For your second assignment, you will collect quantitative data to conduct a conjoint analysis. I provide conceptual background for the conjoint analysis technique, in-depth in
For your second assignment, you will collect quantitative data to conduct a conjoint analysis. I provide conceptual background for the conjoint analysis technique, in-depth instructions, and the evaluation rubric for your assignment in class. The attachments below are necessary to read for this assignment:
Conjoint Assignment Overview Download Conjoint Assignment Overview: provides step-by step instructions for your assignment and explains the components that will be used to evaluate your submission.
In-Depth Conjoint RecordingLinks to an external site.:
https://gauchocast.hosted.panopto.com/Panopto/Pages/Viewer.aspx?id=bc0217ac-f9d8-44fe-8435-b10d014ee9fc
provides a visual guide (by me) to explain how to conduct the statistical analysis required for this assignment, including running the regression analysis, computing partworths, and computing willingness to pay (WTP).
AND THERE IS ALSO AN EXAMPLE IN THE ATTACHMENT.
READING EVERYTHING IF U CAN, ESPECIALLY THE LECTURE PPT
-
ConjointAssignmentOverview_w242.pdf
-
WhyConsumersRebelAgainstSlogansHBR.pdf
-
HowtoThinkabout_ImplicitBias_-ScientificAmerican.pdf
-
PeterandOlsonch6Attitudes.pdf
-
ConjointAssignment_w22_example.pdf
-
160dm_12-JDMII_w24.pdf
-
160dm_09-att_w24.pdf
-
Kahneman2011-Thinkingfastandslow-Chapters1to3.pdf
-
Kahneman2011-Thinkingfastandslow-Chapters1to3-1.pdf
-
160dm_11-JDMI_w24.pdf
Consumer Behavior in a Digital World, Winter 2024 Conjoint Analysis 1 Professor Hamilton
CONJOINT ANALYSIS ASSIGNMENT OVERVIEW Due: Thursday, February 15 by 11:59pm
This assignment is to be completed individually.
Deliverable:
1. A 3-4 page paper in APA format that includes a/an: a. explanation of your research methodology b. report of the major findings from your analysis (further described below)
2. An APA style appendix (in addition to the report) that includes a(n): a. matrix of features/levels with overall ratings (‘U’s), b. raw regression output (from Excel or other stats tool), c. graphical representation of the partworths you calculated, and d. table of your willingness to pay (WTP) calculations.
Overview: Conjoint analysis is a method for determining the relative importance of distinct features of a product or service for determining overall preferences.
In this assignment, you will create a conjoint choice task related to a product or service of your choosing, collect preference ratings from a friend or classmate, analyze these data using a regression, and interpret your results. Your results will yield insight into how much each feature of a product/service contributes to overall preferences, and you will use this insight to calculate your respondent’s trade-offs between levels of each feature in terms of willingness to pay (WTP). Most importantly, you will use the paper component of this assignment to communicate what your data mean and why they matter (see requirements and guidelines below). Just like the Laddering Assignment you just completed, your goal is to collect and analyze data and then interpret your data in a white paper report.
Consumer Behavior in a Digital World, Winter 2024 Conjoint Analysis 2 Professor Hamilton
Detailed instructions 1. Step One – Conduct a Survey
a. Choose a product (e.g., Garmin GPS). i. Specify four features of the product (price + three features of your choice;
e.g., price, accuracy, battery life, color), each with two levels (e.g., 10 feet vs. 32 feet).
ii. Deliverable: In your report, briefly explain why you picked your product. Then, provide a definition of the features of this product and the levels of each feature.
b. Survey someone else (not yourself). i. Ask your respondent to indicate their overall preference for a hypothetical
product with each combination of features. (Hint: Because there are 4 features with 2 levels each, there are 16 combinations. The 16 combinations are reflected in Step 2a).
ii. Here are some ways you can ask them: 1. “On a scale of 1-100, how likely are you to recommend the
[PRODUCT] featured to a friend or colleague? 2. “On a scale of 1-100, How likely or unlikely are you to purchase the
[PRODUCT]?” 3. (Hint: You can do this via survey or through an interview. But,
remember your goal is to ask them about 16 different products that each have their own unique attributes. The better you can help them visualize each product, the more reliable your results will be.)
iii. Record their preferences for all 16 combinations. iv. Transcribe their preferences to a Google sheet (or Excel) where preference
ratings are in Column A and the code for each level (e.g., 10 feet = 0 and 32 feet = 1 for Accuracy) of the four features are in Columns B-E
v. Deliverable: Include a screenshot of recorded data (similar to the image below) in Appendix A of your white paper.
