In your view, how do you think businesses can use the principles/concepts/methods we have discussed in this course so far t
For the exclusive use of P. Vongsumedh, 2022.
This document is authorized for use only by Pubordee Vongsumedh in Online MBA 638-001-Spring 2022 taught by IOANNIS BELLOS, George Mason University from Jan 2022 to Feb 2022.
8047 | Core Reading: MANAGING QUEUES 2
1 Introduction ………………………………………………………………………………. 3
2 Essential Reading …………………………………………………………………….. 5
2.1 Managerial Objectives and the Nature of Waiting
Lines …………………………………………………………………………………. 5
2.2 Basic Assumptions, Symbols, and Definitions …………… 6
2.3 Performance Characteristics of Waiting-Line
Systems ……………………………………………………………………………. 8
2.4 An Approximation Formula for Lq ……………………………… 11
2.5 Some Insights into Waiting-Line Systems ………………… 13
2.6 Sample Applications of the Lq Approximation
Formula ………………………………………………………………………….. 16
3 Supplemental Reading ………………………………………………………….. 20
Perceived Versus Actual Waiting Time …………………….. 20
4 Key Terms ………………………………………………………………………………. 23
5 Endnotes …………………………………………………………………………………. 23
6 Index ………………………………………………………………………………………… 25
This reading contains links to online interactive exercises, denoted by a . In order to access these exercises, you will need to have a broadband Internet connection. Verify that your browser meets the minimum technical requirements by visiting http://hbsp.harvard.edu/list/tech-specs.
Copyright © 2013 Harvard Business School Publishing Corporation. All rights reserved. To order copies or request permission to reproduce materials (including posting on academic websites), call 1-800-545-7685 or go to http://www.hbsp.harvard.edu.
Ta bl e of C o nt ents
For the exclusive use of P. Vongsumedh, 2022.
This document is authorized for use only by Pubordee Vongsumedh in Online MBA 638-001-Spring 2022 taught by IOANNIS BELLOS, George Mason University from Jan 2022 to Feb 2022.
8047 | Core Reading: MANAGING QUEUES 3
1 INTRODUCTION
f this is a service economy, why am I still on hold?” ask Frances Frei and Anne Morriss in their book Uncommon Service: How to Win by Putting Customers at the Core of
Your Business.1 This is just one example of waiting that you may have experienced. Have you recently waited in a checkout line at the supermarket? At a “fast”-food restaurant? For a web page to download? All of these are examples of the phenomenon of queuing: the act of waiting for a service to begin. Queuing is an important concern for operating managers in both service and manufacturing environments. They often need to make decisions regarding capacity levels, staffing, and technology that affect the time customers or goods spend waiting for a service.
Waiting lines, or queues, most often form because of variability in a system. In most service businesses, as well as some manufacturing settings, the operating manager’s job is complicated by an inability to specify either the time of arrival of a customer request or the work content of the service to be provided. In a fast-food business, for instance, customers arrive at unpredictable, random times during the day and expect to be able to order from a wide variety of items. The challenge for the restaurant manager is to fulfill customers’ expectations while consuming as few resources as possible. The manager must determine the appropriate staffing level, the proper technology for preparing the food, and the target for the customer’s waiting time. For example, too few personnel and a grill that takes an excessive amount of time to cook burgers may result in a waiting time that’s too long. Too many personnel and a grill that cooks the burgers more quickly (and is more expensive) may provide a short waiting time but decrease profitability.
Queues can also form in a manufacturing environment, where, for example, physical parts may have to wait for a machine to become available. In this reading, we primarily use service examples to explain queuing phenomena. Those interested in manufacturing can easily apply the frameworks and models discussed here.
Waiting lines can result in delays. The consequences of these delays depend on the context. A shorter delay in a hospital emergency room may prevent serious medical complications for patients with heart attacks. Quicker turnaround times for loan processing may increase the number of loan applications and the lender’s profitability. Long waits at a restaurant may cause potential customers to go elsewhere for a meal.a Shorter delays result, in many cases, in better use of labor, equipment, and facilities, and a greater capability for providing customers with an excellent level of service.
