For Question 1, you will load a dataset into JASP and perform a correlation analysis and a simple regression to determine the relationship betwee
Please see assignment instructions for this assignment you will need to use a free software program named JASP to complete this assignment.
When saving PDF doc please save as Question 2 or Question 4 only
LDL_Chol | Hours_TV |
168 | 2.595041056 |
170 | 2.797427517 |
170 | 3.39 |
164 | 3.159039955 |
159 | 3.092402595 |
168 | 3.7 |
165 | 3.249408427 |
156 | 2.888637523 |
172 | 2.897454286 |
170 | 2.684321261 |
165 | 2.766328258 |
168 | 2.649433141 |
168 | 2.811045366 |
166 | 3.64 |
167 | 4.16 |
163 | 3.35 |
161 | 2.647565999 |
168 | 3.79 |
170 | 2.795904577 |
171 | 3.045671761 |
165 | 3.66 |
162 | 2.626149483 |
175 | 3.01 |
169 | 3.56 |
170 | 4.41 |
176 | 3.03 |
179 | 3.35 |
173 | 3.090394765 |
176 | 3.99 |
170 | 2.715664635 |
172 | 2.697116546 |
175 | 3.01 |
172 | 3.251243031 |
173 | 3.83 |
177 | 4.06 |
171 | 3.27 |
173 | 3.54 |
170 | 3.84 |
163 | 3.01218205 |
173 | 4.04 |
169 | 2.686413374 |
170 | 3.43 |
171 | 3.71 |
174 | 4.41 |
178 | 3.26 |
174 | 3.61 |
176 | 4.11 |
176 | 3.250547806 |
171 | 3.53 |
176 | 4.55 |
176 | 3.25 |
172 | 3.55 |
173 | 3.5 |
176 | 3.23 |
172 | 3.12 |
171 | 4.27 |
173 | 3.31 |
176 | 3.098778487 |
171 | 3.12 |
182 | 3.91 |
180 | 3.91 |
183 | 4.33 |
170 | 2.987071673 |
176 | 3.10493154 |
182 | 3.56 |
174 | 3.27 |
178 | 3.6 |
176 | 2.866647124 |
169 | 3.73 |
172 | 2.577060443 |
176 | 3.222755354 |
175 | 3.13 |
170 | 3.68 |
177 | 4.21 |
180 | 4.21 |
176 | 3.99 |
171 | 2.57324386 |
183 | 3.66 |
177 | 3.23 |
169 | 3.82 |
167 | 4.14 |
171 | 2.798365361 |
170 | 3.23 |
174 | 3.91 |
170 | 4.21 |
170 | 2.64554954 |
173 | 3.56 |
173 | 3.156208077 |
180 | 4.02 |
177 | 3.65 |
168 | 3.303111622 |
179 | 4 |
163 | 3.48 |
179 | 4.43 |
168 | 2.363621942 |
170 | 3.17 |
171 | 3.52 |
171 | 4.1 |
172 | 3.413805439 |
171 | 3.411823675 |
,
caseno | age | weight | heart_rate | gender | VO2max |
1 | 27 | 70.47 | 150 | Male | 55.79 |
2 | 63 | 50.34 | 144 | Female | 35 |
3 | 36 | 87.65 | 162 | Male | 42.93 |
4 | 26 | 89.8 | 129 | Female | 28.3 |
5 | 24 | 103.02 | 143 | Male | 40.56 |
6 | 29 | 77.37 | 152 | Female | 33 |
7 | 24 | 82.48 | 175 | Male | 43.48 |
8 | 27 | 75.94 | 160 | Female | 30.38 |
9 | 25 | 97.11 | 148 | Male | 40.17 |
10 | 22 | 78.42 | 125 | Female | 36.01 |
11 | 30 | 88.02 | 155 | Male | 44.22 |
12 | 45 | 74.47 | 123 | Female | 38.76 |
13 | 25 | 75.98 | 147 | Female | 33.09 |
14 | 36 | 58.97 | 139 | Female | 44.81 |
15 | 23 | 111.8 | 145 | Male | 31.94 |
