household-leveldata set to derive new estimates of willing-
36Land EconomicsFebruary 1999ness to pay for air quality. The study gener-ates a number of insights. First, it shows thathedonic estimates measured at their meanscan be highly robust against functional-formspecification both in the first-stage as well asin the second-stage estimation. Additionally,benefit measures for the air pollution vari-ables are highly stable against functional-form variation.The WTP estimates for marginal changesin the particulate level obtained in the presentstudy are quite comparable with the esti-mates obtained in some earlier studies. Thestudy also provides estimates of willingnessto pay for non-marginal changes in air qual-ity which are, so far, not available in the liter-ature. Moreover, since the present study, un-like the previous ones, compares severalfunctional forms to arrive at the WTP esti-mates, the estimates are more reliable. Fi-nally, the study shows that monetary returnis consistently higher in the case of reductionin PM-10 than in the case of reduction in sul-fur, signifying that households dislike partic-ulate pollution more than they do sulfur.APPENDIX 1Iti the presetit model, the functiotial forms ofthe hedonic price futictioti atid the forms of theutility function are vastly different. Moreover, thepresence of nonlinearity in the endogeneous vari-ables, and the exogenous variables in the systemsmakes the system identifiable. This can be shownby extending the fundamental rank condition bysimply adding the nonlinear functions to the sys-tem as additional endogenous variables (but with-out increasing the number of equations). Mathe-matical treatment of handling non-linearities foridentification may be seen in Fisher (1976) andGreene (1991). Following is an example using thelinear Box-Cox hedonic price function and Die-wert utility function.Let Pi be the marginal price of an attributewhose level of consumption is Z|. f, is also themarginal WTP, in equilibrium. Then, based onequation [5a], the system of equations to be iden-tified is given by:demand shifters C and R are ignored for the pur-pose of exposition. Then augmented coefficientmatrix of this system is given by:EndogenousP, /”-e-1 Tl,-1 0coefficients.(- ^0a,Exogenouscoefficients1 Vx0 00 Pu,where the first column of coefficients of exoge-nous variables is the column of intercepts. Identi-fication of the first equation requires that the rankof the matrix of coefficients in the second equa-tion on variables excluded from the first equationshould be 2-1 = 1. This is true since rank of thevector (ai, 0, P,,) is 1. Similarly, identification ofthe second equation requires that the rank of thevector (Til, 0) is 1, which is again true. Thus, thesystem of equations is identified. This can easilybe extended to the other Box-Cox forms andutility function considered in the paper to showthat in each case the problem of identification issolved.APPENDIX 2Let Ua and U^ be the initial and the final utilitylevels for a change in an attribute, say Zi, fromlevel a to b. With Diewert utility, using equation[3] we have the following:= ^Ti, -I- (a, -I- 8,C -hk(a,. + 5,C +(a,-where P,, Z|, and Vx/z, are the set of endogenousvariables in the system and the house price P andVx are exogenously determined. The exogenous
75(1)Chattopadhyay: Demand for Air Quality in ChicagoNon-marginal WTP is equal to the reduction inX required to keep utility constant as the level ofattribute z increases. [/„ = i/j, yields= (a,- a)WTP = Ax = x» -assuming annual WTP is small compared to an-nual nonhousing expenditure (x) in the case ofhousing attributes such as environmental amenit-ies. Thus, we haveWTP = [2 (a, + h^C +Setting b = 1.25a, this gives equation [7].ReferencesAnderson, R. J., and T. D. Crocker. 1971. “AirPollution and Residential Property Values.”Urban Studies 8 (3):171-80.Appel, D. 1980. “Estimating the Benefits of AirQuality Improvement: An Hedonic Price IndexApproach Applied to the New York Metropol-itan Area.” Ph.D. diss., Rutgers University,UMI Order no. 8022540.Atkinson, S. E., and T. D. Crocker. 1982. “ABaysean Approach to Assessing the Ro-bustness of Hedonic Property Value Studies.”Manuscript. Laramie: University of Wyoming,Department of Economics.. 1987. “A Baysean Approach to Assess-ing the Robustness of Hedonic Property ValueStudies.” Journal of Applied Econometrics 2(l):27-45.Bartik, T. J. 1987. “The Estimation of DemandParameters in Hedonic Price Models.” Jour-nal of Political Economy 95 (l):81-88.. 1988. “Measuring the Benefits of Ame-nity Improvement in Hedonic Price Models.”Land Economics 64 (May): 172-83.Bender, B., T. J. Gronberg, and H. S. Hwang.1980. “Choice of Eunctional Form and theDemands for Air Quality.,” Review of Eco-nomics and Statistics 62 (4):638-43.Brown, J. N., and H. S. Rosen. 1982. “On theEstimation of Structural Hedonic Price Mod-els.” Econometrica 50 (3):765-68.Brucato, P. F., J. C. Murdoch, and M. A. Thayer.1990. “Urban Air Quality Improvements: AComparison of Aggregate Health and WelfareBenefits to Hedonic Price Differential.” Jour-nal of Environment Management 30 (6):265-79.Cropper, M. L., L. B. Deck, and K. E. McConneil.1988. “On the Choice of Functional Forms forHedonic Price Function.” Review of Econom-ics and Statistics 70 (4):668-75.Diamond, D. B., and B. A. Smith. 