The effects of Online Hate speech, summary and comparison of the three articles and analyze Hate speech as a social phenomenon. ?520 pages.??Riegeretal2021hatespeechinfr
The effects of Online Hate speech, summary and comparison of the three articles and analyze Hate speech as a social phenomenon. 520 pages.
Creative Commons CC BY: This article is distributed under the terms of the Creative Commons Attribution 4.0 License (https://creativecommons.org/licenses/by/4.0/) which permits any use, reproduction and distribution of the work without further permission
provided the original work is attributed as specified on the SAGE and Open Access pages (https://us.sagepub.com/en-us/nam/open-access-at-sage).
https://doi.org/10.1177/20563051211052906
Social Media + Society October-December 2021: 1 –14 © The Author(s) 2021 Article reuse guidelines: sagepub.com/journals-permissions DOI: 10.1177/20563051211052906 journals.sagepub.com/home/sms
Article
On 15 March 2019, a right-wing extremist terrorist killed more than 50 people in mosques in Christchurch, New Zealand, and wounded numerous others—livestreaming his crimes on Facebook. Only 6 weeks later, on 27 April, another right-wing extremist attack occurred in a syna- gogue in Poway near San Diego, in which one person was killed and three more injured. The perpetrators were active in an online community within the imageboard 8chan, which is considered as particularly hateful and rife with right-wing extremist, misanthropic, and White-supremacist ideas. Moreover, both the San Diego and Christchurch shooters used 8chan to post their manifestos, providing insights into their White nationalist hatred (Stewart, 2019). Following the attack in New Zealand, Internet service pro- viders in Australia and New Zealand have temporarily blocked access to 8chan and the similar—albeit less extreme—imageboard 4chan (Brodkin, 2019). After yet another shooting in El Paso was linked to activities on 8chan, the platform was removed1 from the Clearnet entirely, with one of 8chan’s network infrastructure pro- viders claiming the unique lawlessness of the site that “has contributed to multiple horrific tragedies” as the main rea- son for this decision (Prince, 2019).
Whether the perpetrators’ activities on 8chan and 4chan actually contributed to their radicalization or motivation can hardly be determined. However, especially the plat- forms’ politics boards (8chan/pol/ and 4chan/pol/, respec- tively) have repeatedly been linked to the so-called alt-right movement, “exhibiting characteristics of xenophobia, social conservatism, racism, and, generally speaking, hate” (Hine et al., 2017, p. 92; see also Hawley, 2017; Tuters & Hagen, 2020). 4chan/pol/, in particular, has attracted the broader public’s attention during Donald Trump’s 2016 presidential campaign, often being the birthplace of conser- vative or even outright hateful and racist memes that circu- lated during the campaign. In addition to the mentioned communities on 4chan and 8chan, the controversial subred- dit “The_Donald” is often referenced as a popular and more
1052906 SMSXXX10.1177/20563051211052906Social Media <span class="symbol" cstyle="Mathematical">+</span> SocietyRieger et al. research-article20212021
1LMU Munich, Germany 2TU Dresden, Germany 3Technical University of Munich (TUM), Germany
Corresponding Author: Diana Rieger, Department of Media and Communication, LMU Munich, Oettingenstrasse 67, 80538 Munich, Germany. Email: [email protected]
Assessing the Extent and Types of Hate Speech in Fringe Communities: A Case Study of Alt-Right Communities on 8chan, 4chan, and Reddit
Diana Rieger1 , Anna Sophie Kümpel2 , Maximilian Wich3, Toni Kiening1, and Georg Groh3
Abstract Recent right-wing extremist terrorists were active in online fringe communities connected to the alt-right movement. Although these are commonly considered as distinctly hateful, racist, and misogynistic, the prevalence of hate speech in these communities has not been comprehensively investigated yet, particularly regarding more implicit and covert forms of hate. This study exploratively investigates the extent, nature, and clusters of different forms of hate speech in political fringe communities on Reddit, 4chan, and 8chan. To do so, a manual quantitative content analysis of user comments (N = 6,000) was combined with an automated topic modeling approach. The findings of the study not only show that hate is prevalent in all three communities (24% of comments contained explicit or implicit hate speech), but also provide insights into common types of hate speech expression, targets, and differences between the studied communities.
Keywords hate speech, alt-right, fringe communities, Reddit, 4chan, 8chan, content analysis, topic modeling
2 Social Media + Society
“mainstreamy” outlet for alt-right ideas as well (e.g., Heikkilä, 2017).
