Utilizing the article attached (citation: Lazzati, N., & Menichini, A. A. (2016). Hot Spot Policing: A Study of Place-Based Strategies for Crime Prevention. Southern Economic Journal, 82(3)
Assessment Description
Utilizing the article attached (citation: Lazzati, N., & Menichini, A. A. (2016). Hot Spot Policing: A Study of Place-Based Strategies for Crime Prevention. Southern Economic Journal, 82(3), 893–913. https://doi-org.lopes.idm.oclc.org/10.1002/soej.12112 )
and conducting your own research, pick below topic and explain how you would implement it most effectively for your own police department:
- Focusing police resources and patrol on high-crime “hot spots”
Acting as the police chief, create a policy proposal of 750-1,000 words by doing the following:
Create a policy proposal on the chosen topic above.
- Explain how you would implement the policy.
- Delineate why this policy is important.
- Discuss how it could effectively reduce crime or improve community relations with police.
- Explain how and when you would evaluate this policy to determine if it is effective or not, and if it producing unintended consequences.
- Analyze how the implementation of this policy could affect the culture of the police department. What possible pitfalls or benefits may occur to the culture when implementing change to the police department?
Be sure to cite three to five relevant scholarly sources in support of your content. Use only sources found at the , government websites or those provided in Class Resources that are not older than 2018.
Prepare this assignment according to the APA 7 guidelines.
This assignment uses a rubric. Please review the attached rubric prior to beginning the assignment to become familiar with the expectations for successful completion.
Hot Spot Policing: A Study of Place-Based Strategies for Crime Prevention
Natalia Lazzati* and Amilcar A. Menichini†‡
Hot spot policing is a popular policing strategy that addresses crime by assigning limited police resources to areas where crimes are more highly concentrated. We analyze this strategy using a game theoretic approach. The main argument against focusing police resources on hot spots is that it would simply displace criminal activity from one area to another. We provide new insights on the nature of the displacement effect with useful implications for the empirical analysis of crime-reduction effects of police reallocation. We also propose alternative place- based policies that display attractive properties in terms of geographic spillovers of crime reduction via optimal police reallocation.
JEL Classification: D7, K4, R1
1. Introduction
Crime mapping is a powerful tool used by analysts in law enforcement agencies to visualize and study crime patterns. Such maps indicate that crimes are often not evenly distributed across geo- graphic locations. Instead, clusters of crimes occur in specific areas, or hot spots. Hot spot policing is a place-based strategy that attempts to reduce crime by assigning limited police resources to places where crimes are more highly concentrated. This approach to crime prevention is relatively new and many crime experts argue it is one of the main reasons why New York City has achieved a dramatic decrease in crimes during the past two decades.1 We analyze this policy via a game theoretic approach and propose alternative strategies that display interesting features in terms of geographic spillovers of crime reduction. We pay special attention to the so-called displacement effect and discuss the implica- tions of our results for the empirical research on police effectiveness regarding crime reduction. While our results support the doctrine on hot spot policing, they also raise some concerns regarding the extreme implementation of this policing strategy.
The model we develop to study the effectiveness of hot spot policing incorporates various crime theories that capture different aspects of crime decisions. These theories have been so far studied in isolation and, by combining them in a single model, we are able to make predictions that are more consistent with observed patterns of crimes. Specifically, our approach is based on the rational choice model and uses game theory to incorporate strategic interactions among poten- tial offenders into the analysis. We also borrow from the theory of environmental criminology,
* Economics Department, University of California, Santa Cruz, 1156 High Street, Santa Cruz, CA 95064, USA; E-mail: [email protected]; corresponding author.
† Economics Department, University of California, Santa Cruz, 1156 High Street, Santa Cruz, CA 95064, USA; E-mail: [email protected].
‡ Graduate School of Business and Public Policy, Naval Postgraduate School, 555 Dyer Road, Monterey, CA 93943-5000, USA; E-mail: [email protected].
Received December 2014; accepted May 2015. 1 See, for example, Zimring (2012).