Consumer Behavior in a Digital World, Winter 2024 Conjoint Analysis 3 Professor Hamilton
2. Step Two – Run a Conjoint Analysis
a. Conduct a regression predicting preferences (‘U’s) from features (‘x’s). Make sure to code the levels of your features as ‘0’s and ‘1’s. Then, select “Regression” where Y is the preference ratings column and X is the four feature columns. (I will include a demo in the lecture of the process of running your regression in Google Sheets in our lecture). i. Deliverable: Include a screenshot of your regression output (similar as below)
in Appendix B.
Consumer Behavior in a Digital World, Winter 2024 Conjoint Analysis 4 Professor Hamilton
b. Compute the partworths of each feature. i. The table below describes how to calculate partworths.
ii. Deliverable: In your report, describe the partworth of each feature and interpret the direction of the relationship between levels on preference ratings. For example, “This individual preferred a GPS with a 32 hour battery life over 12 hour battery life, which accounted for 32% of the variance in their preference ratings.”
iii. Deliverable: Show the relative importance of each feature using a bar graph or pie chart and include the illustration in Appendix C.
3. Step Three – Calculate and Interpret WTP a. Compute Willingness to Pay (WTP) for all the attributes.
i. Deliverable: In your report, explain what the trade-off for price you computed means using plain language and refer to these calculations while providing recommendations about the price trade-off of including different features.
ii. Deliverable: Include a table (like the one below) that explains your WTP calculations in Appendix D.
Feature Partworth (%) WTP Calculation WTP Price ($250 vs. $350) 43% $2.33*43 = $100.19 $100.19 Accuracy 10% $2.33*10 = $23.30 $23.30 Battery 32% $2.33*32 = $74.56 $74.56 Color 16% $2.33*16 = $37.28 $37.28
4. Step Three – Report your findings a. Report your findings in an APA style paper. Your paper must explain your research
methodology and your findings before providing an evidence-based recommendation about the design or promotion of your product. More specifically, your report must: i. Give detail about why you picked your product. Then, provide a definition of
the features of this product, and the levels of each feature (see step 1a.ii). ii. Give detail about your respondent; when presenting your results, make sure to
discuss the extent to which you think your respondent’s ‘w’s are representative of the general population, a particular segment, or perhaps just that individual. Your description of this person should inform your generalizable findings in the recommendation section.
Consumer Behavior in a Digital World, Winter 2024 Conjoint Analysis 5 Professor Hamilton
iii. Describe the partworth of each feature and interpret the direction of the relationship between levels on preference ratings (see step 2b.ii).
iv. Explain what the trade-off for price you computed means using plain language (see step 3a.i). A discussion of trade-offs can inform segmentation, new product creation, profit maximization, and so on.
v. Most importantly, talk about your results in depth. You must show that you’re making connections between the numbers produced by your analysis and what these numbers mean. If you have any odd results, discuss why they might have turned out this way.
vi. Discuss limitations of your data. Limitations could be related to the nature of the person you surveyed, the way you surveyed them, etc.
vii. Describe the ideal product to promote to the perceived “population” you sampled. For example, if you survey (i.e., sample from) a college student about their preferences of laptops, you should be careful not to generalize your insights to all people, and instead be clear to whom you believe you are generalizing your insights based on the details you provide about your respondent. Then, provide a more practical product that considers WTP tradeoffs. This product can have the same features as the ideal product, but you must rationalize why you believe including these features are worth the price they may cost. The insight you generate should be based on evidence you have aggregated about the importance of features, WTP trade-offs, or theoretical findings discussed in lecture.
Consumer Behavior in a Digital World, Winter 2024 Conjoint Analysis 6 Professor Hamilton
CONJOINT ASSIGNMENT EVALUATION CRITERIA
Your assignment will be evaluated out of 22 points, following the rubric below.
Description Level grade
Definition
1 Submission ● Was the assignment fully submitted and
on time?
0 No
1 Yes
1 Formatting and Required Elements ● Does the white paper follow the
prescribed format (i.e., 3-4 page APA format with an appendix)?
● Here is a reference: https://guides.rasmussen.edu/apa6th/abstra ct-appendix
0 No–not all formatting requirements are met.
1 Yes–all formatting requirements are met.
2 Methodology: Product details ● Does the report provide a detailed
discussion about 1) what the product/service is, 2) why the product/service was chosen, 3) definitions of the features of this product, and 4) levels of each feature of the product/service?
0 No– definitions of the features and/or levels of each feature are not described in the report.
1 Somewhat– product details are discussed in the report, but one (or more) crucial aspect is missing. This missing detail makes it harder to follow the conjoint analysis.
