Discuss the potential biases that may exist in different public health datasets. Feel free to focus on specific datasets or types of data that you are familiar with, but you can als
Discuss the potential biases that may exist in different public health datasets. Feel free to focus on specific datasets or types of data that you are familiar with, but you can also consider the following types of data:
- Infectious disease data from public schools
- Fall data from nursing homes
- Opioid overdose data from first responder reports
- Genetic risk profiles from rural regions in developing countries
Instructions:
- Your discussion should include the types of explicit and implicit biases that may be in the data, as well as how both sampling and reporting biases may play a role in the data creation process
- Finally, describe the ideal process for creating the data (as unrealistic and infeasible as it may well be), and
- Identify steps to creating a feasible dataset on the topic that either reduces biases as much as possible or at least would allow public health experts to better understand the limitations of the data
Include references
-
HSCI6348-HealthcareDatabaseManagement_Chapter13-Tagged.pdf
-
HSCI6348-HealthcareDatabaseManagement_Chapter10-Tagged.pdf
-
HSCI6348-HealthcareDatabaseManagement_Chapter11-Tagged.pdf
-
HSCI6348-HealthcareDatabaseManagement_Chapter14-Tagged.pdf
-
HSCI6348-HealthcareDatabaseManagement_Chapter15-Tagged.pdf
-
HSCI6348-HealthcareDatabaseManagement_Chapter12-Tagged.pdf
Database Systems: Design, Implementation, and
Management Tenth Edition
Chapter 13 Business Intelligence and Data
Warehouses
Objectives
In this chapter, you will learn: • How business intelligence provides a
comprehensive business decision support framework
• About business intelligence architecture, its evolution, and reporting styles
• About the relationship and differences between operational data and decision support data
• What a data warehouse is and how to prepare data for one
Database Systems, 10th Edition 2
Objectives (cont’d.)
• What star schemas are and how they are constructed
• About data analytics, data mining, and predictive analytics
• About online analytical processing (OLAP) • How SQL extensions are used to support
OLAP-type data manipulations
Database Systems, 10th Edition 3
The Need for Data Analysis
• Managers track daily transactions to evaluate how the business is performing
• Strategies should be developed to meet organizational goals using operational databases
• Data analysis provides information about short- term tactical evaluations and strategies
Database Systems, 10th Edition 4
Business Intelligence
• Comprehensive, cohesive, integrated tools and processes – Capture, collect, integrate, store, and analyze
data
– Generate information to support business decision making
• Framework that allows a business to transform: – Data into information
– Information into knowledge
– Knowledge into wisdom Database Systems, 10th Edition 5
Business Intelligence Architecture
• Composed of data, people, processes, technology, and management of components
• Focuses on strategic and tactical use of information
• Key performance indicators (KPI) – Measurements that assess company’s
effectiveness or success in reaching goals
• Multiple tools from different vendors can be integrated into a single BI framework
Database Systems, 10th Edition 6
Database Systems, 10th Edition 7
Business Intelligence Benefits
• Main goal: improved decision making • Other benefits
– Integrating architecture
– Common user interface for data reporting and analysis
– Common data repository fosters single version of company data
– Improved organizational performance
Database Systems, 10th Edition 8
Business Intelligence Evolution
Database Systems, 10th Edition 9
Database Systems, 10th Edition 10
Business Intelligence Technology Trends
• Data storage improvements • Business intelligence appliances • Business intelligence as a service • Big Data analytics • Personal analytics
Database Systems, 10th Edition 11
Decision Support Data
• BI effectiveness depends on quality of data gathered at operational level
• Operational data seldom well-suited for decision support tasks
• Need reformat data in order to be useful for business intelligence
Database Systems, 10th Edition 12
Operational Data vs. Decision Support Data
• Operational data – Mostly stored in relational database – Optimized to support transactions representing
daily operations
• Decision support data differs from operational data in three main areas: – Time span
– Granularity
– Dimensionality
Database Systems, 10th Edition 13
Database Systems, 10th Edition 14
Decision Support Database Requirements
• Specialized DBMS tailored to provide fast answers to complex queries
• Three main requirements – Database schema
– Data extraction and loading
– Database size
Database Systems, 10th Edition 15
Decision Support Database Requirements (cont’d.)
• Database schema – Complex data representations – Aggregated and summarized data – Queries extract multidimensional time slices
• Data extraction and filtering – Supports different data sources
• Flat files • Hierarchical, network, and relational databases • Multiple vendors
– Checking for inconsistent data Database Systems, 10th Edition 16
Decision Support Database Requirements (cont’d.)
