After your study of Chapter 4, what is the most important aspect of sampling in research? After your study of Chapter 5, provide a current event/news story that research parti
Minimum Requirements: Your post length should be between 250-400 words All post should reflect critical thinking, and quality writing – be polite and respectful in all posts.
After your study of Chapter 4, what is the most important aspect of sampling in research?
After your study of Chapter 5, provide a current event/news story that research participants is a key element in measurement.
4
Sampling
© 2016 Cengage Learning. All Rights Reserved.
1
Sampling is the process of selecting units from a population of interest
Most often people, groups, and organizations, but sometimes texts like diaries, Internet discussion boards and blogs, or even graphic images
By studying the sample, we can generalize results to the population from which the units were chosen
4.1 Foundations of Sampling
© 2016 Cengage Learning. All Rights Reserved.
2
4.2 Sampling Terminology
© 2016 Cengage Learning. All Rights Reserved.
Figure 4.3 The different groups in the sampling model.
Population: The group you want to generalize to and the group you sample from in a study.
Theoretical population: A group which, ideally, you would like to sample from. This is usually contrasted with the accessible population.
Accessible population: A group you can get access to when sampling. This is usually contrasted with the theoretical population.
Sampling frame: The list from which you draw your sample. In some cases, there is no list; you draw your sample based upon an explicit rule. For instance, when doing quota sampling of passers-by at the local mall, you do not have a list per se, and the sampling frame is the population of people who pass by within the time frame of your study and the rule(s) you use to decide whom to select.
Sample: The actual units you select to participate in your study.
3
Accessible population
A group you can get access to when sampling, usually contrasted with the theoretical population
Bias
A systematic error in an estimate
Can be the result of any factor that leads to an incorrect estimate, and can lead to a result that does not represent the true value in the population
4.2 Sampling Terminology (cont’d.)
© 2016 Cengage Learning. All Rights Reserved.
Generalizing
The process of making an inference that the results observed in a sample would hold in the population of interest – if such an inference or conclusion is valid we can say that it has generalizability
External validity
The degree to which the conclusions in your study would hold for other persons in other places and at other times
4.3 External Validity
© 2016 Cengage Learning. All Rights Reserved.
5
4.3a Two Major Approaches to External Validity in Sampling: The Sampling Model
© 2016 Cengage Learning. All Rights Reserved.
Figure 4.4 The sampling model for external validity. The researcher draws a sample for a study from a defined population to generalize the results to the population.
Sampling model: A model for generalizing in which you identify your population, draw a fair sample, conduct your research, and finally generalize your results to other populations groups.
6
4.3a Two Major Approaches to External Validity: The Proximal Similarity Model
© 2016 Cengage Learning. All Rights Reserved.
Figure 4.5 The proximal similarity model for external validity.
Proximal Similarity Model: A model for generalizing from your study to other contexts based upon the degree to which the other context is similar to your study context.
Gradient of similarity The dimension along which your study context can be related to other potential contexts to which you might wish to generalize. Contexts that are closer to yours along the gradient of similarity of place, time, people, and so on can be generalized to with more confidence that ones that are further away.
7
Nonprobability sampling
Sampling that does not involve random selection
Probability sampling
Sampling that does involve random selection
4.4 Sampling Methods
© 2016 Cengage Learning. All Rights Reserved.
Does not involve random selection
Random selection is a process or procedure that assures that the different units in your population are selected by chance
Two kinds of nonprobability sampling
Accidental
Purposive
4.5 Nonprobability Sampling
© 2016 Cengage Learning. All Rights Reserved.
Sampling by asking for volunteers
Sampling by using available participants, such as college students
Sampling by interviewing people on the streets
The problem: you do not know if your sample represents the population
4.5a Accidental, Haphazard, or Convenience Sampling
© 2016 Cengage Learning. All Rights Reserved.
Several types:
4.5c Modal Instance Sampling
4.5d Expert Sampling: Validity
4.5e Quota Sampling
Proportional Quota Sampling
Nonproportional Quota Sampling
4.5f Heterogeneity Sampling
4.5g Snowball Sampling:
Respondent Driven Sampling
4.5b Purposive Sampling
© 2016 Cengage Learning. All Rights Reserved.
Modal instance sampling: Sampling for the most typical case.
Expert sampling: A sample of people with known or demonstrable experience and expertise in some area.
Validity: The best available approximation of the truth of a given proposition, inference, or conclusion
Quota sampling: Any sampling method where you sample until you achieve a specific number of sampled units for each subgroup of a population.
Proportional quota sampling: A sampling method where you sample until you achieve a specific number of sampled units for each subgroup of a population, where the proportions in each group are the same.
Nonproportional quota sampling: A sampling method where you sample until you achieve a specific number of sampled units for each subgroup of a population, where the proportions in each group are not the same.
