The Influence of the External and Internal Environments on the Process •
The Influence of the External and Internal Environments on the Process • Data and Its Analysis in Decision Making • Technologies for Data Analysis and Decision Support 6 The Decision Making Process • The business world is full of uncertainties and rapid changes. • Thus, the decision making process can determine the success or failure of an organization, and how well it performs. • In the past decision making was based on creativity, instinct, and experience. • Now, decision making is more grounded in scientific approach, and utilizes systematic quantitative methods. 7 The Decision Making Process (cont.) Managers usually undergo the decision making process using the following steps: 1. Understand the decision you have to make 2. Collect all the information (Define the problem) 3. Identify the alternatives (Construct a model & Identify possible solutions) 4. Evaluate the pros and cons 5. Select the best alternative (Compare, choose and recommend the best solution) 6. Make the decision 7. Evaluate the impact of your decision 8 The Influence of External & Internal Environments • Predicting the consequences and the future of any given decisions is complex. This is due to the uncertainty that can arise from multiple factors including: 1. 2. 3. 4. • Political Factors (E.g. government policies, political instability) Economic Factors (E.g. competition, changing demand) Sociological and psychological factors Environment Factors Because of these constant changes, the trial-and-error approach to management is unreliable and unsustainable. Managers must begin to use the new tools and techniques of their fields. 9 Data and Its Analysis in Decision Making 10 • The amount of data doubles every two years. • Decision making process requires an organization to collect and analyze vast stores of data. • Computer applications have moved from transaction-processing and monitoring activities => to problem analysis and solution applications. • These activities are done with cloud-based technologies, mostly accessed through mobile devices. Data and Its Analysis in Decision Making (cont.) • The foundation of modern management lies in 1. analytics and BI tools such as data warehousing, data mining, online analytical processing (OLAP), dashboards, and 2. the use of cloud-based systems for decision support. 11 Technologies for Data Analysis and Decision Support • The following developments have contributed to the growth of decision support and analytics technologies: 1. 2. 3. 4. 5. 6. 7. 8. Group communication and collaboration Improved data management Managing giant data warehouses & Big Data Analytical Support Overcoming cognitive limits in processing and storing information Knowledge Management Anywhere, anytime support Innovation and artificial intelligence 12 1.3 Decision-Making Processes and Computer Decision Support Framework • Simon’s Process: Intelligence, Design, Choice, and Implementation Phases • The Classical Decision Support System Framework • DSS Application • Characteristics of DSS • Components of a Decision Support System 13 Simon’s Process: Intelligence, Design, and Choice • Decision-making process phases: 1. Intelligence: The decision maker examines reality, identifies and defines the problem. 2. Design: A model representing the system is constructed by and then validated. 1. making assumptions to simplify reality and 2. identifying relationships between variables. 3. Choice: Selection of a proposed solution to the model . This solution is tested to determine its viability. 4. Implementation: Successful implementation results in solving the real problem. Failure leads to a return to an earlier phase of the process. 14 15 The Classical Decision Support System Framework • The framework for computerized decision support can be represented in a 3×3 matrix with two dimensions: 1. Type of decisions: o Structured: Routine/repetitive problems for which standard solution methods exist o Semi structured: Fall between structured and unstructured problems o Unstructured: Complex problems where there are no clear, standard solution methods 2. Types of controls: o Strategic planning: Defining long-range goals/policies for resource allocation o Management control: The efficient use of resources in the accomplishment of organizational goals o Operational control: the efficient and effective execution of specific tasks 16 17 DSS Application Decision support • Key difference between DSS and BI applications: 1. Business intelligence (BI) systems monitor situations and identify problems and/or opportunities using analytic methods. 2. DSS is a methodology for supporting decision making:o It uses an interactive, adaptable computer-based information system (CBIS) developed for supporting the solution to a specific unstructured management problem. o It uses data, provides a user-friendly interface, and incorporates the decision maker’s insights. o It includes models that developed through an interactive and iterative process. 18 Characteristics of DSS 19 Components of a Decision Support System • A DSS can be composed of: 1. Data management subsystem 2. Model management subsystem 3. User interface subsystem 4. Knowledge-based management subsystem 20 1. Data Management Subsystem • The data management subsystem is composed of the following elements: 1. DSS database 2. Database management system 3. Data directory 4. Query facility • It can be interconnected with the corporate data warehouse (a repository for corporate relevant decision-making data) 21 2. Model Management Subsystem • The model management subsystem is a component that includes quantitative models that provide the o system’s analytical capabilities and o appropriate software management. • The model management subsystem of a DSS is composed of the following elements: 1. 2. 3. 4. 5. 22 Model base MBMS (Model Base Management System) Modeling language Model directory Model execution, integration, and command processor 3. The User Interface Subsystem • The user is considered part of the system. • The user communicates with and commands the DSS through the user interface subsystem. • The Web browser has been recognized as an effective DSS GUI because it is o flexible, o user-friendly, and a o gateway to almost all sources of necessary information and data. • Web browsers have led to the development of portals and dashboards (front end of many DSS). 23 4. The Knowledge-Based Management Subsystem • The knowledge-based management subsystem provides intelligence o to augment the decision maker’s own intelligence o to help understand a user’s query and provide a consistent answer. • Interconnected with the organization’s knowledge repository or connected to thousands of external knowledge sources. • User interface developments are closely tied to the major new advances in their knowledge-based systems. 24 1.4 Evolution of Computerized Decision Support to Business Intelligence/Analytics/Data Science • Evolution Of Computerized Decision Support • Framework for Business Intelligence • Architecture of BI • The Origins and Drivers of BI • Data Warehouse as a Foundation for Business Intelligence • Transaction Processing versus Analytic Processing • A Multimedia Exercise in Business Intelligence 25 Evolution Of Computerized Decision Support 26 Evolution Of Computerized Decision Support (cont.) 1. Management Information Systems (MIS): a variety of reports used to understand and address the changing business needs and challenges. 2. Decision Support Systems (DSS): combination of individuals intellectual resources with the capabilities of the computer to improve the quality of decisions. o DSS designed and developed specifically for executives and their decisionmaking needs 3. Executive Information Systems (EIS): the need for more versatile reporting leads to the development of EISs; o These systems were designed as graphical dashboards that allow decision makers to keep track of the key performance indicators. 27 A Framework for Business Intelligence (BI) • BI is an umbrella term that combines architectures, tools, databases, analytical tools, applications, and methodologies. • BI process is based on the transformation of data -> information -> decisions -> actions • BI’s major objective is to enable 1. interactive access to data (in real time), 2. data manipulation, 3. appropriate analyses. • By analyzing historical and current data, situations, and performances, decision makers able to make more informed and better decisions. 28 Architecture of BI • BI has four major components: 1. Data Warehouse (DW) (with its source data) 2. Business Analytics (a collection of tools for manipulating, mining, and analyzing the data in the DW) 3. BPM (for monitoring & analyzing performance) 4. User Interface 29 The Origins and Drivers of BI • Business cycle times are extremely compressed; faster, and more informed. • Managers need the right information at the right time and in the right place (core of the modern approaches to BI). • Organizations are being driven to capture, understand, and harness their data to support decision making and improve business operations. • Legislation/regulations require leaders to document their business processes and sign off on the validity of the information they report to stakeholders. 30 Data Warehouse (DW) as a Foundation for BI • BI systems rely on DWs as the information source for creating insight and supporting managerial decisions. • DW is a subject-oriented, integrated, time-variant, nonvolatile collection of data in support of management’s decision-making process. • The three main types of data warehouses are: o Data Marts (DM): a subset of a DW, typically consisting of a single subject area o Operational Data Stores (ODS): provides a form of customer information file whose contents are updated throughout the course of business operations. o Enterprise Data Warehouses (EDW): a large-scale data warehouse used across the enterprise for decision support. 31 Data Warehouse (DW) as a Foundation for BI (cont.) • Data from many different sources can be extracted, transformed, and loaded into a DW for further access and analytics for decision support. • Data is structured to be available in a form ready for analytical processing activities: o OLAP, o data mining, o querying, o reporting, o decision support applications 32 Transaction Processing versus Analytic Processing • Transaction processing systems are constantly involved in handling updates to what may be known as operational databases. • OLTP (OnLine Transaction Processing) systems handle a company’s routine ongoing business, where the computer responds immediately to user requests. • The OLTP system is efficient for transaction processing, but inefficient for enduser ad hoc reports, queries, and analysis. • In contrast, a DW is typically a distinct system that provides storage for data that will be used for analysis by OLAP (OnLine Analytical Processing) systems. 33 Multimedia Exercise in Business Intelligence • The fundamental reasons for investing in BI must be aligned with the company’s business strategy. • BI improve company business processes and transforming it to be more data driven. • BI tools are sometimes integrated among themselves, resulting in six key trends: 1. 2. 3. 4. 5. 6. 34 Big Data Focus on customer experience as opposed to just operational efficiency. Mobile and even newer user interfaces—visual, voice, mobile. Predictive and prescriptive analytics, machine learning, artificial intelligence. Migration to cloud. Much greater focus on security and privacy protection. 1.5 Analytics Overview • Overview • Descriptive, Predictive, Prescriptive Analytics • Big Data 35 Analytics Overview • Analytics is the process of developing actionable/recommendations for actions based on insights generated from historical data. • To solve real problems analytics represents the combination of o computer technology + o management science techniques + o statistics. 