1985. “Simul-taneity in the Market for Housing Characteris-tics.” Journal of Urban Economics 17 (3):280-92.Epple, D. 1987. “Hedonic Prices and ImplicitMarkets: Estimating Demand and SupplyFunctions for Differentiated Products.” Jour-nal of Political Economy 87 (l):59-80.Fisher, F. 1976. The Identification Problem inEconometrics. New York: Krieger.Greene, W. H. 1991. Econometric Analysis. NewYork: Macmillan.Gyourko, J., and J. Tracy. 1991. “The Structureof Local Public Finance and the Quality ofLife.” Journal of Political Economy 99 (4):774-806.Halvorsen, R., and H. O. Pollakowski. 1981.’ ‘Choice of Functional Form for Hedonic PriceEquations.” Journal of Urban Economics 10(l):37-49.Harrison, D., and D. L. Rubinfeld. 1978. “He-donic Housing Prices and the Demand forClean Air.” Journal of Environmental Eco-nomics and Management 5 (l):81-102.Mendelsohn. R. 1984. “Estimating the StructuralEquations of Implicit Markets and HouseholdProduction Functions.” Review of Economicsand Statistics 66 (4):673-77.National Air Quality and Emissions Trend Re-port. 1990. Office of Air Quality Planning andStandard. U.S. Environmental ProtectionAgency.. 1991. Office of Air Quality Planning andStandard. U.S. Environmental ProtectionAgency.Oates, W. E. 1969. “The Effects of PropertyTaxes and Local Public Spending on PropertyValues: An Empirical Study of Tax Capitaliza-tion and the Tiebout Hypothesis.” Journal ofPolitical Economy 77 (6):959-71.Palmquist, R. B. 1984. “Estimating the Demandfor the Characteristics of Housing.” Review ofEconomics and Statistics 66 (3):394-404.. 1988. “Welfare Measurement for Envi-ronmental Improvements Using the HedonicModel: The Case of Non-parametric MarginalPrices.” Journal of Environmental Economicsand Management 15 (4):297-312.
38Land EconomicsFebruary 1999. 1991. “Hedonic Methods.” In Measur-ing the Demand for Environmental Quality,eds. J. B. Braden and C. D. Kolstad, 77-120.Amsterdam, The Netherlands: Elsevier.Rosen, S. 1974. “Hedonic Prices and ImplicitMarkets: Product Differentiation in PerfectCompetition.” Journal of Political Economy82 (l):34-55.Smith, B. A. 1978. “Measuring the Value of Ur-ban Amenities.” Journal of Urban Economics5 (4):370-87.Smith, V. K., and Ju-Chin Huang. 1995. “CanMarket Value Air Quality? A Meta-Analysisof Hedonic Property Value Models.” Journalof Political Economy 103 (l):209-27.Tiebout, C. 1956. “A Pure Theory of Local Ex-penditure.” Journal of Political Economy 64(4):414_24.Zabel, J. E., and K. A. Kiel. 1997. “Estimatingthe Demand for Clean Air in Four UnitedStates Cities.” Tufts University.
Econ 312: Modeling Project General Instructions The Modeling project for this course is intended to give you hands on experience to construct an econometric model for a real-world problem. You must keep a copy of this project to show your prospective employers to substantiate the fact that you have learnt quite a lot of econometric modeling. They will really like it in your resume. However, in this project you are not able to involve yourself in the data collection effort, which is a major learning and exciting experience in any econometric analysis. The data that are being provided to you have the features described in the following section. The modeling project Report must be typewritten, double-spaced, and must not exceed eight pages. The Report must not be in EXCEL sheet or in STATA sheet. Over and above the 8-page limit, you must attach STATA print out of the regression results as APPENDIX. On your title page, you should have the name of the course, the semester (for instance, Summer 2023), the nice title you have decided to give to your report, and your name. Data Description You are an economist at the headquarters of a major real estate company interested in the Chicago urban area. Your task is to investigate the effects of various structural, locational, access factors and factors relating to the local government spending on home value. Your programming assistant has compiled data for a randomly selected sample of about 2000 property transactions from Cook and DuPage counties of the Chicago Metropolis. The data set for this project is up on the Canvas site. You need only to download the data set assigned to you. The details of the data, such as variable descriptions, original source, units in which they are measured are available in the library or on a specific Internet site. You need to have them ready before you start working on your modeling project. Do the following: • Go to the SFSU Library website http://www.library.sfsu.edu/. • Click on the journals under Databases | Books & Media | Journals | Reserves | Illiad. • Type in the journal title “Land Economics”. • Click on the Land Economics (online) journal and then “EBSCO Environment Complete”. • Click on the year 1999 and then search for the following article: Written by Sudip Chattopadhyay in the journal Land Economics, volume 75, number 1, pp. 22-38, 1999. • When you download a PDF copy of the journal article, look for Table 3 in the article for variable definition, source, etc.
