Complete the final research proposal document for AI Based Predictive Maintenance for Semiconductor Manufacturing Processes ? My topic is AI Based Predictive Maintenance For Semic
Complete the final research proposal document for AI Based Predictive Maintenance for Semiconductor Manufacturing Processes
- My topic is AI Based Predictive Maintenance For Semiconductor Manufacturing Processes
- Please fill all the required fields in that sample document (The document is just a sample template
- Go through it and let me know if you have questions09:45
- Also, you need to fill Week_6_Assign_5_Citation_and_References_Exercise.docx
- You need to complete the final research proposal document named Research_Proposal_Final.docx
Instructions |
List Keywords used in search |
Number of Results |
Search using your original Topic |
Predictive maintenance, Semiconductor manufacturing, |
13 |
Add additional focused keywords based on the guidance for narrowing or broadening |
IOT, predictive analytics, manufacturing (Broadening) |
20 |
Add additional focused keywords based on the guidance for narrowing or broadening |
PdM Fab-Wide Solution Architecture, classifier approach, Machine learning, semiconductor equipment (Narrowing) |
9 |
Add additional focused keywords based on the guidance for narrowing or broadening |
Predictive maintenance, IOT, AI, Fab equipment, Semiconductor manufacturing, PdM Fab-Wide Solution Architecture, classifier approach, Machine learning, (Narrowing) |
7 |
Add additional focused keywords based on the guidance for narrowing or broadening |
Planned maintenance, Semiconductor Fab, Semiconductor manufacturing equipment, Equipment data for detection and fault reduction, fault classification, predictive maintenance, PdM Fab-Wide Solution Architecture, classifier approach, Machine learning, (Narrowing) |
6 |
1) Please list three (3) insights that you have noted about your research topic through this exercise.
Predictive maintenance can be of huge advantage in manufacturing, especially in semiconductor industry where a small equipment breakdown can affect billions of dollars’ worth of chip production. While doing some research on refining the topic, I learnt few key things mentioned below
1. Predictive maintenance can identify the equipment failures ahead by analyzing the trained models and can assure the reliability and uptime of the manufacturing equipment
2. Predictive maintenance reduces the overall cost and increases the efficiency of the fabrication unit by scheduled maintenance rather than unplanned downtimes, catastrophic failures. Sudden equipment failures can cause billions of production losses.
3. Predictive maintenance uses machine learning and Artificial Intelligence algorithms to analyze the data collected from network of IoT sensors that give information about performance data like pressure, temperature, flow, current, power, vibration of the equipment and to provide a prediction about the expected downtime of that machinery. This prediction will help the company to understand anticipated downtimes and can plan the chip manufacturing maintenance ahead.
2) Based on these preliminary searches, has the focus of your research changed? In what way?
Not really. The focus of my research since the beginning has been the predictive maintenance of the semiconductor manufacturing equipment. But this exercise has really helped me a lot in understanding the importance of keyword search in refining the topic, getting more specific information, on how to narrow down the focus of a particular topic. It helped me in getting familiarized with content search on various Library platforms like HU, MIT and Google Scholar.
3) Have you identified any gaps in the existing literature or recommendations for future research? (These are often found in the conclusion section of articles and research studies)
Although AI based predictive maintenance has significant benefits, there are some gaps in there. There are some challenges including limited availability of labeled data, data quality/reliability issues, current AI models are industry specific, scalability and real-time integration challenges.
4) How does your proposed topic take a different/new approach or add to the existing body of knowledge?
In my proposed research, I would like to address the challenges/gaps existing in current literature, thus improvising the predictive maintenance methodology. This includes proper preprocessing of data to identify missing data, remove the outliers, noise, and biases. Portability of trained models across various industries, transferring learning techniques can be explored. Scalability and real-time implementation that includes processing huge amounts of streamed real-time data from the sensors and analyzing it for the better predictions based on the real-time data.