� 2015 by the Southern Economic Association 893
Southern Economic Journal 2016, 82(3), 893–913 DOI: 10.1002/soej.12112
which highlights the role of spatial factors in the choice of crime location.2 More formally, we pro- pose a two-stage game. We first divide the region under study into a finite number of areas that dif- fer in terms of attractiveness for potential offenders. For instance, if the overall region represents NYC, then an area may correspond to one of its neighborhoods. We capture crime attractiveness via two attributes, namely, risk of apprehension and potential productivity. The riskiness of a place for a potential offender can be thought of as an index function that captures structural factors affecting the successful apprehension of offenders in that location, such as the presence of illumina- tion or video cameras. The second attribute, potential productivity, relates to the expected gains from committing a crime in that place, such as the presence of a shopping mall or a bank. In the first period of the two-stage game, the enforcement agency decides how to allocate the limited police resources across alternative areas. In the second period, upon observing police allocation, people decide whether to commit a crime and, in case of doing so, where to perform the criminal act.
Using the standard backward induction principle, we solve the game by first modeling peo- ple�s choices for a given police assignment. This first part of our work sheds light on one of the most controversial issues associated with hot spot policing, namely, the displacement effect. That is, the possibility that increasing police resources in hot spots would simply displace criminal activ- ity from one area to the others.3 Empirical research has found some evidence against this argu- ment (see, e.g., Di Tella and Schargrodsky 2004; Braga 2008, Cook and MacDonald 2011). Our model features this characteristic when the value of the outside option (not to commit a crime) does not depend on the number of people who opt not to become a criminal and there are no com- plementarities in the criminal activity. Under these circumstances, the value of the outside option regulates people�s utilities, and increasing resources in an area simply discourages people in that area from committing a crime. This lack of displacement effect also relies on the assumption that, in order to increase the police resources in a specific area, the enforcing agency does not need to diminish them from an alternative one. When at least one of these conditions fails, the previous result does no longer hold. Under these alternative circumstances, increasing police resources in areas with high criminality pushes criminals from these locations to the others. This result raises a simultaneity issue in the empirical studies that aim to measure the effect of increases in police on crime reduction using panel data. More precisely, any such study should take into account that the crime rate in each area depends not only on the police resources allocated to that specific area but on the whole vector of police allocation. We provide specific conditions under which researchers should use more sophisticated econometric approaches.
After we characterize the decisions of potential offenders, we go back to the first stage of the game and describe the crime-minimizing police allocation. We find that, at the optimal police allo- cation, areas that are a priori more attractive to offenders (i.e., display a larger productivity-to-risk ratio) receive indeed more police attention. However, in our model, the extra efforts in these areas do not fully offset the impact of their initial structural differences. That is, some hot spots still remain under the crime-minimizing allocation strategy. We then contrast the optimal police allo- cation with the behavior of an enforcement agency that aims to eliminate crime differences across areas. This egalitarian policy can be thought of as taking the implementation of hot spot policing to the limit. We find that the latter implies a higher overall crime rate and its opportunity cost
2 See, for example, Brantingham and Brantingham (1991). 3 Reppeto (1976) offers an early discussion on the different types of displacement effects in criminal activity. Ellen,
Lacoe, and Sharygin (2013) study the displacement of crimes due to foreclosures.
894 Natalia Lazzati and Amilcar A. Menichini
increases with the variability of the productivity-to-risk ratio across areas. In summary, we find that the optimal crime reduction strategy does involve differential targeting of potentially high crime locations, as argued by the doctrine on hot spot policing. Nevertheless, our results also sug- gest that extreme implementations of this strategy should be carefully evaluated in terms of the ultimate objectives, as it might have the unintentional effect of increasing the overall crime rate. These results are robust to all the extensions we consider for our initial model. In particular, they remain valid independently of the displacement effects.
We then study an alternative place-based strategy. Specifically, we analyze the implications of introducing structural changes that aim to reduce the productivity-to-risk ratio of a certain area (e.g., installing video cameras on the streets). This policy has been suggested by a number of crime theorists, including Braga and Weisburd (2010), who state that:
The attributes of a place are viewed as key factors in explaining clusters of criminal events. . . To reduce and better manage problems at crime hot spots, the police need to change the under- lying conditions, situations, and dynamics that make them attractive to criminals and disor- derly persons.
We find that this policy reduces crime not only in the target area but also in all other locations. Positive (or negative) external effects of structural changes have been proposed ear- lier (see, e.g., Ehrlich 1973; Glaeser and Gottlieb 2008). An interesting aspect of our result is the mechanism that produces this outcome. The direct effect of the policy is to make the tar- get area less attractive for potential offenders, thereby reducing its criminal activity. The indi- rect effect is due to subsequent police reallocation from the improved area to the other ones, where the criminal activity diminishes as well. In other words, structural improvements in an area generate geographic spillovers of crime reduction in all other locations via optimal police reallocation.