Although these political fringe communities are consid- ered as particularly hateful in the public debate, only few studies (Hine et al., 2017; Mittos, Zannettou, Blackburn, & De Cristofaro, 2019) have investigated these communities with regard to the extent of hate speech. Moreover, the men- tioned studies are exclusively built on automated dictionary- based approaches focusing on explicit “hate terms,” thus being unable to account for more subtle or covert forms of hate. To better understand the different types of hate speech in these communities, it also seems advisable to cluster com- ments in which hate speech occurs.
Addressing these research gaps, we (a) provide a system- atic investigation of the extent and nature of hate speech in alt-right fringe communities, (b) examine both explicit and implicit forms of hate speech, and (c) merge manual coding of hate speech with automated approaches. By combining a manual quantitative content analysis of user comments (N = 6,000) and unsupervised machine learning in the form of topic modeling, this study aims at understanding the extent and nature of different types of hate speech as well as the thematic clusters these occur in. We first investigate the extent and target groups of different forms of hate speech in the three mentioned alt-right fringe communities on Reddit (r/The_Donald), 4chan (4chan/pol/), and 8chan (8chan/pol/). Subsequently, by means of a topic modeling approach, the clusters in which hate speech occurs are analyzed in more detail.
Hate Speech in Online Environments
Hate speech was certainly not invented with the Internet. Being situated “in a complex nexus with freedom of expres- sion, individual, group, and minority rights, as well as con- cepts of dignity, liberty, and equality” (Gagliardone, Gal, Alves, & Martínez, 2015, p. 10), it has been in the center of legislative discussion in many countries for many years. Hate speech is considered to be an elusive term, with extant definitions oscillating between strictly legal rationales and generic understandings that include almost all instances of incivility or expressions of anger (Gagliardone et al., 2015). For the context of this study, we deem both the content and the targets as crucial for conceptualizing hate speech. Accordingly, hate speech is defined here as the expression of “hatred or degrading attitudes toward a collective” (Hawdon, Oksanen, & Räsänen, 2017, p. 254), with people being devalued not based on individual traits, but on account of their race, ethnicity, religion, sexual orientation, or other group-defining characteristics (Hawdon et al., 2017, see also Kümpel & Rieger, 2019).
There are a number of factors—resulting from the over- arching characteristics of online information environments— suggesting that hate speech is particularly problematic on the Internet. First, there is the problem of permanence
(Gagliardone et al., 2015). Especially fringe communities are heavily centered on promoting users’ freedom of expression, making it unlikely that hate speech will be removed by mod- erators or platform operators. But even if hateful content is removed, it might have already been circulated to other plat- forms, or it could be reposted to the same site again shortly after deletion (Jardine, 2019). Second, the shareability and ease of disseminating content in online environments further facilitates the visibility of hate speech (Kümpel & Rieger, 2019). During the 2016 Trump campaign, hateful anti-immi- gration and anti-establishment memes were often spread beyond the borders of fringe communities, surfacing to mainstream social media and influencing discussions on these platforms (Heikkilä, 2017). Third, the (actual or per- ceived) anonymity in online environments can encourage people to “be more outrageous, obnoxious, or hateful in what they say” (Brown, 2018, p. 298), because they feel disinhib- ited and less accountable for their actions. Moreover, ano- nymity can also change the relative salience of one’s personal and social identity, thereby increasing conformity to per- ceived group norms (Reicher, Spears, & Postmes, 1995). Indeed, research has found that exposure to online comments with ethnic prejudices leads other users to post more preju- diced comments themselves (Hsueh, Yogeeswaran, & Malinen, 2015), suggesting that the communication behavior of others also influences one’s own behavior. Fourth, and closely related to anonymity, there is the problem of the full or partial invisibility of other users (Brown, 2018; Lapidot- Lefler & Barak, 2012): The absence of facial expressions and other visibility originated interpersonal communication cues makes hate speech appear less hurtful or damaging in an online setting, thus increasing inhibitions to discriminate others. Last, one has to consider the community-building aspects that are particularly distinctive for online hate speech (Brown, 2018; McNamee, Peterson, & Peña, 2010). Not least in alt-right fringe communities, hate is often “meme- ified” and mixed with humor and domain-specific slang, cre- ating a situation in which the use of hate speech can play a crucial role in strengthening bonds among members of the community and distinguishing one’s group from clueless outsiders (Tuters & Hagen, 2020). Taken together, the men- tioned factors facilitate not only the creation and use of hate speech in online environments, but also its wider dissemina- tion and visibility.
