I need someone to my thesis work. It is of finance.? ?? The topic is Predicting the Risk and Return of Indian Sectoral Indices Using Econometric and Machine Learning
I need someone to my thesis work. It is of finance.
The topic is Predicting the Risk and Return of Indian Sectoral Indices Using Econometric and Machine Learning Models. I have attached my Research Proposal. I also attached the dissertation guidelines. It is of 8000 words
I also attached one image which says what’s needs to do. You need to compare the data of stock market of India and China for the past 15 years from 2007-2022 and form models such as GARCH AND CAPM and perform test such as Dickeyfuller, Phillips curve, etc.
Research Proposal and Literature Review
Predicting the Risk and Return of Indian Sectoral Indices Using Econometric and Machine Learning Models
Table of Contents
Abstract 2 Introduction 2 Background to the study 3 The literature review 4 Methodology and analysis 6 Conclusion 7 References 7
Abstract
This research aims to develop a predictive model for the risk and return of Indian sectoral indices using a combination of econometric and machine learning techniques. The study will focus on technology, healthcare, retail, manufacturing, and finance, which are significant contributors to India's GDP.
The study will use a variety of econometric models, including GARCH, VAR, and ARIMA, as well as machine learning algorithms such as gradient boosting, random forests, and neural networks. By combining these approaches, the study aims to identify the factors that influence the risk and return of various sector indices and develop a more effective strategy for predicting their volatility and profitability.
The significance of this research lies in its potential to provide valuable insights for investors, policymakers, and the Indian economy as a whole. By accurately predicting the performance of sectoral indices, investors can make more informed decisions about where to invest their capital. The study's findings may also have broader implications for understanding the complex dynamics of the Indian economy.
In conclusion, this research represents an important step towards developing a more comprehensive understanding of the risk and return of Indian sectoral indices. By integrating econometric and machine learning approaches, this study aims to provide a powerful tool for predicting the performance of these indices and unlocking their full potential for investors and policymakers alike.
1. Introduction
This research aims to develop a method for quantifying the risk and return of Indian sectoral indexes by using econometric and machine learning models. The research will focus on the sectors of financial services, transportation, manufacturing, retail,
( 2 RESEARCH PROPOSAL AND LITERATURE REVIEW )
( RESEARCH PROPOSAL AND LITERATURE REVIEW 3 )
healthcare, and utilities. The research will use a dataset of historical market data from 2011 to 2020. Machine learning models will be used to develop a predictive model for risk and return. Econometric models will be used to identify the factors that influence risk and return.
Previous research has shown that a variety of factors can influence the risk and return of stock markets. These factors include macroeconomic variables, such as GDP growth and inflation, as well as industry-specific factors, such as the level of competition and the regulatory environment.
Previous research on the factors that influence the risk and return of Indian sectoral indices has been limited. This research will address this gap by using a larger dataset and a more comprehensive set of econometric and machine learning models.
2. Background to the study
The market has proliferated due to Indian investors' increased interest in stock markets. Thus, the risk and return potential of various Indian stock market segments must be assessed. Beloskar & Rao states that sectoral indexes give investors, policymakers, and researchers a complete economic picture (2022). Each business in India has pros and cons; hence sectoral indexes can help investors evaluate firms as the Indian stock market grows. This article covers technology, healthcare, retail, manufacturing, and finance. These companies were chosen because they contribute significantly to India's GDP, as stock investing seeks high returns with low risk. The stock market is a complicated, challenging success; its volatility affects risk-reward. Investors must predict future volatility since stock market volatility influences risk.
Econometric methods can calculate stock market risk and return as forecasts often use the Autoregressive Integrated Moving Average (ARIMA) model. Below is a table showing the evolution of the number of listed stocks and the capitalization of the stock market in India as per showing the development of the number of listed stores and the capitalization of the stock market in India as per Beloskar & Rao (2022).