We finally consider various extensions to our initial model. The outside option people face (not to commit a crime) could be interpreted as the possibility to get a legal job. We then intro- duce alternative specifications of the outside option and study the effects of improvements in the job market on the proportion of people who choose not to commit a crime, for example, the labor supply of the economy. We find that, when the outside option displays congestion effects, increasing police resources in a given area pushes some criminals from this area to the others—even if we do not reduce the police resources from these other areas. Congestion effects in the outside option might occur if, for instance, an increment in the number of people search- ing for a legal job pushes salaries down or increases unemployment, thereby making this out- side option less attractive. As we mentioned earlier, this result raises a simultaneity issue in the empirical studies that address the effect of police levels on crime reduction using panel data. Finally, we investigate the consequences of reversing the interaction effects among potential offenders. We show that, when interactions are positive—as in Freeman, Grogger, and Sonstelie (1996) and Sah (1991)—interventions become a delicate matter. (By positive interactions, we mean that the payoff of committing a crime in a specific area increases when more people decide to do so.) The reason is that these models often display multiple equilibria and policy interventions can easily affect equilibrium selection (see Blume 2006). In this context, the dis- placement effect can also take place and be quite extreme. We explore this possibility with a simple example.
Hot Spot Policing 895
Literature Review
Our research contributes to work in both criminal studies and economics. In an early study, Becker (1968) examines individual decisions to commit crimes from an economic perspective.4 His cost-benefit analysis is consistent with the rational choice approach used by Cornish and Clarke (1986), which we follow as well.5 Our study also relates to subsequent work on the importance of social interactions in motivating criminal behavior (see, e.g., Sah 1991; Freeman, Grogger, and Sonstelie 1996, Glaeser, Sacerdote, and Scheinkman 1996; Ballester, Calv�o-Armengol, and Zenou 2006, 2010; Chen and Shapiro 2007). Bayer and Timmins (2005) develop the equilibrium properties of an estima- ble model of location choice that incorporates social interactions. Following the latter, we assume that each individual decision depends on other people�s criminal choices. To incorporate spatial factors into the analysis, we model people�s expected payoffs as in Hugie and Dill (1994), who study habitat selection by modeling the behavior of predators and prey (see also Helsley and Zenou 2014).
As we mentioned above, several studies find empirical evidence of no displacement effect in the criminal activity. Among them, Di Tella and Schargrodsky (2004) analyze the aftermath of a terrorist attack in Argentina in 1994, which led to massive police presence in certain sensitive loca- tions. They find large effects on motor vehicle theft with very limited evidence of displacement. Cook and MacDonald (2011) evaluate private security activities by business improvement dis- tricts. They also document no evidence that crime is displaced to neighboring areas. See also Braga (2008) and Braga and Weisburd (2010) for a large review of empirical evidence on this phenom- enon. As noted earlier, we derive specific conditions under which the displacement effect does and does not take place.
Our work is also related to Espejo, L�Huillier, and Weber (2011), who provide an evaluation of hot spot policing using a leader and follower model, as we do in this investigation. Our article differs from theirs in a few dimensions. First, the model of Espejo, L�Huillier, and Weber (2011) does not include an outside option for the potential criminals. As a consequence, the overall level of criminality does not depend on the police allocation and they are not able to characterize the displacement effect—which is one of our main focuses. Second, their model does not provide insights regarding the determinants of payoffs and, therefore, they cannot evaluate the alternative place-based policies we study here. Finally, Espejo, L�Huillier, and Weber (2011) do not discuss the possibility of positive interactions.
In this study, we assume that people observe police allocations and make subsequent choices regarding crime decisions and the location of the criminal acts. Lazear (2006) shows that it may be optimal, under certain circumstances, to keep police allocations secret. Although our model is quite different from his—for instance, we introduce social interactions—it is important to remark that our results remain unchanged if we allow for randomized police allocations. The reason is that the effect of police enters people�s utilities in a linear fashion. It would be interesting to study the advantages of secret police allocations in a model similar to the one of Lazear (2006) but with the additional feature of social interactions. We leave this analysis for future research.