Page 1 of 2
,
Assignment 1 – Week 1 – “Selecting A Research Topic”
Item |
Question |
Your Response to Question |
1 |
If you could put a “title” to your Topic, what would be that title? |
AI Based Predictive Maintenance for Semiconductor Manufacturing Process |
2 |
Why did you select this topic? |
This topic piqued my interest as I’m currently working in Semiconductor Industry. This research topic would be inline with my current work. |
3 |
What specifically do you want to research or develop (and become expert on) regarding this topic? |
AI integration in Semiconductor manufacturing, IoT techniques, data analytics Improved Productivity and Reducing to-the-market time |
4 |
How will your research benefit your field of study and the overall “body of knowledge” on your topic? Why is your proposed work important? |
This research would benefit my current work as it reduces the maintenance cost, increase productivity, safety and it will improve the manufacturing equipment uptime in semiconductor industry |
5 |
Post links to five (5) research articles related to your considered topic: |
|
Link to research article 1: Decision Making in Predictive Maintenance: Literature Review and Research Agenda for Industry 4.0 by Alexandros Bousdekis https://www.sciencedirect.com/science/article/pii/S2405896319311772Link to research article 2: "Artificial Intelligence for Predictive Maintenance” by H.Kamel https://iopscience.iop.org/article/10.1088/1742-6596/2299/1/012001/pdf Link to research article 3: “Predictive Maintenance – Bridging Artificial Intelligence and IoT” by Gerasimos G. Samatas, Seraphim S. Moumgiakmas, George A. Papakostas https://arxiv.org/ftp/arxiv/papers/2103/2103.11148.pdf Link to research article 4: “Big Data Capabilities Applied to Semiconductor Manufacturing Advanced Process Control” by J. Moyne, Jamini Samantaray, M. Armacost https://www.semanticscholar.org/paper/Big-Data-Capabilities-Applied-to-Semiconductor-Moyne-Samantaray/80df1aa2f048fec2017277af9b880b63cdfc0025Link to research article 5: Modelling and analysis of semiconductor manufacturing in a shrinking world: Challenges and successes https://www.researchgate.net/publication/229424061_Modelling_and_analysis_of_semiconductor_manufacturing_in_a_shrinking_world_Challenges_and_successes |
||
6 Finally…to gain expanded scope and learn from your peers, plan to carefully listen to o ther students’ Topic ideas. They may provide helpful insights into their selected topic. |
Page 1 of 1
,
Name of Literature Review 1: Predictive maintenance in the Industry 4.0: A systematic literature review
Link to Literature Review 1: https://www.sciencedirect.com/science/article/pii/S0360835220305787
Summary of Literature Review 1 (250 words): These days majority of the industries are going towards new technological revolution called Industry 4.0. Industry 4.0 transforms the businesses and manufacturing processes by integration and automation of new technologies like Big Data, Data Analytics, Artificial Intelligence (AI), Internet of Things (IoT), Cloud computing to significantly increase the productivity and efficiency. Predictive Maintenance (PdM) is an integrated approach to proactively predict/anticipate and prevent the equipment failures before they occur using predictive analytics. Predictive maintenance (PdM) collects the real-time operational data from the manufacturing equipment sensors and using machine learning models it helps to accurately predict the equipment failures. This significantly helps in reducing the unplanned downtime, optimizing the manufacturing maintenance planning schedules, minimizing the cost. The article focusses on the importance of PdM in the manufacturing processes. Maintenance accounts to 30-60% of the total operating costs of manufacturing equipment, hence unplanned breakdown of the equipment can halt the entire manufacturing process and can significantly impact the production and the repairing/operating costs. There is an old saying, ‘Prevention is always better than cure’, the similar applies to the manufacturing processes as well. It is always better to predict the equipment failures and proactively fix it or schedule a maintenance rather than the unexpected/sudden downtimes. The literature focusses on the various predictive maintenance models like Physical model-based, knowledge-based, and data-driven. The article talks about the implementation of PdM and methodologies are aimed at improvising the manufacturing capabilities, mitigating the unscheduled downtime impacts, reducing the overall cost and maximizing the efficiency of the manufacturing equipment.
Gaps in Literature/ Future Research Ideas: While the there are advantages with the predictive maintenance (PdM), there are some gaps and challenges that need to be addressed. Predictive maintenance hugely relies on the data collected from the sensors integrated into manufacturing equipment. Data inconsistency, missing data, data class imbalances hinder the accuracy of prediction in PdM. Collecting huge amount of data from various equipment, storage and handling can be challenging. Scalability and Interoperability of ML models to handle data from various equipment can be challenging. Data collected from different equipment can be in different formats, developing Machine Learning (ML) algorithms to handle and integrate these formats can be bit challenging with increased amounts of data.
Application to your research: This literature review provides an interesting insight on the importance of Predictive Maintenance (PdM) with significantly increasing industrial move towards Industry 4.0. It gives an idea about various ML data modelling models and methodologies. This will help in choosing the correct method while building the ML model that best fits to handle the data pertaining to semiconductor manufacturing process in turn providing the accurate prediction about the equipment failures and downtimes.