Heterogeneity sampling: Sampling for diversity or variety.
Snowball sampling: A sampling method in which you sample participants based upon referral from prior participants.
Respondent Driven Sampling: RDS combines a modified form of chain referral, or snowball, sampling, with a mathematical system for weighting the sample to compensate for its not having been drawn as a simple random sample
11
4.6a The Sampling Distribution – Statistical Terms
© 2016 Cengage Learning. All Rights Reserved.
Figure 4.11 Statistical terms in sampling
Response: A specific measurement value that a sampling unit supplies.
Statistic: A value that is estimated from data
Population parameter: The mean or average you would obtain if you were able to sample the entire population.
12
4.6a The Sampling Distribution – A Theoretical Distribution
© 2016 Cengage Learning. All Rights Reserved.
Figure 4.12 The sampling distribution
Sampling distribution: The theoretical distribution of an infinite number of samples of the population of interest in your study.
13
Standard Deviation
Standard Error
Sampling Error
4.6b Sampling Error
© 2016 Cengage Learning. All Rights Reserved.
Standard deviation: The square root of the variance. The standard deviation and variance both measure dispersion, but because the standard deviation is measured in the same units as the original measure and the variance is measured in squared units, the standard deviation is usually the more
directly interpretable and meaningful.
Standard error: The spread of the averages around the average of averages in a sampling distribution.
Sampling error: Error in measurement associated with sampling.
14
4.6c The Normal Curve In Sampling
© 2016 Cengage Learning. All Rights Reserved.
Figure 4.13 The 68, 95, 99 Percent Rule
Normal curve: A common type of distribution where the values of a variable have a bell-shaped histogram or frequency distribution. In a normal distribution, approximately 68 percent of cases occur within one standard deviation of the mean or center, 95 percent of the cases fall within two standard deviations, and 99 percent are within three standard deviations.
We call these intervals the 68, 95, and 99 percent confidence intervals.
15
4.7a Definitions:
N is the number of cases in the sampling frame
n is the number of cases in the sample
NCn is the number of combinations (subsets) of n from N
f = n/N is the sampling fraction
4.7 Probability Sampling: Procedures
© 2016 Cengage Learning. All Rights Reserved.
4.7b Simple Random Sampling
© 2016 Cengage Learning. All Rights Reserved.
Figure 4.15 Simple random sampling.
Simple random sampling: A method of sampling that involves drawing a sample from a population so that every possible sample has an equal probability of being selected.
17
4.7c Stratified Random Sampling
© 2016 Cengage Learning. All Rights Reserved.
Figure 4.16 Stratified random sampling.
Stratified Random Sampling: A method of sampling that involves dividing your population into homogeneous subgroups and then taking a simple random sample in each subgroup.
18
4.7d Systematic Random Sampling
© 2016 Cengage Learning. All Rights Reserved.
Figure 4.17 Systematic random sampling
Systematic random sampling: A sampling method where you determine randomly where you want to start selecting in the sampling frame and then follow a rule to select every xth element in the sampling frame list (where the ordering of the list is assumed to be random).
19
4.7e Cluster (Area) Random Sampling
© 2016 Cengage Learning. All Rights Reserved.
Figure 4.19 A county level map of New York state used for cluster (area) random sampling
Cluster random sampling: A sampling method that involves dividing the population into groups called clusters, randomly selecting clusters, and then sampling each element in the selected clusters. This method is useful when sampling a population that is spread across a wide area geographically.
20
The combining of several sampling techniques to create a more efficient or effective sample than the use of any one sampling type can achieve on its own
4.7f Multistage Sampling
© 2016 Cengage Learning. All Rights Reserved.
21
Bigger is better, as it increases the confidence in results
However, this has to be balanced with cost and time considerations
Sample size is also determined by the sampling technique used
4.7g How Big Should the Sample Be?
© 2016 Cengage Learning. All Rights Reserved.
Can the results be generalized to other people?
Can the results be generalized to other places?
Can the results be generalized to other time periods?
4.8 Threats to External Validity
© 2016 Cengage Learning. All Rights Reserved.
Do a good job of drawing a sample from a population
Random selection is always better
Use proximal similarity effectively
Replication
When a study is repeated with a different group of people, in a different place, at a different time, and the results of the study are similar, external validity is increased
4.9 Improving External Validity
© 2016 Cengage Learning. All Rights Reserved.
What does the term population really mean? What are some examples of populations?
What are the advantages and disadvantages of each sampling technique in this chapter, and when would you choose one technique over another?
What is external validity, and what is the best way to strengthen it?
Discuss and Debate
© 2016 Cengage Learning. All Rights Reserved.
