Read Chapter 8, review the algorithms for the different research designs. ? You are going to compare two groups of students (school team or no school team). ?This is like having a
· Read Chapter 8, review the algorithms for the different research designs.
· You are going to compare two groups of students (school team or no school team). This is like having an intervention group and a control group.
· Select (and cite) the correct quantitative algorithm from your text that will aid you in identifying the research design you should use.
· State the quantitative research design and the algorithm you used in this decision.
· Explain your choice.
· What type of sampling will you do to reach the highest level of research design (experimental?). Select a quasi-experimental or experimental sampling method.
· What method did you select and why?
Week 3 Assignment 3.5
In this discussion, you'll be critically thinking about a research study with different groups of students as the subjects. You'll use your thinking, textbook, and other resources to answer this discussion question. You'll learn about research designs and sampling techniques.
Put your thinking caps on!
Sample study: The research study you are interested in conducting will look at the two groups of junior high school students, those that play sports on an official school team and those that do not play sports on a school team.
You want to know if the average (mean) number of minutes of physical activity per week is different between the two groups, whether or not their nutritional calorie intake is different, and how many minutes of sleep they get each night.
Your research question is this: Are junior high school students that play sports on a school team receiving more calories a week, more minutes of sleep a week, and more minutes of physical activity per week than junior high school students who do not play sports on a school team?
Approved Resources to Use in Writing Discussion Posts:
· The course textbook,
Grove, S. K. & Gray, S. G. (2019). Understanding nursing research: Building an evidence-based practice (7th ed.). Elsevier.
· any published peer-reviewed full-text article from the CINAHL database
· org, or .gov website with published credible information.
· The use of AI is not permitted in this DQ.
· All sources must be published within the last 5 years.
This post must be a minimum of 250 words.
For your initial post, complete the following:
· Read Chapter 8, review the algorithms for the different research designs.
· You are going to compare two groups of students (school team or no school team). This is like having an intervention group and a control group.
· Select (and cite) the correct quantitative algorithm from your text that will aid you in identifying the research design you should use.
· State the quantitative research design and the algorithm you used in this decision.
· Explain your choice.
· What type of sampling will you do to reach the highest level of research design (experimental?). Select a quasi-experimental or experimental sampling method.
· What method did you select and why?
,
CHAPTER 8
Clarifying Quantitative Research Designs
Susan K. Grove
A research design is a blueprint for conducting a study. Over the years, several quantitative research designs have been developed for conducting descriptive, correlational, quasi-experimental, and experimental studies. Descriptive and correlational designs are focused on describing and examining relationships of variables in natural settings. Quasi-experimental and experimental designs have been developed to examine causality, or the cause and effect relationships between interventions and outcomes. The designs focused on causality were developed to maximize control over factors that could interfere with or threaten the validity of the study design. The strengths of the design validity increase the probability that the study findings are an accurate reflection of reality. Well-designed studies, especially those focused on testing the effects of nursing interventions, are essential for generating sound research evidence for practice (Melnyk, Gallagher-Ford, & Fineout-Overholt, 2017).
Being able to identify a study design and evaluate its strengths and weaknesses are an important part of critically appraising studies. Therefore, this chapter introduces you to the different types of quantitative study designs and provides an algorithm for determining whether a study design is descriptive, correlational, quasi-experimental, or experimental. Algorithms are also provided so that you can identify specific types of designs in published studies. The concepts relevant for understanding quantitative research designs are defined. The different types of validity—construct, internal, external, and statistical conclusion—are described. Guidelines are provided for critically appraising designs in quantitative studies. The chapter concludes with an introduction to randomized controlled trials (RCTs), with a flow diagram provided to examine the quality of these trials conducted in nursing.
Identifying quantitative research designs in nursing studies
A variety of quantitative research designs are implemented in nursing studies; the four most common types are descriptive, correlational, quasi-experimental, and experimental. These designs are categorized in different ways in textbooks (Kerlinger & Lee, 2000; Shadish, Cook, & Campbell, 2002). Sometimes, descriptive and correlational designs are referred to as noninterventional or nonexperimental designs because the focus is on examining variables as they naturally occur in environments and not on the implementation of an intervention by the researcher.
