python convolutional neural network Exercise
USE Jupyter Notebook
NB: Please only do Tasks 1-2
We will combine what we’ve learned about convolution, max-pooling and feed-forward layers, to build a ConvNet classifier for images.
Given Code:
in[]from __future__ import absolute_import, division, print_function
# Prerequisits
!pip install pydot_ng
!pip install graphviz
!apt install graphviz > /dev/null
# import statements
import tensorflow as tf
import tensorflow.contrib.eager as tfe
import numpy as np
import matplotlib.pyplot as plt
from IPython import display
%matplotlib inline
# Enable the interactive TensorFlow interface, which is easier to understand as a beginner.
try:
tf.enable_eager_execution()
print(‘Running in Eager mode.’)
except ValueError:
print(‘Already running in Eager mode’)
in[]cifar = tf.keras.datasets.cifar10
(train_images, train_labels), (test_images, test_labels) = cifar.load_data()
cifar_labels = [‘airplane’, ‘automobile’, ‘bird’, ‘cat’, ‘deer’, ‘dog’, ‘frog’, ‘horse’, ‘ship’, ‘truck’]
in[]# Take the last 10000 images from the training set to form a validation set
train_labels = train_labels.squeeze()
validation_images = train_images[-10000:, :, :]
validation_labels = train_labels[-10000:]
train_images = train_images[:-10000, :, :]
train_labels = train_labels[:-10000]
in[]print(‘train_images.shape = {}, data-type = {}’.format(train_images.shape, train_images.dtype))
print(‘train_labels.shape = {}, data-type = {}’.format(train_labels.shape, train_labels.dtype))
print(‘validation_images.shape = {}, data-type = {}’.format(validation_images.shape, validation_images.dtype))
print(‘validation_labels.shape = {}, data-type = {}’.format(validation_labels.shape, validation_labels.dtype))
in[]plt.figure(figsize=(10,10))
for i in range(25):
plt.subplot(5,5,i+1)
plt.xticks([])
plt.yticks([])
plt.grid(‘off’)
in[]# Define the convolutinal part of the model architecture using Keras Layers.
model = tf.keras.models.Sequential([
tf.keras.layers.Conv2D(filters=48, kernel_size=(3, 3), activation=tf.nn.relu, input_shape=(32, 32, 3), padding=’same’),
tf.keras.layers.MaxPooling2D(pool_size=(3, 3)),
tf.keras.layers.Conv2D(filters=128, kernel_size=(3, 3), activation=tf.nn.relu, padding=’same’),
tf.keras.layers.MaxPooling2D(pool_size=(3, 3)),
tf.keras.layers.Conv2D(filters=192, kernel_size=(3, 3), activation=tf.nn.relu, padding=’same’),
tf.keras.layers.Conv2D(filters=192, kernel_size=(3, 3), activation=tf.nn.relu, padding=’same’),
tf.keras.layers.Conv2D(filters=128, kernel_size=(3, 3), activation=tf.nn.relu, padding=’same’),
tf.keras.layers.MaxPooling2D(pool_size=(3, 3)),
in[]model.summary()
in[]model.add(tf.keras.layers.Flatten()) # Flatten “squeezes” a 3-D volume down into a single vector.
model.add(tf.keras.layers.Dense(1024, activation=tf.nn.relu))
model.add(tf.keras.layers.Dropout(rate=0.5))
model.add(tf.keras.layers.Dense(1024, activation=tf.nn.relu))
model.add(tf.keras.layers.Dense(10, activation=tf.nn.softmax))
in[]tf.keras.utils.plot_model(model, to_file=’small_lenet.png’, show_shapes=True, show_layer_names=True)
display.display(display.Image(‘small_lenet.png’))
in[]batch_size = 128
num_epochs = 10 # The number of epochs (full passes through the data) to train for
# Compiling the model adds a loss function, optimiser and metrics to track during training
model.compile(optimizer=tf.train.AdamOptimizer(),
loss=tf.keras.losses.sparse_categorical_crossentropy,
metrics=[‘accuracy’])
# The fit function allows you to fit the compiled model to some training data
model.fit(x=train_images,
y=train_labels,
batch_size=batch_size,
epochs=num_epochs,
validation_data=(validation_images, validation_labels.astype(np.float32)))
print(‘Training complete’)
in[]metric_values = model.evaluate(x=test_images, y=test_labels)
print(‘Final TEST performance’)
for metric_value, metric_name in zip(metric_values, model.metrics_names):
print(‘{}: {}’.format(metric_name, metric_value))
in[]img_indices = np.random.randint(0, len(test_images), size=[25])
sample_test_images = test_images[img_indices]
sample_test_labels = [cifar_labels[i] for i in test_labels[img_indices].squeeze()]
predictions = model.predict(sample_test_images)
max_prediction = np.argmax(predictions, axis=1)
prediction_probs = np.max(predictions, axis=1)
in[]plt.figure(figsize=(10,10))
for i, (img, prediction, prob, true_label) in enumerate(
zip(sample_test_images, max_prediction, prediction_probs, sample_test_labels)):
plt.subplot(5,5,i+1)
plt.xticks([])
plt.yticks([])
plt.grid(‘off’)
plt.imshow(img)
plt.xlabel(‘{} ({:0.3f})’.format(cifar_labels[prediction], prob))
plt.ylabel(‘{}’.format(true_label))
NB: Please only do Tasks 1-2
Tensorflow documentation: from ‘tensorflow.org’ website: search: ‘tf.keras.layers.BatchNormalization’
research paper: search on web: ‘proceedings.mlr.press/v37/ioffe15.pdf’
NB: Please only do Tasks 1-2
Collepals.com Plagiarism Free Papers
Are you looking for custom essay writing service or even dissertation writing services? Just request for our write my paper service, and we'll match you with the best essay writer in your subject! With an exceptional team of professional academic experts in a wide range of subjects, we can guarantee you an unrivaled quality of custom-written papers.
Get ZERO PLAGIARISM, HUMAN WRITTEN ESSAYS
Why Hire Collepals.com writers to do your paper?
Quality- We are experienced and have access to ample research materials.
We write plagiarism Free Content
Confidential- We never share or sell your personal information to third parties.
Support-Chat with us today! We are always waiting to answer all your questions.
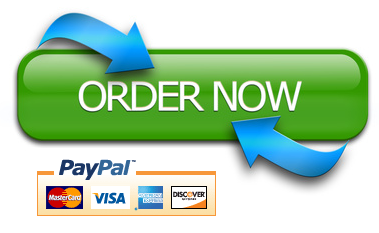