In this reading, we present frameworks and tools that managers can use to understand the characteristics of waiting-line systems and improve operations where queues may form. We first discuss queuing-related questions that managers ask in designing a service operation and the nature of waiting-line systems. We then lay out some basic assumptions about those systems and describe various characteristics of them.
a We are reminded of the quote by the famous American baseball player and “philosopher” Yogi Berra about a popular restaurant: “Nobody goes there anymore; it’s too crowded.”
“I
For the exclusive use of P. Vongsumedh, 2022.
This document is authorized for use only by Pubordee Vongsumedh in Online MBA 638-001-Spring 2022 taught by IOANNIS BELLOS, George Mason University from Jan 2022 to Feb 2022.
8047 | Core Reading: MANAGING QUEUES 4
We also present a formula for approximating line length in a system, some of the insights into queuing systems that arise from this formula, and some further applications of the formula. In the Supplemental Reading, we address ways to manage customers’ perceptions of waiting.
For the exclusive use of P. Vongsumedh, 2022.
This document is authorized for use only by Pubordee Vongsumedh in Online MBA 638-001-Spring 2022 taught by IOANNIS BELLOS, George Mason University from Jan 2022 to Feb 2022.
8047 | Core Reading: MANAGING QUEUES 5
2 ESSENTIAL READING
The objective for managers in applying the calculations in this reading is to understand and estimate the various costs and performance characteristics of waiting-line systems in specific service processes so that they can make appropriate design choices. Costs might be related to the number and type of servers; performance characteristics include considerations such as customer waiting time and line length.
Thus, in designing a service, managers might ask the following queue-related questions:
• On average, how many customers will be waiting for service? How long, on average, will a customer wait?
• How many servers are necessary to achieve a specified limit to customer waiting time?
• What number of servers (in a particular situation) leads to an average waiting time for the customers and an average idle time for the servers that minimize the total cost of the service system?
• Should servers be combined into one or more centralized areas?
• Which process improvements should be implemented given different service rates, operating costs, and target cost and service objectives?
• What is the impact of a reduction in the variability of either the time between customer arrivals or the service times?
The answers clearly depend on the relative costs (in a particular situation) of customers and servers. As the above questions imply, a waiting-line system in a service setting has three basic components:
1 Servers
2 Customers, who may arrive at random or unscheduled times
3 Service encounters, which typically vary in their completion times
At times, a customer’s waiting time and a server’s idle time incur easily measurable out-of-pocket costs. For example, every minute a prospective customer waits on the telephone for a customer service representative costs the organization long-distance telephone charges. But at other times, there are no clear costs associated with a waiting customer. Customers queued in restaurants, post offices, banks, airports, and so forth, do not impose direct costs to the service provider, but if the lines become too long, some customers may leave before completing the transaction or choose a competitor the next time they need that service. Thus, an operations manager must plan for waiting lines and make staffing decisions accordingly—all the while striving to manage the ongoing trade- off between excessive service capacity and needless waiting.
It is important for managers to understand that a line can form even if the capacity of the server(s) exceeds customer demand. Waiting time cannot be entirely eliminated
2.1 Ma nag erial O bj ecti ves a nd t he Nat ure of Waiti ng Li nes
For the exclusive use of P. Vongsumedh, 2022.
This document is authorized for use only by Pubordee Vongsumedh in Online MBA 638-001-Spring 2022 taught by IOANNIS BELLOS, George Mason University from Jan 2022 to Feb 2022.
8047 | Core Reading: MANAGING QUEUES 6
because of the variability we mentioned earlier. A large number of customers may arrive over a short period of time, or there might be a few closely spaced service encounters that take an excessive amount of time. Conversely, even if few servers are present and waiting lines prevail, the servers may be idle some of the time if there is a long gap between arrivals or there are some successive short service encounters.
Interactive Illustration 1 shows a graphical representation of a queuing system. There is one server, and service time is always exactly 4 minutes per customer. Therefore, during a 25-minute interval, the server could serve 5 customers and have 5 minutes of idle time. Likewise, it is possible for all 5 customers to be served without having to wait for service. However, that situation occurs only if customer arrivals are evenly spaced during the 25-minute interval.