• Database size – In 2005, Wal-Mart had 260 terabytes of data in
its data warehouses
– DBMS must support very large databases (VLDBs)
Database Systems, 10th Edition 17
The Data Warehouse
• Integrated, subject-oriented, time-variant, and nonvolatile collection of data – Provides support for decision making
• Usually a read-only database optimized for data analysis and query processing
• Requires time, money, and considerable managerial effort to create
Database Systems, 10th Edition 18
Database Systems, 10th Edition 19
Data Marts
• Small, single-subject data warehouse subset • More manageable data set than data
warehouse • Provides decision support to small group of
people • Typically lower cost and lower implementation
time than data warehouse
Database Systems, 10th Edition 20
Twelve Rules That Define a Data Warehouse
Database Systems, 10th Edition 21
Star Schemas
• Data-modeling technique – Maps multidimensional decision support data
into relational database
• Creates near equivalent of multidimensional database schema from relational data
• Easily implemented model for multidimensional data analysis while preserving relational structures
• Four components: facts, dimensions, attributes, and attribute hierarchies
Database Systems, 10th Edition 22
Facts
• Numeric measurements that represent specific business aspect or activity – Normally stored in fact table that is center of star
schema
• Fact table contains facts linked through their dimensions
• Metrics are facts computed at run time
Database Systems, 10th Edition 23
Dimensions
• Qualifying characteristics provide additional perspectives to a given fact
• Decision support data almost always viewed in relation to other data
• Study facts via dimensions • Dimensions stored in dimension tables
Database Systems, 10th Edition 24
Attributes
• Use to search, filter, and classify facts • Dimensions provide descriptions of facts
through their attributes • No mathematical limit to the number of
dimensions • Slice and dice: focus on slices of the data cube
for more detailed analysis
Database Systems, 10th Edition 25
Attribute Hierarchies
• Provide top-down data organization • Two purposes:
– Aggregation
– Drill-down/roll-up data analysis
• Determine how the data are extracted and represented
• Stored in the DBMS’s data dictionary • Used by OLAP tool to access warehouse
properly
Database Systems, 10th Edition 26
Star Schema Representation
• Facts and dimensions represented in physical tables in data warehouse database
• Many fact rows related to each dimension row – Primary key of fact table is a composite primary
key
– Fact table primary key formed by combining foreign keys pointing to dimension tables
• Dimension tables are smaller than fact tables • Each dimension record is related to thousands
of fact records Database Systems, 10th Edition 27
Performance-Improving Techniques for the Star Schema
• Four techniques to optimize data warehouse design: – Normalizing dimensional tables
– Maintaining multiple fact tables to represent different aggregation levels
– Denormalizing fact tables
– Partitioning and replicating tables
Database Systems, 10th Edition 28
Performance-Improving Techniques for the Star Schema (cont’d.)
• Dimension tables normalized to: – Achieve semantic simplicity – Facilitate end-user navigation through the
dimensions
• Denormalizing fact tables improves data access performance and saves data storage space
• Partitioning splits table into subsets of rows or columns
• Replication makes copy of table and places it in different location
Database Systems, 10th Edition 29
Data Analytics
• Subset of BI functionality • Encompasses a wide range of mathematical,
statistical, and modeling techniques – Purpose of extracting knowledge from data
• Tools can be grouped into two separate areas: – Explanatory analytics
– Predictive analytics
Database Systems, 10th Edition 30
Data Mining
• Data-mining tools do the following: – Analyze data – Uncover problems or opportunities hidden in
data relationships
– Form computer models based on their findings – Use models to predict business behavior
• Runs in two modes – Guided
– Automated
Database Systems, 10th Edition 31
Database Systems, 10th Edition 32
Predictive Analytics
• Employs mathematical and statistical algorithms, neural networks, artificial intelligence, and other advanced modeling tools
• Create actionable predictive models based on available data
• Models are used in areas such as: – Customer relationships, customer service,
customer retention, fraud detection, targeted marketing, and optimized pricing
Database Systems, 10th Edition 33
Online Analytical Processing
• Three main characteristics: – Multidimensional data analysis techniques – Advanced database support
– Easy-to-use end-user interfaces
Database Systems, 10th Edition 34
Multidimensional Data Analysis Techniques
• Data are processed and viewed as part of a multidimensional structure
• Augmented by the following functions: – Advanced data presentation functions
– Advanced data aggregation, consolidation, and classification functions
– Advanced computational functions
– Advanced data modeling functions
Database Systems, 10th Edition 35
Advanced Database Support
• Advanced data access features include: – Access to many different kinds of DBMSs, flat
files, and internal and external data sources
– Access to aggregated data warehouse data
– Advanced data navigation – Rapid and consistent query response times
– Maps end-user requests to appropriate data source and to proper data access language
– Support for very large databases
Database Systems, 10th Edition 36
Easy-to-Use End-User Interface
• Advanced OLAP features are more useful when access is simple
• Many interface features are “borrowed” from previous generations of data analysis tools – Already familiar to end users
– Makes OLAP easily accepted and readily used
Database Systems, 10th Edition 37
OLAP Architecture
• Three main architectural components: – Graphical user interface (GUI) – Analytical processing logic
– Data-processing logic
Database Systems, 10th Edition 38
OLAP Architecture (cont’d.)