Implicit Forms of Hate Speech
While many types of online hate speech are relatively straightforward and “in your face” (Borgeson & Valeri, 2004), hate can also be expressed in a more implicit or covert form (see Ben-David & Matamoros-Fernández, 2016 ; Benikova, Wojatzki, & Zesch, 2018; ElSherief, Kulkarni, Nguyen, Wang, & Belding, 2018; Magu & Luo, 2018; Matamoros-Fernández, 2017)—for example, by spreading negative stereotypes or strategically elevating one’s ingroup.
Rieger et al. 3
Implicit hate speech shares characteristics with what Buyse (2014, p. 785) has labeled fear speech, which is “aimed at instilling (existential) fear of another group” by highlighting harmful actions the target group has allegedly engaged in or speculations about their goals to “take over and dominate in the future” (Saha, Mathew, Garimella, & Mukherjee, 2021, p. 1111). Indeed, one variety of implicit hate speech can be seen in the intentional spreading of “fake news,” in which deliberate false statements or conspiracy theories about social groups are circulated to marginalize them (Hajok & Selg, 2018). This could be observed in connection with the European migrant crisis during which online disinformation often focused on the degradation of immigrants, for exam- ple, through associating them with crime and delinquency (Hajok & Selg, 2018, see also Humprecht, 2019).
Implicitness is a major problem for the automated detec- tion of hate speech, as it “is invisible to automatic classifiers” (Benikova et al., 2018, p. 177). Using such implicit forms of hate speech is a common strategy to even avoid automatic detection systems and to cloak prejudices and resentments in “ordinary” statements (e.g., “My cleaning lady is really good, even though she is Turkish,” see Meibauer, 2013). Thus, implicit hate speech points to the importance of acknowledging the wider context of hate speech instead of just focusing on the occurrence of single (and often ambigu- ous) hate terms.
Extent of Hate Speech
Considering the mentioned problems with the (automated) detection of hate speech, it is hard to determine the overall prevalence of hate speech in online environments. To account for individual experiences, extant studies have often relied on surveys to estimate hate speech exposure. Across differ- ent populations around the globe, such self-reported expo- sure to online hate speech ranges from about 28% (New Zealanders 18+, see Pacheco & Melhuish, 2018), to 64% (13- to 17-year-old US Americans, see Common Sense, 2018), and up to 85% (14- to 24-year-old Germans, see Landesanstalt für Medien NRW, 2018). In studies focusing both on younger and older online users (Landesanstalt für Medien NRW, 2018; Pacheco & Melhuish, 2018), exposure to online hate was more commonly reported by younger age groups, which might be explained by different usage patterns and/or perceptual differences. However, while these survey figures suggest that many online users seem to have been exposed to hateful comments, they tell us only little about the overall amount of hate speech in online environments. In fact, even a single highly visible hate comment could be responsible for survey participants responding affirmatively to questions about their exposure to online hate. Thus, to determine the actual extent of hate speech, content analyses are needed—although the results are equally hard to general- ize. Indeed, the amount of content labeled as hate speech seems to differ considerably, depending on the studied
platforms and (sub-)communities, the topic of discussions, or the lexical resources and dictionaries used to determine what qualifies as hate speech (ElSherief et al., 2018; Hine et al., 2017; Meza, 2016). Considering our focus on alt-right fringe communities, we will thus aim our attention at the pre- sumed and actual hatefulness of these discussion spaces.
The “Alt-Right” Movement and Fringe Communities
What Is the Alt-Right?
The alt-right (= abbreviated form of alternative right) is a rather loosely connected and largely online-based political movement, whose ideology centers around ideas of White supremacy, anti-establishmentarianism, and anti-immigra- tion (see Hawley, 2017; Heikkilä, 2017; Nagle, 2017). Gaining momentum during Donald Trump’s 2016 presiden- tial campaign, the alt-right “took an active role in cheerlead- ing his candidacy and several of his controversial policy positions” (Forscher & Kteily, 2020, p. 90), particularly on the mentioned message boards on Reddit (r/The_Donald), 4chan, and 8chan (/pol/ on both platforms). Similar to other online communities, the alt-right uses a distinct verbal and visual language that is characterized by the use of memes, subcultural terms, and references to the wider web culture (Hawley, 2017; Tuters & Hagen, 2020; Wendling, 2018). Another common theme is “the cultivation of a position that sees white male identity as threatened” (Heikkilä, 2017, p. 4), which is connected both to strongly opposing policies related to “political correctness” (e.g., affirmative action) and to condemning social groups that are perceived to be profiting from these policies (Phillips & Yi, 2018). Openly expressing these ideas often culminates in the use of hate speech, particularly against people of color and women. However, while discussion spaces linked to the alt-right are routinely described as hateful, there is little published data on the quantitative amount of hate speech in these fringe communities.