Instruction on the Modeling Project Write Up 1. Explain, in your own words, what economic issues you are addressing in the project. Explain, in your own words, why the subject may be interesting. Discuss, in specific terms, what you wish to predict or explain (the subject of your paper). Explain the dependent and each of the explanatory variables. Specify the units in which they are measured. Write down before doing any estimation, the original population regression model with SPRICE, NROOMS, LVAREA, HAGEEFF, LSIZE, PTAXES, MEDINC, DFCL, SSPEND, MSPEND in natural logarithm form. Keep the rest of the variables in unlogged form, since they have zero values in the sample (variables that take “0” values cannot be logged). Discuss how you expect each of your explanatory variables to influence the dependent variable (i.e., positive or negative relationship). You must explain why you expect so. 2.. i) State (mathematically and in words), all the assumptions you need to make in order to estimate the model. ii) Write out the estimated regression equation for the first computer run, with standard errors in parenthesis under each coefficient. Also, present statistic-F and 2Rfor the estimated model. You must use all the available explanatory variables for this run of the OLS model. iii) Interpret 2R. iv) Perform a test of the overall significance of the regression equation (F-test for the full set of regression parameters). Provide all the details of the test, including decision and conclusion. v) Perform the test to see if the variable hageeff. is statistically significant at 5% level. Provide all the details of the test. vi) Drop the insignificant variables, one at a time, by looking at the p-value from the regression results. This means you need to drop the one with the highest p-value, then run the regression, look for the highest p-value again, then drop the associated variable….and continue this way until all coefficients are significant at the 0.05 level of significance. vii) Now do the subset test (i.e., the test for linear restrictions). That is, using the full regression model from (ii) and the final model obtained in (vi), test whether the variables you dropped are significant as a group, using F-test for the subset of the explanatory variables you finally keep. Rejection of the null hypothesis would suggest that you might have dropped an important variable and you should reconsider including one or more variables you have dropped earlier. viii) Write out your final regression equation, with standard error in parentheses under each coefficient. Also, present statistic-F and2Rfor this final regression.
3. The following pertains to the revised model (i.e., after dropping all the insignificant explanatory variables), or pertains to the original model if no revisions were made: Interpret three most highly significant estimated regression coefficients in the context of the problem. Choose two explanatory variables from the final regression and construct and interpret the confidence intervals for the population coefficients of your chosen explanatory variables. 5. Conclusion State in your own words your conclusions regarding the model(s) you have estimated. Carefully review in a paragraph the original and the revised models. Discuss any problems your model might have. Do not hesitate to write the strengths and weaknesses of your model and your results. Finally, offer any interesting implications of your findings that you might convey to your boss in a non-technical way. 4. Complete Report (8 pages maximum) and Appendix printouts Write out the complete report in maximum of 8 pages. Attach as pages 9, 10, etc. the STATA printout of the full-set and the final regressions, to the report. No data set print out please. Write your name on each page of the printout AND MAKE A PDF COPY OF THE ENTIRE REPORT INCLUDING THE APPENDIX. Upload the PDF copy of the report on Canvas.
Collepals.com Plagiarism Free Papers
Are you looking for custom essay writing service or even dissertation writing services? Just request for our write my paper service, and we'll match you with the best essay writer in your subject! With an exceptional team of professional academic experts in a wide range of subjects, we can guarantee you an unrivaled quality of custom-written papers.
Get ZERO PLAGIARISM, HUMAN WRITTEN ESSAYS
Why Hire Collepals.com writers to do your paper?
Quality- We are experienced and have access to ample research materials.
We write plagiarism Free Content
Confidential- We never share or sell your personal information to third parties.
Support-Chat with us today! We are always waiting to answer all your questions.
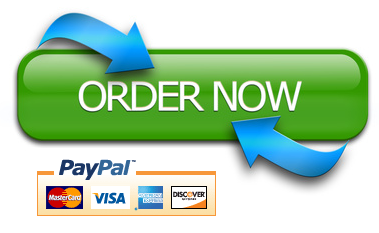