What observations about statistical process control can you make after reading the article? How important is implementing corrective action techniques in maintaining an efficient SPC system? One of the significant difficulties in advancing healthcare quality is the lack of specificity in defining healthcare processes, establishing performance standards, and measuring compliance with standards after they are defined. Explain how the use of SPC can help solve these issues. CaseStudy.docxRoss2006AStatisticalProcessControlCaseStudy.pdf
Please see both attachments for guidance in completing the assignment and for the case study that the assignment is on.
Read the attached article then answer the following questions:
- What observations about statistical process control can you make after reading the article?
- How important is implementing corrective action techniques in maintaining an efficient SPC system?
- One of the significant difficulties in advancing healthcare quality is the lack of specificity in defining healthcare processes, establishing performance standards, and measuring compliance with standards after they are defined. Explain how the use of SPC can help solve these issues.
Attached Files:
· Ross (2006) A Statistical Process Control Case Study.pdf (783.085 KB)
Read the attached article then answer the following questions:
1. What observations about statistical process control can you make after reading the article?
2. How important is implementing corrective action techniques in maintaining an efficient SPC system?
3. One of the significant difficulties in advancing healthcare quality is the lack of specificity in defining healthcare processes, establishing performance standards, and measuring compliance with standards after they are defined. Explain how the use of SPC can help solve these issues.
You will be graded accordingly
Identification of Main Issues/Problem Identification |
|
25 pts |
||||
This criterion is linked to a Learning Outcome Comments on Effective Solution/Strategies |
|
25pts |
||||
This criterion is linked to a Learning Outcome Answers to Assigned/case Questions |
|
25 pts |
||||
This criterion is linked to a Learning Outcome Style and Mechanics, including APA Style |
|
25 pts |
image1.gif
,
Q Manage Health Care Vol. 15, No. 4, pp. 221–236 c© 2006 Lippincott Williams & Wilkins, Inc.
A Statistical Process Control Case Study Thomas K. Ross, PhD
Statistical process control (SPC) charts can be applied to a wide number of health care applications, yet widespread use has not occurred. The greatest obstacle preventing wider use is the lack of quality management training that health care workers receive. The technical nature of the SPC guarantees that without explicit instruction this technique will not come into widespread use. Reviews of health care quality management texts inform the reader that SPC charts should be used to improve delivery processes and outcomes often without discussing how they are created. Conversely, medical research frequently reports the improved outcomes achieved after analyzing SPC charts. This article is targeted between these 2 positions: it reviews the SPC technique and presents a tool and data so readers can construct SPC charts. After tackling the case, it is hoped that the readers will collect their own data and apply the same technique to improve processes in their own organization.
Key words: case study, control charts, quality management, SPC, statistical process control
O ne of Edward Deming’s quality princi- ples is to make quality everyone’s job.1
Health care organizations have always as- sumed that quality is everyone’s job and
everyone knows the difference between quality care and substandard care. Yet health care by relying on individualistic definitions of quality without perfor- mance evaluation systems has never made quality everyone’s job. Consequently, quality management is left to quality improvement director, department, and/or committee, fails to achieve widespread sup- port in the organization, and produces few tangible improvements in processes or outcomes.
Implementing Deming’s principle requires em- ployees to understand what quality is, be capable of identifying substandard performance, and have the authority to make changes that will improve perfor- mance. According to Deming, it is the responsibility of management to suffuse this quality focus into their organizations. Of course, this is the problem in health care; other industries have developed rigorous sys- tems to monitor quality and modify processes when output does not meet expectations. Berwick bluntly addressed this problem; doctors, nurses, reception- ists, and other health care workers are not trained in or capable of changing how they work.2
Health care has been implementing quality im- provement programs for decades but efforts to cross into the fertile fields of quality management always seem to flounder. The inability to apply the quality management techniques of other industries is due to the more complex nature of health care but part of the problem is the way health care has approached quality management. Berwick recognizes that health
From the Department of Health Services and Informa- tion Management, School of Allied Health Sciences, East Carolina University, Greenville, NC.