2 Yes– All criteria are met.
3 Methodology: Respondent details ● Does the report provide details about the
respondent and whether this respondent may (or may not) be representative of a particular segment (or segments) of the population?
0 No– details about who the respondent reflects in the population are missing.
1 Somewhat– respondent details are discussed in the report, but one (or more) crucial aspect is missing about the product details. This makes it difficult to connect these details to the
Consumer Behavior in a Digital World, Winter 2024 Conjoint Analysis 7 Professor Hamilton
limitations they report.
2 Yes– All criteria are met.
4 Conjoint: Product features + Regression ● Does the product/service reported in
Appendix A vary on four features (price + three others), with two levels for each feature?
● Does the report describe the regression analysis and include the raw regression output in Appendix B?
0 No– one (or more) crucial aspect is missing from the conjoint analysis.
1 Somewhat– it is clear a conjoint analysis was conducted, but one (or more) aspect is not reported or incorrect.
2 Yes– All criteria are met.
5 Partworths: Computation + Interpretation ● Does the report include partworth
calculations for each feature, and explain what these partworths mean with respect to the relationship between levels of that feature?
0 No– the partworth calculations are missing.
1 Somewhat– it is clear partworths were calculated, but one (or more) aspect is not reported or the calculations are incorrect.
2 Yes– All criteria are met.
Partworths: Computation + Interpretation ● Does the report include a graph (e.g., bar
graph, pie chart) of partworths in Appendix C? Give credit based on whether the illustration clarifies the weighted preference (i.e., importance) for a particular feature.
0 No– a graphical representation of partworths is missing or something about the figure is incorrect.
1 Yes– All criteria are met.
6 Trade-offs: WTP + non-monetary features ● Does the report include WTP for each
non-monetary feature and explain what these WTP calculations mean?
0 No– WTP calculations are not explained in the report
1 Somewhat– it is clear WTP was calculated for each non-monetary feature, but one (or more) aspect is not reported or the calculations are incorrect.
2 Yes– All criteria are met.
Consumer Behavior in a Digital World, Winter 2024 Conjoint Analysis 8 Professor Hamilton
Trade-offs: WTP + non-monetary features ● Does the report describe WTP
calculations in Appendix D?
0 No– WTP calculations are not reported in Appendix D or the calculations are incorrect.
1 Yes– All criteria are met.
7 Recommendation ● Does the report provide details about an
ideal product with features that are based on partworth or WTP calculations, or are in consideration of theoretical findings discussed in lecture?
● Ultimately, does the recommendation illuminate how a company might design or promote this product based on their conjoint analysis data?
0 No– the submission does not provide a recommendation for the product analyzed.
1 Somewhat– It is clear the report fully attempts to provide a recommendation, but their recommendation is not clearly connected to their conjoint data or course material.
2 Yes– All criteria are met.
8 Insight ● Did the conjoint data yield insight that
was explained in a way that felt novel and interesting?
0 No– The insights generated from the conjoint data were not interpreted in a way that yielded much insight (Hint: Usually in this case data are described, and then ignored).
1 Yes– conjoint data were interpreted in a way that yielded insight someone could use to design or promote a better product.
2 Yes (+ special recognition)– conjoint data were interpreted in a way that yielded insight someone could use to design or promote a better product AND the insight generated was probably more novel and interesting than 90% of class submissions.
9 Clarity ● Is it clear how data from the analysis
connects to the insight generated in the
0 No– it is not clear whether insight was generated from data.
Consumer Behavior in a Digital World, Winter 2024 Conjoint Analysis 9 Professor Hamilton
report? Is the insight generated from the results confusing to understand?
1 Somewhat– the insight generated was confusing to follow.
2 Yes– the insight is clear and it is easy to see how this insight was informed by data.
10 Organization ● In general, was the paper well-organized
(i.e., did the formatting contribute to a better reading experience)?
0 No– the formatting made the paper harder to read.
1 Somewhat– the paper followed basic formatting standards.
2 Yes–the formatting structure or writing organization made the paper easy to read.
,
CUSTOMERS
Why Consumers Rebel Against
Slogans by Juliano Laran, Amy N. Dalton, and Eduardo B. Andrade
FROM THE NOVEMBER 2011 ISSUE
B
Logos Can Be Tricky, Too
Bigger isn’t always better. Unsurprisingly, consumers of low-end goods favor unobtrusive logos—but so do many
rand names, logos, and slogans are integral parts of any company’s marketing
message. All have the same aim: to make consumers react positively to a product or
a business.