YEAR |
Market Capitalization |
No. of listed companies |
2011 |
240000 |
350 |
2012 |
260500 |
400 |
2013 |
280000 |
420 |
2014 |
290000 |
460 |
2015 |
250000 |
495 |
2016 |
245000 |
485 |
2017 |
330000 |
470 |
2018 |
260000 |
460 |
2019 |
250000 |
460 |
2020 |
230000 |
430 |
Table 1: shows the evolution of the number of listed stocks and the capitalization of the stock market in India.
Time series models, such as the ARIMA model, can predict the future value of a variable based on its historical importance. Economists frequently employ the generalized autoregressive conditional heteroskedasticity (GARCH) model to investigate the tendency of market instability to cluster (Beloskar & Rao, 2022). The Vector Autoregression (VAR) model, which is based on multiple time series, assists in predicting the future direction of industry indices. Increasingly, machine learning systems are utilized to forecast price fluctuations and the growth in the value of various financial assets. Numerous machine learning techniques, such as Random Forest, Gradient Boosting, and Neural Networks, are utilized for economic forecasting. These models can display nonlinear interactions between factors and operate on extensive datasets.
( The number of listed stocks against the capitalization of the stock market YEAR Market Capitalization No. of listed companies 350000 300000 250000 200000 150000 100000 50000 0 1 2 3 4 5 6 7 8 9 10 )Last but not least, due to the volatility of India's financial markets, investors and government officials must have complete knowledge of the risk and return of Indian industry indexes. This study aims to contribute by devising a method to estimate the risk and return of Indian industry indexes using a combination of econometric and machine learning models. This study could benefit investors if it helps them comprehend the risk and return dynamics of Indian sectoral indices.
Figure 1: The evolution of the number of listed stocks and the capitalization of the stock market in India.
3. The literature review
Sen argues that forecasting stock prices is difficult and complex because the time series of these factors vary in unpredictable ways (2022). This study examines the Bombay Stock Exchange's (BSE) time series statistics for the Indian healthcare sector. We examined the BSE S&P healthcare sector index monthly from January 2010 until
November 2021. The time series is divided according to the trend, the seasons, and what can be anticipated. Before and after the outbreak, the values of the various components provide insight into the business's most vital aspects. Five of the models of forecasting that we examine employ exponential smoothing and the autoregressive integrated moving average. Using data from January 2010 to December 2020, these models forecast the monthly index numbers for 2021. There is an abundance of supporting evidence for the beliefs.
Padhi et al. (20220) state that investors can make improved decisions when credible stock market models are in place. Using a trading model, investors can select the most profitable companies and reduce their risk of financial loss. Because stock prices frequently exhibit strong correlations, it can be challenging to research the stock market using batch processing techniques. Recent advances in machine learning have made it feasible to combine forecasting theory and risk optimization in several ways. A new two-stage model is proposed in the investigation. Using the mean-variance method, potential stocks are chosen as the first stage in reducing investment risk (building a portfolio). Second, we discuss a web-based machine learning technique that employs a "passive-aggressive algorithm” and a "perceptron" to predict a stock's price movement over the next few months. We utilized real-world datasets containing 20 health sector scores from four distinct domains to evaluate the efficacy of our methodologies. We calculated the Hamming loss, AUC score for accuracy, and classification reports. The results of our procedure are numerically compared to those of previous studies that employed conventional solutions. Our research indicates that non-selection techniques are less effective than portfolio-based learning-based ensemble techniques.
According to Mohapatra et al. (2022), it is possible to predict the stock returns of Indian institutions using technical indicators. This research develops an ensemble machine learning model to achieve this objective. Price, volume, and change are the three key concepts of technical analysis that support these indications. Mean Absolute Percentage Error (MAPE), Mean Absolute Error (MAE), Root Mean Square Error (RMSE), and Mean Squared Error (MSE) have been used to monitor the performance of the model. Three of the four examined ensemble models performed better than XGBoost. Both the RMS error and the MAE are approximately 5%. The models' feature importance diagrams illustrate the input variables' significance for making accurate predictions. By relying less on macroeconomic factors, the proposed machine learning models can predict stock market trends and returns more accurately, particularly for banking equities. This is beneficial for traders, investors, and portfolio managers. Market participants can also utilize these techniques to forecast price-volume patterns, liquidity profiles, and trading patterns for equities of all sizes. Lastly, the methodologies are exact and promise to predict trends, particularly significant shifts.