• Designed to use both operational and data warehouse data
• In most implementations, data warehouse and OLAP are interrelated and complementary
• OLAP systems merge data warehouse and data mart approaches
Database Systems, 10th Edition 39
Database Systems, 10th Edition 40
Relational OLAP
• Relational online analytical processing (ROLAP) provides the following extensions: – Multidimensional data schema support within the
RDBMS
– Data access language and query performance optimized for multidimensional data
– Support for very large databases (VLDBs)
Database Systems, 10th Edition 41
Multidimensional OLAP
• Multidimensional online analytical processing (MOLAP) extends OLAP functionality to multidimensional database management systems (MDBMSs) – MDBMS end users visualize stored data as a 3D
data cube
– Data cubes can grow to n dimensions, becoming hypercubes
– To speed access, data cubes are held in memory in a cube cache
Database Systems, 10th Edition 42
Relational vs. Multidimensional OLAP
• Selection of one or the other depends on evaluator’s vantage point
• Proper evaluation must include supported hardware, compatibility with DBMS, etc.
• ROLAP and MOLAP vendors working toward integration within unified framework
• Relational databases use star schema design to handle multidimensional data
Database Systems, 10th Edition 43
Database Systems, 10th Edition 44
SQL Extensions for OLAP
• Proliferation of OLAP tools fostered development of SQL extensions
• Many innovations have become part of standard SQL
• All SQL commands will work in data warehouse as expected
• Most queries include many data groupings and aggregations over multiple columns
Database Systems, 10th Edition 45
The ROLLUP Extension
• Used with GROUP BY clause to generate aggregates by different dimensions
• GROUP BY generates only one aggregate for each new value combination of attributes
• ROLLUP extension enables subtotal for each column listed except for the last one – Last column gets grand total
• Order of column list important
Database Systems, 10th Edition 46
The CUBE Extension
• CUBE extension used with GROUP BY clause to generate aggregates by listed columns – Includes the last column
• Enables subtotal for each column in addition to grand total for last column – Useful when you want to compute all possible
subtotals within groupings
• Cross-tabulations are good candidates for application of CUBE extension
Database Systems, 10th Edition 47
Materialized Views
• A dynamic table that contains SQL query command to generate rows – Also contains the actual rows
• Created the first time query is run and summary rows are stored in table
• Automatically updated when base tables are updated
Database Systems, 10th Edition 48
Summary
• Business intelligence generates information used to support decision making
• BI covers a range of technologies, applications, and functionalities
• Decision support systems were the precursor of current generation BI systems
• Operational data not suited for decision support
Database Systems, 10th Edition 49
Summary (cont’d.)
• Data warehouse provides support for decision making – Usually read-only
– Optimized for data analysis, query processing
• Star schema is a data-modeling technique – Maps multidimensional decision support data
into a relational database
• Star schema has four components: – Facts, dimensions, attributes, and attribute
hierarchies Database Systems, 10th Edition 50
Summary (cont’d.)
• Data analytics – Provides advanced data analysis tools to extract
knowledge from business data
• Data mining – Automates the analysis of operational data to
find previously unknown data characteristics, relationships, dependencies, and trends
• Predictive analytics – Uses information generated in the data-mining
phase to create advanced predictive models
Database Systems, 10th Edition 51
Summary (cont’d.)
• Online analytical processing (OLAP) – Advanced data analysis environment that
supports decision making, business modeling, and operations research
• SQL has been enhanced with extensions that support OLAP-type processing and data generation
Database Systems, 10th Edition 52
- Database Systems: Design, Implementation, and Management Tenth Edition
- Objectives
- Objectives (cont’d.)
- The Need for Data Analysis
- Business Intelligence
- Business Intelligence Architecture
- PowerPoint Presentation
- Business Intelligence Benefits
- Business Intelligence Evolution
- Slide 10
- Business Intelligence Technology Trends
- Decision Support Data
- Operational Data vs. Decision Support Data
- Slide 14
- Decision Support Database Requirements
- Decision Support Database Requirements (cont’d.)