36 Descriptive, Predictive, Prescriptive Analytics • Three types of analytics: 1. Descriptive 2. Predictive 3. Prescriptive 37 Big Data • Big Data refers to data that cannot be stored in a single storage unit. • Different forms: o structured o unstructured • Characteristics of big data: o volume o velocity o variety • These are evolving quickly to encompass stream analytics, IoT, cloud computing, and deep learning– enabled AI. 38 Big Data (cont.) • There are two aspects to managing data on this scale: 1. Storing: o an extremely expensive storage solution vs. store data in chunks on different machines connected by a network. o Hadoop Distributed File System (HDFS): store a copy or two of this chunk in different locations on the network, both logically and physically. 2. Processing: o To process vast amounts of data computation done by one powerful computer vs. processing data sets with a parallel distributed algorithm on a cluster o MapReduce programming paradigm 39 1.7 Artificial Intelligence Overview • What Is Artificial Intelligence? • The Major Benefits of AI • The Landscape of AI • The Three Flavors of AI Decisions • Technology Insights 1.1 40 What Is Artificial Intelligence (AI)? • The major goal of AI is to create intelligent machines that can do tasks currently done by people. • AI tasks include o reasoning, o thinking, o learning, and o problem solving. • AI can also be defined as: o Technology that can learn to do things better over time. o Technology that can understand human language. 41 o Technology that can answer questions. The Major Benefits of AI • Significant reduction in the cost of performing work. This reduction continues over time while the cost of doing the same work manually increases with time. • Work can be performed much faster. • Work is consistent in general, more consistent than human work. • Increased productivity and profitability as well as a competitive advantage are the major drivers of AI. 42 The Landscape of AI • We defined the landscape/ecosystem of AI into 5 categories: 1. Major Technologies: machine learning, deep learning, intelligent agents 2. Knowledge-Based Technologies: expert systems, recommendation engines, chatbots. 3. Biometric-Related Technologies: natural language processing, image recognition 4. Support Theories, Tools, & Platforms: computer science, cognitive science, mathematics, statistics, sensors, augmented reality, neural networks, APIs, knowledge management. 5. AI Applications: smart cities, smart homes, automatic decisions, translation, robotics, fraud detection. 43 The Landscape of AI (cont.) • AI Applications are in area like o business, o medicine & healthcare o transportation o education • AI field divided into two major categories of applications: 1. Narrow (Weak): Focuses on one narrow field (Domain). Examples include SIRI and Alexa that operate in limited, predefined areas. 2. General (Strong): To exhibit real intelligence, machines need to perform the full range of human cognitive capabilities (e.g. reasoning and problem solving). 44 The Three Levels of AI Systems • The capabilities of AI systems can be divided into three levels: 1. Assisted Intelligence: equivalent mostly to the weak AI, which works only in narrow domains. It requires clearly defined inputs and outputs such as monitoring systems. 2. Autonomous AI: in the realm of the strong AI but in a narrow domain. Eventually, the computer will take over as very narrow expert and have absolute decision-making power. 3. Augmented Intelligence: between assisted and autonomous. The technology focuses on augmenting computer abilities to extend human cognitive abilities, resulting in high performance. Examples: Cybercrime fighting, E-Commerce decisions, High-frequency stock market trading. 45 Technology Insights • Differences between traditional and augmented AI: 1. Augmented machines extend rather than replace human decision making 2. Augmentation excels in solving complex human and industry problems in specific domains in contrast with strong, general AI. 3. In contrast with a “black box” model of some AI and analytics, augmented intelligence provides insights and recommendations, including explanations. 46 1.7 Convergence of Analytics and AI • Major Differences between Analytics and AI • Why Combine Intelligent Systems? • How Convergence Can Help? • Big Data Is Empowering AI Technologies • The Convergence of AI and the IoT • The Convergence with Blockchain and Other Technologies 47 Major Differences between Analytics and AI • Analytics processes historical data using statistical, management science and other computational tools to describe situations, predict results, and propose recommendations for solutions to problems. • AI’s major objective is to mimic the manner in which people think, learn, reason, make decisions, and solve problems. • The emphasis in AI is on knowledge and intelligence as major tools for solving problems rather than relying on computation, which we do in analysis. 48 Why Combine Intelligent Systems? • Analytics, AI and their different technologies have limitations, resulting in only a small chance that they can be used to reach organizational excellence. • There are several reasons for this situation including: 1. Predictive models have unintended effects 2. The results of analytics may be good for some applications but not for others 3. Models are as good as their input data and assumptions 4. Data could be incomplete/inaccurate. 5. Data collected from different sources can vary in format and quality. • A major reason for the high failure rate of AI is that some of its technologies need a large amount of data (Big Data). Without this continuous flow of data, there would not be good learning in AI. 49 How Convergence Can Help? • BI and its analytics answer most of the why and what questions regarding the sufficiency of problem solving. • The next generation of business intelligence platforms will use AI to automatically locate, visualize, and narrate important things. This can also be used to create automatic alerts and notifications. • Machine learning and deep learning can support analytics by conducting pattern recognition and more accurate predictions. AI will help to compare actual performance with the predicted one. 50 Big Data Is Empowering AI Technologies • Technologies and methods that enable capturing, cleaning, and analyzing Big Data, can also enable companies to make real-time decisions. • The availability of new Big Data analytics enables new capabilities in AI technologies that were not possible until recently. • Big Data can empower AI due to: o The new capabilities of processing Big Data at a much reduced cost. o The availability of large data sets online. o The scale up of algorithms, including deep learning, is enabling powerful AI capabilities. 51 The Convergence of AI and the IoT • The IoT collects a large amount of data from sensors and other “things.” These data need to be processed for decision support. • Combining AI and IoT can o lead to the “next-level solutions and experiences.” The emphasis in such combination is on learning more about customers and their needs. o facilitate competitive analysis and business operation. • Three examples of combining AI and IoT: o The smart thermostat of Nest Labs o Automated vacuum cleaners o Self-driving vehicles 52 The Convergence with Blockchain & Other Technologies • Several experts raise the possibility of the convergence of AI, analytics, and blockchain. This convergence also can include the IoT. • The blockchain technology can add security to data shared by all parties in a distributed network, where transaction data can be recorded. • This combination can be very useful in complex applications such as autonomous vehicles. 53 1.8 Overview of the Analytics Ecosystem • Analytics Ecosystem 54 Analytics Ecosystem • Purpose of analytics ecosystem is to be aware of organizations, new offerings, and opportunities in sectors allied with analytics. • The components of the ecosystem are represented by the petals of an analytics flower. 55 • Grouped into categories: o inner petals o outer petals o seed Main Reference Chapter 1: “An overview of Decision Support system, Business Intelligence, Analysis, and AI” from “Analytics, Data Science, & Artificial Intelligence: Systems for Decision Support”. Week self-review exercises Application Case 1.1 to Application Case 1.10 from “Analytics, Data Science, & Artificial Intelligence: Systems for Decision Support” 56 Decision Support Systems College of Computing and Informatics 1 Contents o 2.2 – Introduction to Artificial Intelligence o 2.3 – Human and Computer Intelligence o 2.4 – Major AI Technologies and Some Derivatives o 2.5 – AI Support for Decision Making o 2.6 – AI Applications in Accounting o 2.7 – AI in Human Resource Management (HRM) o 2.8 – AI in Marketing, Advertising, and CRM o 2.9 – AI Applications in Financial Services o 2.10 – AI Applications in Production-Operation Management (POM) 3 Weekly Learning Outcomes 1. Understand the concepts of artificial intelligence (AI) 2. Become familiar with the drivers, capabilities, and benefits of AI 3. Describe human and machine intelligence 4. Describe the major AI technologies and some derivatives 5. Discuss the manner in which AI supports decision making 6. Describe AI applications in accounting, human resource management, marketing, financial Services and in Production-Operation Management (POM) 4 Required Reading Chapter 2: “Analytics, Data Science, & Artificial Intelligence: Systems for Decision Support” from “Analytics, Data Science, & Artificial Intelligence: Systems for Decision Support”. Recommended Reading AI-powered decision support systems, what are they? https://blog.pwc.lu/ai-powered-decision-support-systems-what-are-they/ Recommended Videos Artificial intelligence and decision-making (by Thorbjørn Knudsen) https://www.youtube.com/watch?v=Sujww4njwE4 5 2.2 Introduction To Artificial Intelligence • Definition of AI • Major Characteristics of AI Machines • Major Elements of AI • AI Applications • Major Goals of AI • Drivers of AI • Benefits of AI • Some Limitations of AI Machines • Three Flavors of AI Decisions • Artificial Brain 6 Definition of AI • Artificial intelligence has several definitions that is concerned with two basic ideas: o The study of human thought processes (to understand what intelligence is) o The representation and duplication of those thought processes in machines (e.g., computers, robots) • Another definition of AI is “the capabilities of a machine to imitate intelligent human behavior” 7 Major Characteristics of AI Machines • There is an increasing trend to make computers “smarter”. o Web 3.0 enables computerized systems that exhibit more intelligence than Web 2.0. • Several applications are already based on multiple AI techniques. o Machine translation of languages is helping people who speak different languages to collaborate in real time as well as to buy online products that are advertised in different languages. 8 Major Elements of AI • AI components can be divided into two groups: Foundations, and Technologies & Applications. 9 AI Applications Smart or intelligent applications include: • Machines to answer customers’ questions asked in natural languages • Knowledge-based systems which can provide advice, assist people to make decisions, and even make decisions on their own • Automatic generating of online purchasing orders and arranging fulfillment of orders placed online. • Shipping prices are determined automatically based on the dimensions, weight, and packaging. 