Name of Literature Review 2: Optimal Predictive Maintenance Technique for Manufacturing Semiconductors using Machine Learning
Link to Literature Review 2: https://ieeexplore.ieee.org/stamp/stamp.jsp?tp=&arnumber=10075658
Summary of Literature Review 2 (250 words): The competition in the semiconductor industry is intensifying day-by-day, hence there is significant need to increase the efficiency and productivity. This literature review focus on building various manchine learning model techniques to perform analysis on the computational data collected from the sensors to accurately predict the failures in the wafer manufacturing and to predict the semiconductor manufacturing equipment downtime, helping to mitigate the production losses that are caused due to sudden equipment failures and unpredicted, unexpected equipment shutdowns. This literature review provides an insight about various ML model building technique like Random Forest Classifier, Support Vector Machine, Decision Tree Classifier, Logistic Regression, Extreme Gradient Boost, and Neural Networks, and their respective accuracy in predicting the equipment downtime. Random Forest Classifier technique provides the highest accuracy among all the ML Model building techniques with an accuracy of 94%. Random Forest model can better handle the complex data by generalizing well to unseen data. Predictive Analysis on numerous datasets from the semiconductor manufacturing sensors have been modelled using ML models built using Random Forest Classifier have significantly improved the accuracy in the prediction.
Gaps in Literature/ Future Research Ideas: Even though there are multiple machine learning models like Random Forest Classifier, Support Vector Machine, Decision Tree Classifier, Logistic Regression, Extreme Gradient Boost, and Neural Networks etc, one generic model can’t be used in common across all the industries. Choosing the model that best fits the specific industry needs and address specific industry challenges is crucial in getting the better accuracy of prediction.
Application to your research: This literature review provides an interesting insight on various Machine Learning Model building techniques with their respective predictive accuracy. This will really help me to decide on the right technique in building my machine learning model
Name of Literature Review 3: Machine learning-based techniques for fault diagnosis in the semiconductor manufacturing process
Link to Literature Review 3: https://eds.p.ebscohost.com/eds/detail/detail?vid=0&sid=5c02f5f2-c279-4677-958f-11839a5d91c3%40redis&bdata=JkF1dGhUeXBlPXNzbyZzaXRlPWVkcy1saXZlJnNjb3BlPXNpdGU%3d#AN=edssjs.A8FB77B4&db=edssjs
Summary of Literature Review 3 (250 words): This paper focusses on the use of Machine Learning models for the predictive maintenance in the semiconductor manufacturing process. As the industries are going towards the fourth industrial revolution (Industry 4.0), the ML models are used extensively for early fault diagnosis and predictive maintenance. The quality of dataset plays a crucial role in the building the ML models, when the dataset has some missing values, noisy data, or class imbalance problems, it becomes harder to accurately predict the minority class due to majority class. Literature review focus on the Synthetic Data Generation Technique (SDGT) to alleviate this class imbalance problem in the dataset. This technique tries to eliminate the problems with missing values, noisy dataset, and the dataset imbalances. This technique implemented ML model proves an accuracy of 99.5 to 100% accuracy in the prediction.
Gaps in Literature/ Future Research Ideas: One of the problems exist with the current ML models is noisy/missing values dataset. Addressing this gap with the input Dataset plays a key role in building robust machine learning model to accurately provide the prediction.
Application to your research: This literature review provides an interesting insight about Synthetic Data Generation Techniques (SDGT) to address the issues with the dataset like missing data, noisy data, and class imbalances in the dataset. This is very good method to provide a high-quality dataset to build an accurate ML model.
Name of Literature Review 4 Planned Maintenance Schedule Update Method for Predictive Maintenance of Semiconductor Plasma Etcher
Link to Literature Review 4: https://ieeexplore.ieee.org/stamp/stamp.jsp?tp=&arnumber=9377534
Summary of Literature Review 4 (250 words): This paper explains a fault prediction method used in predictive maintenance of Plasma Etcher Equipment in a Semiconductor fabrication plant. Rather than waiting for equipment failure and performing maintenance work on semiconductor etchers, this method proposes using a Machine Learning algorithm to work on a dynamic data set; this data comes from sensors connected with wafers during the etching process. The method is known as Predictive Maintenance (PdM) with the objective to calculate the Remaining Useful Life (RUL) of the equipment. The wafer-etching monitoring sensor data from all the etchers are collected and is used to calculate a feature value, based on the feature value and the cost of replacement, maintenance is scheduled. There are several factors considered while predicting the next optimal time for a maintenance cycle, some of which are time required for maintenance including time taken in shutting down the chambers and then starting them up again along with cost of the wafer scrap due to maintenance and cost of equipment being replaced. The paper explains how statistical analysis is applied considering the feature value and cost of maintenance based on the lifetime data for etcher components, the calculations involve taking the actual life (average life from one maintenance to next) of all the etchers, dividing by the mean and then normalized, a Weibull distribution is created with this data which is used in failure modeling. The result section of the paper suggests that there is an overall 71% reduction in maintenance cost compared to conventional maintenance practices that are followed in semiconductor manufacturing practices, which is very promising.
