Explain when a z-test would be appropriate over a t-test
HLT 362 Topic 3 DQ 1
Explain when a z-test would be appropriate over a t-test.
ADDITIONAL DETAILS
Explain when a z-test would be appropriate over a t-test
Introduction
If you’re interested in testing whether two populations have the same mean, a t-test is your go-to option. If you want to compare the standard deviations of two different populations, the z-test can be used. When it comes down to it, however, both methods help us determine if our results are significant or not. But what happens when we have data that doesn’t follow a normal distribution? In this article I’ll explain when each method is appropriate for measuring statistical significance and how these methods differ from each other as well as their respective advantages and disadvantages.
The t-test and z-test are statistical methods developed to measure the level of significance.
The t-test and z-test are statistical methods developed to measure the level of significance. The t-test is used to determine whether a sample is significantly different from the population mean, while the z-test is used for determining whether two samples are statistically independent.
The t-test and z-test can be used as complementary tools in your research study if you want to compare two groups with unequal variances (i.e., when one group has large variance), but they do not always yield similar results when applied together; however this doesn’t mean that there aren’t any benefits from using both tests at once!
A z-test is used when you know the standard deviation (sigma) of the entire population.
A z-test is used when you know the standard deviation (sigma) of the entire population. The z-test was first developed by Karl Pearson in 1894, who was trying to find out whether his hypothesis “the mean is constant” held up, or whether there was some change in slope over time. This result would be important for understanding trends in data and predicting future outcomes based on past performance.
The general form of an hypothesis test requires three things: a null hypothesis (H0), an alternative hypothesis (H1), and a decision rule that tells us how we should choose between them if we reject H0 at some point during our analysis process (and this might include checking out different significance levels). In this case, we have chosen to use two different significance levels: 0% and 5%. If either value falls within these limits then we say our results are significant; otherwise they aren’t statistically significant enough yet so we can’t tell which way things went!
A z-test is used when the sample size is greater than 30.
A z-test is used when the sample size is greater than 30. In other words, if you have a small sample size and your population standard deviation is unknown, then you should use a t-test.
A T-Test:
-
Sample Size Must Be Smaller Than 30
A t-test is used if the sample size is less than 30.
A t-test is used if the sample size is less than 30. This is because you don’t know the standard deviation of your entire population and need to test whether or not there are differences between groups.
A t-test can be used when data does not follow a normal distribution, but it’s never used on its own without knowing some other information first (e.g., sample size).
A t-test is used when the standard deviation of the entire population is not known.
A t-test is used when the standard deviation of the entire population is not known. For example, suppose you have a sample of 100 people and want to find out if their weight varies from person to person. You could calculate their average weight by adding all 100 weights together and dividing by 100, which would be an estimate of their mean (average) weight.
But what about those who weigh less than average? Do they have higher or lower weights than others in your sample? If so, then your sample distribution might not be normal—that means it won’t look like a bell curve with just two peaks at its ends (like this one). In fact, if there were only two peaks instead of three or four, then we’d know that our data were definitely non-normal because our distributions don’t fit into any known normal distribution curve! So now what?
When your data follows a normal distribution, you can use either a t-test or a z-test.
The t-test and z-test are both statistical methods that measure the level of significance. They’re used to compare samples with different degrees of variability, but they have slightly different assumptions about how samples are distributed.
The t-test is used when your data follows a normal distribution, which means it has an average value (the mean) and standard deviation around that mean. In other words, if you had data points with an average height (say 5 feet), 2 inches high on either side of the average and 4 inches tall at the extremes, then this would be a normal distribution because each point falls in between those two extremes—in theory anyway!
The z-test works when your data doesn’t follow a typical bell curve shape like most statistical tests do; instead it’s very skewed toward either extreme end of what would be considered normal for our purposes here: low values or high values (more specifically very small minima or maxima).
There are times when it makes sense to use a z-test and other times when it makes sense to use a t-test.
There are times when it makes sense to use a z-test and other times when it makes sense to use a t-test. When you have a large sample size, then the z-test is preferable. For example, if your sample size is 30 or more and you want to test whether two populations have equal means but unequal variances (variation), then using the appropriate test for this situation would be using one of these tests:
-
ANOVA (one way ANOVA) – This type of analysis uses both an F test with df=n-1 degrees of freedom and an F ratio with df=n degrees of freedom so that there will be enough information in both cases. It’s similar in concept but different from what we’ll call “ANOVAs” here because they don’t always involve comparing means between groups; instead they compare groups against each other directly rather than only looking at differences between groups themselves across time points/conditions etc… So even though there might seem like many similarities between these two approaches, please remember that they’re still different!
Conclusion
As you can see, there are times when it makes sense to use a t-test and other times when it makes sense to use a z-test. The main thing is to understand which test is best suited for your data, and then stick with it!
Collepals.com Plagiarism Free Papers
Are you looking for custom essay writing service or even dissertation writing services? Just request for our write my paper service, and we'll match you with the best essay writer in your subject! With an exceptional team of professional academic experts in a wide range of subjects, we can guarantee you an unrivaled quality of custom-written papers.
Why Hire Collepals.com writers to do your paper?
Quality- We are experienced and have access to ample research materials.
We write plagiarism Free Content
Confidential- We never share or sell your personal information to third parties.
Support-Chat with us today! We are always waiting to answer all your questions.
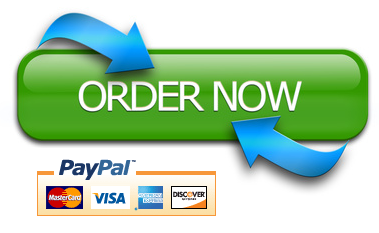