10 Major Goals of AI • The overall goal of AI is to create intelligent machines that are capable of executing a variety of tasks currently done by people. • AI machines should be able to reason, think abstractly, plan, solve problems, and learn. • Some specific goals are to: o Perceive and properly react to changes in the environment that influence specific business processes and operations o Introduce creativity in business processes and decision making 11 Drivers of AI • The use of AI has been driven by the following: o People’s interest in smart machines and artificial brains o The low cost of AI applications versus the high cost of manual labor (doing the same work) o The desire of large tech companies to capture competitive advantage and market share of the AI market and their willingness to invest billions of dollars in AI o The pressure on management to increase productivity and speed o The availability of quality data contributing to the progress of AI o The increasing functionalities and reduced cost of computers in general o The development of new technologies, particularly cloud computing 12 Benefits of AI o AI has the ability to complete certain tasks faster than humans. o The consistency of AI work. AI machines do not stop, or sleep. o AI systems allow for continuous improvement projects. o AI can be used for predictive analysis via its capability of pattern recognition. o AI can manage delays and blockages in business processes. o AI machines can work autonomously or be assistants to humans. o AI machines can learn, improve its performance, and work in hazardous environments. o AI machines can facilitate innovations by human (i.e., support research and development) o AI excels in fraud detection and in security facilitations. o AI can free employees to work on more complex and productive jobs. o AI can solve difficult problems that previously were unsolved. 13 Some Limitations of AI Machines • The following are the major limitations of AI machines: o Lack human touch and feel o Lack attention to non-task surroundings o Can lead people to rely on AI machines (e.g., people may stop to think on their own) o Can be programmed to create destruction o Can cause many people to lose their jobs o Can start to think by themselves, diminishing with time. However, risks exist. Therefore, it is necessary to properly causing significant damage • Some of the limitations are diminishing with time. However, risks exist. Therefore, it is necessary to improve AI development and minimize the risks. 14 Artificial Brain • The artificial brain is a machine that is desired to be as intelligent, creative, and self-aware as humans. To date, no one has created such a machine. • The following are some differences between traditional and augmented AI: o Augmented machines extend rather than replace human decision making o Augmentation excels in solving complex human and industry problems in specific domains in contrast with strong, general AI. o In contrast with a “black box” model of some AI and analytics, augmented intelligence provides insights and recommendations, including explanations. 15 2.3 Human and Computer Intelligence • A. What Is Intelligence? • B. How Intelligent Is AI? • C. Measuring AI 16 What Is Intelligence? • Intelligence is a broad term measured by an IQ test. • To understand what artificial intelligence is, it is useful to first examine those abilities that are considered signs of human intelligence: o Learning or understanding from experience o Making sense out of ambiguous, incomplete, or even contradictory messages and information o Responding quickly and successfully to a new situation o Understanding/inferring in a rational way, and solving problems o Applying knowledge to manipulate environments and situations o Recognizing & judging the relative importance of elements in a situation 17 How Intelligent Is AI? • AI machines have demonstrated superiority over humans in playing complex games such as chess, Jeopardy!, and Go by defeating the world’s best players. • Despite this many AI applications still show significantly less intelligence than humans. 18 Measuring AI • The Turing Test is a well-known attempt to measure the intelligence level of AI machines. • It aims to determine whether a computer exhibits intelligent behavior. A computer can be considered smart only when a human interviewer asking the same questions to both an unseen human and an unseen computer cannot determine which is which. • To pass the Turing Test, a computer needs to be able to understand a human language (NLP), to possess human intelligence (e.g., have a knowledge base), to reason using its stored knowledge, and to be able to learn from its experiences (machine learning). 19 2.4 Major AI Technologies And Some Derivatives • Intelligent Agents • Machine Learning • Deep Learning • Machine and Computer Vision • Robotic Systems • Natural Language Processing • Knowledge and Expert Systems and Recommenders • Chatbots 20 • Emerging AI Technologies Intelligent Agents • An intelligent agent (IA) is a small computer software program that observes and acts upon changes in its environment by running specific tasks autonomously. • An IA directs an agent’s activities to achieve specific goals related to the changes in the surrounding environment. • IAs have the ability to learn by using their knowledge Example 1: An example of an intelligent software agent is a virus detection program. It resides in a computer, scans incoming data, and removes viruses automatically while learning to detect new virus types and detection methods. 21 Example 2: Allstate Business Insurance uses an intelligent agent to reduce call center traffic and provide human insurance agents during the rate-quoting process with business customers Machine Learning • Machine Learning (ML) is a discipline concerned with design & development of algorithms that allow computers to learn based on incoming data. • ML allows computer systems to monitor and sense their environment, so that the machines can adjust their behavior to deal with the changes • ML scientists teach computers to identify patterns and make connections by showing the machines a large volume of examples and related data. • ML used for predicting, recognizing patterns, & supporting decision makers. An example is computers detecting credit card fraud. • ML applications are expanding due to the availability of Big Data sources, especially those provided by the IoT. 22 Deep Learning • One subset of machine learning is called deep learning; a technology tries to mimic how the human brain works. • Deep learning (DL) uses artificial neural technology and deals with complex applications that regular machine learning and AI technologies can not handle. • For example, DL is a key technology in autonomous vehicles by helping to interpret road signs and road obstacles. • DL is mostly useful in real-time interactive applications in the areas of machine vision, scene recognition, robotics, and speech and voice processing. 23 Machine and Computer Vision • Machine vision includes “technology and methods used to provide imagingbased automated inspection and analysis for applications such as robot guidance, process control, autonomous vehicles, and inspection.” • Computer vision “is an interdisciplinary field that deals with how computers can be made for gaining high-level understanding from digital images or videos. From the perspective of engineering, it seeks to automate tasks that the human visual system can do.” 24 Machine and Computer Vision • The two technologies are combined with image processing that facilitates complex applications, such as visual quality control. • Applied area of machine vision is scene recognition, done by computer vision. • Video analytics is a derivative application of computer vision, where techniques are applied to videos to enable pattern recognition and identify events. • Example of Applications: o The machine vision wood identification project developed a prototype machine vision system for wood identification to help identify illegal logging. o AI computer vision mixed with deep learning identifies illegal animal poachers. o Facial recognition that employ smart glasses to identify potential suspects. 25 Robotic Systems • A robot is a device guided by a program to perform manual/mental tasks. • An “intelligent” robot has a sensory apparatus (e.g., camera) to collects information about the surroundings and can respond to the changes in the environment. • Autonomous robots (programmed to do tasks completely on their own, even repair themselves), are equipped with AI intelligent agents. • Example: Walmart Is Using Robots o In Walmart, 2-foot-tall robots use cameras/sensors to scan the shelves for misplaced, missing, or mispriced items. The results are transmitted to humans for corrective actions. The robots carry out their tasks faster and frequently more accurately than humans. 26 Natural Language Processing • Natural language processing (NLP) allows users to communicate with a computer in their native language. NLP includes two subfields: o NLP that investigates methods of enabling computers to comprehend instructions or queries provided in English or other human languages. o NLP generation that strives to have computers produce ordinary spoken language so that people can understand the computers more easily. • Speech/Voice Understanding: recognition & comprehension of spoken languages by a computer. This has been adopted in automated call centers. 27 • Machine Translation of Languages: uses computer programs to translate words and sentences from one language to another. Knowledge and Expert Systems and Recommenders • These systems are computer programs that store knowledge, which their applications use to generate expert advice and/or perform problem solving. • Knowledge-based expert systems help people to verify information and make certain types of automated routine decisions. • Recommendation systems are knowledge-based systems that make recommendations to people. Another knowledge system is chatbots. • Knowledge Sources And Acquisition For Intelligent: Intelligent systems must gain knowledge through knowledge acquisition. 28 • Knowledge acquisition includes extracting and structuring knowledge from data, and then experts may be used to verify it. Chatbots • Robots come in several shapes and types, of which is a chatbot. A chatbot is a conversional robot that is used for chatting with people using NLP technology. • Depending on the purpose of the chat, which can be done in writing or by voice, bots can be in the form of intelligent agents that retrieve information or personal assistants that provide advice. 29 Emerging AI Technologies • Several new AI technologies are emerging. Here are a few examples: o Effective computing. Technologies that detect emotional conditions of people and suggest how to deal with discovered problems o Biometric analysis. Technologies that verify an identity based on unique biological traits that are compared to stored ones (e.g., facial recognition). • Cognitive Computing: The application of knowledge derived from cognitive science so that computers can exhibit and/or support decision-making and problem-solving capabilities. • Augmented Reality: Augmented reality (AR) refers to the real time integration of digital information with a users environment (mostly vision and sound). The technology provides a real-world interactive experience with the environment. 30 2.5 AI Support For Decision Making • Issues and Factors in Using AI in Decision Making • AI Support of the Decision-Making Process • Automated Decision Making 31 Issues and Factors in Using AI in Decision Making • These factors determine the justification of AI usage and its chance of success: o The nature of the decision (E.g., routine decisions = likely automated) o The method of support, what technologies are used • Cost-benefit & Risk Analyses: necessary for large-scale decisions, but hard to compute with AI models due to difficulties in measuring costs, risks, & benefits. • Using Business Rule: AI systems can be based on business rules, whose quality determines that of the automated. • AI Algorithms: are the basis for automated decisions & decision support. • Speed: Decision automation is dependent on the speed decisions needs to be made. Some decisions takes too much time to get all the relevant input data. 32 AI Support of the Decision-Making Process AI support can be applied to the various steps of the decision-making process: 33 1. Problem Identification: collecting data through technology that can be used by AI algorithms. Performance levels of machines are compared to standards, and trend analysis can point to opportunities. 