Braga and Weisburd (2010) offer a deep analysis of place-based policies to crime fighting.6 In addition to new interesting insights about hot spots and crime prevention, they provide a thorough
4 See also Ehrlich (1973). 5 Durlauf, Navarro, and Rivers (2010) provide a general description of criminal choices at the individual level to under-
stand the implicit assumptions in aggregate crime regressions. They highlight the relevance of modeling the microfoun- dations of the empirical analysis of crimes.
6 See also Braga (2008), Eck et al. (2005), Felson and Clarke (1998), Sherman (1995), and Weisburd and Green (1995).
896 Natalia Lazzati and Amilcar A. Menichini
and updated overview of the theoretical and empirical research regarding this topic. Our theoreti- cal modeling assumptions are inspired by all their discussions and the literature therein. On the applied side of the literature, Fu and Wolpin (2013) perform a structural estimation of the effects of police reallocation on crime reduction. They assume that increasing the police resources in a given area does not affect the criminal activity in other areas. We show that, in our model, this happens if the interactions are negative and the outside option (e.g., working in a legal activity) does not display crowding out effects. This result provides some key lower-level restrictions under which the assumption in Fu and Wolpin (2013) holds.
The rest of the article is organized as follows. Section 2 describes our model. Section 3 presents our main findings. Section 4 evaluates the decision of an enforcement agency that aims to reduce crime by changing the attributes of a certain area. Section 5 discusses three extensions of our model. Section 6 concludes. We collect all proofs in the Appendix.
2. The Model
Main Variables
This subsection describes the main variables of our model, making a clear distinction between the features that we assume are exogenous to the incumbents (i.e., people and the enforce- ment agency) and those that are under their control. Sections 4 and 5 examine some extensions to this initial model structure.
Exogenous Variables
We let N and M represent the size of the mass of people and police, respectively. There are K alternative areas where criminal activity can take place. With only a slight abuse of notation, K represents the set as well as the number of locations. These areas differ with respect to three attrib- utes, namely, size of the area, risk of apprehension, and productivity of the criminal activity, which we describe next.
Sk refers to the geographic size of Area k (e.g., in square feet). Riskiness Rk is a probability measure of the successful apprehension of offenders in Area k. Differences in riskiness across areas capture different structural characteristics of the areas that affect the ability of police to capture offenders. For example, better street lighting may increase the risk of apprehension as offenders are more likely to be seen by someone who might call the police. Conversely, the presence of nearby highways may reduce this risk, as it becomes easier for criminals to escape. We use f to indi- cate the fee an offender must pay if apprehended. The fee f could capture, for instance, the oppor- tunity cost of time spent in prison.
Productivity Ak captures the richness of Area k in terms of expected benefits to criminals. For example, a rich area may be a neighborhood that is populated by high-income people whose houses contain high-value items. It may also be a location with stores or banks available as potential targets.
Endogenous Variables
The incumbents in the model are the people and the law enforcement agency. Specifically, people decide whether to commit a crime and, if they do so, where to perform the criminal act.
Hot Spot Policing 897
In our model, pk represents the fraction of total people N who decide to commit a crime in loca- tion k. We indicate the density of offenders in that location by dk5pkN=Sk; that is, the number of criminals in the area pkN divided by the size Sk of that area.
The enforcement agency decides how to assign police resources to the different areas. We let qk denote the proportion of total police resources M that is assigned to location k; consequently, ek5qkM=Sk is the corresponding police density (i.e., the number of police officers in the area qk
M divided by the size Sk of that area.). We assume M=Sk � 1, for all k 2 K , so that the per capita apprehension rate (defined below) lies between 0 and 1.
Payoffs of People
We model encounters between police and offenders as a random process, such that overall apprehension in location k is given by
A k; pk; qkð Þ5dkekRkSk:
That is, overall apprehension A k; pk; qkð Þ is the product of the density of criminals in the location dk, the density of police officers in the area ek, the riskiness of the location Rk, and the geographic size of the area Sk. It represents the expected number of criminals to be captured in Area k.
Furthermore, the per capita apprehension rate of an offender in location k is denoted by
P k; pk; qkð Þ5A k; pk; qkð Þ=dkSk5Rkek:
In other words, the per capita apprehension rate of an offender P k; pk; qkð Þ in location k is just the riskiness of the area Rk times the density of police resources ek in that area, and represents the probability that a criminal is captured by the police in Area k.