Hate Speech in Alt-Right Fringe Communities
To our knowledge, empirical studies addressing the extent of hate speech in alt-right fringe communities have exclusively relied on automated dictionary-based approaches, estimating the amount of hate speech by identifying posts that contain hateful terms (Hine et al., 2017; Mittos et al., 2019). Focusing on 4chan/pol/, Hine and colleagues (2017) use the hatebase dictionary to assess the prevalence of hate speech in the “Politically Incorrect” board. They find that 12% of posts on 4chan/pol/ contain hateful terms, thus revealing a substan- tially higher share than the two examined “baseline” boards 4chan/sp/ (focusing on sports) with 6.3% and 4chan/int/ (focusing on international cultures/languages) with 7.3%. However, 4chan generally seems to be more hateful than
4 Social Media + Society
other social media platforms: Analyzing a sample of Twitter posts for comparison, the authors find that only 2.2% of the analyzed tweets contained hateful terms. Looking at the most “popular” hate terms used in 4chan/pol/, it is also possible to draw cautious conclusions about the (main) target groups of hate speech. The hate terms appearing most—“nigger,” “fag- got,” and “retard”—are indicative of racist, homophobic, and ableist sentiments and suggest that people of color, the les- bian, gay, bisexual, transgender and queer or questioning (LGBTQ) community, and people with disabilities might be recurrent victims of hate speech.
Utilizing a similar analytical approach, but exclusively focusing on discussions about genetic testing, Mittos and colleagues (2019) investigate both Reddit and 4chan/pol/ with regard to their levels of hate. For Reddit, their analysis shows that the most hateful subreddits alluding to the topic of genetic testing are associated with the alt-right (e.g., r/ altright, r/TheDonald, r/DebateAltRight), with posts dis- playing “clear racist connotations, and of groups of users using genetic testing to push racist agendas” (Mittos et al., 2019, p. 9). These tendencies are even more amplified on 4chan/pol/ where discussion about genetic testing are rou- tinely combined with content exhibiting racial and anti- Semitic hate speech. Reflecting the findings of Hine and colleagues (2017), racial and ethnic slurs are prevalent and illustrate the boards’ close association with White- supremacist ideologies.
While these studies offer some valuable insights into the hatefulness of alt-right fringe communities, the dictionary- based approaches are unable to account for more veiled and implicit forms of hate speech. Moreover, although the most “popular” terms hint at the targets of hate speech, a system- atic investigation of the addressed social groups is missing. Based on the literature review and theoretical considerations, our study thus sought to answer three overarching research questions:
Research Question 1. What percentage of user comments in the three fringe communities contains explicit or implicit hate speech?
Research Question 2. (a) In which way is hate speech expressed and (b) against which persons/groups is it directed?
Research Question 3. What is the topical structure of the coded user comments?
Method
Our empirical analysis of alt-right fringe communities focuses on three discussion boards within the platforms Reddit (r/The_Donald), 4chan (4chan/pol/), and 8chan (8chan/pol/), thus spanning from central and highly used to more peripheral and less frequented communities. While
Reddit, the self-proclaimed “front page of the Internet,” rou- tinely ranks among the 20 most popular websites worldwide, 4chan and 8chan have (or had) considerably less reach. However, due to their connection with the perpetrators of Christchurch, Poway, and El Paso, 4chan and 8chan are nev- ertheless of high relevance for this investigation. All three platforms follow a similar structure and are divided into a number of different subforums (called “subreddits” on Reddit and “boards” on 4chan/8chan). While Reddit requires users to register to post or comment, both 4chan and 8chan do not have a registration system, thus allowing everyone to contribute anonymously. The specific discussion boards—r/ The_Donald, 4chan/pol/, and 8chan/pol/—were chosen due to their association with alt-right ideas as well as their rela- tive centrality within the three platforms. Moreover, all three boards have previously been discussed as important outlets of right-wing extremists’ online activities (Conway, Macnair, & Scrivens, 2019).