2. Generating/Finding Alternative Solutions: matching problem characteristics with best practice, or proven solutions stored in databases. Tools such as case-based reasoning and neural computing are for this purpose. 3. Selecting a Solution: evaluate proposed solutions, predict future impacts, asses chance of success, or predict a reply to actions taken by a competitor. 4. Implementing the Solutions: demonstrates the superiority of proposals and to assess resistance to changes. Automated Decision Making • The process of automated decision making starts with knowledge acquisition and creation of a knowledge repository. • The system generates and submits responses to user’s questions. Solutions are evaluated to improve the knowledge repository, and complex situations are forwarded to humans. • Companies use automated decision making for both their external operations (e.g., sales) and internal operations (e.g., resource allocation) Example: Supporting Nurses’ Diagnosis Decisions • Researchers used AI tools to conduct data mining to predict the probable success of automated nursing diagnoses based on patient characteristics. 34 Main Reference Chapter 2: “Analytics, Data Science, & Artificial Intelligence: Systems for Decision Support” from “Analytics, Data Science, & Artificial Intelligence: Systems for Decision Support”. Week self-review exercises Application Case 2.1 – 2.7 from “Analytics, Data Science, & Artificial Intelligence: Systems for Decision Support” 35 Decision Support Systems College of Computing and Informatics 1 Contents o 3.7 – Business Reporting o 3.8 – Data Visualization o 3.9 – Different Types of Charts and Graphs o 3.10 – Emergence of Visual Analytics o 3.11 – Information Dashboards o Analyzing data with pivottables and pivotcharts using excel 3 3.7 Business Reporting • Overview 7 Overview • Decision makers need information to make accurate and timely decisions. • Information is usually provided to decision makers in the form of a written report that contains organized information referring to specific time periods. • Business reports can fulfill many different functions: o To ensure that all departments are functioning properly o To provide information o To provide the results of an analysis o To persuade others to act o To create an organizational memory 8 Overview (cont.) • Business reporting (also called OLAP or BI) is an essential part of the larger drive toward improved, evidence-based, optimal managerial decision making. • The foundation of these business reports is various sources of data coming from both inside and outside the organization (OLTP systems). • Creation of these reports involves extract, transform, and load (ETL) procedures in coordination with a data warehouse and reporting tools. • This reporting process involves querying structured data sources, which were created using different logical data models and data dictionaries, to produce a human-readable, easily digestible report. 9 Overview (cont.) • These types of business reports allow managers to stay informed and involved, review options and alternatives, and make informed decisions. • Metric Management Reports: In many organizations, business performance is managed through outcome-oriented metrics. For external groups, these are service-level agreements. For internal management, they are key performance indicators (KPIs). • Dashboard-type Reports: A popular idea in business reporting has been to present a range of different performance indicators on 1 page like a dashboard. 10 • Balanced Scorecard–type Reports: This method attempts to present an integrated view of success in an organization. In addition to financial performance, balanced scorecard–type reports also include customer, business process, and learning and growth perspectives. 3.8 Data Visualization • • 11 Overview History of Data Visualization Overview • Data visualization has been defined as “the use of visual representations to explore, make sense of, and communicate data”. • Information is the aggregation, summarization, and contextualization of data, what is portrayed in visualizations is the information, not the data. • Data visualization is closely related to the fields of information graphics, information visualization, scientific visualization, and statistical graphics. • Until recently, the major forms of data visualization available in both BI applications have included charts and graphs as well as the other types of visual elements used to create scorecards and dashboards. 12 History of Data Visualization • Although visualization has not been widely recognized as a discipline until fairly recently, today’s most popular visual forms date back a few centuries. • Companies and individuals are interested in data; that interest has in turn sparked a need for visual tools that help them understand it. • Countless applications, tools, and code libraries help people collect, organize, visualize, understand data. • The future of data visualization holds more 3D imaging, experience with multidimensional data, and holographs of information. 13 3.9 Different Types of Charts and Graphs • • • 14 Basic Charts & Graphs Specialized Charts & Graphs Which Chart/Graph should you use? Basic Charts & Graphs • Line Chart: Line charts show the relationship between two variables; they are most often used to track changes or trends over time. • Bar Chart: Effective when you have nominal/numerical data that splits into different categories so you can quickly see comparative results and trends. • Pie Chart: Pie charts illustrate relative proportions of a specific measure. If the number of categories is more than a few consider using a bar chart. 15 Basic Charts & Graphs • Histogram: Histograms are used to show the frequency distribution of 1+ variables. The x-axis shows categories, and the y-axis the frequencies. • Scatter Plot: The scatter plot is often used to explore the relationship between two or three variables (in 2D or 3D visuals). • Bubble Chart: The bubble chart is an enhanced scatter plot. By varying the circles, one can add additional data dimensions, offering more enriched data. 16 Specialized Charts & Graphs • Geographic Map: Used when the data set includes any kind of location data. • Gantt Chart: A special case of bar charts used to portray project timelines, project tasks/activity durations, and overlap among the tasks/activities. • PERT Chart: developed primarily to simplify the scheduling of large/complex projects. A PERT chart shows relationships among project activities/tasks. 17 Specialized Charts & Graphs • Bullet: Used to show progress towards a goal. It is a variation of a bar chart. • Tree Map: A tree map displays hierarchical data as a set of nested rectangles. Each branch of the tree is given a rectangle, which is then tiled with smaller rectangles representing subbranches. 18 Specialized Charts & Graphs • Heat Map: A visual that illustrates the comparison of continuous values across two categories using color. • Highlight Table: Two-dimensional tables with cells populated with numerical values and gradients of colors. 19 Which Chart/Graph should you use? • The capabilities of the charts helps select the proper chart for a specific task.. 20 Which Chart/Graph should you use? • The taxonomic structure is organized around the purpose of the chart or graph. • The taxonomy divides the purpose into four different types—relationship, comparison, distribution, and composition—and further divides the branches into subcategories based on the number of variables involved and time dependency of the visualization. • The current trend is to combine and animate these charts for better-looking and more intuitive visualization of today’s complex and volatile data sources. 21 3.10 Emergence of Visual Analytics • • • • • Overview Visual Analytics What is a good story? Analysis as a Story High-Powered Visual Analytics Environments 22 Overview • In BI and analytics, the key challenge of visualization is representation of large, complex data sets with multiple dimensions and measures. • Typical charts, graphs, and visual elements used involve two dimensions, sometimes three, and fairly small subsets of data sets. • In contrast, data in visual systems reside in a data warehouse. At a minimum, these warehouses involve a range of dimensions (e.g., product, location, organizational structure, time), a range of measures, and millions of data cells. • In an effort to address these challenges, a number of researchers have developed a variety of new visualization techniques. 23 Visual Analytics • What is meant by visual analytics is the combination of visualization and predictive analytics. • Information visualization is aimed at answering “What happened?” and “What is happening?” and is closely associated with BI (routine reports, scorecards, and dashboards) • Visual analytics is aimed at answering “Why is it happening?” and “What is more likely to happen?” and is usually associated with business analytics (forecasting, segmentation, correlation analysis). 24 What is a Good Story? • Most people can easily remember a funny story because it contains certain characteristics and components, such as: o Good characters. o The character is faced with a challenge that is difficult but believable. o There are hurdles that the character overcomes. o The outcome or prognosis is clear by the end of the story. o The situation may not be resolved—but the story has a clear endpoint. 25 Analysis as a Story • With story elements in place, write out the storyboard, that represents the structure and form of your story. • The storyboard will help you think about the best analogies, clearly set up challenge or opportunity, and finally see the flow and transitions needed. • By following the best practices, you can get people to focus on your message: o Think of your analysis as a story—use a story structure. o Be authentic—your story will flow. o Be visual—think of yourself as a film editor. o Make it easy for your audience and you. o Invite and direct discussion. 26 High-Powered Visual Analytics Environments • There is a movement towards highly efficient visualization systems. SAS Visual Analytics, is a very high-performance computing, in-memory solution for exploring massive amounts of data in a very short time. • Benefits proposed by the SAS analytics platform are the following: o Empowers users with data exploration techniques and approachable analytics to drive improved decision making. o Has easy-to-use, interactive interfaces that broaden the audience for analytics. o Improves information sharing and collaboration. o Liberates IT by giving users a new way to access the information they need. o Provides room to grow at a self-determined pace. 27 E. High-Powered Visual Analytics Environments 28 3.11 Information Dashboards • • • • • • • • • • • 29 Overview Dashboard Design Best Practices in Dashboard Design Benchmark KPIs with Industry Standards Wrap the Dashboard Metrics with Contextual Metadata Validate the Dashboard Design by a Usability Specialist Prioritize and Rank Alerts/Exceptions Streamed to the Dashboard Enrich the Dashboard with Business-User Comments Present Information in Three Different Levels Pick the Right Visual Construct Using Dashboard Design Principles Provide for Guided Analytics Overview • Information dashboards are common components of BI platforms, business performance management systems, and measurement software suites. • Dashboards provide visual displays of important information arranged on a single screen so that the information can be digested at a single glance. • This executive dashboard shows functional groups surrounding the products intended to give executives a quick and accurate idea of what is going on within the organization. 