It follows from the last two expressions that the expected penalty for a person who commits a crime is
P k; pk; ekð Þf
Thus, the cost-side of each individual�s analysis depends on both his perceived probability of being apprehended P k; pk; ekð Þ and the penalty f he would have to pay in that case.7 (In section 5, we allow for congestion effects in the cost-side of the crime decision. These congestion effects can be motivated by the fact that a police officer cannot be in two different places at the same time; thus, as the criminal activity in a certain area increases, the probability of being apprehended in that area goes down.)
Conversely, the offender�s expected benefit of committing a crime in Area k is
Y k; pk; qkð Þ5Ak=dk:
It follows that the expected payoff of the criminal act increases with the productivity of the area Ak; by contrast, it decreases with the density of offenders in the area dk, as the total potential productivity has to be shared among more people.
7 Durlauf and Nagin (2011) suggest that increasing the perceived risk of apprehension seems to have considerable deter- rent effects on crimes.
898 Natalia Lazzati and Amilcar A. Menichini
Thus, the overall expected utility of an offender in location k is given by
U k; pk; qkð Þ5Y k; pk; qkð Þ2P k; pk; qkð Þf 5Ak=dk2Rkekf :
Recall that our model allows people not to commit a crime. This outside option can be thought of as the possibility to work in a legal activity. Under this interpretation, the number of people who opt not to commit a crime comprises the labor supply in the economy. To simplify the exposition, we initially assume the expected payoff of this outside option is 0. We relax this restric- tion in section 5 to evaluate the impact on crimes of public policies that affect the labor market in the economy. We refer to the outside option as k 5 0, so that U 0ð Þ � 0 and the choice set of each person is K0 � 0 [ K : The outcome of their decisions is a probability vector p � pkð Þk2K 2 DK0 where
DK0 � p : pk � 0 and XK
k50 pk51
n o :
Thus, p0 represents the proportion of total people N who decide not to commit a crime, and expression p0N represents the number of people who choose so (i.e., the labor supply in the economy).
Payoffs of Police Allocation Strategies
The public authority decides how to assign the police to different locations. Specifically, it chooses q � qkð Þk2K 2 DK, where qk denotes the fraction of total police M allocated to Area k and
DK � q : qk � 0 and XK
k51 qk51
n o
is the set of all possible police allocations. If the purpose of the enforcement agency is to minimize the overall level of criminal activity, then its payoff function is represented by
V pð Þ5p0 � 0
and its objective is to maximize p0 (i.e., the fraction of people who decide not to commit a crime). In our subsequent analysis, we contrast the behavior of a public authority interested in minimizing the overall crime rate to that of a public authority aiming to minimize criminality while it keeps an even distribution of crimes across areas.
Structure of the Game
We model interactions between incumbents using a leader and follower game, with the public authority as the leader and potential offenders as the followers.
In this game, the public authority first decides how to assign police to different locations with the objective of minimizing the overall crime rate. This problem can be specified as follows
maxq V pð Þ : q 2 DK� � :
Upon observing the distribution of police q, each person, taking as given the decisions of the others, decides whether to commit a crime and, in that case, where to perform the criminal act. Thus, the problem faced by each person is
Hot Spot Policing 899
maxk U k; pk; qkð Þ : k 2 K0f g:
In the next section, we solve the game using the standard backward induction principle.
3. Equilibrium Analysis
People�s Choices
The second stage of the game is itself a game among potential offenders. We use Nash equi- librium as our solution concept. Given a strategy profile p, we let b pð Þ indicate the best-response correspondence of an arbitrary person, that is,
b pð Þ5 k0 2 K0 : k0 2 arg maxk U k; pk; qkð Þf g:
It follows that p qð Þ 2 DK0 is a Nash equilibrium if, for each k0 2 K0, we obtain
pk0 qð Þ > 0 if k0 2 b pð Þ and pk0 qð Þ50 otherwise:
Given an initial police assignment and some beliefs regarding crime location, all people face the same choice set and expected payoffs. Thus, any action that is selected with a strictly positive probability will be among the options with the highest expected value. As people are indifferent across these possibilities, we can interpret p qð Þ as either an asymmet- ric equilibrium in pure strategies or a symmetric mixed strategy equilibrium (see Hugie and Dill 1994).