Often, students hear the word population, and automatically assume that it must be something very large, like the entire population of the United States. In reality, a population can be any group of people in a given setting. For example, everyone who works for a given company can be a population. All students enrolled in a given university can be a population. Student responses will vary, but the idea is to have them to see that populations do not have to be large groups of people.
Responses will vary.
External validity is the ability of the researcher to generalize the results of a study back to the population from which it was drawn. The best way of strengthening external validity is through replication.
25
image1.emf
image2.emf
image3.png
image4.png
image5.png
image6.png
image7.png
image8.png
image9.png
image10.png
image11.png
image12.png
image13.png
,
5
Introduction to Measurement
© 2016 Cengage Learning. All Rights Reserved.
1
Research topics are often abstract
These topics must be translated into measurable constructs
No measurement is perfect
Example: your weight may be different on even the same scale!
5.1 Foundations of Measurement
© 2016 Cengage Learning. All Rights Reserved.
2
5.1a Levels of Measurement
© 2016 Cengage Learning. All Rights Reserved.
Figure 5.3 Relationship between attributes and values in a measure.
For nominal data, such as party affiliation, we need to assign values (codes) to each category, so we can analyze the data numerically.
3
5.1a The Hierarchy of Levels of Measurement
© 2016 Cengage Learning. All Rights Reserved.
Figure 5.4 The hierarchy of levels of measurement.
Nominal Level of Measurement: Measuring a variable by assigning a number arbitrarily in order to name it numerically so that it might be distinguished from other objects. The jersey numbers in most sports are measured at a nominal level.
Ordinal Level of Measurement: Measuring a variable using rankings. Class rank is a variable measured at an ordinal level.
Interval Level of Measurement: Measuring a variable on a scale where the distance between numbers is interpretable. For instance, temperature in Fahrenheit or Celsius is measured on an interval level.
Ratio Level of Measurement: Measuring a variable on a scale where the distance between numbers is interpretable and there is an absolute zero value. For example, weight is a ratio measurement.
4
Two key concepts:
Reliability
Validity
5.2 Quality of Measurement
© 2016 Cengage Learning. All Rights Reserved.
True score theory
Measurement error
Random error
Systematic error
Pay attention to potential bias
5.2a Reliability
© 2016 Cengage Learning. All Rights Reserved.
True score theory: maintains that every observable score is the sum of two components: true ability (or the true level) of the respondent on that measure; and random error. The true score is essentially the score that a person would have received if the score were perfectly accurate.
Random error: A component or part of the value of a measure that varies entirely by chance. Random error adds noise to a measure and obscures the true value.
Bias: A systematic error in an estimate. A bias can be the result of any factor that leads to an incorrect estimate. In measurement a bias can either be systematic and consistent or random. In either case, when bias exists, the values that are measured do not accurately reflect the true value.
6
Pilot test instruments
When using observers as data collectors, make sure they are trained
Double check the data
Use statistical procedures to adjust for measurement error
Triangulate: combine multiple measurements
5.2a How to Reduce Measurement Error
© 2016 Cengage Learning. All Rights Reserved.
Reliable means a measurement is repeatable and consistent
Reliability is a ratio:
The proportion of truth in your observation
Determined using a group of individuals, not a single observation
Variance and standard deviation
The higher the correlation, the more reliable the measure
5.2b Theories of Reliability
© 2016 Cengage Learning. All Rights Reserved.
Inter-rater or inter-observer reliability
Cohen’s Kappa
5.2c Types of Reliability: Inter-rater
© 2016 Cengage Learning. All Rights Reserved.
Figure 5.17 If only there were consensus!
Inter-rater or inter-observer reliability is used to assess the degree to which different raters/observers give consistent estimates of the same phenomenon.
Courtesy of Robert Michael/Corbis
Cohen’s kappa: A statistical estimate of inter-rater agreement or reliability that is more robust than percent agreement because it adjusts for the probability that some agreement is due to random chance.
9
5.2c Types of Reliability: Test-Retest
© 2016 Cengage Learning. All Rights Reserved.
Figure 5.19 Test-retest reliability.
You estimate test-retest reliability when you administer the same test to the same (or a similar) sample on two different occasions
10
5.2c Types of Reliability: Parallel-Forms Reliability
© 2016 Cengage Learning. All Rights Reserved.
Figure 5.20 Parallel-forms reliability.
Two forms of the instrument are created. The correlation between the two parallel forms is the estimate of reliability.
11
Average inter-item correlation
Average item-total correlation
Split-half reliability
Cronbach’s alpha (α)
5.2c Types of Reliability: Internal Consistency
© 2016 Cengage Learning. All Rights Reserved.
Average Inter-Item Correlation: The average inter-item correlation uses all of the items on your instrument that are designed to measure the same construct. You first compute the correlation between each pair of items.