In the following sections, we will first describe the data collection process and then outline the two methodological/ analytical approaches used in this study: (a) a manual quan- titative content analysis of user comments in the three dis- cussion boards and (b) an automated topic modeling approach. While 4chan and 8chan are indeed imageboards, (textual) comments play an important role on these platforms as well. On Reddit, pictures can easily be incorporated in the original post that constitutes the beginning of a thread, but comments are by default bound to text. Due to our two- pronged strategy, the nature of these communities, and to ensure comparability between the discussion boards, we focused our analyses on the textual content of comments and did not consider (audio-)visual materials such as images or videos. However, we refer to their importance in the context of hate speech in the discussion.
Data Collection
Since accessing and collecting content from the three discus- sion boards varies in complexity, we relied on different sam- pling strategies. Comments from r/The_Donald were obtained by querying the Pushshift Reddit data set (Baumgartner, Zannettou, Keegan, Squire, & Blackburn, 2020) via redditsearch.io. Between 21 April and 27 April 2019, we downloaded a total of 70,000 comments, of which 66,617 could be kept in the data set after removing duplicates and deleted/removed comments. Comments from 4chan/pol/ were obtained by using the independent archive page 4plebs. org and a web scraper. Between 14 April and 29 April 2019, a total of 16,000 comments were obtained, of which 15,407 remained after the cleaning process.2 Finally, comments from 8chan/pol/ were obtained by directly scraping the plat- form: All comments in threads that were active on 24 April 2019 were downloaded, resulting in a data set of 63,504 comments for this community. For the manual quantitative content analysis, 2,000 comments were randomly sampled
Rieger et al. 5
from the data set of each of the three communities, thus lead- ing to a combined sample size of 6,000 comments.
Approach I: Manual Quantitative Content Analysis
As our first main category, we coded explicit hate speech in accordance with recurrent conceptualizations in the litera- ture. Within this category, we defined insults (attacks to individuals/groups on the basis of their group-defining char- acteristics, e.g., Erjavec & Kovačič, 2012) as offensive, derogatory, or degrading expressions, including the use of ethnophaulisms (Kinney, 2008). Instead of coding insults in general, we distinguished between personal insults (i.e., attacks of a specific individual) and general insults (i.e., attacks of a collective), also coding the reference point of personal insults and the target of general insults. The spe- cific reference points [(a) Ethnicity, (b) Religion, (c) Country of Origin, (d) Gender, (e) Gender Identity, (f) Sexual Orientation, (g) Disabilities, (h) Political Views/Attitudes] or targets [(a) Black People, (b) Muslims, (c) Jews, (d) LGBTQ, (e) Migrants, (f) People with Disabilities, (g) Social Elites/Media, (h) Political Opponents, (i) Latin Americans*, (j) Women, (k) Criminals*, (l) Asians) were compiled on the basis of research on frequently marginal- ized groups (Burn, Kadlec, & Rexer, 2005; Mondal, Silva, Correa, & Benevenuto, 2018), and inductively extended (targets marked with *) during the coding process. Furthermore, we have coded violence threats as a form of explicit hate speech (Erjavec & Kovačič, 2012; Gagliardone et al., 2015), including both concrete threats of physical, psychological, or other types of violence and calls for vio- lence to be inflicted on specific individuals or groups.
As our second main category, we coded implicit hate speech. To distinguish different subcategories of this type of hate speech, we relied more strongly on an explorative approach by focusing on communication forms that have been described in the literature as devices to cloak hate (see section “Implicit Forms of Hate Speech”). The first subcate- gory of implicit hate speech is labeled negative stereotyping and was coded when users expressed overly generalized and simplified beliefs about (negative) characteristics or behav- iors of different target groups. The second subcategory—dis- information/conspiracy theories—reflects both “simple” disinformation and false statements about target groups and “advanced” conspiracy theories that represent target groups as maliciously working together toward greater ideological, political, or financial power (e.g., “the Jew media controls everything”). A third subcategory was labeled ingroup eleva- tion and was coded when statements elevated or accentuated belonging to a certain (racial, demographic, etc.) group, oftentimes implicitly excluding and devaluing other groups. The last subcategory of implicit hate speech was labeled inhuman ideology. Here, it was coded whether a user com- ment supported or glorified hateful ideologies such as
National Socialism or White supremacy, including the wor- shiping of prominent representatives of such ideologies.
In addition, a category spam was added to exclude com- ments containing irrelevant content such as random charac- ter combinations or advertisements. The entire coding scheme as well as an overview of the main content categories described in the previous paragraphs can be accessed via an open science framework (OSF) repository3.