Our research shows, however, that many slogans backfire—for example, causing consumers
to spend money when they’re told they can save, or vice versa.
In five studies of several hundred undergraduates each, in which computers were used to
simulate shopping behavior, we found that consumers typically follow the prompt of a brand
name or a logo. After participants were exposed to brands associated with luxury (such as
Tiffany and Neiman Marcus), they decided to spend 26% more, on average, than after they
were exposed to neutral brands (such as Publix and Dillard’s). After they were exposed to
brands associated with saving money (such as Dollar Store and Kmart), they decided to spend
37% less than after they were exposed to neutral brands. The brands had the intended
“priming” effect.
But when it came to slogans, the same
participants exhibited the opposite of the
desired behavior. After reading a slogan meant
consumers of ultra-high-end goods, who prefer to send a quiet signal to others in the know.
Source: Jonah Berger and Morgan Ward, “Subtle Signals of Inconspicuous Consumption,” Journal of Consumer Research
to incite spending (“Luxury, you deserve it”),
they decided to spend 26% less than after
reading a neutral slogan (“Time is what you
make of it”). When a slogan invited them to
save (“Dress for less”), they decided to spend—
an additional 29%, on average. The slogans
had a “reverse priming” effect.
In many cases, then, brands and slogans work
at cross-purposes. For example, the name
Walmart tends to induce thriftiness, but the
company’s slogan (“Save money. Live better”) causes indulgence.
What makes slogans so different? Our studies suggest that reverse priming occurs because
consumers recognize that slogans deliberately attempt to persuade them, whereas (in their
perception) brands do not. The recognition may not be conscious: We found that consumers
automatically resisted a slogan’s message.
There’s actually good news here for marketers, who need not simply abandon slogans for fear
of adverse reactions. Slogans can exert a positive influence, we believe, if the consumer is led
to focus on something other than the effort to persuade. To test this theory, we asked one
group of participants to rate a set of slogans on the basis of intent to persuade, while a second
group rated them on creativity. The group that evaluated creativity decided to spend 58%
more than the other group. Of course, getting consumers to focus on creativity instead of
persuasion may be easier in a lab setting than in real-world marketing.
More research is needed to understand why consumers perceive certain tactics as efforts to
persuade. In the meantime, marketers should be aware that messages seen even
subconsciously as manipulative can cause significant backlash.
A version of this article appeared in the November 2011 issue of Harvard Business Review.
,
B E H A V I O R & S O C I E T Y
How to Think about "Implicit Bias"
Amidst a controversy, it’s important to remember that implicit bias is real—and it matters
By Keith Payne, Laura Niemi, John M. Doris on March 27, 2018
Credit: theprint Getty Images
When is the last time a stereotype popped into your mind? If you are like most
people, the authors included, it happens all the time. That doesn’t make you a racist,
sexist, or whatever-ist. It just means your brain is working properly, noticing
patterns, and making generalizations. But the same thought processes that make
people smart can also make them biased. This tendency for stereotype-confirming
thoughts to pass spontaneously through our minds is what psychologists call implicit
bias. It sets people up to overgeneralize, sometimes leading to discrimination even
when people feel they are being fair.
Studies of implicit bias have recently drawn ire from both right and left. For the right,
talk of implicit bias is just another instance of progressives seeing injustice under
every bush. For the left, implicit bias diverts attention from more damaging instances
of explicitbigotry. Debates have become heated, and leapt from scientific journals to
the popular press. Along the way, some important points have been lost. We highlight
two misunderstandings that anyone who wants to understand implicit bias should
know about.
First, much of the controversy centers on the most famous implicit bias test, the
Implicit Association Test (IAT). A majority of people taking this test show evidence of
implicit bias, suggesting that most people are implicitly biased even if they do not
think of themselves as prejudiced. Like any measure, the test does have limitations.
The stability of the test is low, meaning that if you take the same test a few weeks
apart, you might score very differently. And the correlation between a person’s IAT
scores and discriminatory behavior is often small.
The IAT is a measure, and it doesn’t follow from a particular measure being flawed
that the phenomenon we’re attempting to measure is not real. Drawing that
conclusion is to commit the Divining Rod Fallacy: just because a rod doesn’t find
water doesn’t mean there’s no such thing as water. A smarter move is to ask, “What
does the other evidence show?”
In fact, there is lots of other evidence. There are perceptual illusions, for example, in
which white subjects perceive black faces as angrier than white faces with the same
expression. Race can bias people to see harmless objects as weapons when they are in
the hands of black men, and to dislike abstract images that are paired with black
faces. And there are dozens of variants of laboratory tasks finding that most
participants are faster to identify bad words paired with black faces than white faces.