Average Item-Total Correlation: This approach also uses the inter-item correlations. In addition, you compute a total score for the items and treat that in the analysis like an additional variable.
Split-Half Reliability: In split-half reliability, you randomly divide into two sets all items that measure the same construct. You administer the entire instrument to a sample and calculate the total score for each randomly divided half of the measure. The split-half reliability estimate is simply the correlation between these two total scores.
Cronbach’s Alpha: One specific method of estimating the reliability of a measure. Although not calculated in this manner, Cronbach’s Alpha can be thought of as analogous to the average of all possible split-half correlations.
12
Construct validity
Operationalization
The act of translating a construct into its manifestation, for example, translating the idea of your treatment or program into the actual program, or translating the idea of what you want to measure into the real measure
The result is also referred to as an operationalization, that is, you might describe your actual program as an operationalized program
5.2d Validity
© 2016 Cengage Learning. All Rights Reserved.
Translation validity
Face validity
Content validity
Criterion-related validity
Predictive validity
Concurrent validity
Convergent validity
Discriminant validity
5.2e Construct Validity and Other Measurement Validity Labels
© 2016 Cengage Learning. All Rights Reserved.
Translation validity: A type of construct validity related to how well you translated the idea of your measure into its operationalization.
Face validity: A validity that checks that “on its face” the operationalization seems like a good translation of the construct.
Content validity: A check of the operationalization against the relevant content domain for the construct.
Criterion-related validity: The validation of a measure based on its relationship to another independent measure as predicted by your theory of how the measures should behave.
Predictive validity: A type of construct validity based on the idea that your measure is able to predict what it theoretically should be able to predict.
Concurrent validity: An operationalization’s ability to distinguish between groups that it should theoretically be able to distinguish between.
Convergent validity: the degree to which the operationalization is similar to (converges on) other operationalizations to which it theoretically should be similar.
Discriminant validity: The degree to which concepts that should not be related theoretically are, in fact, not interrelated in reality.
14
5.2e Construct Validity; Putting it Together
© 2016 Cengage Learning. All Rights Reserved.
Figure 5.29 Convergent and discriminant validity correlations in a single table or correlation matrix
15
Inadequate preoperational explication of constructs
Mono-operation and mono-method bias
Interaction of different treatments, or of testing and treatment
Restricted generalizability across constructs
Confounding constructs and levels of constructs
5.2f Threats to Construct Validity
© 2016 Cengage Learning. All Rights Reserved.
Inadequate Preoperational Explication of Constructs: failing to define what you meant by the construct before you tried to translate it into a measure or program.
Mono-operation bias: A threat to construct validity that occurs when you rely on only a single implementation of your independent variable, cause, program,
or treatment in your study.
Mono-method bias: A threat to construct validity that occurs because you use only a single method of measurement.
Interaction of different treatments: When participants are involved in more than one course of treatment, you cannot be sure it was your treatment that caused a change.
Interaction of testing and treatment: Sometimes, the act of being tested itself can cause participants performance to change on a given construct.
Restricted generalizability across constructs: Is spending money on fun things the same as spending money on bills? The two constructs are different, and therefore generalizing is restricted.
Confounding constructs and levels: Is spending $5 the same as spending $500? The five dollar and five hundred dollar categories are levels of the construct “spending.” A different level may have a greater or lesser effect on the dependent variable.
16
Hypothesis guessing
Evaluation apprehension
Researcher expectations
5.2g The Social Threats to Construct Validity
© 2016 Cengage Learning. All Rights Reserved.
Hypothesis guessing: A threat to construct validity and a source of bias in which participants in a study guess the purpose of the study and adjust their
responses based on that.
Collepals.com Plagiarism Free Papers
Are you looking for custom essay writing service or even dissertation writing services? Just request for our write my paper service, and we'll match you with the best essay writer in your subject! With an exceptional team of professional academic experts in a wide range of subjects, we can guarantee you an unrivaled quality of custom-written papers.
Get ZERO PLAGIARISM, HUMAN WRITTEN ESSAYS
Why Hire Collepals.com writers to do your paper?
Quality- We are experienced and have access to ample research materials.
We write plagiarism Free Content
Confidential- We never share or sell your personal information to third parties.
Support-Chat with us today! We are always waiting to answer all your questions.
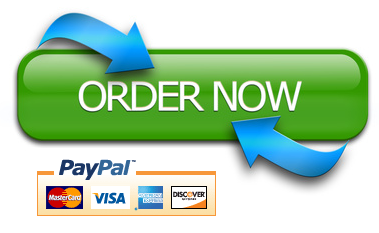
All Rights Reserved Terms and Conditions
College pals.com Privacy Policy 2010-2018