The manual quantitative content analysis was conducted by two independent coders. Both coders coded the same sub- sample of 10% from the full sample of comments to calcu- late inter-rater reliability with the help of the R package “tidycomm” (Unkel, 2021). Using both percent agreement and Brennan and Prediger’s Kappa, all reliability values were satisfactory (κ ⩾ 0.83, see also Table 1). Prior to the analyses, all comments coded as spam were removed, lead- ing to a final sample size of 5,981 comments.
Approach II: Topic Modeling
Topic modeling is an unsupervised machine learning approach to identify topics within a collection of documents and to clas- sify these documents into distinct topics. Günther and Domahidi (2017) generally describe a topic as “what is being talked/writ- ten about” (p. 3057). Each topic would thus be represented in a cluster. Consequently, each cluster is assigned a set of words that are representative of the comments within the cluster. For our analysis, we first generated a topic model (TM1) for all 5,981 comments to gain an understanding of the topics within the entire data set. Combined with the manual coding, these results provide insights on which topics are more hateful than others. Second, another topic model (TM2) was created only for the comments identified as hateful (n = 1,438) to examine the clusters of the comments in which hate speech occurs. To do so, TM1 and TM2 were compared by investigating the tran- sitions between the models. In addition, TM2 was also com- bined with the manually coded data, allowing to establish a connection between the cluster, type, and targets of hate speech.
CluWords was selected as the topic model algorithm—a state-of-the-art short-text topic modeling technique (Viegas et al., 2019). The reason for not choosing a more conven- tional technique such as Latent Dirichlet Allocation (LDA) is that these do not perform well on shorter texts because they rely on word co-occurrences (Campbell, Hindle, & Stroulia, 2003; Cheng, Yan, Lan, & Guo, 2014; Quan, Kit, Ge, & Pan, 2015). CluWords overcomes this issue by combining non- probabilistic matrix factorization and pre-trained word- embeddings (Viegas et al., 2019). Especially the latter allows enriching the comments with “syntactic and semantic infor- mation” (Viegas et al., 2019, p. 754). For this article, the fast- Text word vectors pre-trained on the English Common Crawl dataset were used because it is trained on web data and thus an appropriate basis (Mikolov, Grave, Bojanowski, Puhrsch, & Joulin, 2019).
6 Social Media + Society
One challenge of topic modeling is to find a meaningful number of clusters. Since topic modeling is an unsupervised learning approach, there is no single right solution. To cope with this problem, the following five criteria have been used to determine an appropriate number of clusters: (a) the same number of topics for TM1 and TM2, (b) a meaningful and manageable number of topics, (c) comprehensibility of the topics, (d) standard deviation of the topics’ sizes, and (e) (normalized) pointwise mutual information.
Results
Results of Manual Quantitative Content Analysis
Addressing RQ1 (extent of explicit/implicit hate speech), we found that almost a quarter (24%, n = 1,438) of the analyzed 5,981 comments contained at least one instance of explicit or implicit hate speech (see Table 2). In 821 of the comments (13.7%), forms of explicit hate speech were identified (i.e., at least one of the categories personal insult, general insult, or violence threat was coded). Implicit hate speech (i.e., nega- tive stereotyping, disinformation/conspiracy theories, ingroup elevation, and inhuman ideologies) occurred slightly more often and was observed in 928 comments (15.5%).
Focusing on RQ2a (forms of hate speech), general insults were the most common form of hate speech and observed in 570 comments: they were included in almost every 10th com- ment of the entire sample (9.5%) and in more than one-third of all identified hateful comments (39.6%). Disinformation and conspiracy theories followed next and made up 31.8% of all comments with hate speech (n = 458). Within this category, conspiracy theories (n = 294) were observed almost
Collepals.com Plagiarism Free Papers
Are you looking for custom essay writing service or even dissertation writing services? Just request for our write my paper service, and we'll match you with the best essay writer in your subject! With an exceptional team of professional academic experts in a wide range of subjects, we can guarantee you an unrivaled quality of custom-written papers.
Get ZERO PLAGIARISM, HUMAN WRITTEN ESSAYS
Why Hire Collepals.com writers to do your paper?
Quality- We are experienced and have access to ample research materials.
We write plagiarism Free Content
Confidential- We never share or sell your personal information to third parties.
Support-Chat with us today! We are always waiting to answer all your questions.
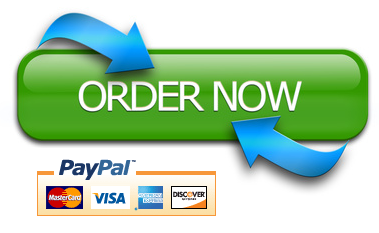