Some of the noninterventional designs include a time element, such as the cross-sectional design, which involves data collection on variables at one point in time. For example, cross-sectional designs might involve examining a group of study participants simultaneously in various stages of development, levels of education, severity of illness, or stages of recovery to describe changes in a phenomenon across stages. The assumption is that the stages are part of a process that will progress over time. Selecting participants at various points in the process provides important information about the totality of the process, even though the same subjects are not monitored throughout the entire process (Gray, Grove, & Sutherland, 2017). For example, researchers might describe the depression levels of three different groups of women with breast cancer who are prechemotherapy, receiving chemotherapy, or postchemotherapy treatment to understand depression levels based on the phase of treatment. Longitudinal design involves collecting data from the same study participants at multiple points in time and might also be referred to as repeated measures. Repeated measures might be included in descriptive, correlational, quasi-experimental, or experimental study designs. With a longitudinal design, a sample of women with breast cancer could be monitored for depression before, during, and after their chemotherapy treatment.
Quasi-experimental and experimental studies are designed to examine causality or the cause and effect relationship between a researcher-implemented intervention and selected study outcomes. The designs for these studies are sometimes referred to as interventional or experimental because the focus is on examining the differences in dependent variables thought to be caused by independent variables or interventions. For example, the researcher-implemented intervention might be a home monitoring program for patients initially diagnosed with hypertension, and the dependent or outcome variables could be systolic and diastolic blood pressure values measured at 1 week, 1 month, and 6 months. This chapter introduces you to selected interventional designs and provides examples of these designs from published nursing studies. Details on other study designs can be found in a variety of methodology sources (Campbell & Stanley, 1963; Creswell, 2014; Gray et al., 2017; Kerlinger & Lee, 2000; Shadish et al., 2002).
The algorithm shown in Fig. 8.1 may be used to determine the type of design (e.g., descriptive, correlational, quasi-experimental, experimental) used in a study. This algorithm includes a series of yes or no responses to specific questions about the design. The algorithm starts with the question, “Is there an intervention?” The answer leads to the next question, with the four types of designs being identified in the algorithm. For example, if researchers conducted a study to identify the characteristics of nurses who either passed or failed their registered nurse (RN) licensure on the first try, Fig. 8.1 indicates that a descriptive design would be used. If the researchers examined the relationships among the nurses’ characteristics and their score on the RN licensure examination, a correlational design would be implemented. If researchers tested the effectiveness of a relaxation intervention on graduates’ RN licensure examination scores, either a quasi-experimental or experimental design would be implemented. Experimental designs have the greatest control because (1) a tightly controlled intervention is implemented and (2) study participants are randomly assigned to either the intervention or control group (see Fig. 8.1).
FIG 8.1 Algorithm for determining the type of quantitative study design.
Understanding concepts relevant to quantitative research designs
Concepts relevant to quantitative research designs include causality, multicausality, probability, bias, prospective, retrospective, control, and manipulation. These concepts are described to provide a background for understanding noninterventional and interventional research designs.
Causality
Causality basically means that things have causes, and causes lead to effects. In a critical appraisal, you need to determine whether the purpose of the study is to examine causality, examine relationships among variables (correlational designs), or describe variables (descriptive designs). You may be able to determine whether the purpose of a study is to examine causality by reading the purpose statement and propositions within the framework (see Chapter 7). For example, the purpose of a causal study may be to examine the effect of an early ambulation program after surgery on the length of hospital stay. The framework proposition may state that early physical activity following surgery improves recovery time. However, the early ambulation program is not the only factor affecting the length of hospital stay. Other important factors or extraneous variables that affect the length of hospital stay include the diagnosis, type of surgery, patient’s age, physical condition of the patient before surgery, and complications that occurred after surgery. Researchers usually design quasi-experimental and experimental studies to examine causality or the effect of an intervention (independent variable) on a selected outcome (dependent variable), using a design that controls for relevant extraneous variables.
Multicausality
Very few phenomena in nursing can be clearly linked to a single cause and a single effect. A number of interrelating variables can be involved in producing a particular effect. Therefore studies developed from a multicausal perspective will include more variables than those using a strict causal orientation. The presence of multiple causes for an effect is referred to as multicausality. For example, patient diagnosis, age, presurgical condition, and complications after surgery are interrelated causes of the length of a patient’s hospital stay. Because of the complexity of causal relationships, a theory is unlikely to identify every element involved in causing a particular outcome. However, the greater the proportion of causal factors that can be identified and examined or controlled in a single study, the clearer the understanding will be of the overall phenomenon. This greater understanding is expected to increase the ability to predict and control the effects of study interventions.