30 Dashboard Design • Today, it would be rather unusual to see a large company using a BI system that does not employ some sort of performance dashboards. • The most distinctive feature of a dashboard is its three layers of information: o Monitoring: Graphical, abstracted data to monitor key performance metrics o Analysis: Summarized dimensional data to analyze the root cause of problems o Management: Data that identifies actions needed to resolve a problem • “The fundamental challenge of dashboard design is to display all the required information on a single screen.” 31 • Because of these layers, dashboards pack a large amount of information into a single screen Best Practices in Dashboard Design • Data is one of the most important things to focus on in dashboard design. • Even if a dashboard’s appearance looks professional, is aesthetically pleasing, and includes graphs and tables, it is also important to ask about the data: o Are they reliable? o Are they timely? o Are any data missing? o Are they consistent across all dashboards? 32 Benchmark KPIs with Industry Standards • Many customers want to know if the metrics they are measuring are the right metrics to monitor. • Sometimes customers have found that the metrics they are tracking are not the right ones to track. • Doing a gap assessment with industry benchmarks aligns you with industry best practices. 33 Wrap the Dashboard Metrics with Contextual Metadata • When a report or a visual dashboard/scorecard is presented to business users, questions remain unanswered. The following are some examples: o Where did you source these data? o While loading the data warehouse, what percentage of the data was rejected/ encountered data quality problems? o Is the dashboard presenting “fresh” information or “stale” information? o When was the data warehouse last refreshed? o When is it going to be refreshed next? 34 o Were any high-value transactions that would skew the overall trends rejected as a part of the loading process? Validate the Dashboard Design by a Usability Specialist • In most dashboard environments, the dashboard is designed by a tool specialist without giving consideration to usability principles. • Even though it is a well-engineered data warehouse that can perform well, many business users do not use the dashboard. • It is perceived as not being user friendly, leading to poor adoption of the infrastructure and change management issues. • Up-front validation of the dashboard design by a usability specialist can mitigate this risk. 35 Prioritize and Rank Alerts/Exceptions Streamed to the Dashboard • There are tons of raw data, having a mechanism by which important exceptions are proactively pushed to the information consumers is important. • A business rule can be codified, which detects the alert pattern of interest. • It can be coded into a program, using database-stored procedures, which crawl through the fact tables and detect patterns needing immediate attention. • This way, information finds the business user as opposed to the business user polling the fact tables for the occurrence of critical patterns. 36 Enrich the Dashboard with Business-User Comments • In the event that dashboard information is presented to multiple business users, a small text box can be provided to capture the comments from an end user’s perspective. • This can often be tagged to the dashboard to put the information in context, adding perspective to the structured KPIs being rendered. 37 Present Information in Three Different Levels • Information can be presented in three layers depending on the granularity of the information: o the visual dashboard level o the static report level o the self-service cube level • When a user navigates the dashboard, a simple set of 8 to 12 KPIs can be presented, which would give a sense of what is going well and what is not. 38 Pick Right Visual Construct Using Dashboard Design Principles • In a dashboard, some information is presented best with bar charts, or timeseries line graphs. • When presenting correlations, a scatter plot is useful. Sometimes merely rendering it as simple tables is effective. • Once the dashboard design principles are explicitly documented, all the developers working on the front end can adhere to the same principles while rendering the reports and dashboard. 39 Provide for Guided Analytics • In an organization, users can be at various levels of analytical maturity. • The capability of the dashboard can be used to guide a business user to access the same navigational path as that of an analytically savvy business user. 40 8.2 Model-Based Decision Making 6 • Prescriptive Analytics Model Examples • Identification of the Problem and Environmental Analysis • Model Categories • Current Trends in Modeling Prescriptive Analytics Model Examples • Modeling is a key element for prescriptive analytics. • Depending on the problem we are addressing, there are many classes of models, and there are often many specialized techniques for solving each one. • Prescriptive analytics involves the application of mathematical models, sometimes the term data science is more commonly associated with the application of such mathematical models. 7 Identification of the Problem and Environmental Analysis • It is important to analyze the scope of the domain and the forces and dynamics of the environment when making a decision. • A decision maker needs to identify the organizational culture and the corporate decision-making processes. 1. Environmental scanning and analysis is the monitoring, scanning, and interpretation of collected information. 2. Variable Identification is critical, as are the relationships among the variables. 1. Influence diagrams can facilitate the identification process. 8 2. A cognitive map, can help a decision maker develop a better understanding of a problem, and variable interactions. Identification of the Problem and Environmental Analysis 3. Predictive analytics (forecasting) is essential for constructing and manipulating models because the results of an implemented decision occur in the future. • There is no point in running a what-if (sensitivity) analysis on the past because decisions made then have no impact on the future. • Online commerce and communication has created an immense need for forecasting and an abundance of available information for performing it. • These activities occur quickly, yet information about such purchases is gathered and should be analyzed to produce forecasts. • Forecasting models use product life-cycle needs and information about the marketplace to analyze the situation. 9 Model Categories • The following table classifies decision models into seven groups and lists several representative techniques for each category. • Each technique can be applied to a static or a dynamic model, which can be constructed under assumed environments of certainty, uncertainty, or risk. 10 Model Categories (cont.) • To expedite model construction, we can use special decision analysis systems that have modeling languages and capabilities embedded in them. • These include spreadsheets, data mining systems, online analytic processing (OLAP) systems, and modeling languages. • Model Management: Models must be managed to maintain integrity, and applicability. This is done with the aid of model-based management systems, which are analogous to database management systems (DBMS). • Knowledge-based Modeling DSS: use quantitative models, whereas expert systems use qualitative, knowledge-based models in their applications. 11 Current Trends in Modeling • One trend in modeling involves the development of model libraries and solution technique libraries. • There is a clear trend toward developing and using cloud-based tools and software to run software to perform modeling, optimization, simulation, etc. • With management models, the amount of data and model sizes is large, necessitating data warehouses and parallel computing for solutions. • There is a trend toward making analytics models transparent to decision makers, and using influence diagrams (a model of a model to help in analysis). • Many decision makers accustomed to slicing and dicing data cubes are now using OLAP systems that access data warehouses. 12 8.3 Structure of Mathematical Models for Decision Support • Components of Decision Support Mathematical Models • Examples of Components of Models • The Structure of Mathematical Models 13 Components of Decision Support Mathematical Models • Quantitative models are made up of four basic components: result variables, decision variables, uncontrollable variables, and intermediate result variables. • Mathematical relationships link these components together. • In non-quantitative models, the relationships are symbolic or qualitative. The results of decisions are determined based on decisions made, uncontrollable variable, and relationships among variables. • The modeling process involves identifying the variables and relationships. Solving a model determines the values of these and the result variable(s). 14 Components of Decision Support Mathematical Models • Result/Outcome/Output Variables: reflect level of effectiveness of a system. • Decision Variables: Decision variables describe alternative courses of action. The decision maker controls the decision variables. • Uncontrollable Variables/Parameters: fixed/varying factors that affect the result variables but are not under the decision maker control. • Intermediate Result Variables: reflect intermediate outcomes in models. 15 Components of Decision Support Mathematical Models Examples 16 The Structure of Mathematical Models • Components of a quantitative model are linked by mathematical expressions. • A very simple financial model is: • Another financial model is the simple present-value cash flow model. • It is possible to determine the present value of a payment of $100,000 to be made 5 years from today, at a 10% interest rate, as follows: 17 8.4 Certainty, Uncertainty, and Risk 18 • Decision Making under Certainty • Decision Making under Uncertainty • Decision Making under Risk (Risk Analysis) Decision Making under Certainty • In decision making under certainty, complete knowledge is available so decision maker know the outcome of each course of action. • This is done with structured problems and short time horizons (up to 1 year). • Outcomes are not 100% known, but this assumption simplifies the model. • The decision maker is viewed as a perfect predictor of the future because it is assumed that there is only one outcome for each alternative. • Certainty models are easy to develop and solve, and yield optimal solutions. • Financial models are constructed under assumed certainty. 19 Decision Making under Uncertainty • Decision maker considers situation where several outcomes are possible for each course of action. • In contrast to the risk situation, the decision maker does not know, or cannot estimate, the probability of occurrence of the possible outcomes. • Modeling such situations involves assessment of the decision maker’s attitude toward risk. • Instead of dealing with uncertainty, manager’s sometimes attempt to obtain more information so that the problem can be treated under certainty. • If more information is not available, the problem must be treated under a condition of uncertainty, which is less definitive than the other categories. 20 Decision Making under Risk (Risk Analysis) • Risk analysis is a decision-making method that analyzes risk associated with alternatives, each with a given probability of occurrence. • The probabilities that the given outcomes will occur are assumed to be known or can be estimated. Under these assumptions, the decision maker can assess the degree of risk associated with each alternative (calculated risk). • Risk analysis can be performed by calculating the expected value of each alternative and selecting the one with the best expected value. 