Corresponding author: Thomas K. Ross, PhD, Department of Health Services and Information Management, School of Allied Health Sciences, East Carolina University, 600 Moye Blvd, Greenville, NC 27858 (e-mail: [email protected]).
221
222 QUALITY MANAGEMENT IN HEALTH CARE/VOLUME 15, ISSUE 4, OCTOBER–DECEMBER 2006
care workers have not been given the tools to sys- tematically incorporate quality measurement in their work.
The following case was developed to demonstrate how statistical process control (SPC) can be applied to health care processes. The SPC is a tool that de- fines what quality is, how performance is measured, and when investigation and possibly correction must occur. The SPC, once understood, empowers the doc- tor, nurse, department manager, and other health care workers to enter into the quality management process on a more equal basis with those trained in quality management techniques. The initial audi- ence for this article is health care managers, but as Deming and Berwick note, improvement occurs only when all employees understand and embrace these quality improvement techniques. Getting managers and opinion leaders on board is only the first step to institutionalizing quality practices throughout the organization.
This article does not assume that the reader is versed in statistics or quality management tech- niques, it does however assume familiarity with spreadsheet software. The goal is to demonstrate that quality improvement techniques can be used produc- tively by all health care workers. At the end of this case it is hoped that the reader will be able to identify a use for the SPC in their area of responsibility, create SPC charts using Excel, and interpret these charts to improve outcomes.
QUALITY IMPROVEMENT IN HEALTH CARE
One of the greatest obstacles to quality improve- ment in health care is the belief of providers that quality management tools developed in other indus- tries are not relevant to health care services. Not only are the initiatives of other industries deemed irrele- vant to health care, many providers hold that quality improvement mechanisms developed in other health care organizations are not applicable to the institu- tion they work in. This belief is the direct result of the second factor, and health care providers are not famil- iar with the purpose, operation, and interpretation
of quality management tools. Without understand- ing quality management techniques and the ends to which they can be applied, it is understandable that health care providers would think these tools have little to offer them.
Health care quality management texts inform the reader that SPC charts should be used to improve delivery processes and outcomes often without dis- cussing how they are created. Conversely, medical research frequently reports the improved outcomes achieved after analyzing SPC charts. This article is targeted between these 2 positions: it reviews the SPC technique and presents a tool and an application so readers can learn how to construct SPC charts and offers suggestions as to when they can be used to im- prove health care delivery processes.
A case study is developed and followed to con- clusion to demonstrate one potential application of the SPC to health care. The intent is to provide the readers with a basic understanding of how to build SPC charts and encourage them to access and analyze the case data using Excel to evaluate performance on their own. The case question is: are medicines de- livered in a timely manner to maximize medical ef- fectiveness and patient comfort? The control charts developed are used to draw conclusions regarding the operation of the system, explore potential rea- sons for the performance observed, and speculate on system changes that could improve the timeli- ness of medicine delivery. After working through the case, it is hoped that the reader will be motivated to apply the principles and techniques to their own work.
One of the major difficulties in advancing health care quality is the lack of specificity in defining health care processes, establishing performance standards, and measuring compliance with standards after they are defined. Attempts to improve operations and out- comes are difficult if not impossible when standards and measures are ill-defined or absent. Efforts to de- fine medical processes continue to be hotly debated, and opponents argue that there is too much variation in medical practice to establish one way of treating patients and dictating to physicians will not improve patient care and may hurt patients.3
A Statistical Process Control Case Study 223
On the other hand, countless research has been done to define proper medical practice; much of this work is amendable to and should be tracked by the SPC to improve health care outcomes for patients. McGlynn et al found that patients received 54.9% of recommended care, more important than their con- clusion was their technical supplement that provided the indicators of what should occur and when it should occur for 30 conditions.4
Other industries recognize that poor performance is the result of variation, that is, deviations from how things should be done that adversely impact out- comes. Controlling variation is the key to process improvement. Health care providers are arguably saddled with more sources of variation than pro- ducers of other goods and services but the issue re- mains; is there too much variation in treatment and will a more systematic approach to health care de- livery processes improve outcomes? The SPC is a tool health care workers can use to determine when variation is a routine part of patient care, neces- sary and beneficial for patients and the health care system, and when variation is excessive and poten- tially harmful. Armed with this information, it is the duty of health care providers to reduce inappropriate variation.