To run the simulation, click “Start” and then click “Customer Arrival” four times, once for each customer. When the 25 simulated minutes end, you can see data on the length of queues that form the waiting time per customer. Try different arrival patterns to see the effect on performance metrics.
Interactive Illustration 1 Cumulative Arrivals and Departures
The calculations discussed in this reading depend on the following assumptions about waiting-line situations:
1 First come, first served. Customers form single queues while waiting for service and are taken for service on a first-come, first-served basis. Customers move into a server channel (for instance, a teller’s window at a bank) as it becomes available.
2. 2 Basi c Ass um pti ons , Sy mb ols, and D efi nitio ns
For the exclusive use of P. Vongsumedh, 2022.
This document is authorized for use only by Pubordee Vongsumedh in Online MBA 638-001-Spring 2022 taught by IOANNIS BELLOS, George Mason University from Jan 2022 to Feb 2022.
8047 | Core Reading: MANAGING QUEUES 7
2 Servers perform identical services. Servers can also be referred to as channels performing services; thus, the number of servers equals the number of channels. There may be multiple servers operating in parallel to service one line.
3 Random arrivals and service. Customer arrivals occur randomly at an average rate, called the arrival rate. Services are performed at an average rate, called the service rate, at each of the servers. Note that the units of average arrival rate and average service rate are customers per unit time. The reciprocal value of the service rate, service time, is the average time for serving a customer and is measured in time units. Similarly, the reciprocal value of the average arrival rate is the average time between arrivals, called the inter-arrival time, and is also measured in time units.
4 Statistical equilibrium (steady state). On average, the capability to process customers must be greater than the rate at which they arrive; otherwise, arriving customers might have to wait in lineb indefinitely. The average capability of the system to process customers per unit time is given by the average total service rate, which equals (number of servers) · (service rate per server). The average number of customers requiring service per unit time is given by the arrival rate. In transient situations, such as system startup—for example, when an amusement park first opens in the morning—the number in the queue and in service depends on the number of customers who waited for the system to go into operation and on how long the system has been in operation. Over time, the impact of the initial conditions tends to dampen out, and the number of customers in the system and in line is independent of time. The calculations described in this reading are not appropriate for transient situations.
5 Infinite source of potential customers. We assume an infinite source of potential customers. We may also use these calculations when the population of the queuing system is not greater than 1% of the population of the source of potential customers.
6 Appropriate queue behavior. We assume the arrival rate equals the demand rate. This assumption implies no instances of: a. balking (potential recipients refusing to join the queue) b. reneging (customers in the queue leaving before being served) c. cycling (recipients of service returning to the queue following service)
7 Uniform service effectiveness. The effectiveness of service at each server channel is uniform over time and throughout the system.
b The use of in line and on line to describe a person in a queue varies regionally in the United States. The author is from Philadelphia, so he has chosen to use in line.
For the exclusive use of P. Vongsumedh, 2022.
This document is authorized for use only by Pubordee Vongsumedh in Online MBA 638-001-Spring 2022 taught by IOANNIS BELLOS, George Mason University from Jan 2022 to Feb 2022.
8047 | Core Reading: MANAGING QUEUES 8
2. 3 Perfo rma nce C hara ct eristi cs of Waiti ng -Li ne Sy stems
The calculations in this reading also use the symbols and definitions outlined in Table 1.
Table 1 Definitions of Queuing Parameters
Facility A total servicing unit; a facility may be composed of several channels (as defined below) or as few as one
Channel A servicing point within a facility A Arrival rate; average number of arrivals into the system per unit of time
S Service rate (per server); average number of services per unit of time per channel
Ts Average service time (1/S)
m Number of servers or number of channels in the facility mS Average service rate of the facility (m · service rate per server) u A/mS = (arrival rate)/(m · service rate per server ) = utilization or utilization factor Ns Average number of customers in service
L Average number of customers in the system; those being served plus the waiting line
Lq Average number of customers waiting in line; this number may be approximated using a formula provided later in this reading
W Total average time in the system
Wq Average time spent waiting
Now that we have established the assumptions and definitions provided in the preceding section, we can describe the performance characteristics of waiting-line systems (utilization factor, average number of customers in service, average number of customers waiting in the queue, average number of customers in the system, expected waiting time in the queue, expected total average time in the system, and expected costs) and explore the relationships among them.
