use the link below and the attached reading to answer the question (50-70 words) Conceptualize the general concept of evi
use the link below and the attached reading to answer the question (50-70 words)
Conceptualize the general concept of “eviction” (see Evicted; “How we undercounted…”; and MARS [pages 21-22 and the diagrams “Reasons for Moving”]).
1
MARS MILWAUKEE AREA RENTERS STUDY
USER’S GUIDE
UNIVERSITY OF WISCONSIN SURVEY CENTER UNIVERSITY OF WISCONSIN – MADISON
1800 UNIVERSITY AVENUE MADISON, WISCONSIN 53726
608-262-8403 (FAX) 608-262-9032
2
MARS MILWAUKEE AREA RENTERS STUDY
KEY PERSONNEL
Matthew Desmond, Ph.D. Principal Investigator Department of Sociology Harvard University 640 William James Hall 33 Kirkland Street Cambridge, MA 02138 Phone: (617) 495-4751 Email: [email protected] Jeffrey Blossom Senior GIS Specialist Center for Geographic Analysis Harvard University Phone: (617) 496-6063 Email: [email protected] Kerryann DiLoreto Senior Project Director University of Wisconsin Survey Center Phone: (608) 265-6598 Email: [email protected] Charles Palit, Ph.D. Sampling Statistician University of Wisconsin Email: [email protected] John Stevenson Associate Director University of Wisconsin Survey Center Phone: (608) 262-9032 E-mail: [email protected]
3
MARS MILWAUKEE AREA RENTERS STUDY RESEARCH TEAM Weihua An, Ph.D. Assistant Professor of Sociology and Statistics Indiana University Email: [email protected] Carl Gershenson Doctoral Student in Sociology Harvard University Email: [email protected] Barbara Kiviat Doctoral Student in Sociology and Social Policy Harvard University Email: [email protected] Kristin Laurel Perkins Doctoral Student in Sociology and Social Policy Harvard University Email: [email protected] Tracey Shollenberger, Ph.D. Research Associate Urban Institute Email: [email protected] Adam Travis Doctoral Student in Sociology and Social Policy Harvard University Email: [email protected] Nathan Wilmers Doctoral Student in Sociology Harvard University Email: [email protected]
4
MILWAUKEE AREA RENTERS STUDY
I. Introduction
Designed to collect new data related to housing, poverty, and urban life, the Milwaukee Area
Renters Study (MARS) is an in-person survey of 1,086 households in Milwaukee. One person
per household, usually an adult leaseholder, was interviewed. The MARS instrument was
comprised of more than 250 unique items and administered in-person in English and Spanish.
The University of Wisconsin Survey Center supervised data collection, which took place
between 2009 and 2011.1 The MARS sample was limited to renters.2 Nationwide, the majority
of low-income families live in rental housing, and most receive no federal housing assistance
(Desmond 2015). Except in exceptional cities with very high housing costs, the rental
population is comprised of some upper- and middle-class households who prefer renting and
most of the cities’ low-income households who are excluded both from public housing and
homeownership.3 To focus on urban renters in the private market, then, is to focus on the lived
experience of most low-income families living in cities.4 MARS was funded by the John D. and
Catherine T. MacArthur Foundation, through its “How Housing Matters” initiative.
II. Purpose of the Study & Policy Needs
Despite a vast and rich literature on urban poverty, housing problems remain largely absent from
much poverty research. Yet housing dynamics are just as central to our understanding of social
inequality and everyday life in inner-city neighborhoods as are dynamics associated with
families, crime, education, jobs, or welfare. “Housing problems,” Jason DeParle (1996: 52) has
written, “are far more central to the lives of the poor than a number of issues—immunizations,
5
school lunches—that have made recent headlines. The cost of shelter breaks the budget of low-
income Americans, crowds them into violent ghettos, far from good jobs and schools—or both.”
This study was designed to help revitalize the sociology of housing and to restore housing
dynamics to a position of prominence in the fields of social stratification, inequality, and urban
life.