16 | 29 | 79.81 | 128 | Female | 34.48 |
17 | 37 | 56.18 | 163 | Male | 47.23 |
18 | 30 | 86.13 | 156 | Male | 45.06 |
19 | 36 | 87.3 | 127 | Male | 55.12 |
20 | 36 | 88.52 | 147 | Male | 45.58 |
21 | 26 | 68.52 | 147 | Female | 37.52 |
22 | 31 | 76.7 | 129 | Female | 36.27 |
23 | 30 | 62.51 | 140 | Male | 62.5 |
24 | 24 | 60.9 | 172 | Female | 37.09 |
25 | 26 | 53.43 | 158 | Male | 44.27 |
26 | 23 | 68.52 | 133 | Female | 40.34 |
27 | 45 | 72.37 | 147 | Male | 55.19 |
28 | 38 | 82.16 | 144 | Female | 49.87 |
29 | 30 | 90.92 | 177 | Male | 38.06 |
30 | 21 | 95.51 | 139 | Male | 48.13 |
31 | 39 | 73.14 | 108 | Female | 42.42 |
32 | 26 | 62.59 | 111 | Male | 48.23 |
33 | 28 | 91.38 | 151 | Male | 42.96 |
34 | 41 | 94.29 | 145 | Male | 42.53 |
35 | 40 | 58.07 | 161 | Male | 40.9 |
36 | 25 | 90.25 | 132 | Female | 27.35 |
37 | 21 | 78.45 | 111 | Female | 38.7 |
38 | 22 | 74.78 | 147 | Male | 62.13 |
39 | 33 | 85.13 | 156 | Male | 45.69 |
40 | 28 | 101.25 | 133 | Male | 40.73 |
41 | 21 | 58.94 | 154 | Male | 41.82 |
42 | 37 | 101.06 | 131 | Male | 35.6 |
43 | 21 | 97.75 | 136 | Male | 42.2 |
44 | 22 | 72.5 | 123 | Male | 45.3 |
45 | 27 | 88.45 | 175 | Male | 40.02 |
46 | 24 | 72.29 | 187 | Male | 47.17 |
47 | 31 | 94.59 | 134 | Male | 44.43 |
48 | 28 | 115.42 | 134 | Male | 38.12 |
49 | 30 | 51.96 | 134 | Female | 50.05 |
50 | 31 | 89.35 | 129 | Male | 48.64 |
51 | 33 | 62.01 | 172 | Female | 36.49 |
52 | 23 | 68.03 | 127 | Male | 61.71 |
53 | 29 | 79.39 | 133 | Female | 33.84 |
54 | 25 | 81.91 | 138 | Female | 51.2 |
55 | 32 | 64 | 178 | Female | 35 |
56 | 29 | 59.78 | 122 | Female | 47.71 |
57 | 21 | 71.03 | 122 | Male | 52.3 |
58 | 34 | 50 | 183 | Female | 42 |
59 | 25 | 80.98 | 119 | Male | 55.66 |
60 | 31 | 112.59 | 116 | Male | 37.34 |
61 | 64 | 73.38 | 117 | Female | 40.58 |
62 | 26 | 62.85 | 149 | Male | 60.55 |
63 | 45 | 68.29 | 145 | Female | 37.93 |
64 | 21 | 94.68 | 131 | Male | 44.94 |
65 | 28 | 103.23 | 186 | Male | 35.01 |
66 | 45 | 81.01 | 169 | Male | 45.57 |
67 | 22 | 69.02 | 150 | Female | 36.63 |
68 | 20 | 55 | 141 | Female | 60 |
69 | 22 | 73.44 | 117 | Female | 40.52 |
70 | 33 | 60.31 | 119 | Female | 47.92 |
71 | 38 | 85.94 | 153 | Male | 45.84 |
72 | 30 | 64.65 | 141 | Male | 60.92 |
73 | 52 | 88.83 | 109 | Male | 32 |
74 | 22 | 82.41 | 146 | Male | 49.4 |
75 | 23 | 63 | 149 | Female | 50 |
,
Jeffreys's Amazing Software Program ($0.00)
· JASP v19.03 available for free download
o Download JASP – JASP – Free and User-Friendly Statistical SoftwareLinks to an external site.