According to Ghosh et al. (2022), the study provides a model for estimating futures prices of securities sold on India's National Stock Exchange (NSE) before and after the COVID-19 pandemic. In a structural model, the variables that explain the study, such as past and implied volatility, currency rate volatility, sector outlook, crude price volatility, market sentiment, and current prices, are assigned weights. Ensemble Feature Selection (EFS), which employs the Boruta and Regularized Random Forest (RRF) algorithms, is our technique for this purpose. Predictions are made using
Autoencoder (AE) and Kernel Principal Component Analysis (KPCA) in conjunction with Deep Neural Network (DNN) and Regularized Greedy Forest (RGF). Explainable Artificial Intelligence (AI) was used to determine the magnitude and nature of the explanatory sections' impact. Number-based evaluations demonstrate that our hybrid structure is adequate. The results indicate that the relative importance of the variables that explain futures prices varies across firms and over time..
4. Methodology and analysis
I will predict the level of risk and return associated with Indian economic indices by employing a strategy that combines econometric and machine learning algorithms. In order to establish which elements influence the returns and risks of these sector indexes, algorithms for machine learning will be applied. At the same time, statistical methods will be used to uncover historical trends and patterns. The first step in this process is to gather historical data on sector indexes, which may include daily closing prices, volumes, and other economic and financial indicators (Yang et al., 2023). Following that, the data will be preprocessed to eliminate any outliers and clean up the format before an accurate analysis can be performed on them. After that, the data will be separated into two sets, which will be the test set and the research set, respectively. Following the fitting of multiple econometric and machine learning models to the training data, those models will be tested on the test set.
In order to create the economic models claimed by Faridi et al. (2023), techniques such as time-series analysis, panel data analysis, and linear regression will be utilized. The historical relationship between sectoral indices and economic and monetary factors will be modeled and displayed using these models. The computed coefficients of these models can be used to investigate the risks and rewards associated with various industry indexes. There are many different types of machine learning models, but some of the ones that will be employed include support vector machines, neural networks, random forests, and decision trees. When given information about previous returns and economic and financial conditions, these models will be able to make accurate forecasts regarding the potential returns and risks associated with sectoral indexes in the future. The quality and quantity of the training data play a significant role in determining the accuracy of the models.
In order to validate the efficacy of the approach, both the econometric and machine learning models will be subjected to a battery of diagnostic examinations. These investigations reveal problems like multicollinearity, heteroskedasticity, and overfitting (Faridi et al., 2023). If issues are discovered, they will be fixed by analyzing several alternative models or adjusting the data. Following the teaching and validation of the models, they will be used to make projections regarding the potential returns and risks associated with sector indexes. These forecasts will be used by portfolio managers and investors in order for them to make informed investment decisions. An investor may choose to expand their investment in a sectoral index if industry analysts predict that the index will produce strong returns in the future with a relatively low level of risk.
Combining econometric models with machine learning models could efficiently
anticipate the volatility and profitability of Indian industry indexes (Shin & Woo, 2022). This technique will be more effective than either strategy alone since it will combine the benefits that may be gained from both methods. Because no model can ever be accurate to the hundredth of a percent, buyers must always be prepared for the possibility of risk and uncertainty. Therefore, in order to lessen their risk exposure, prudent business owners should continuously diversify their holdings.