One aspect of housing that disproportionately affects low-income communities—
eviction—exists as perhaps the most understudied process affecting the lives of the urban poor.
This omission is disconcerting, not only because eviction has been linked to homelessness, job
loss, and suicide (Burt 2001; Serby et al. 2006) and not only because the dearth of sociological
research on evictions increasingly hampers the informed design of suitable policy interventions,
but also because studies have found eviction to be a common occurrence in inner-city
neighborhoods (Desmond 2012). Accordingly, the Milwaukee Area Renters Study (MARS) was
designed to generate new data that will allow social scientists to determine if and how certain
aspects of the low-income housing market—and eviction, in particular—affect a variety of social,
psychological, and economic outcomes. A rigorous analysis of eviction’s antecedents,
consequences, and social ramifications advances our knowledge of urban poverty and low-
income housing and is fundamental to developing effective policy initiatives. Accordingly,
MARS was designed to gather data that enables social scientists to determine if eviction (and
other low-income housing dynamics) affects a variety of outcomes organized under five rubrics:
residential stability, poverty, health, community, and intimate life.
MARS was designed to respond to three (3) critical policy needs. First, it was designed
to respond to the need to identify the major causes of eviction. Without sound empirical
evidence of the primary causes of eviction, anti-eviction policies are grasping their way in the
6
dark. Because this study will identify the primary causes of eviction, it will inform eviction
prevention policies, shedding light on precisely where and how to intervene. This study,
additionally, responds to the need to understand the prevalence and consequences of eviction.
Because we know neither the frequency nor the consequences of evictions in low-income urban
neighborhoods, we are at a loss when attempting to assign importance to anti-eviction policies
vis-à-vis other policy priorities. How big of a problem is eviction? Should municipalities and
community organizations devote more attention (and money) to preventing eviction or should
they focus on other matters? The MARS study was designed to provide insight into these
questions by offering an accurate estimate of the frequency of (formal and informal) evictions as
well as an assessment of the degree to which eviction brings about a variety of negative social
outcomes. This research, then, not only will inform current eviction prevention policies, but it
will also underscore the need for policymakers to focus their attention on eviction, treating it as
an important social problem implicated in the reproduction of urban poverty, homelessness, and
a host of other negative outcomes.
Second, this study was designed to respond to the need to document and eradicate
housing discrimination. Since the passage of the Fair Housing Act in 1968, policymakers have
been concerned primarily with discrimination when it comes to buying or renting housing. Yet
they have virtually ignored the degree to which discrimination influences the eviction decision.
Our efforts to monitor and reduce housing discrimination, in other words, have been almost
wholly concentrated on getting in; we have overlooked, meanwhile, discrimination involved in
the process of getting (put) out. This study is the first of its kind to examine if protected groups
are evicted at higher rates, net of other important factors. If this study produces evidence of
discrimination in the eviction process, then the policy implication would be to expand equal
7
opportunity programs to prevent certain classes of renters from being disproportionately targeted
for eviction. To fully monitor discrimination, then, policymakers need to focus not only on the
front end of the housing process (the freedom to obtain housing anywhere) but also on the back
end (the freedom to maintain housing anywhere).
Third, MARS was designed to respond to the need to understand how housing dynamics
are implicated in the reproduction of urban poverty and other social problems. Educational
inequality and underachievement are problems that cannot be solved in the school alone. Health
disparities must be addressed beyond the antiseptic walls of the hospital. This study was
designed to draw connections between housing problems and a variety of non-housing related
outcomes. In particular, it seeks to identify some ways in which housing dynamics perpetuate
urban poverty and, in so doing, will inform anti-poverty initiatives. These data will allow policy
researchers to identify possible ways in which we can improve citizens’ quality of life—their
health, education, economic stability, civic engagement, and so forth—by centering efforts on
housing. By pulling housing back to the center of urban policy analysis—by generating evidence
of the ways in which low-income housing dynamics (and eviction, in particular) are implicated
in the reproduction of urban poverty, racial inequality, health disparities, community
disorganization, relational instability, and so forth—this study hopes to elevate housing issues to
a more prominent position on the national agenda.