Probability
Probability addresses relative rather than absolute causality. A cause may not produce a specific effect each time that a particular cause occurs, and researchers recognize that a particular cause probably will result in a specific effect. Using a probability orientation, researchers design studies to examine the probability that a given effect will occur under a defined set of circumstances. The circumstances may be variations in multiple variables. For example, while assessing the effect of multiple variables on length of hospital stay, researchers may choose to examine the probability of a given length of hospital stay under a variety of specific sets of circumstances. One specific set of circumstances may be that the patient had undergone a knee replacement, had no chronic illnesses, and experienced no complications after surgery. Sampling criteria could be developed to control most of these extraneous variables. The probability of a given length of hospital stay could be expected to vary as the set of circumstances are varied or controlled in the design of the study.
Bias
The term bias means a slant or deviation from the true or expected. Bias in a study distorts the findings from what the results would have been without the bias. Because studies are conducted to determine the real and the true, quantitative researchers place great value on identifying and removing sources of bias in their study and controlling their effects on the study findings. Any component of a study that deviates or causes a deviation from a true measurement of the study variables contributes to distorted findings. Many factors related to research can be biased; these include attitudes or motivations of the researcher (conscious or unconscious), components of the environment in which the study is conducted, selection of the study participants, composition of the sample, groups formed, measurement methods, data collection process, and statistical analyses (Gray et al., 2017; Grove & Cipher, 2017). For example, some of the participants for the study might be taken from a unit of the hospital in which the patients are participating in another study involving quality nursing care or a nurse, selecting patients for a study, might include only those who showed an interest in the study (Gray et al., 2017). Researchers might use a scale with limited reliability and validity to measure a study variable (Waltz, Strickland, & Lenz, 2017). Each of these situations introduces bias to nonintervention and intervention studies.
An important focus in critically appraising a study is to identify possible sources of bias. This requires careful examination of the methods section in the research report, including the strategies for obtaining study participants, methods of measurement, implementation of a study intervention, and data collection process. However, not all biases can be identified from the published study report. The article may not provide sufficient detail about the methods of the study to detect possible biases.
Prospective Versus Retrospective
Prospective is a term that means looking forward, whereas the term retrospective means looking backward, usually in relation to time. In research, these terms are used most frequently to refer to the timing of data collection. Are the data obtained in real time, with measurements being obtained by the research team, or are the study’s data obtained from information collected at a prior time? Data collection in noninterventional research can be either prospective or retrospective because, by definition, it lacks researcher intervention. Many noninterventional studies in health care use retrospective data obtained from national electronic databases and clinical and administrative databases of healthcare agencies. Secondary analysis of data from a previous study to address a newly developed study purpose is also considered retrospective. However, prospective data collection is usually more accurate than retrospective data collection, especially when researchers are passionate about their phenomenon of study and are rigorous in the measurement of study variables and the implementation of the data collection process.
Data collection in interventional research, however, must be prospective because the researcher enacts an intervention in real time. This is not to say that the research team does not access current data from the health record for real-time studies. A researcher collecting arterial blood pressure data in critically ill infants might collect data over a 24-hour period for several days. Nurses on the various shifts would record arterial blood pressure at least hourly, as is common practice, and the research team would retrieve that information during daily data collection. Although information retrieval of the infants’ electronic chart data does look back in time over the preceding 24-hour period, this study would be considered prospective because data are generated and recorded at the same time that the infants are hospitalized.
Control
One method of reducing bias is to increase the amount of control in the design of a study. Control means having the power to direct or manipulate factors to achieve a desired outcome. For example, in a study of an early ambulation program, study participants may be randomly selected and then randomly assigned to the intervention group or control group. The researcher would control the duration of and the assistance during the ambulation program or intervention. The time that the ambulation occurred in relation to surgery would also be controlled, as well as the environment in which the patient ambulated. Measurement of the length of hospital stay could be controlled by ensuring that the number of days, hours, and minutes of the hospital stay is calculated exactly the same way for each participant. Limiting the characteristics of the study participants, such as diagnosis, age, type of surgery, and incidence of complications, would also be a form of control. The greater the researcher’s control over the study situation, the more credible (or valid) the study findings.
Manipulation
Manipulation is a form of control generally used in quasi-experimental and experimental studies. Controlling an intervention is the most common manipulation in these studies. In descriptive and correlational studies, little or no effort is made to manipulate factors regarding the circumstances of the study. Instead, the purpose is to examine the phenomenon and its characteristics as they exist in a natural environment or setting. However, when quasi-experimental and experimental designs are implemented, researchers must manipulate the intervention under study. Researchers need to develop quality interventions that are implemented in consistent ways by trained individuals (Eymard & Altmiller, 2016). This controlled manipulation of a study’s intervention decreases the potential for bias and increases the validity of the study findings.