Utilization factor (u): The utilization factor measures the percentage of time that servers are busy with customers.
Note that u is a percentage and is a unit-less number. Consider a system with an arrival rate of 10 people per hour, where each server can service 11 per hour. For m = 1 server, the utilization is u = 10/11 = 91%. This indicates that the server is busy 91% of the time. As you can see in Figure 1, the utilization factor decreases as the number of servers increases.
A u
mS =
For the exclusive use of P. Vongsumedh, 2022.
This document is authorized for use only by Pubordee Vongsumedh in Online MBA 638-001-Spring 2022 taught by IOANNIS BELLOS, George Mason University from Jan 2022 to Feb 2022.
8047 | Core Reading: MANAGING QUEUES 9
Figure 1 Utilization Factor
Average number of customers in service (Ns): The average number of customers in service is A/S, derived by multiplying the utilization factor (u) times the number of servers (m). (This does not include the number of customers waiting in line.)
Ns will vary as a direction function of the utilization, which is a function of the arrival and service rates of the system, as shown above.
Average number of customers waiting in the queue (L q): The average number of customers waiting in the queue excludes the customers that are being served. For most systems, L q cannot be calculated directly because of the complex interactions caused by the probability distributions describing the arrival and service processes; its value must be approximated. An approximation formula (or queuing approximation) for this performance characteristic is presented in the next section. We will show that the queue length is a function of the number of servers, the utilization factor, and the arrival and service variability.
The remaining performance characteristics of waiting-line systems all depend on the value of L q—either observed empirically or estimated from the approximation formula described in the next section.
Average number of customers in the system (L): By definition, the average number of customers in the system is equal to the average number of customers in line plus the average number of customers in service. That is:
Expected waiting time in the queuec (W q): The expected waiting time can be determined by dividing the average queue length by the average arrival rate:
c There is often a large difference between the actual waiting time and the perceived waiting time. Methods for managing customers’ perceptions are discussed in the Supplemental Reading.
s A A
N m u m mS S
= ⋅ = ⋅ =
q sL L N= +
= qq L
W A
For the exclusive use of P. Vongsumedh, 2022.
This document is authorized for use only by Pubordee Vongsumedh in Online MBA 638-001-Spring 2022 taught by IOANNIS BELLOS, George Mason University from Jan 2022 to Feb 2022.
8047 | Core Reading: MANAGING QUEUES 10
The formula that relates the waiting time and line length is commonly referred to as Little’s Law. This relationship, L q = A · W q for the queue and L = A · W for the entire system, was initially proven by John D. C. Little.2 The formula states that the length of a line is directly related to the time spent in the line (or that the number of customers or items in the system is directly related to the total time spent in the system). Given any two of the parameters, the third can be determined. For example, if we know that the average length of the line at a doughnut store is 3.5 customers, and we know that the average time spent in line is two minutes, we can infer that the arrival rate to the store is given by:
3.5 1.75 customers minute 105 customers hour
2 q
q
L A
W = = = =
Figure 2 shows the impact of changes in the queue length on the average waiting time. Obviously, the longer the line, the longer the wait. The slope of the line is the arrival rate. The slope is not the service rate because Little’s Law deals with average wait time and queue lengths at steady state. The departure rate from a steady state system must equal the arrival rate. Thus, the relationship between line length and queue time is a function of the average rate at which the line decreases. This average rate must weight busy and nonbusy times.
Figure 2 Little’s Law
Expected total average time in the system (W): Because the average service time per person or item is equal to the quantity 1/S, the total average time expected in the system is:
The above relationships among W q , L q , A, and S are not dependent on any assumptions regarding the distribution of arrival and service times but instead are true in general.