III. Sample Design
Dr. Charles Palit (Survey Statistician, University of Wisconsin-Madison) designed the MARS
sample.
Setting. In its socioeconomic profile, Milwaukee is a fairly typical Midwestern city, one
8
marked by a steady erosion of economic prosperity since the 1970s, owing mainly to the flight of
manufacturing jobs and the rise of racially segregated neighborhoods. Milwaukee’s racial
composition, population size, and unemployment rate is similar to those of many other midsize
American cities, although its racial segregation is more acute than most. Just over half of
Milwaukee’s housing units (52%) are occupied by renters, similar to the proportions of Chicago,
Houston, Dallas, San Diego, Columbus, and Baltimore (National Multi Housing Council 2009).
In terms of median rent, Milwaukee County falls in the most expensive third of the country,
ranking 1,420th out of 4,763 counties in the United States and Puerto Rico. Cities with similar
rent distributions include Portland, OR; Charlotte; Gary; and Baton Rouge (U.S. Department of
Housing and Urban Development 2009). Renter protections in Milwaukee are fairly typical.
Cities with a stalwart tradition of tenant unionizing and an economically-diverse rental
population—e.g., New York, Boston—tend to boast of toothier tenant protections than those,
like Milwaukee, in which most middle- and upper-class households own their home (Manheim
1989).
Table A1 (in the appendix) displays economic and demographic information for the fifty
most populous cities in the United States, Milwaukee ranking 30th on the list. Milwaukee’s
median household income is lower than other large cities’ ($35,851 vs. the fifty-city median of
$47,425). Several Rust Belt cities (e.g., Cleveland, Baltimore, Detroit, Philadelphia,
Indianapolis) as well as Southern and Southwestern cities (e.g., Miami, Memphis, El Paso,
Tucson, Tulsa) have similar median household incomes. The percentage of adults in Milwaukee
who are 25 years or older and have at least a high school education (81%) is comparable to the
fifty-city median of 84%. With respect to racial demographics, Milwaukee has a smaller
percentage of white residents (45% vs. the fifty-city median of 59%), a larger percentage of
9
black residents (40% vs. 19%), and a relatively equal percentage of Hispanic residents (17%).
Other major cities—e.g., Chicago, Atlanta, Charlotte, Washington, DC—have a similar racial
and ethnic makeup.
Power Analysis. The data were conceived as analyzable through two conceptual
frameworks: (1) a finite population framework for estimating the characteristics of the specific
finite population sampled and (2) a super-population framework. For the finite population
framework, assuming a design effect of D = 2 to account for the loss of precision in a clustered
sample design, a sample size of N = 1,000 was found to be able to detect an eviction rate as small
as 10% in the population at the 5% level of statistical significance, CI95[.08; .12]. An analysis of
Milwaukee County eviction records from 2003 to 2007 (Desmond 2012) found that in high-
poverty neighborhoods, where more than 40% of the population lived at or below 150% of the
poverty line, 9.9% of those living in renter-occupied households were evicted each year. This
figure almost certainly underestimates the full extent of evictions, as it is based only on formal,
court-ordered evictions. By surveying the city’s tenants, asking survey questions that document
both formal and informal evictions, and oversampling recently evicted tenants, the MARS in-
sample eviction rate was expected to exceed 10%.
Analyses of the difference between evicted households and non-evicted households for
the most part will be executed using a super-population framework, where the target sample size
(1,000) was expected to yield statistically reliable findings. To take one possible social outcome
of eviction, sensitivity tests suggest that a sample size of N = 1,000 will be adequate to produce
reasonably good estimates of the effect of evictions on family stability (see Figure 1). Estimates
of the differences in percentage effects between evicted households and non-evicted households
generally would have a confidence interval of less than plus or minus 4%. (The actual size of the
10
confidence interval would vary with the percentage for the effect in the pooled sample under the
null hypothesis.) That is, assuming that the incidence of family stability in the real population is
somewhere between 30 and 50%, a percent difference in family stability between evicted and
non-evicted respondents that exceeds 4% will be detected at the .05 significance level. If the
incidence of family stability in the real population falls either below or above the 30 to 50%
range, the sample will be able to detect at the .05 significance level percent differences in family
stability smaller than 4%. (The figure below shows the behavior of the confidence interval in
more detail.) Accordingly, to be well powered to detect meaningful statistical effects, the MARS
sample size goal began as N = 1,000.