5. Conclusion
Conclusively, this study highlights the benefits and drawbacks of investing in various areas of the Indian economy by utilizing econometric and machine learning models to predict the risk and return of Indian sectoral indexes. These models demonstrate the advantages and downsides of investing in these areas. It has been demonstrated that the strategy proposed in this dissertation, which makes use of both econometric and machine learning models, is an efficient tool for forecasting how industry benchmarks would behave. The outcomes of the study can help investors and stock administrators decide how to invest their funds by providing guidance based on their preferences. Risk and uncertainty are inherent to every transaction; therefore, it is impossible for any model to provide 100% accurate forecasts of the future.
Intelligent investors should diversify their holdings so that they are protected from these dangers to the greatest extent that is possible. The technique used in this dissertation could be strengthened by conducting additional studies into other elements that have the potential to affect the performance of sectoral indices. This dissertation has made major contributions to the field of finance and investments, and its conclusions have the potential to be utilized in a range of developing countries around the world.
6. References
Beloskar, V. D., & Rao, S. V. D. N. (2022). Did ESG Save the Day? Evidence From India During the COVID-19 Crisis. Asia-Pacific Financial Markets. https://doi.org/10.1007/s10690-022-09369-5
Faridi, S., Madanchi Zaj, M., Daneshvar, A., Shahverdiani, S., & Rahnamay Roodposhti, F. (2023). Portfolio rebalancing based on a combined method of ensemble machine learning and genetic algorithm. Journal of Financial Reporting and Accounting, 21(1), 105-125.
https:// www.emerald.com/insight/content/doi/10.1108/JFRA-11-2021-
0413/full/html
Ghosh, I., Chaudhuri, T. D., Alfaro-Cortés, E., Gámez, M., & García, N. (2022). A hybrid approach to forecasting futures prices with simultaneous consideration of optimality in ensemble feature selection and advanced artificial intelligence. Technological Forecasting and Social Change, 181, 121757. https:// www.sciencedirect.com/science/article/pii/S0040162522002827
Mohapatra, S., Mukherjee, R., Roy, A., Sengupta, A., & Puniyani, A. (2022). Can Ensemble Machine Learning Methods Predict Stock Returns for Indian Banks Using Technical Indicators? Journal of Risk and Financial Management, 15(8), 350. https:// www.mdpi.com/1911-8074/15/8/350
Padhi, D. K., Padhy, N., Bhoi, A. K., Shafi, J., & Yesuf, S. H. (2022). An intelligent fusion model with portfolio selection and machine learning for stock market prediction. Computational Intelligence and Neuroscience, 2022. https:// www.hindawi.com/journals/cin/2022/7588303/
Patni, S., & Gadekar, A. R. (2022). Stock Market Prediction With Risk Analysis Using Two ml Module. International Journal of Intelligent Systems and Applications in Engineering, 10(3s), 40-44.
https://ijisae.org/index.php/IJISAE/article/view/2409
Sen, J. (2022). A forecasting framework for the Indian healthcare sector index. International Journal of Business Forecasting and Marketing Intelligence, 7(4), 311-350.
https:// www.inderscienceonline.com/doi/abs/10.1504/IJBFMI.2022.125783
Shin, S. Y., & Woo, H. G. (2022). Energy Consumption Forecasting in Korea Using Machine Learning Algorithms. Energies, 15(13), 4880.
https:// www.sciencedirect.com/science/article/abs/pii/S0952197623000271
Yang, C. H., Lee, C. F., & Chang, P. Y. (2023). Export-and import-based economic models for predicting global trade using deep learning. Expert Systems with Applications, 218, 119590.