You have two simple problems. For Question 1, you will load a dataset into JASP and perform a correlation analysis and a simple regression to determine the relationship between time spent watching television and cholesterol numbers. Once you answer the questions about the analyses, you will export your results to pdf (Save each PDF as Question 2, Question 4 ). In Question 3, you will load a dataset into JASP and perform a multiple regression analysis to estimate a regression equation to predict aerobic performance based on several predictors. Once you perform the analyses requested and answer the questions, you will export your results to pdf save each PDF as Question 2, Question 4
In this dataset, the 100 respondents were included in a study which recorded the number of hours spent watching television along with the LDL cholesterol value in an attempt to determine if television leads to a sedentary lifestyle which, in turn, increases the LDL cholesterol concentration.
Load the dataset into JASP then follow the directions below.
Step 1 – Correlation.
Open a new correlation (classical) module in JASP and name it Q1 Correlation Your Name. Enter both variables and perform Pearson's r. Include the significance, flag significant results. Also include the scatterplot with densities and check statistics. Assess multivariate normality using the Shapiro Wilks test.
Use the results from the Q1 Correlation module to answer the following question:
Q1-1. What is the correlation value?
Step 2 – Simple Regression
Open a new analytical module under regression and name it Q1 Regression Your Name. Perform a linear regression analysis to determine if time watching television significantly increases LDL cholesterol concentrations. Load LDL_Chol as the dependent variable and Hours_TV as the predictor and include the intercept. Include the model fit, descriptives, coefficient estimates, Durbin-Watson results and casewise diagnostics to standardized residuals greater than 3. Use default values for specification. Plot the residuals vs predicted, the residuals histogram with standardized residuals, and the Q-Q plot of the residuals to establish normality.
Use the results from Q1 Regression to answer the following questions (round all answers to 3 decimal places):
Q1-2. What is the coefficient of determination for the model?
Q1-3. What would the LDL be for someone who never watches television?
Q1-4. What is F-value for the model?
Q1-5. Every hour per day spent watching television increases LDL cholesterol by how much?
Export the results of Question 1 to pdf, attach them and save them in Question 2.
A health researcher wants to be able to predict maximal aerobic capacity (VO2max), an indicator of fitness and health. Normally, to perform this procedure requires expensive laboratory equipment and necessitates that an individual exercise to their maximum (i.e., until they can longer continue exercising due to physical exhaustion). This can put off those individuals that are not very active/fit and those individuals that might be at higher risk of ill health (e.g., older unfit subjects). For these reasons, it has been desirable to find a way of predicting an individual's VO2max based on more easily and cheaply measured attributes. To this end, the researcher recruits 75 participants to perform a maximum VO2max test, but also records their age, weight, and heart rate. Heart rate is the average of the last 5 mins of a 20 mins much easier, lower workload cycling test. The researcher's goal is to be able to predict VO2max based on age, weight, and heart rate.
Step 1 – Pearson's Correlation.
Open a new (classical) correlation analysis in JASP and name the module VO2 Correlation Your Name. For this one, just report Pearson's r, significance, and include the heatmap.
Step 2 – Multiple Regression.
Open a new linear regression analysis and name it VO2 OLS Your Name. Create a linear regression equation which estimates the impact of weight and heart rate on VO2 Max while controlling for age and gender. Enter VO2 Max as the dependent, enter all scale variables as covariates and enter gender as a factor. Under model, enter age and gender under M0 to control for those effects. Under model summary, select R2 change, F2 change, and Durbin Watson. Under coefficients, select estimates and Tolerance/VIF. Set casewise diagnostics to standardized re
Collepals.com Plagiarism Free Papers
Are you looking for custom essay writing service or even dissertation writing services? Just request for our write my paper service, and we'll match you with the best essay writer in your subject! With an exceptional team of professional academic experts in a wide range of subjects, we can guarantee you an unrivaled quality of custom-written papers.
Get ZERO PLAGIARISM, HUMAN WRITTEN ESSAYS
Why Hire Collepals.com writers to do your paper?
Quality- We are experienced and have access to ample research materials.
We write plagiarism Free Content
Confidential- We never share or sell your personal information to third parties.
Support-Chat with us today! We are always waiting to answer all your questions.
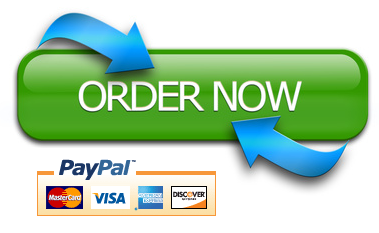