Figure 1. Power analysis to determine MARS sample size.
Sample and Neighborhood Quality. Households were selected through multi-stage
stratified sampling. Drawing on Census data, Milwaukee block groups were sorted into three
strata based on racial composition. Block groups were classified as white, black, or Hispanic if
at least two-thirds of their residents were identified as such.5 Then, each of these strata was
subdivided into high- and moderate-poverty census block groups based on the overall income
distribution of each racial or ethnic group in the city.6 Additionally, a probability score was
Anticipated Behavior of CI95 for Difference in Proportions Under a Null Hypothesis of No Difference between Evicted and Non-Evicted
Households
0% 1%
2% 3%
4% 5%
0% 10% 20% 30% 40% 50% 60%
Incidence for Family Stability Variable for Pooled Data
Si ze
o f C
I9 5
11
assigned to each block based on the perceived likelihood that the block contained persons who
had been evicted in the previous two years. We derived this score by drawing on court records
and mapping evictions that occurred in Milwaukee in years prior to the survey being fielded.
Blocks from within each of these six strata (which were based on block group boundaries,
the smallest geographic unit for which income data was available) were randomly selected.
When a block was selected into the sample, files were ordered from Genesys Inc., which
provided up-to-date postal route address lists. Interviewers took these lists and visited every
household in the selected blocks, saturating the targeted areas. Sampled areas were put into the
field systematically. That is, as opposed to fielding all selected blocks at once, the Survey
Center began by strategically fielding the selected blocks it expected would produce the highest
yield of interviews with the types of respondents most valuable to the study’s goals. Once
interviewers saturated those blocks, they moved on to additional blocks, while the Survey Center
monitored the yields as they went.
Field interviewers conducted on-the-spot listing of household dwellings to account for all
households in a selected block, so as to complete the sample frame. Because the sample is
limited to renting households, the majority of blocks selected into the sample (being low-income
areas) had relatively high rental densities7, and interviewers screened out all individuals who
lived in owner-occupied dwellings.
Interviewers visited every household in the selected block, saturating the targeted areas
(see Figure 2). To focus on renting households, interviewers screened out owner-occupied
dwellings. This sampling strategy resulted in renting households from across the city being
included in the study, including those in low-poverty and racially integrated blocks. The MARS
study drew from 168 of 591 unique block groups, representing 28% of Milwaukee
12
neighborhoods. Renting households from across Milwaukee were included in the MARS sample.
Plotting the location of households that participated in the study, Figure 2 shows that households
from multiple parts of the city, and located in neighborhoods with widely varying amounts of
disadvantage, were sampled.
Figure 2. Location and neighborhood disadvantage of households that participated in the Milwaukee Area Renters Study. We created a neighborhood disadvantage scale via factor analysis to measure neighborhood quality, loading seven neighborhood characteristics onto this single scale: median household income, violent crime rate, and the percentages of families below the poverty line, of the population under 18, of residents with less than a high school education, of residents receiving public assistance, and of vacant housing units. The scale is standardized within our sample with a zero mean and a unit standard deviation (see Desmond and An 2015).
As shown in Figure 3, there is considerable variation in neighborhood disadvantage
within the MARS sample. In a weighted sample, our standardized neighborhood disadvantage
13
measure varies from -1.57 to 2.9, a range of 5.7 standard deviation (mean = .79, sd = -.61).
Figure 3. Distribution of neighborhood disadvantage within the MARS sample (N = 1,075).