Examining the design validity of quantitative studies
Study validity is a measure of the truth or accuracy of the findings obtained from a study. The validity of a study’s design is central to obtaining accurate trustworthy results and findings from a study. Design validity encompasses the strengths and threats to the quality of a study design. Critical appraisal of studies requires that you identify the design strengths and think through the threats to validity or the possible weaknesses in a study’s design. Four types of design validity relevant to nursing research include construct validity, internal validity, external validity, and statistical conclusion validity (Gray et al., 2017; Kerlinger & Lee, 2000; Shadish et al., 2002). Table 8.1 describes these four types of design validity and summarizes the threats common to each. Understanding these types of validity and their possible threats are important in critically appraising quantitative study designs.
Table 8.1
Types of design validity critically appraised in studies
Types of design validity Description Threats to design validity
Construct validity Validity is concerned with the fit between the conceptual and operational definitions of variables and that the instrument measures what it is supposed to in the study.
Inadequate definitions of constructs: Constructs or concepts examined in a study lack adequate conceptual or operational definitions, so the measurement method is not accurately capturing what it is supposed to in a study.
Mono-operation bias: Only one measurement method is used to measure the study variable.
Experimenter expectancies (Rosenthal effect): Researchers’ expectations or bias might influence study outcomes, which could be controlled by researchers designating research assistants to collect study data. Another option is blinding researchers and data collectors to the group receiving the study intervention.
Internal validity Validity is focused on determining if study findings are accurate or are the result of extraneous variables.
Participant selection and assignment to group concerns: The participants are selected by nonrandom sampling methods and are not randomly assigned to groups.
Participant attrition: The percentage of participants withdrawing from the study is high or more than 25%, which can affect the findings of any quantitative study.
History: An event not related to the planned study occurs during the study and could have an impact on the findings.
Maturation: Changes in participants, such as growing wiser, more experienced, or tired, which might affect study results.
External validity Validity is concerned with the extent to which study findings can be generalized beyond the sample used in the study.
Interaction of selection and intervention: The participants included in the study might be different than those who decline participation. If the refusal to participate is high, this might alter the study results.
Interaction of setting and intervention: Bias exists in study settings and organizations that might influence implementation of a study intervention and data collection process. For example, some settings are more supportive and assist with a study, and others are less supportive and might encourage patients not to participate in a study.
Interaction of history and intervention: An event, such as closing a hospital unit, changing leadership, or high nursing staff attrition, might affect the implementation of the intervention and the measurement of study outcomes, which would decrease generalization of findings.
Statistical conclusion validity Validity is concerned with whether the conclusions about relationships or differences drawn from statistical analysis are an accurate reflection of the real world.
Low statistical power: This refers to concluding that there are no differences between samples when one exists (Type II error), which is usually caused by small sample size.
Unreliable measurement methods: Scales or physiological measures used in a study are not consistently measuring study variables. Reliability or consistency of scales is determined using the Cronbach alpha, which should be greater than 0.70 in a study (see Chapter 10).
Intervention fidelity concerns: The intervention in a study is not consistently implemented because of lack of study protocol or training of individuals implementing the intervention.
Extraneous variances in study setting: Extraneous variables in the study setting influence the scores on the dependent variables, making it difficult to detect group differences.
Construct Validity
Construct validity examines the fit between the conceptual and operational definitions of variables. Theoretical constructs or concepts are defined within the study framework when a framework is identified. When the researchers do not identify a specific study framework, the variables may be defined according to how they have been defined in other studies. These abstract statements about the variables are the conceptual definitions, which provide the basis for the operational definitions of the variables. Operational definitions (methods of measurement) must accurately reflect the theoretical constructs or concepts. Construct validity is the extent of the congruence or consistency between the conceptual definitions and operational definitions (see Chapter 5). The process of developing construct validity for an instrument often requires years of scientific work, and researchers need to discuss the construct validity of the instruments that they used in their study (see Chapter 10; Shadish et al., 2002; Waltz et al., 2017). The threats to construct validity are related to previous instrument development and to the development of measurement techniques as part of the methodology of a particular study. Threats to construct validity are described here and summarized in Table 8.1.
Inadequate Definitions of Constructs
Measurement of a construct stems logically from a concept analysis of the construct by the theorist who developed the construct or by the researcher. Ideally, the conceptual definition should emerge from the concept analysis, which is an in-depth study of the meanings of a construct or concept provided by theorists and researchers. The method of measurement (operational definition) should clearly reflect both the framework concept and study variable. A deficiency in the conceptual or operational definition leads to low construct validity.