Gaps in Literature/ Future Research Ideas: In this paper, the details of specific sensor used in the study is not mentioned along with details of the silicon wafer being worked on, moreover the results are shared in percentage only and not actual cost or downtime reduction with this method.
Application to your research: This paper gives insights on utilizing machine learning algorithms during semiconductor etching process and also mentions that the same technique of predictive maintenance can be expanded onto other verticals of the semiconductor manufacturing process.
Page 1 of 1
,
Topic: AI-Based Predictive Maintenance Systems for Semiconductor Manufacturing Processes
Getting Started with Your Problem Statement
There is a problem with predicting maintenance and equipment failures in semiconductor manufacturing processes (topic).
Despite advancements in data analytics and machine learning, accurately and precisely predicting the maintenance needs and equipment failures in the Semiconductor manufacturing processes is not effectively achieved. (Something that should be happening), In practice, the maintenance decisions are often reactive approaches rather than proactive, data-driven predictions. The accuracy of predicting maintenance and equipment failures in semiconductor industry is not consistently high, still there is room for improvement. [1]
(Occurring provide the supporting research).
This problem has negatively affected the overall productivity and efficiency of the semiconductor manufacturing process. The unexpected equipment failures and unplanned downtime can cause billions of dollar production loss, it also increases the maintenance cost in semiconductor fabrication facilities. In current times, semiconductor manufacturers are often following reactive maintenance practices rather than proactive, that results in reduced overall productivity and efficiency (victims).
A possible cause of this problem is the complexity and dynamic nature of the semiconductor manufacturing processes. Various parameters such as highly dynamic operating conditions, complex/intricate interactions among equipment and inaccurate, limited availability of the data from the equipment sensors make it difficult to build accurate machine learning models, thus contributing to the challenges in achieving reliable predictions. [2]
If we don’t solve it, semiconductor manufacturers will continue to experience unexpected interruptions in their production processes in turn causing increased maintenance costs, decreased equipment reliability. This will negatively impact the competitiveness, profitability, productivity and impacting the ability to meet the customer demands effectively. (Will happen consequence).
Perhaps research that investigates advancements in machine learning algorithms, improving the quality of the input data, following methods like Synthetic Data Generation Techniques (SDGT) and integrating high reliability sensors to collect real-time data from the semiconductor manufacturing equipment could remedy this situation. Undertaking these measures can significantly increase the accuracy and effectiveness of the predictions thus reducing equipment downtimes and improving maintenance strategies.
Resources that Support this Problem Statement (Provide two of your best resources including links)
1. Optimal Predictive Maintenance Technique for Manufacturing Semiconductors using Machine Learning
https://ieeexplore.ieee.org/document/10075658
2. Machine learning-based techniques for fault diagnosis in the semiconductor manufacturing process
My Problem Statement/ Question
There is a problem with predicting maintenance and equipment failures in semiconductor manufacturing processes (topic).
Despite advancements in data analytics and machine learning, accurately and precisely predicting the maintenance needs and equipment failures in the Semiconductor manufacturing processes is not effectively achieved. (Something that should be happening), In practice, the maintenance decisions are often reactive approaches rather than proactive, data-driven predictions. The accuracy of predicting maintenance and equipment failures in semiconductor industry is not consistently high, still there is room for improvement. [1]
(Occurring provide the supporting research).
This problem has negatively affected the overall productivity and efficiency of the semiconductor manufacturing process. The unexpected equipment failures and unplanned downtime can cause billions of dollar production loss, it also increases the maintenance cost in semiconductor fabrication f
Collepals.com Plagiarism Free Papers
Are you looking for custom essay writing service or even dissertation writing services? Just request for our write my paper service, and we'll match you with the best essay writer in your subject! With an exceptional team of professional academic experts in a wide range of subjects, we can guarantee you an unrivaled quality of custom-written papers.
Get ZERO PLAGIARISM, HUMAN WRITTEN ESSAYS
Why Hire Collepals.com writers to do your paper?
Quality- We are experienced and have access to ample research materials.
We write plagiarism Free Content
Confidential- We never share or sell your personal information to third parties.
Support-Chat with us today! We are always waiting to answer all your questions.
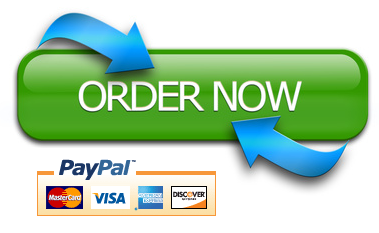