https:// www.sciencedirect.com/science/article/abs/pii/S095741742300091X
image2.png
image3.png
image4.png
image5.png
image6.png
image7.png
image8.png
image9.png
image10.jpeg
image11.png
image1.png
,
10 |
Dissertation Guidelines |
Dissertation Guidelines |
9 |
Dissertation Guidelines Table of Contents 1. Objective of the Dissertation 2 2. Layout 2 3. Format 5 4. Originality and Plagiarism 5 5. References 6 6. Ethics Form 7 7. Submission Instruction 7 8. Support 7 9. Assessment 9 Appendix Grade Criteria 10 There is no set way to write a dissertation. The purpose of these guidelines is to highlight the necessary items and possibly give a few tips and ideas. Objective of the Dissertation The objectives of the dissertation are to develop the ability to investigate specific issues relevant to your degree and to communicate findings in an appropriate manner. You should be worrying more about properness of the investigation and communication rather than trying to show something new. Layout The first page (not necessarily the cover) of the final report should follow this pattern: PORTSMOUTH BUSINESS SCHOOL **TITLE OF DEGREE** Title: Student Name: Student Number: Supervisor: Year of submission: Word Count: Signed statement of originality: “This project is submitted in partial fulfilment of the requirements for the degree of MSc. I hereby declare that this dissertation is substantially my own work. I do/do not (please delete as appropriate) consent to my dissertation in this attributed format (not anonymous), subject to final approval by the Board of Examiners, being made available electronically in the Library Dissertation Repository and/or Department/School/Subject Group digital repositories. Dissertations will normally be kept for a maximum of ten years; – I understand that if I consent, this dissertation will be accessible only to staff and students for reference only; – This permission may be revoked at any time by e-mailing [email protected] Signed………………………………………….” Permission for inter-library loan: I give permission that this report may be photocopied and made available for inter-library loan for the purpose of research. Signed …………………………………………………..” [Note: These statements must be signed and dated. Omit the second if your project is confidential.] You should include an abstract (summary) of the project, inclusive of results and conclusions. This should be no more than 500 words (not counted for the total 8,000) and should fit on a single sheet. The dissertation title, your name, and the date should appear at the top of the abstract. As for the actual dissertation, the following are only guidelines. Every dissertation is different and the appropriate sections and structure can very depending on the subject. This is why you should agree on a reasonable structure with your supervisor. As an example, a dissertation in theoretical economics or theoretical finance will not have ‘data’ and ‘methodology’ sections but a ‘model’ and ‘equilibrium characterization’ sections. The following guidelines are written with an empirical dissertation in mind, however this does not dispense you from discussing the structure with your supervisor. The dissertation should start with an introduction clearly stating the research question and highlighting the aims and background of the study. The background should also provide a motivation for the research. Besides giving theoretical justification for your research, remember to include figures that give a sense of magnitude for the problem at hand. For instance, if you are looking into SME in some particular country, give their contribution to GDP; if you are looking at IPOs, provide some market caps figures. Nothing gives a sense of relevance for a topic like its magnitude. If you like, you can devote a paragraph outlining and motivating the structure of the dissertation. After the introduction, the literature review usually follows. From a practical point of view, the literature review should be the first thing you work on, leaving the introduction, conclusion and abstract last. You can use the literature review you have submitted in May as a starting point, expanding it as you read more, and better understand the topic. Remember that the review should also critically evaluate the previous literature and put it in direct relationship with your own project, from a theoretical, conceptual and methodological point of view. After completing the literature review you should have a clear idea of whether the
Collepals.com Plagiarism Free Papers
Are you looking for custom essay writing service or even dissertation writing services? Just request for our write my paper service, and we'll match you with the best essay writer in your subject! With an exceptional team of professional academic experts in a wide range of subjects, we can guarantee you an unrivaled quality of custom-written papers.
Get ZERO PLAGIARISM, HUMAN WRITTEN ESSAYS
Why Hire Collepals.com writers to do your paper?
Quality- We are experienced and have access to ample research materials.
We write plagiarism Free Content
Confidential- We never share or sell your personal information to third parties.
Support-Chat with us today! We are always waiting to answer all your questions.
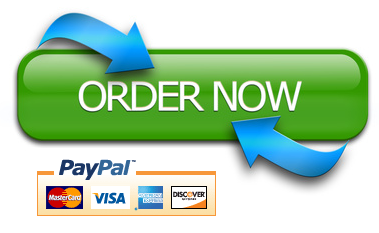