To simplify notation, we define hk � Skð Þ2Ak=RkMNf , for all k 2 K . We can now describe, for each police assignment q, the distribution of criminal activity across areas.
PROPOSITION 1. Fix some q 2 DK. The proportion of the population that decides to commit a crime in location k, for each k 2 K , is given by
pk qð Þ5SkAk=N u qð Þ1RkqkMf =Sk½ �;
with u qð Þ � 0. Moreover, p0 qð Þ > 0 if and only if X
k2K uk=qk < 1; in which case u qð Þ50.
The equilibrium is unique.
REMARK. u qð Þ is the utility level obtained by each person at the second-stage equi- librium when the police assignment is q. In addition, the proportion of people who opt not to commit a crime is given by p0 qð Þ512
P k2Kpk qð Þ. According to Proposition 1, p0
qð Þ > 0 if and only if P
k2K pk qð Þ5 P
k2Kuk=qk < 1. The latter condition can be re- expressed as M >
P k2K Skð Þ2Ak=RkNfqk. This means that, in our model, some people will
opt not to commit a crime if and only if the mass of police M is large enough. Under this scenario, u qð Þ50.
Proposition 1 shows that the criminal activity in a certain location pk qð Þ increases with the perceived productivity of the area Ak and decreases with both its apprehension risk Rk and the number of police officers in place qkM. This proposition allows us to determine the patterns of dis- placement of criminal activity across locations as the public authority changes the initial police allocation. We elaborate next on this description.
900 Natalia Lazzati and Amilcar A. Menichini
DISPLACEMENT. Let Q � q 2 DK : P
k2Kuk=qk < 1 � �
be the set of all possible vectors of police allocations q such that
P k2Kuk=qk < 1 (i.e., some people choose the outside
option, or p0 qð Þ > 0), and assume this set is nonempty.8 For all police distribution q 2 Q and each area k 2 K , we get that overall expected utility of an offender in location k is U k; pk qð Þ; qkð Þ50 and the fraction of people who decide to commit a crime is given by pk
qð Þ5uk=qk: That is, when the mass of police M is large enough, the outside option (not to commit a crime) regulates the second-stage equilibrium payoffs of potential offenders, and the level of criminal activity in each area pk depends only on the proportion of police assigned to that specific area qk (rather than on the whole vector of police allocation q). This means that, if qk increases, then location k becomes less attractive to potential offenders and its criminal activity pk decreases. Increasing qk in and of itself does not induce any initial displacement of criminality from Area k to the other areas. However, we do observe an increase in crime in other areas due to the removal of police from the latter. In other words, the displacement effect occurs in our model because, in order to increase the police force in Area k, the law enforcement agency has to reduce it in other areas, which then experience an increase in crime rates. This displacement mechanism changes when either the outside option displays congestion effects or there are comple- mentarities in criminal decisions. We evaluate these possibilities in sections 5, and state an important implication of these alternative specifications for the econometric analysis of the effect of police on criminal activity.
As we mentioned before, the literature on criminality defines a hot spot as an area with above-average level of crime relative to the entire space. Using our notation, this means that Area k is defined as a hot spot if pk qð ÞN=Sk > 1=Kð Þ
P k2K pk qð ÞN=Sk (i.e., its crime dens
Collepals.com Plagiarism Free Papers
Are you looking for custom essay writing service or even dissertation writing services? Just request for our write my paper service, and we'll match you with the best essay writer in your subject! With an exceptional team of professional academic experts in a wide range of subjects, we can guarantee you an unrivaled quality of custom-written papers.
Get ZERO PLAGIARISM, HUMAN WRITTEN ESSAYS
Why Hire Collepals.com writers to do your paper?
Quality- We are experienced and have access to ample research materials.
We write plagiarism Free Content
Confidential- We never share or sell your personal information to third parties.
Support-Chat with us today! We are always waiting to answer all your questions.
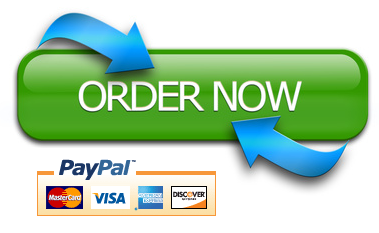