IMPROVING HEALTH CARE
Root cause analysis or routine monitoring?
Current quality control efforts revolve around root cause analysis, that is, a comprehensive examina- tion of an adverse event with the goal of reducing harm to patients by preventing the reoccurrence of the event. JCAHO-accredited health care organiza- tions must conduct a root cause analysis focusing on systems and processes when a sentinel event oc- curs. Root cause analysis is limited by its initiating cause; it is undertaken after an adverse event has oc- curred and examines only those processes that were part of the care episode. In arguing for a broader ap- proach it has been noted “when multiple sources of variation are present, isolated observations provide insufficient information on which to base objective decision making.”5
The SPC is a broader approach that continuously examines processes to identify undesirable trends in performance and institute corrective action before harm arises. Fasting and Gisvold used the SPC in anesthesia to study a range of adverse events that are less severe and more frequent than sentinel events that contained the potential for harm. As a result of their study, they were able to reduce anesthesia accidents.6 The SPC complements root cause analysis and extends process improvement efforts by adding ongoing monitoring of a broad range of events to qual- ity management. JCAHO (LD.5.2) notes that sentinel event analysis is reactive and does not meet the intent of the JCAHO patient safety standard.7 The SPC is not concerned with particular cases but rather with the ongoing function of systems and thus is a proactive approach to operations that monitors performance to detect changes in system performance before prob- lems arise.
The 6 steps of SPC
The goal of the SPC as envisioned by Walter Shewhart is to determine when a system is out of con- trol and requires adjustment to improve its output. An in-control system simply indicates that it is oper- ating close to its historical performance; this perfor- mance, however, may fail to meet generally accepted standards or customer expectations. A system that is out of control indicates performance that is sig- nificantly different from historical performance. The goals of the SPC are to identify when performance deviates sufficiently to endanger quality and improve in-control performance to improve outcomes.
Shewhart’s second goal was to devise a quality monitoring and improvement system that could be operated effectively by workers whose expertise lies in areas other than statistics.8 Such a system required clear signals to indicate when system performance fluctuates too much and be easy to use. Shewhart cre- ated his system in a manufacturing environment for those trained in engineering and, as will be demon- strated, it is as applicable for service industries and those trained in medical sciences. The SPC is de- signed for ease of use; the technique can be described and completed in 6 steps.
224 QUALITY MANAGEMENT IN HEALTH CARE/VOLUME 15, ISSUE 4, OCTOBER–DECEMBER 2006
The SPC can be used to monitor the behavior of any system that produces outputs that can be mea- sured numerically. The SPC can monitor the percent- age of defects in a sample (P charts), the number of de- fects in a sample (c charts), or output characteristics (average and variance, X̄ and R charts) among other things. These applications require employees to rou- tinely collect simple performance measures to moni- tor quality: percent defective, number of defects, the average weight or length of a product, time required to deliver a product or service, and the variance in these characteristics. The case uses X̄ and R charts since the concern is the interval between prescribed medication time and the actual delivery of medicines to patients. An earlier article in this journal provides a concise explanation of when the different control charts should be used.9
Step 1: Data collection
Data collection is the most time consuming part of the SPC process. A manager wanting to assess per- formance using the SPC must determine the desired sample size, how often samples are to be drawn, procedures to ensure that the samples are random, and who is responsible for data collection. Managers must realize that they face a trade-off between the cost of collecting data (sample size and sampling fre- quency) and the accuracy of information obtained from the collected data. Larger samples typically pro- duce more accurate information but are more costly and time consuming to collect. The events sampled must be randomly drawn to ensure that the sample is not biased; that is, the information gained from the sample must be representative of the larger pop- ulation for it to accurately assess the performance of the nonsampled phenomena. The procedures and re- sponsibility for data collection should ensure that the data collector has no incentive to collect either favor- able or nonfavorable performance. After the data are collected, it should be recorded in a spreadsheet.