None of these measures is without limitations, but they show the same pattern of
reliable bias as the IAT. There is a mountain of evidence—independent of any single
test—that implicit bias is real.
The second misunderstanding is about what scientists mean when they say a measure
predicts behavior. It is frequently complained that an individual’s IAT score doesn’t
tell you whether they will discriminate on a particular occasion. This is to commit the
Palm Reading Fallacy: unlike palm readers, research psychologists aren’t usually in
the business of telling you, as an individual, what your life holds in store. Most
measures in psychology, from aptitude tests to personality scales, are useful for
predicting how groups will respond on average, not forecasting how particular
individuals will behave.
The difference is crucial. Knowing that an employee scored high on conscientiousness
won’t tell you much about whether her work will be careful or sloppy if you inspect it
right now. But if a large company hires hundreds of employees who are all
conscientious, this will likely pay off with a small but consistent increase in careful
work on average.
Implicit bias researchers have always warned against using the tests for predicting
individual outcomes, like how a particular manager will behave in job interviews—
they’ve never been in the palm-reading business. What the IAT does, and does well, is
predict average outcomes across larger entities like counties, cities, or states. For
example, metro areas with greater average implicit bias have larger racial disparities
in police shootings. And counties with greater average implicit bias have larger racial
disparities in infant health problems. These correlations are important: the lives of
black citizens and newborn black babies depend on them.
Field experiments demonstrate that real-world discrimination continues, and is
widespread. White applicants get about 50 percent more call-backs than black
applicants with the same resumes; college professors are 26 percent more likely to
respond to a student’s email when it is signed by Brad rather than Lamar; and
physicians recommend less pain medication for black patients than white patients
with the same injury.
Today, managers are unlikely to announce that white job applicants should be chosen
over black applicants, and physicians don’t declare that black people feel less pain
than whites. Yet, the widespread pattern of discrimination and disparities seen in
field studies persists. It bears a much closer resemblance to the widespread
stereotypical thoughts seen on implicit tests than to the survey studies in which most
people present themselves as unbiased.
One reason people on both the right and the left are skeptical of implicit bias might be
pretty simple: it isn’t nice to think we aren’t very nice. It would be comforting to
conclude, when we don’t consciously entertain impure intentions, that all of our
intentions are pure. Unfortunately, we can’t conclude that: many of us are more
biased than we realize. And that is an important cause of injustice—whether you know
it or not.
ABOUT THE AUTHOR(S)
Keith Payne
Keith Payne is a Professor of Psychology and Neuroscience at UNC Chapel Hill. He studies
implicit bias and the psychological effects of inequality.
Laura Niemi
Laura Niemi is a Postdoctoral Fellow in the Department of Philosophy and the Center for
Cognitive Neuroscience at Duke University and an Affiliate of the Department of Psychology at
Harvard University. She studies moral judgment and the implications of differences in moral
values.
John M. Doris
John M. Doris is Professor in the Philosophy–Neuroscience–Psychology Program and
Philosophy Department, Washington University in St. Louis. He works at the intersection of
cognitive science, moral psychology, and philosophical ethics.
,
126
The Gap began in 1969 with a single
store in San Francisco selling jeans
and records. Fueled by heavy adver-
tising, The Gap grew rapidly in the 1970s to
200 stores. In the process, The Gap became
the epitome of “cool” by offering basic items
such as T-shirts and jeans t hat looked like
designer clothing, but without the arrogance.
Although the company experienced a few
bumps along the way, growth continued
through the 1980s and most of the
1990s.
By 2000 there were 1,800
stores in Europe, North
America, and Japan, includ-
ing new stores such as Gap-
Collepals.com Plagiarism Free Papers
Are you looking for custom essay writing service or even dissertation writing services? Just request for our write my paper service, and we'll match you with the best essay writer in your subject! With an exceptional team of professional academic experts in a wide range of subjects, we can guarantee you an unrivaled quality of custom-written papers.
Get ZERO PLAGIARISM, HUMAN WRITTEN ESSAYS
Why Hire Collepals.com writers to do your paper?
Quality- We are experienced and have access to ample research materials.
We write plagiarism Free Content
Confidential- We never share or sell your personal information to third parties.
Support-Chat with us today! We are always waiting to answer all your questions.
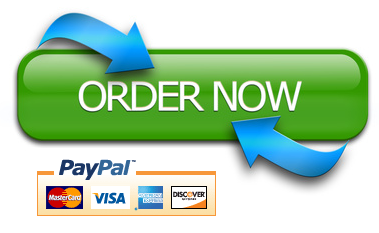