IV. Mode of Interviewing, Fielded Cases, and Weighting
To bolster response rate and data quality, surveys were administered via in-person interviews
carried out by trained interviewers at tenants’ place of residence. During the first phase of
MARS, interviewers recorded respondents’ answers on paper instruments. Later, these
instruments were entered into a computer program. During the second phase of MARS,
interviewers employed a Computer-Assisted Personal Interviewing (CAPI) technology: entering
respondents’ answers directly into a laptop computer.
All interviewers (eight [8] in all) were recruited, trained, certified, and supervised by the
University of Wisconsin Survey Center. Interviewers underwent three full days of training on
MARS, during which they studied the introductory script and practiced interview questions. A
0 20
40 60
80 Fr
eq ue
nc y
-2 -1 0 1 2 3 Standardized Values of Neighborhood Disadvantage
14
week before interviewers visited selected blocks, all households in those blocks received an
advance mailing (see appendix), which briefly explained the study’s aims and announced
interviewers’ upcoming visits. It is likely that distributing an advance letter to prospective
respondents improved the survey’s response rate and allowed interviewers to quickly establish
rapport with respondents. After knocking on the door and making contact with a resident,
interviewers requested to speak to “an adult whose name is on the lease.” If that adult was not
there, they asked to speak to “the adult most knowledgeable about household financial matters.”
Interviewers followed an introductory script (see appendix)—the script explained the purpose of
the study, offered a $20 incentive, carefully described how the tenants’ responses would be kept
anonymous—and requested an interview.8 When tenants agreed to participate, interviewers
conducted the survey (taking on average 35 minutes), asking tenants questions and recording
their answers on a paper form.
Table 1 displays the total fielded cases and response rates for the MARS sample. The
American Association for Public Opinion Research (AAPOR) offers several ways to compute a
response rate. The most conservative calculation (AAPOR Response Rate 1) places in the
numerator only fully completed interviews and in the denominator refusals and all cases of
unknown eligibility. According to this metric, MARS has a response rate of 83.4%. An
alternative calculation places in the denominator the proportion of unknown eligibility cases that
is equal to the proportion of eligible cases for all known eligibility cases. By so doing, it
estimates what the response rate would have been had we been able to screen all of the unknown
eligibility cases. The percentage of eligible cases in the sample is estimated by: 1 – (Ineligible
cases/[All fielded cases – unknown eligibility]). This metric is known as AAPOR Response Rate
3, and it is 91% for MARS.
15
Total fielded cases 3,379 AAPOR Response rate 1* 83.4% AAPOR Response rate 3** 90.1% AAPOR Cooperation rate 1*** 93.2%
Table 1. Final Dispositions Interviewed respondents
Completed Interviews 988 Partially completed interviews 2 Total 990
Eligible, did not interview Refused 41
Unable to enter building 3 Language barrier, eligible 1 Non-contact after screener completed 25 Total 70
Unknown eligibility Language barrier, unknown eligibility 1
Non-contact, screener not completed 103 Screener refused 21 Total 125
Ineligible
Out of sample/address does not exist 88 Business or government/not a housing unit 17 Institution 3 Subsidized housing unit 101 Vacant 368 Temporary or seasonal residence 1 Owner-occupied 1,746 Full-time college student 19 Block density of college students 146 Block gentrification 82 New residents at previous refusal 23 Total 2,594
16
*RR1: This response rate does not calculate partials with completed interviews. It places all cases of unknown eligibility in the denominator. This is the most conservative response rate.
Interviews (Interviews + Partials) + (Eligible, did not interview) + (Unknown eligibility)
**RR3: This response rate does not calculate partials with completed interviews. In the denominator, it places the proportion of unknown eligibility cases that is equal to the proportion of eligible cases for all known-eligibility cases. (In this study it is 29%). By doing so, it estimates what the response rate would be if we had been able to screen all of the unknown eligibility cases. This response rate is less conservative, but can also be appropriate in many instances. When reporting this response rate, it is important to document the formula as being AAPOR RR3.