21 8.6 Mathematical Programming Optimization • Overview • Linear Programming Model • Implementation • Modeling in LP: An Example 22 Overview • Mathematical programming are tools that helps decision makers allocate scarce resources among competing activities to optimize a measurable goal. • Linear programming (LP) is the best-known technique in a family of optimization tools called mathematical programming. • In LP, all variable relationships are linear. Applications include supply chain management, product decisions, etc. LP allocation problems usually display the following characteristics: o Limited quantity of resources, most are used in product/service production. o Two or more ways resources can be used. Each is called a solution/program. o Each activity where resources are used, yields a return in terms of stated goal. 23 Linear Programming Model • The LP allocation model is based on the following economic assumptions: o Returns from allocations are independent and measured by a common unit o The total return is the sum of the returns yielded by the different activities. o All data are known with certainty, and resources are used economically • Allocation problems have a large number of possible solutions. Depending on the assumptions, the number of solutions can be either infinite or finite. • Different solutions yield different rewards. The solution with highest degree of goal attainment is called optimal solution, and found by a special algorithm 24 Linear Programming Model (cont.) • Every LP model is composed of: o Decision variables: Unknown values that are being searched for o Objective Function: A linear mathematical function that relates the decision variables to the goal, measures goal attainment, and is to be optimized o Coefficients: indicate contribution to objective of one unit of a decision variable o Constraint: Linear (in)equalities that limit resources o Capacities: Describe upper and lower limits on the constraints and variables o Input/output & Coefficients: Indicate resource utilization for a decision variable 25 Implementation • Implement the model in “standard form”, where constraints are written with decision variables on the left and a number on the right. • Alternatively, use spreadsheet to calculate the model in a less rigid manner. • LP models can be specified directly in a number of user-friendly modeling systems. Models are specified in the same way they are defined algebraically. • Optimization models can be solved by mathematical programming methods: o Assignment & Network models for planning and scheduling o Dynamic, Goal, Linear, Nonlinear and Integer programming o Investment & Replacement 26 o Simple inventory models & Transportation Modeling in LP: An Example • MBI Corporation, which manufactures special-purpose computers, needs to make a decision: How many computers should it produce next month at the Boston plant? • MBI is considering two types of computers: o CC-7, which requires 300 days of labor and $10,000 in materials, & o CC-8, which requires 500 days of labor and $15,000 in materials. • The profit contribution of each CC-7 is $8,000, and of each CC-8 is $12,000. • The plant has a capacity of 200,000 working days per month, and the material budget is $8 million per month. • Marketing requires that at least 100 units of the CC-7 and at least 200 units of the CC-8 be produced each month. • Problem: Maximize the company’s profits by determining how many units of the CC-7 and how many units of the CC-8 should be produced each month. 27 Modeling in LP: An Example (cont.) • The problem is to find the values of the decision variables X1, X2, such that the value of the result variable Z is maximized, subject to a set of linear constraints that express the technology, market conditions, and other uncontrollable variables. • The mathematical relationships are all linear equations and inequalities. 28 Modeling in LP: An Example (cont.) • Theoretically, any allocation problem of this type has an infinite number of possible solutions. • Using special mathematical procedures, the LP approach applies a unique computerized search procedure that finds the best (optimal) solution/s (ex. maximizes total profit) in a matter of seconds. • Excel ‘add-in Solver’ is used to obtain an optimal (best) solution to this problem. Open spreadsheet DS498_week7-Ch8d.xlsx 1. Activate ‘Solver’ Under the Data tab and on the Analysis ribbon. 29 If it is not there, you should be able to enable it by going to File -> Excel’s Options Menu and selecting Add-ins. Modeling in LP: An Example (cont.) 2. Enter these data directly into an Excel spreadsheet. 3. Identify the goal (by setting Target Cell equal to Max). 4. Identify decision variables (by setting By Changing Cells). 5. Identify constraints on labor capacity, budget, and the desired minimum production of the two products X1 and X2. 6. Clicking on the Solver Add-in opens a dialog box, o Specify the cells or ranges that define the objective function cell, decision/changing variables (cells), and the constraints. o Select the solution method (usually Simplex LP) o solve the problem. 7. Next, we select all three reports—Answer, Sensitivity, and Limits (optimal solution of X1 = 333.33, X2 = 200, Profit = $5,066,667) 30 8.7 Multiple Goals, Sensitivity Analysis, What-If Analysis, and Goal Seeking • Multiple Goals • Sensitivity Analysis • Types of Sensitivity Analysis • What-If Analysis • What-If Analysis Example • Goal Seeking • Goal Seeking Example 31 Multiple Goals • Today’s management systems are complex, and managers want to attain simultaneous goals, some of which may conflict. • Transform a multiple-goal problem into a single-measure-of effectiveness problem before comparing the effects of the solutions. • Certain difficulties may arise when analyzing multiple goals: o It is difficult to obtain the organization’s goals explicitly o Goals are viewed differently at various levels of the organization o Goals and their importance change in response to the organization o Complex problems are solved by decision makers with different agendas 32 Sensitivity Analysis • Sensitivity analysis assesses impact of input data changes on proposed solution. • Sensitivity analysis allows for: o Adaptation to conditions of different decision-making situations o Provides a better understanding of the model o Permits the input of data to increase model confidence. 33 Sensitivity Analysis (cont.) Sensitivity analysis tests relationships such as the following: • Impact of parameter change, and decision variables on outcome variable(s) • The effect of uncertainty in estimating external variables • The effect of different dependent interactions among variables • The robustness of decisions under changing conditions Sensitivity analyses are used for: • Revising models to eliminate too-large sensitivities • Detailing variables and obtaining estimates of sensitive external variables • Altering a real-world system to reduce actual sensitivities 34 Types of Sensitivity Analysis • The two types of sensitivity analyses are automatic and trial and error. Automatic Sensitivity Analysis: • This is performed in standard quantitative model implementations such as LP. • It is usually limited to one change at a time, and only for certain variables. • It is powerful because of its ability to establish ranges and limits very fast Trial-and-error Sensitivity Analysis: • Impact of changes in variable(s) is determined by trial-and-error approach • When changes are repeated, better and better solutions may be discovered. • Such experimentation has two approaches: what-if analysis and goal seeking. 35 What-If Analysis • What-if analysis is structured as: What will happen to the solution if an input variable, assumption, or parameter value is changed? o Total inventory cost if the carrying inventories cost increases by 10%? o Market share if the advertising budget increases by 5%? • With the appropriate user interface, managers can ask a computer model these types of questions and get immediate answers. • Performs multiple cases and change the percentage, or other data as needed. • What-if analysis is common in many decision systems. Users are given the opportunity to change their answers to the system’s questions, and a revised recommendation is found. 36 What-If Analysis Example A what-if query for a cash flow problem: the user changes the cells containing the initial sales (from 100 to 120) and the sales growth rate (from 3% to 4% per quarter), the program immediately re-computes the value of the annual net profit cell (from $127 to $182) . 37 Goal Seeking • Goal seeking calculates the values of the inputs necessary to achieve a desired level of an output (goal). The following are some examples: o Annual R&D budget is needed for an annual growth rate of 15% by 2018? o How many nurses needed to reduce the average waiting time of a patient in the emergency room to less than 10 minutes? • Computing A Break-even Point By Using Goal Seeking: o Determining the value of the decision variables that generate zero profit. o Some modeling software packages can directly compute break-even points, which is an important application of goal seeking. o Sensitivity analysis as the prewritten routines present a limited opportunity for asking what-if questions. 38 Goal Seeking • In a financial planning model, the internal rate of return (IRR) is the interest rate that produces a net present value (NPV) of zero. • Given a stream of annual returns in Column E, we can compute the NPV of planned investment through goal-seeking. • An NPV equal to zero determines the IRR of this cash flow, including the investment. We set the NPV cell to 0 by changing the interest rate cell. • The answer is 38.77059%. 39 8.8 Decision Analysis with Decision Tables & Decision Trees • • • Decision Tables Decision Tables Example Decision Trees 40 Decision Tables • Decision tables organize information in systematic, tabular form for analysis. • Treating Uncertainty: Several methods are available for handling uncertainty. o Optimistic approach: assumes and selects best outcomes for alternatives o Pessimistic approach: assumes worst outcome for alternatives; selects the best o Another approach simply assumes that all states of nature are equally possible. • When possible, analysts should attempt to gather information to treat the problem under assumed certainty. • Treating Risk: The most common method for solving this risk analysis problem is to select the alternative with the greatest expected value. 41 Decision Tables Example • An investor estimates: solid growth (50%), stagnation (30%), and inflation (20%) • Expected value is computed by multiplying result probabilities and adding them Bond investment yields an expected return of 12(0.5) + 6(0.3) + 3(0.2) = 8.4% • This approach can sometimes be a dangerous strategy such as a financial advisor presents a $1,000 investment with 0.9999 chance to double your money, and 0.0001 chance you’ll lose $500,000. • The expected value of this investment is $949.80 • The potential loss could be catastrophic for any investor 42 Decision Trees • Decision trees are alternative representations of a decision table; it shows a problem’s relationship graphically and handles complex situations compactly. • TreeAge Pro & PrecisionTree are systems that show decision trees in practice. • You can apply mathematical programming to decision-making situations under risk. These include simulation, certainty factors, and fuzzy logic. • A simplified investment case of multiple goals is shown in the table. The three goals are yield, safety, and liquidity. This situation is under assumed certainty. 43 8.9 Introduction to Simulation • • • • • • • Major Characteristics of Simulation Advantages of Simulation Disadvantages of Simulation The Methodology of Simulation Simulation Types Monte Carlo Simulation Discrete Event Simulation 44 Major Characteristics of Simulation • Simulation involves building a model of reality to the extent practical. • Simulation models may suffer from fewer assumptions about the decision situation as compared to other prescriptive analytic models. • Simulation is a technique for conducting experiments. Therefore, it involves testing specific values of the decision or uncontrollable variables in the model and observing the impact on the output variables. • Simulation is used only when a problem is too complex to be treated using numerical optimization techniques. • Complexity in this situation means either that the problem cannot be formulated for optimization, that the formulation is too large, that there are too many interactions among the variables. 