Step 2: Calculation of descriptive statistics
After the data are recorded, descriptive statistics must be calculated. Descriptive statistics provide the information necessary to understand how the sys-
tem is operating, the first statistic is the mean. The mean is the measure of central tendency and re- ports “average” performance. The mean is used to determine whether performance meets the desired standard. The mean for a sample is calculated as follows: X̄ = ∑
xi/n, the sum of the sampled ob- servations divided by the sample size. If Excel is used to record data, the mean is calculated as fol- lows: =AVERAGE(range of observations). In medica- tion management the mean can be used to determine whether patients received the specified dose—larger or smaller dosage is a problem. Likewise, science and common sense dictate that the most effective inter- ventions are delivered proximate to a medical event; the case seeks to determine whether medicine is de- livered to patients within (plus or minus) 2 hours of the prescribed time.
The second statistic, range (R), provides a mea- sure of the variance in the sample. Range is the high- est value in the sample minus the lowest value and is used to assess the variability in performance. Ex- cel can be directed to scan a series of numbers and identify the maximum and minimum values and per- form the subtraction by the command: =MAX(range of observations)-MIN(range of observations).
The need for both statistics is demonstrated by assuming that the average turnaround time for a labo- ratory test is 60 minutes. Those awaiting results pre- fer a process in which 50% of tests are available in 65 minutes and the remaining 50% in 55 min- utes to a process where one half of results are avail- able in 10 minutes and the other half take 110 min- utes. Average turnaround time, X̄ , for each process is 60 minutes but the first process has a 10-minute range (65–55 minutes) while the second has a 100-minute range (110–10 minutes). Lack of predictability in the second process is a problem. The person awaiting re- sults from the second process does not know whether he or she will receive them quickly or have to wait almost 2 hours. Processes with the narrowest range are generally superior; the process with a 10-minute range provides users with a clear idea of when re- sults will be available and allows them to schedule work accordingly. The variance in the second process can lead to inefficiency if delays in the availability of
A Statistical Process Control Case Study 225
Figure 1. The 6 steps of statistical process control.
information impacts the effectiveness of treatment or providers must repeatedly check for results because they do not know when they will be available.
Differences in system performance are the result of variance, and all systems are affected by variance. Variance is categorized as natural or special cause; natural variation is inherent to a process and is the result of noncontrollable forces such as the capabil- ities of labor and equipment, the impossibility of 100% accurate measurements, and/or environmen- tal factors (ie, the weather). Special cause variation can be traced to a controllable cause(s) that should not be present in a properly operating system. Spe- cial cause variation may arise from system design, in- puts used, fatigue, wear and tear, lack of equipment maintenance, lack of training, etc. Any of these causes could reduce performance below desired or achiev- able levels.10 When assignable variation arises, it is the responsibility of managers to identify its cause and control it to improve outcomes.
Process improvement is built on meeting a desired standard and reducing the variance in performance. Managers must monitor performance on an ongoing basis, using the mean and range to ensure that perfor- mance targets are met. The X̄ chart monitors how ac- tual performance varies from historical performance while the R chart monitors uniformity—the differ- ence between the best and worst performance in a sample. Both charts seek to identify when perfor- mance should be investigated, that is, when observed performance deviates significantly (is too high or too low) from historical performance.
Step 3: Calculation of control limits
The calculation of control limits requires 4 formu- las and provides a quantitative answer to how much variance will be accepted before a process is investi- gated. The formulas for the upper control limit (UCL) and lower control limit (LCL) of the X̄ chart are as follows:
UCL : ¯̄X + (A ∗ R̄)
LCL : ¯̄X − (A ∗ R̄)
The new terms in these formulas are ¯̄X , aver- age performance across the samples collected ( ¯̄X = ∑
X̄ i/number of samples); this is an average of aver- ages, R̄, the average range across the samples (R̄ = ∑
Ri/number of samples), and A, the control chart factor. ¯̄X and R̄ establish the historical performance against which individual samples will be judged. ¯̄X specifies what average performance has been over a large number of samples or extended time period and R̄ defines how performance has varied across the samples or time period. The control chart factor is multiplied by the average range to determine the ac- ceptable range of performance.