- Slide 17
- The Data Warehouse
- Slide 19
- Data Marts
- Twelve Rules That Define a Data Warehouse
- Star Schemas
- Facts
- Dimensions
- Attributes
- Attribute Hierarchies
- Star Schema Representation
- Performance-Improving Techniques for the Star Schema
- Performance-Improving Techniques for the Star Schema (cont’d.)
- Data Analytics
- Data Mining
- Slide 32
- Predictive Analytics
- Online Analytical Processing
- Multidimensional Data Analysis Techniques
- Advanced Database Support
- Easy-to-Use End-User Interface
- OLAP Architecture
- OLAP Architecture (cont’d.)
- Slide 40
- Relational OLAP
- Multidimensional OLAP
- Relational vs. Multidimensional OLAP
- Slide 44
- SQL Extensions for OLAP
- The ROLLUP Extension
- The CUBE Extension
- Materialized Views
- Summary
- Summary (cont’d.)
- Slide 51
- Slide 52
,
Database Systems: Design, Implementation, and
Management Tenth Edition
Chapter 10 Transaction Management and Concurrency Control
Objectives
• In this chapter, you will learn: – About database transactions and their properties – What concurrency control is and what role it
plays in maintaining the database’s integrity
– What locking methods are and how they work
Database Systems, 10th Edition 2
Objectives (cont’d.)
– How stamping methods are used for concurrency control
– How optimistic methods are used for concurrency control
– How database recovery management is used to maintain database integrity
Database Systems, 10th Edition 3
What Is a Transaction?
• Logical unit of work that must be either entirely completed or aborted
• Successful transaction changes database from one consistent state to another – One in which all data integrity constraints are
satisfied
• Most real-world database transactions are formed by two or more database requests – Equivalent of a single SQL statement in an
application program or transaction
Database Systems, 10th Edition 4
Database Systems, 10th Edition 5
Evaluating Transaction Results
• Not all transactions update database • SQL code represents a transaction because
database was accessed • Improper or incomplete transactions can have
devastating effect on database integrity – Some DBMSs provide means by which user can
define enforceable constraints
– Other integrity rules are enforced automatically by the DBMS
Database Systems, 10th Edition 6
Database Systems, 10th Edition 7
Figure 9.2
Transaction Properties
• Atomicity – All operations of a transaction must be
completed
• Consistency – Permanence of database’s consistent state
• Isolation – Data used during transaction cannot be used by
second transaction until the first is completed
Database Systems, 10th Edition 8
Transaction Properties (cont’d.)
• Durability – Once transactions are committed, they cannot
be undone
• Serializability – Concurrent execution of several transactions
yields consistent results
• Multiuser databases are subject to multiple concurrent transactions
Database Systems, 10th Edition 9
Transaction Management with SQL
• ANSI has defined standards that govern SQL database transactions
• Transaction support is provided by two SQL statements: COMMIT and ROLLBACK
• Transaction sequence must continue until: – COMMIT statement is reached
– ROLLBACK statement is reached
– End of program is reached
– Program is abnormally terminated
Database Systems, 10th Edition 10
The Transaction Log
• Transaction log stores: – A record for the beginning of transaction – For each transaction component:
• Type of operation being performed (update, delete, insert)
• Names of objects affected by transaction • “Before” and “after” values for updated fields
• Pointers to previous and next transaction log entries for the same transaction
– Ending (COMMIT) of the transaction
Database Systems, 10th Edition 11
Database Systems, 10th Edition 12
Concurrency Control
• Coordination of simultaneous transaction execution in a multiprocessing database
• Objective is to ensure serializability of transactions in a multiuser environment
• Three main problems: – Lost updates
– Uncommitted data
– Inconsistent retrievals
Database Systems, 10th Edition 13
Lost Updates
• Lost update problem: – Two concurrent transactions update same data
element
– One of the updates is lost • Overwritten by the other transaction
Database Systems, 10th Edition 14
Database Systems, 10th Edition 15
Uncommitted Data
• Uncommitted data phenomenon: – Two transactions are executed concurrently – First transaction rolled back after second already
accessed uncommitted data
Database Systems, 10th Edition 16
Database Systems, 10th Edition 17
Inconsistent Retrievals
• Inconsistent retrievals: – First transaction accesses data – Second transaction alters the data
– First transaction accesses the data again
• Transaction might read some data before they are changed and other data after changed
• Yields inconsistent results
Database Systems, 10th Edition 18
Database Systems, 10th Edition 19
Database Systems, 10th Edition 20
The Scheduler
• Special DBMS program – Purpose is to establish order of operations within
which concurrent transactions are executed
• Interleaves execution of database operations: – Ensures serializability
– Ensures isolation
• Serializable schedule – Interleaved execution of transactions yields
same results as serial execution
Database Systems, 10th Edition 21
Concurrency Control with Locking Methods
• Lock – Guarantees exclusive use of a data item to a
current transaction
– Required to prevent another transaction from reading inconsistent data
– Pessimistic locking • Use of locks based on the assumption that conflict
between transactions is likely
– Lock manager • Responsible for assigning and policing the locks
used by transactions Database Systems, 10th Edition 22
Lock Granularity
• Indicates level of lock use • Locking can take place at following levels:
– Database
– Table
– Page
– Row – Field (attribute)
Database Systems, 10th Edition 23
Lock Granularity (cont’d.)