Expected costs: The expected costs of a queuing system to the organization are usually determined by some function of the number of servers and the expected customers’ waiting time or the number of customers in the system. The cost of waiting
1 qW = W S
+
For the exclusive use of P. Vongsumedh, 2022.
This document is authorized for use only by Pubordee Vongsumedh in Online MBA 638-001-Spring 2022 taught by IOANNIS BELLOS, George Mason University from Jan 2022 to Feb 2022.
8047 | Core Reading: MANAGING QUEUES 11
can be either an out-of-pocket cost or an opportunity cost. When the cost of waiting is known, the cost of providing service may be added to the cost of waiting to obtain a total system cost. For example, consider a queuing system with three channels and with a cost per channel of $25 per hour. The total channel cost is $75 per hour. If the average waiting time per customer is 15 minutes (0.25 hours), and the opportunity cost of waiting to the firm is $15 per hour per customer, each customer arrival would cost (0.25 hours) · $15 = $3.75 per customer. If 10 customers arrived per hour, the cost of waiting would be $37.50 per hour. Added to the $75 cost of service, the total cost would be $112.50 per hour.
Adding an extra server would cost $25 per hour. The server would be added if the total system cost dropped to less than $112.50 per hour. Given that the cost of servers is now 4 · $25 = $100, four servers would be optimal if the total opportunity cost of waiting is now less than $12.50 per hour because the new total system cost is $100 + the cost of waiting. This implies that the average waiting time for a customer would need to be less than 0.0833 hours ($12.50/[$15 waiting cost per hour per customer · 10 customers per hour]). Note that at 0.0833 hours per customer, the waiting cost per customer would be (0.0833 hours) · $15 = $1.25 per customer. Multiplying by the 10 customers per hour yields a total cost of waiting of $12.50 per hour.
Often, the waiting cost for customers is not known. Although there may be an opportunity cost to the individuals or items in line, there may be no direct cost to the firm. In these cases, managers may set targets for the average waiting time or for probabilities of waiting no more than a specified period of time. They may also consider some trade-offs. Consider the previous example. If we did not know or could not estimate the $15/hour waiting time cost, we might ask, “Would you spend $25/hour to reduce the average waiting time from 0.25 hours to x hours?” (where x is the estimated average waiting time for customers in the new system). Note that rather than have an explicit numerical answer to this question, we must rely on managerial judgment. One could also factor in the lost revenue per customer and the probability of losing customers if the lines are excessively long.
We have ignored the cost of the customer(s) in service (that is, customers who are being served and so are not waiting in a queue) in our calculations. This may be included but will not affect how many servers that managers decide to include in the system because the time in service is not a function of the number of servers in these examples. (Recall that N s = A/S, and neither A nor S is a function of the number of servers.) If managers had to decide the actual service rate at each channel, it would be necessary to include the time spent in service as part of the total system cost.
In this section, we present an approximation formula for calculating the value of L q . Recall that once we have this value for the line length, other important performance characteristics, such as W q , W, and L, can be determined. The relationships among W q , L q , W, L, A, and S, described in the preceding section, are not dependent on the nature or shape of the probability distributions describing the arrival and service processes. The values of the measures W q , L q , W, and L depend highly on the assumptions regarding these distributions, as we will see.
The L q approximation formula below can be used for approximating the line length of a queuing system. It is often referred to as the Sakasegawa approximation.3 Although many approximation formulas are available for queuing systems, we present this
2. 4 A n Ap p roxi matio n Form ula fo r L q
For the exclusive use of P. Vongsumedh, 2022.
This document is authorized for use only by Pubordee Vongsumedh in Online MBA 638-001-Spring 2022 taught by IOANNIS BELLOS, George Mason University from Jan 2022 to Feb 2022.
8047 | Core Reading: MANAGING QUEUES 12
particular formula for its relative ease of use, its use for developing insight regarding queuing systems, and the relative quality of its approximation to “true” values for systems with significant waiting lines.