The magnitude of the control chart factor (A) is de- termined by sample size that is the number of items (n) in a sample. A larger sample size generally pro- duces more accurate statistics, and consequently the control chart factor is reduced producing tighter lim- its (see Fig 2). When selecting a control chart factor, it is a common mistake for students of the SPC to confuse the number of samples taken with the sam- ple size—control chart factors are determined by the
Figure 2. Control chart factors.11 LCL indicates lower control limit; UCL, upper control limit.
226 QUALITY MANAGEMENT IN HEALTH CARE/VOLUME 15, ISSUE 4, OCTOBER–DECEMBER 2006
sample size (the number of observations in the sam- ple) rather than by the number of samples drawn.
The product of the control chart factor and the aver- age range is added (or subtracted) from the historical performance (the average of the averages) to deter- mine the upper (or lower) control limit. For example, when samples of 25 are drawn, the average output of a process is expected to routinely fluctuate around its historical performance by ±15.3% of its average range (±0.153 ∗ R̄). Investigation is required when- ever the average for a sample varies by more than 15.3% of the average range.
The upper and lower limits for the R chart also require 2 formulas:
UCL :D2 ∗ R̄
LCL :D1 ∗ R̄
D1 and D2 are control chart factors and, similar to A, these factors produce tighter control limits as sam- ple size increases. When samples of 10 are drawn, a sample range could be up to 77.7% above or be- low the average range (1.000 ± 0.541 ∗ R̄) before in- vestigation is warranted. Using a larger sample of 25, acceptable variation is reduced to 54.1% above or be- low the average range (1.000 ± 0.541 ∗ R̄). It is only when a sample range exceeds the control chart limits that investigation and potential corrective action are required.
Step 4: Graphing performance
Steps 2 and 3 provide all the necessary informa- tion to graph actual performance, the average, and the range between the highest and lowest values, against historical and expected performance. Sam- ple averages (or ranges) are graphed as XY charts with the x-axis defining when the sample was col- lected (reported in a chronological order) and the y-axis recording the value of the sample average or range. The centerline (CL), ¯̄X or R̄, and the upper and lower control limits are also graphed to provide the baselines against which actual performance is measured. Routine monitoring of performance, after the control limits are established, requires the rela- tively simple task of collecting data, calculating the
mean and range, and charting the values for new samples.
Step 5: Interpreting performance
Step 5 is the examination of actual and expected performance to determine whether the process is in control or out of control. An in-control or stable pro- cess is one where actual performance, X̄ or R, falls within the control limits with data points lying on either side of the centerline, without exhibiting a pat- tern. Figure 3 presents 6 configurations to look for when examining control charts. Chart 1 shows an in- control process in which data points do not breach the upper or lower control limits and are randomly distributed around the centerline. Chart 2 presents the classic out-of-control process where multiple sample values (samples 2, 5, and 8) breach the es- tablished control limits. Charts 3–6 show no samples breaching the control limits but all have recogniz- able patterns that indicate instability in the process or a systemic change. Sample values fall only on one side of the centerline in chart 3, an upward trend be- ginning in period 5 is evident on chart 4, a cyclical pattern occurs on chart 5, and the lack of varianc
Collepals.com Plagiarism Free Papers
Are you looking for custom essay writing service or even dissertation writing services? Just request for our write my paper service, and we'll match you with the best essay writer in your subject! With an exceptional team of professional academic experts in a wide range of subjects, we can guarantee you an unrivaled quality of custom-written papers.
Get ZERO PLAGIARISM, HUMAN WRITTEN ESSAYS
Why Hire Collepals.com writers to do your paper?
Quality- We are experienced and have access to ample research materials.
We write plagiarism Free Content
Confidential- We never share or sell your personal information to third parties.
Support-Chat with us today! We are always waiting to answer all your questions.
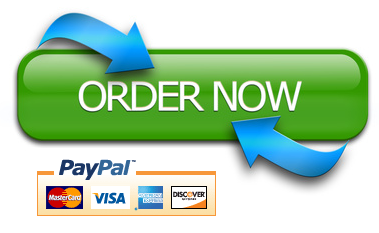