Interviews (Interviews + Partials) + (Eligible, did not interview) +
[(estimate of eligible cases in sample^)*(Unknown eligibility)] ^1 – (Ineligible cases/(All fielded cases – unknown eligibility)) ***CR1: This is the proportion of interviews we completed out of the total number of eligible respondents we contacted. Partials are included in the denominator only. This is the most conservative cooperation rate. Interviews
(Interviews + Partials) + (Eligible, did not interview)
Oversample. MARS also includes an oversample of 100 recently evicted tenants, who
were randomly selected from closed eviction cases in Milwaukee County that occurred 12 to 24
months prior to the final fielding of the survey. The total universe of closed cases with eviction
judgments for the study period was extracted from the Milwaukee County Small Claims Court.
These records contained people’s names and addresses from which they were evicted. For the
oversample, we drew random replicates of cases and fielded cases in small representative batches
in a manner that maximized response rate. The UW Survey Center used its extensive system of
tracking and locating resources to locate individuals, administering an in-person survey virtually
identical to the main sample survey.
17
The University of Wisconsin Survey Center maintains first-rate tracking resources and
staff. The Tracking and Locating Department serves to provide support on projects by obtaining
telephone numbers and/or addresses of respondents selected for our surveys. Using a variety of
state-of-the-art locating tools, which include nation-wide credit bureau databases, fee-for-service
locater websites (e.g. Lexus Nexus, DirectoryNet), CD-ROM directories, correctional services
databases, professional web-based information resources, and other “hands-on” investigative
research methods, the Tracking and Locating Department assists the UW Survey Center in
achieving high response rates with difficult-to-reach populations.
The most conservative response rate estimate for the oversample is 28.6%. This estimate
includes selected eviction cases that were never attempted because contact information was
unavailable. An adjusted estimate that removes the cases for which tracing was unsuccessful
returns a response rate of 35.5%. Every selected eviction case was re-traced several times
throughout the study period, but 68 cases were not found in any of those efforts. On the back
end, appropriate weights to surveys generated by oversampling efforts were applied in the master
dataset.
Weights. After data collection, custom design weights for the regular sample and
oversample were calculated by Dr. Charles Palit to reflect the inverse of selection probability,
facilitated by a Lahiri (1951) procedure, based on the demographic characteristics of
Milwaukee’s rental population and adjusted to MARS’s sample size. The Lahiri procedure
allows the sampler to select probability samples (with a probability proportional to size) and to
compute the selection probabilities for the resulting sample. Selection probabilities were then
used to calculate the design weights for the overall sample.
Table 2 compares the weighted and unweighted MARS sample to the broader Milwaukee
18
population along key socioeconomic and demographic indicators. The median annual household
income among MARS respondents is $25,003, considerably lower than that of the Milwaukee
population ($35,851). Only 16% of MARS respondents are married, compared to 41% of
Milwaukeeans; and a fifth of the sample has less than a high school education, compared to
14.8% of Milwaukee adults. Seventeen percent of MARS respondents have a criminal record,
and only 44% are full-time workers. Almost 20% of households in the MARS
Collepals.com Plagiarism Free Papers
Are you looking for custom essay writing service or even dissertation writing services? Just request for our write my paper service, and we'll match you with the best essay writer in your subject! With an exceptional team of professional academic experts in a wide range of subjects, we can guarantee you an unrivaled quality of custom-written papers.
Get ZERO PLAGIARISM, HUMAN WRITTEN ESSAYS
Why Hire Collepals.com writers to do your paper?
Quality- We are experienced and have access to ample research materials.
We write plagiarism Free Content
Confidential- We never share or sell your personal information to third parties.
Support-Chat with us today! We are always waiting to answer all your questions.
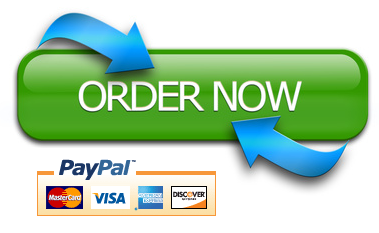