Mono-Operation Bias
Mono-operation bias occurs when only one method of measurement is used to assess a construct. When only one method of measurement is used, fewer dimensions of the construct are measured. Construct validity greatly improves if the researcher uses more than one instrument (Waltz et al., 2017). For example, if pain were a dependent variable, more than one measure of pain could be used, such as a pain rating scale, verbal reports of pain, physical responses (e.g., increased pulse, blood pressure, respirations), and observations of behaviors that reflect pain (e.g., crying, grimacing, guarding of painful area, pulling away). It is sometimes possible to apply more than one measurement of the dependent variable with little increase in time, effort, or cost. Using multiple methods of measuring a construct increases the construct validity (see Chapter 10).
Experimenter Expectancies (Rosenthal Effect)
The expectancies of the researcher can bias the data. For example, experimenter expectancy occurs if a researcher expects a particular intervention to relieve pain. The data that he or she collects may be biased to reflect this expectation. If another researcher who does not believe that the intervention would be effective had collected the data, results could have been different. The extent to which this effect actually influences studies is not known. Because of their concern about experimenter expectancy, some researchers choose not to be involved in the data collection process. In other studies, data collectors do not know which study participants were assigned to the intervention and control groups, which means that they were blinded to group assignment. Using nonbiased data collectors or those who are blinded to group assignment increases the construct design validity of a study.
Internal Validity
Internal validity is the extent to which the effects detected in the study are a true reflection of reality, rather than the result of extraneous variables. Internal validity is a concern in all studies, but is a major focus in studies examining causality. When examining causality, the researcher must determine whether the dependent variables may have been influenced by a third, often unmeasured, variable (an extraneous variable). The possibility of an alternative explanation of cause is sometimes referred to as a rival hypothesis (Shadish et al., 2002). Any study can contain threats to internal design validity, and these validity threats can lead to false-positive or false-negative conclusions (see Table 8.1). The researcher must ask, “Is there another reasonable (valid) explanation (rival hypothesis) for the finding other than the one I have proposed?” Some of the common threats to internal validity, such as study participant selection and assignment to groups, participant attrition, history, and maturation, are discussed in this section.
Participant Selection and Assignment to Groups
Selection addresses the process whereby participants are chosen to take part in a study and how they are grouped within a study. A selection threat is more likely to occur in studies in which randomization is not possible (Gray et al., 2017; Shadish et al., 2002). In some studies, people selected for the study may differ in some important way from people not selected for the study. In other studies, the threat is a result of the differences in participants selected for study groups. For example, people assigned to the control group could be different in some important way from people assigned to the intervention group. This difference in selection could cause the two groups to react differently to the intervention; in this case, the groups’ outcomes would not be due to the intervention, but to the differences in the individuals selected for the two groups. Random selection of participants for nursing studies is often not possible, and the number of participants available for studies is limited. The random assignment of participants to groups decreases the possibility of their selection being a threat to internal validity.
Participant Attrition
Attrition involves participants dropping out of a study before it is completed. Participant attrition becomes a threat (1) when those who drop out of a study are a different type of person from those who remain in the study or (2) there is a difference in the number and types of people who drop out of the intervention group and the people who drop out of the control or comparison group (see Chapter 9). If the attrition in a study is high (> 25%), this could affect the accuracy of the study results (Cohen, 1988; Gray et al., 2017).
History
History is an event that is not related to the planned study but that occurs during the time of the study. History could influence a participant’s response to the intervention or to the variables being measured and alter the outcome of the study. For example, if researchers studied the effect of an emotional support intervention on a participant’s completion of his or her cardiac rehabilitation program, and several nurses quit their job at the rehabilitation center during the
Collepals.com Plagiarism Free Papers
Are you looking for custom essay writing service or even dissertation writing services? Just request for our write my paper service, and we'll match you with the best essay writer in your subject! With an exceptional team of professional academic experts in a wide range of subjects, we can guarantee you an unrivaled quality of custom-written papers.
Get ZERO PLAGIARISM, HUMAN WRITTEN ESSAYS
Why Hire Collepals.com writers to do your paper?
Quality- We are experienced and have access to ample research materials.
We write plagiarism Free Content
Confidential- We never share or sell your personal information to third parties.
Support-Chat with us today! We are always waiting to answer all your questions.
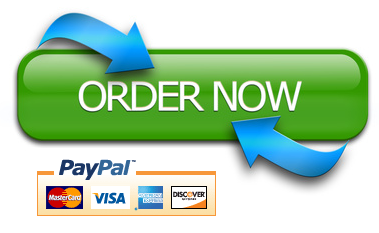