45 Advantages of Simulation Simulation is used in decision support modeling for the following reasons: • The theory is straightforward, and model is built from manager’s perspective. • Time compression is attained quickly to give idea of policies’ long-term effects. • Descriptive rather than normative, allowing managers to ask what-if questions, and use a trial-and-error approach with less expense and risk. • Requires intimate knowledge; model builder constantly interact with manager. • Can handle a variety of problem types and higher-level managerial functions. • Produces performance measures, and includes real complexities of problems. • Can readily handle relatively unstructured problems. 46 Disadvantages of Simulation • An optimal solution cannot be guaranteed, but relatively good ones are generally found. • Simulation model construction can be a slow and costly process, although newer modeling systems are easier to use than ever. • Solutions and inferences from a simulation study are usually not transferable to other problems because the model incorporates unique problem factors. • Simulation is sometimes so easy to explain to managers that analytic methods are often overlooked. • Simulation software sometimes requires special skills because of the complexity of the formal solution method. 47 The Methodology of Simulation Simulation involves setting up a model of a real system through the steps: • Define the problem: Examine problem, and specify need for simulation • Construct model: Determine variables, relationships, and gather data. • Test and validate model: Ensure model properly represents studied system. • Design experiment: There are two conflicting objectives: accuracy and cost. • Conduct experiment: can involve issues like number generation. • Evaluate results: Statistical tools/sensitivity analyses used to interpret results. • Implement results: Managerial involvement leads to implementation success. 48 The Methodology of Simulation 49 Simulation Types • Simulation model consists of relationships that present the real-world operations. Simulation results depend on the set of parameters given as inputs. • There are various simulation paradigms such as Monte Carlo simulation, discrete event, agent based, or system dynamics. • The level of abstraction in a problem can determine simulation technique. • Discrete events and agent based models are used for low levels of abstraction. • They consider individual elements such as people in the simulation models, whereas systems dynamics is more appropriate for aggregate analysis. • Here we introduce the major types of simulation: probabilistic simulation, timedependent and time-independent simulation, and visual simulation. 50 Simulation Types • Probabilistic Simulation: One or more independent variables are probabilistic. They follow probability distributions, which can be discrete or continuous: o Discrete involves situations with limited event numbers and finite values. o Continuous distributions are situations with unlimited numbers of possible events that follow density functions, such as the normal distribution. • Time-dependent Versus Time-independent Simulation: o Time-independent refers to situations where time of event occurrence is unimportant. For example, we may know that the demand for a product is 3 units/day, but do not care when during the day the item is demanded. o However, in waiting-line problems applicable to e-commerce, it is important to know the precise time of arrival. This is a time-dependent situation. 51 Monte Carlo Simulation • In business decision problems, we employ probabilistic simulations. The Monte Carlo simulation is commonly used. • This method begins with building a model of the decision problem without having to consider the uncertainty of any variables. • Then we recognize that certain variables are uncertain or follow an estimated probability distribution. This estimation is based on analysis of past data. • Then we begin running sampling experiments. This consists of generating random values of uncertain parameters and then computing values of the variables that are impacted by such parameters or variables. • We then analyze the behavior of these performance variables by examining their statistical distributions. 52 Discrete Event Simulation • Discrete event simulation refers to building a model of a system where the interaction between different entities is studied. • An example of this is modeling the customers arriving at various rates and the server serving at various rates, we can estimate the average system performance, waiting time, number of waiting customers, etc. Such systems are viewed as collections of customers, queues, and servers. • There are thousands of documented applications of discrete event simulation models in engineering, business, and so on. • Tools for building discrete event simulation models have been around for a long time, but these have evolved to take advantage of developments in graphical capabilities for building and understanding the results of such simulation models. 53 8.10 Visual Interactive Simulation • • 54 Visual Interactive Simulation Visual Interactive Models and DSS Visual Interactive Simulation • Visual interactive simulation (VIS), visual interactive modeling (VIM) and visual interactive problem solving, is a simulation method that lets decision makers see what a model is doing, how it interacts with made decisions. • Users employ knowledge to try different decision strategies while interacting with the model. Decision makers can contribute to model validation. • VIS uses animated computer graphic displays to present the impact of different managerial decisions. It differs from regular graphics in that the user can adjust the decision-making process and see results of the intervention. • VIS can represent static or dynamic systems. Static models display a visual image of the result of one decision alternative at a time. Dynamic models display evolving systems over time. The evolution is represented by animation. 55 Visual Interactive Models (VIM) and DSS • VIM in DSS has been used in several operations management decisions. • The method consists of priming a visual interactive model of a company with its current status. • Waiting-line management is a good example of VIM. Such a DSS usually computes measures of performance for the various decision alternatives. • Complex waiting-line problems require simulation. VIM can display the size of the waiting line as it changes during the simulation runs and can graphically present the answers to what-if questions regarding changes in input variables. • The VIM approach can be used with AI. Integration of the two techniques adds several capabilities that range from the ability to build systems graphically to learning about the dynamics of the system. 56 6.2 Introduction to Deep Learning • Introduction to Deep Learning • Classic Machine-Learning vs Deep Learning Introduction to Deep Learning • Deep learning is among the latest trends in AI that come with great expectations. • The initial idea of deep learning goes back to the late 1980s. • Goal: mimic the thought process of humans—using mathematical algorithms to learn from data pretty much the same way that humans learn (similar to those of the other machine-leaning methods). • It has added the ability to automatically acquire the features required to accomplish highly complex and unstructured tasks (e.g. image recognition) to the classic machine-learning methods that contribute to the superior system performance. • The recent emergence and popularity of deep learning can largely be attributed to very large data sets and rapidly advancing commuting infrastructures. • Many deep learning applications have promised to make our life easier. E.g., Google Home, Amazon’s Alexa, Google Translate, …) Introduction to Deep Learning • Deep learning is an extension of neural networks with the idea that deep learning is able to deal with more complicated tasks with a higher level of sophistication. • Neural networks are extended by employing many layers of connected neurons along with much larger data sets to automatically characterized variables and solve the problems. • The initial idea of deep learning had to wait more than two decades until some advanced computational and technological infrastructure emerged, because of: 1. Very high computational requirement. 2. The need for very large data sets. Introduction to Deep Learning Placement of Deep Learning within the Overarching AI-Based Learning Methods • Deep learning is categorized as part of the representation learning within the AI learning family of methods • Representation learning focus on learning and discovering features by the system in addition to discovering the mapping from those features to the output/target. Classic Machine-Learning vs Deep Learning • In Knowledge-based systems and classic machine-learning methods, features (i.e., the representation) are created manually by data scientists to achieve the desired output. • Deep learning enables the computer to derive some complex features from simple concepts that would be very effort intensive to be discovered by humans manually. 6.3 Basics of “Shallow” Neural Networks • Artificial Neural Networks (ANN) • Elements of an Artificial Neural Network • Common Transfer Functions in Neural Networks • The human brain has a set of billions of interconnected neurons that facilitate our thinking, learning, and understanding of the world around us. • Artificial neural networks emulate the way the human brain works. • The basic processing unit is a neuron. Multiple neurons are grouped into layers and linked together. VS neurons A Biological Neural Network: Two Interconnected Cells/Neurons. ANN with single neuron, single inputs and outputs • The basic processing unit is a neuron (processing element – PE). • PE: perform a set of predefined mathematical operations on the numerical values coming from the input or from the other neuron outputs to create and push out its own outputs. PE PE PE • A neuron can have more than a single input p, each of the individual input values would have its own adjustable weight w. • In a neural network, knowledge is stored in the weight associated with the connections between neurons. Typical Neural Network with Three Layers and Eight Neurons. • Multiple neurons are grouped into layers and linked together. Ele me nts of an Arti fici al N eu ral N etw ork • Processing element (PE) • Network architecture • Hidden layers • Parallel processing • Network information processing • Inputs • Outputs • Connection weights • Summation function • Transfer Function Elements of an Artificial Neural Network Summation Function for a Single Neuron/PE (a), and Several Neurons/PEs (b) Neural Network with One Hidden Layer • Various types of transfer functions are commonly used in the design of neural networks. • Common Transfer Function types (Linear function, Sigmoid (log) function [0 1] and Tangent Hyperbolic function [-1 1]). • Example of ANN Transfer Function (sigmoid-type activation function) • The selection of proper transfer functions for a network requires a broad knowledge of neural networks ( e.g. characteristics of the data as well as the specific purpose for which the network is created). • There are some guidelines for choosing the appropriate transfer function especially for the neurons located at the output layer of the network. • E.g., if the nature of the output for a model is binary, it is advised to use Sigmoid transfer functions at the output layer so that it produces an output between 0 and 1. some of the most common transfer functions and their corresponding operations 6.4 Process of Developing Neural Network–Based Systems • Development Process of an ANN Model • Learning Process in ANN • Backpropagation Learning for ANN • Overfitting in ANN • Developing neural network–based systems requires a step-by-step process. • A supervised learning process. • The learning process is inductive; that is, connection weights are derived from existing cases. • The usual process of learning involves three tasks: Compute temporary outputs. Compare outputs with desired targets. Adjust the weights and repeat the process. Supervised Learning Process of an ANN. • Backpropagation is the most popular supervised learning paradigm for ANN. Backpropagation of Error for a Single Neuron Backpropagation Learning for ANN The learning algorithm procedure: 1. Initialize weights with random values and set other parameters. 