( )
( ) ( ) ( )( )2 22 1
1 2
m IAT ST
q
CV CVu L
u
+ + ≈ ⋅
−
u = the utilization factor, as defined above m = the number of servers
CV IAT = the coefficient of variation of the inter-arrival times CV ST = the coefficient of variation of the service time
The coefficient of variation of a probability distribution provides a relative measure of its variability and is defined as the standard deviation of a distribution divided by its mean. It is used in recognition of the fact that the magnitude of the standard deviation of a distribution is more relevant with respect to the size of its mean than as an absolute measure. Thus, a service time distribution with a standard deviation of 10 minutes is much more significant if the mean is 10 minutes than if the mean is 50 minutes.d
Whereas the general principles of queue management—such as Little’s Law, the effects of increasing variability, and the nature of pooling or the combination of queues (which we’ll discuss later)—are applicable in a wide variety of situations, the L q approximation formula is more limited and should be used only in situations with first come, first served arrivals; a single type of customer; no limit on the waiting room; and stationary, steady state conditions. For systems with finite waiting areas, priority queues, different customer types, and overflow queues, more-sophisticated models and simulation tools are available. For example, the Erlang loss formula can be used for systems where customers are turned away when the system is full.4 Many Excel add-ins that handle specific situations can be downloaded from the Internet (see, for example, Queuing ToolPak from the University of Alberta School of Business). The Extend simulation package is also often used to model complex queuing situations.
In addition, the Lq approximation is useful for calculating the average line length and waiting time. In many instances, we are interested in the probability that line lengths or wait times will exceed a certain threshold. For example, a call center’s goal may be to have an average hold time of 30 seconds, with no more than a 5% chance of waiting 45 seconds or longer. In this situation, more-detailed results on the shape of the waiting time distribution would be needed to determine staffing requirements.
d In general, distributions with a CV < 0.75 are considered low variability, 0.75 < CV < 1.33 are considered moderate variability, and CV > 1.33 are considered high variability. The actual values of CV can be measured from empirical data, as in the previous example. Often, for convenience and with no other information, we assume that CV = 1.0. If the probability distribution describing the number of arrivals in a given period is Poisson, a common occurrence, then CVIAT = 1.0; if the probability distribution describing the service time is exponential, a common assumption, then CVIAT = 1.0.
For the exclusive use of P. Vongsumedh, 2022.
This document is authorized for use only by Pubordee Vongsumedh in Online MBA 638-001-Spring 2022 taught by IOANNIS BELLOS, George Mason University from Jan 2022 to Feb 2022.
8047 | Core Reading: MANAGING QUEUES 13
At first glance, the L q formula appears intimidating; however, its form provides some general insights into queuing systems. Here we discuss these and …
,
Table oof Conteents
For the exclusive use of P. Vongsumedh, 2022.
This document is authorized for use only by Pubordee Vongsumedh in Online MBA 638-001-Spring 2022 taught by IOANNIS BELLOS, George Mason University from Jan 2022 to Feb 2022.
8007 | Core Reading: PROCESS ANALYSIS 2
1 Introduction …………………………………………………………………………………. 3
2 Essential Reading ……………………………………………………………………….. 5
2.1 Process Flow Diagrams ………………………………………………….. 5
2.2 Batch Processes ………………………………………………………………. 8
2.3 Assessing Capacity ……………….
Collepals.com Plagiarism Free Papers
Are you looking for custom essay writing service or even dissertation writing services? Just request for our write my paper service, and we'll match you with the best essay writer in your subject! With an exceptional team of professional academic experts in a wide range of subjects, we can guarantee you an unrivaled quality of custom-written papers.
Get ZERO PLAGIARISM, HUMAN WRITTEN ESSAYS
Why Hire Collepals.com writers to do your paper?
Quality- We are experienced and have access to ample research materials.
We write plagiarism Free Content
Confidential- We never share or sell your personal information to third parties.
Support-Chat with us today! We are always waiting to answer all your questions.
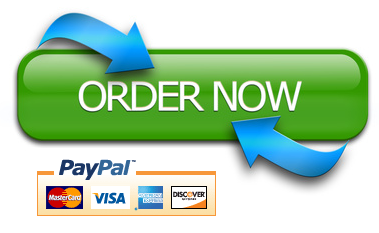