• Database-level lock – Entire database is locked
• Table-level lock – Entire table is locked
• Page-level lock – Entire diskpage is locked
Database Systems, 10th Edition 24
Lock Granularity (cont’d.)
• Row-level lock – Allows concurrent transactions to access
different rows of same table • Even if rows are located on same page
• Field-level lock – Allows concurrent transactions to access same
row • Requires use of different fields (attributes) within
the row
Database Systems, 10th Edition 25
Database Systems, 10th Edition 26
Database Systems, 10th Edition 27
Database Systems, 10th Edition 28
Database Systems, 10th Edition 29
Lock Types
• Binary lock – Two states: locked (1) or unlocked (0)
• Exclusive lock – Access is specifically reserved for transaction
that locked object
– Must be used when potential for conflict exists
• Shared lock – Concurrent transactions are granted read
access on basis of a common lock
Database Systems, 10th Edition 30
Database Systems, 10th Edition 31
Two-Phase Locking to Ensure Serializability
• Defines how transactions acquire and relinquish locks
• Guarantees serializability, but does not prevent deadlocks – Growing phase
• Transaction acquires all required locks without unlocking any data
– Shrinking phase • Transaction releases all locks and cannot obtain
any new lock
Database Systems, 10th Edition 32
Two-Phase Locking to Ensure Serializability (cont’d.)
• Governed by the following rules: – Two transactions cannot have conflicting locks – No unlock operation can precede a lock
operation in the same transaction
– No data are affected until all locks are obtained
Database Systems, 10th Edition 33
Database Systems, 10th Edition 34
Deadlocks
• Condition that occurs when two transactions wait for each other to unlock data
• Possible only if one of the transactions wants to obtain an exclusive lock on a data item – No deadlock condition can exist among shared
locks
Database Systems, 10th Edition 35
Deadlocks (cont’d.)
• Three techniques to control deadlock: – Prevention – Detection
– Avoidance
• Choice of deadlock control method depends on database environment – Low probability of deadlock; detection
recommended
– High probability; prevention recommended
Database Systems, 10th Edition 36
Database Systems, 10th Edition 37
Concurrency Control with Time Stamping Methods
• Assigns global unique time stamp to each transaction
• Produces explicit order in which transactions are submitted to DBMS
• Uniqueness – Ensures that no equal time stamp values can
exist
• Monotonicity – Ensures that time stamp values always increase
Database Systems, 10th Edition 38
Wait/Die and Wound/Wait Schemes
• Wait/die – Older transaction waits and younger is rolled
back and rescheduled
• Wound/wait – Older transaction rolls back younger transaction
and reschedules it
Database Systems, 10th Edition 39
Database Systems, 10th Edition 40
</
Collepals.com Plagiarism Free Papers
Are you looking for custom essay writing service or even dissertation writing services? Just request for our write my paper service, and we'll match you with the best essay writer in your subject! With an exceptional team of professional academic experts in a wide range of subjects, we can guarantee you an unrivaled quality of custom-written papers.
Get ZERO PLAGIARISM, HUMAN WRITTEN ESSAYS
Why Hire Collepals.com writers to do your paper?
Quality- We are experienced and have access to ample research materials.
We write plagiarism Free Content
Confidential- We never share or sell your personal information to third parties.
Support-Chat with us today! We are always waiting to answer all your questions.
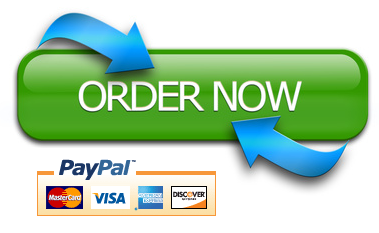