2. Read in the input vector and the desired output. 3. Compute the actual output via the calculations, working forward through the layers. 4. Compute the error. 5. Change the weights by working backward from the output layer through the hidden layers. • Occurs when neural networks are trained for a large number of iterations with relatively small data sets. • To prevent overfitting, the training process is controlled by an assessment process using a separate validation data set. 6.5 ILLUMINATING THE BLACK BOX OF ANN Overfitting in ANN—Gradually Changing Error Rates in the Training and Validation Data Sets As the Number of Iterations Increases. • Sensitivity Analysis on ANN Models • • • • ANNs are known as black-box models. But, “how the model does what it does?” ANNs lack of explanation/transparency -> black-box syndrome! To shed light into the black-box syndrome sensitivity analysis is applied. Sensitivity analysis: 1. Preformed on a trained ANN 2. Perturbed the inputs to the network systematically within the allowable value ranges. 3. The corresponding change in the output is recorded for each and every input variable. 4. The relative importance of input variables are illustrated in the result. • Sensitivity analysis extract the cause-and-effect relationships among the inputs and the outputs of a trained neural network model. •6.6 Deep Deep Neural Networks Neural Networks • Feedforward Multilayer Perceptron (MLP) • Most neural network applications involved network architectures with only a few hidden layers and a limited number of neurons in each layer. • Deep neural networks broke the generally accepted notion of “no more than two hidden layers are needed to formulate complex prediction problems.” • They promote increasing the hidden layer to arbitrarily large numbers to better represent the complexity in the data set. • Different types of deep networks involve various modifications to the architecture of standard neural networks. Typically equipped with distinct capabilities of dealing with particular data types for advanced purposes (e.g. image or text processing). • MLP deep networks (a.k.a deep feedforward networks) are the most general type of deep networks. • MLP Consists of an input layer, an output layer, and a number of hidden layers. • The nodes in one layer are connected to the nodes in the next layer. • Each node at the input layer typically represents a single attribute that may affect the prediction. • The flow of information is always forwarding and no feedback connections, hence it is called “called feedforward network”. More Hidden Layers versus More Neurons? it is still an open research question, practically using more layers in a network seems to be more and computationally more efficient than using many neurons in a few layers. The First Three Layers in a Typical MLP Network. Deep COMPUTER FRAMEWORKS FOR IMPLEMENTATION •6.7 Frameworks OF DEEP LEARNING • Example DL Applications • Deep learning implementation frameworks (open-source) include: Torch: is a scientific computing framework for implementing machine-learning algorithms using GPUs. Caffe: The deep learning libraries are written in the C++ programming language, everything is done using text files instead of code. TensorFlow: a popular deep learning framework, It was originally developed by the Google Brain Group. Theano: one of the first deep learning frameworks Keras: functions as a high-level application programming interface (API) and is able to run on top of various deep learning frameworks including Theano and TensorFlow. Source: https://www.mygreatlearning.com/blog/what-is-deep-learning/ •6.8 Conceptual Cognitive ComputingFramework for Cognitive Computing • How Does Cognitive Computing Work? • Cognitive Computing and AI • Typical use cases for cognitive computing • Cognitive analytics and Search • IBM Watson • Cognitive computing makes a new class of problems computable. • It address highly complex situations that are characterized by ambiguity and uncertainty. • Handles the kinds of problems that are thought to be solvable by human ingenuity and creativity. • Computing system offers a synthesis not just of information sources but also of influences, contexts, and insights that help users understand their problems. • To provide the best possible answers to a given question or problem, cognitive computing: finds and synthesizes data from various information sources, And weighs the context and conflicting evidence inherent in the data. And suggest an answer that is “best” rather than “right.” a general framework for cognitive computing where data and AI technologies are used to solve • Cognitive computing works much like a human thought process, reasoning mechanism, and cognitive system. • It includes self-learning technologies that use data mining, pattern recognition, deep learning, and NLP to mimic the way the human brain works. • Cognitive systems may draw on multiple sources of vast amounts of information, including structured and unstructured data and visual, auditory, or sensor data solve the types of problems that humans are typically tasked. • Over time, cognitive systems are able to refine the way in which they learn and recognize patterns and the way they process data to become capable of anticipating new problems and modelling and proposing possible solutions. H o w D oe s C og nitiv e C om pu ting W ork ? The key attributes of cognitive computing capabilities: Adaptability: be flexible enough to learn as information changes and goals evolve. Interactivity: Users must be able to interact with cognitive machines and define their needs as those needs change. Iterative and stateful: ability to maintaining information about similar situations that have previously occurred. Contextual: must understand, identify, and mine contextual data, such as syntax, time, location, domain, requirements, and a specific user’s profile, tasks, or goals. Cognitive Computing and AI • Development of smart and adaptive search engines. • Effective use of natural language processing. • Speech recognition. • Language translation. • Context-based sentiment analysis. • Face recognition and facial emotion detection. • Risk assessment and mitigation. • Fraud detection and mitigation. • Behavioral assessment and recommendations. Typical use cases for cognitive computing • Cognitive analytics is a term that refers to cognitive computing–branded technology platforms. E.g., IBM Watson specialize in the processing and analysis of large unstructured data sets. • The benefit of utilizing cognitive analytics over traditional Big Data analytics tools is that for cognitive analytics such data sets do not need to be pretagged. • Cognitive analytics systems can use machine learning to adapt to different contexts with minimal human supervision. These systems can be equipped with a chatbot or search assistant that understands queries, explains data insights, and interacts with humans in human languages. • Searching for information is a tedious task. • Cognitive search is the new generation of search method that uses AI (e.g., advanced indexing, NLP, and machine learning) to return results that are much more relevant to the user than traditional search methods. • It creates searchable information out of non-searchable content by leveraging cognitive computing algorithms to create an indexing platform. • Cognitive search proposes the next generation of search tailored for use in enterprises. Cognitive search is different from traditional search because, according to Gualtieri (2017), it: Can handle a variety of data types. Can contextualize the search space. Employ advanced AI technologies. Enable developers to build enterprisespecific search applications. The progressive evolution of search methods. • IBM Watson is perhaps the smartest computer system built to date. It has coined and popularized the term cognitive computing. • It is an extraordinary computer system—a novel combination of advanced hardware and software—designed to answer questions posed in natural human language. • IBM Watson beat the best of men (the two most winning competitors) at the quiz game Jeopardy!, showcasing the ability of commuters to do tasks that are designed for human intelligence. • Watson and systems like it are now in use in many application areas including: Healthcare, finance, security, retail, education, government and research. • DeepQA is the system behind Watson, which is a massively parallel, text mining– focused, probabilistic evidence–based computational architecture. • Goal: to bring their strengths to bear and contribute to improvements in accuracy, confidence, and speed. Principles in DeepQA Massive parallelism. Many experts. Pervasive confidence estimation. Integration of shallow and deep knowledge. A High-Level Depiction of DeepQA Architecture College of Computing and Informatics Assignment 1 Deadline: Day 05/03/2024 @ 23:59 [Total Mark for this Assignment is 8] Student Details: Name: ### ID: ### CRN: ### Instructions: • You must submit two separate copies (one Word file and one PDF file) using the Assignment Template on Blackboard via the allocated folder. These files must not be in compressed format. • It is your responsibility to check and make sure that you have uploaded both the correct files. • Zero mark will be given if you try to bypass the SafeAssign (e.g. misspell words, remove spaces between words, hide characters, use different character sets, convert text into image or languages other than English or any kind of manipulation). • Email submission will not be accepted. • You are advised to make your work clear and well-presented. This includes filling your information on the cover page. • You must use this template, failing which will result in zero mark. • You MUST show all your work, and text must not be converted into an image, unless specified otherwise by the question. • Late submission will result in ZERO mark. • The work should be your own, copying from students or other resources will result in ZERO mark. • Use Times New Roman font for all your answers. Question One Pg. 01 Learning Outcome(s):CLO1 Demonstrate an understanding of the concepts of Question One Explain the concept of Business Intelligence (BI) and its significance in decision-making processes within organizations. Provide examples to illustrate how BI tools and techniques can help businesses gain insights and make informed decisions. decision analysis and decision support
Collepals.com Plagiarism Free Papers
Are you looking for custom essay writing service or even dissertation writing services? Just request for our write my paper service, and we'll match you with the best essay writer in your subject! With an exceptional team of professional academic experts in a wide range of subjects, we can guarantee you an unrivaled quality of custom-written papers.
Get ZERO PLAGIARISM, HUMAN WRITTEN ESSAYS
Why Hire Collepals.com writers to do your paper?
Quality- We are experienced and have access to ample research materials.
We write plagiarism Free Content
Confidential- We never share or sell your personal information to third parties.
Support-Chat with us today! We are always waiting to answer all your questions.
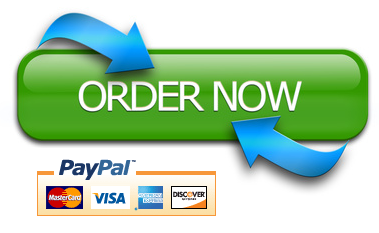