Please analyze the Boston housing data. The codes are provided in the week’s module. Some analyses are discussed in class. Please replicate the coding and interpret the results. Write a
the pdf instructions and sample are attached below
Please analyze the Boston housing data. The codes are provided in the week's module. Some analyses are discussed in class. Please replicate the coding and interpret the results. Write a simple report to present the results.
https://www.cs.toronto.edu/~delve/data/boston/bostonDetail.html
https://www.kaggle.com/code/prasadperera/the-boston-housing-dataset/data
To: Mr. Zhu, Director of Investment Analysis From: Lexi Cioffi, Investment Analysis Intern Date: November 11, 2022 Subject: Boston Housing Exploratory Analysis
Introduction As an intern at the Investment Analysis Group, I have prepared this evaluative report in order to make recommendations for investors on which residential properties are the best to invest in within the Boston area. Recently, our new clients asked our firm to determine the variables that most significantly influence the median home value in Boston in order to make a recommendation of which residential properties within the area are the best to invest in. It is important for our clients to know what variables significantly influence median home value in order to make wiser investments and hopefully increase their return on investment. There are 14 values that I will use throughout my analysis: CRIM (per capita crime rate by town), ZN (proportion of residential land zoned for lots over 25,000 sq. ft.), INDUS (proportion of non- retail business acres per town), CHAS (Charles River dummy variable; 1 if tract bounds river; 0 otherwise), NOX (nitric oxides concentration; parts per 10 million), RM (average number of rooms per dwelling), AGE (proportion of owner-occupied units built prior to 1940), DIS (weighted distances to five Boston employment centers), RAD (index of accessibility to radial highways), TAX (full-value property- tax rate per $10,000), PTRATIO (pupil-teacher ratio by town), B (1000(Bk-0.63)^2 where Bk is the proportion of blacks by town), LSTAT (% lower status of the population), and MEDV (median value of owner-occupied homes in $1000’s). Our clients would like us to consider all of the variables in our evaluation, and then present to them the most significant as well as the least significant variables that play a role in predicting the median home value within the Boston area.
Exploratory Analyses and Visualization Before conducting a full linear regression analysis, I began by exploring the Boston Housing Data using plots and summary statistics. Using the R code summary(Boston) I was able to retrieve summary statistics on each of the 14 variables. For a more specific description of the quantities that I retrieved, please reference Figure 1.
Figure 1. Summary statistics of the Boston Housing Data, providing values of minimum, maximum, mean, median, and interquartile range for each of the 14 variables.
After evaluating the summary data for each of the 14 variables, I noticed that there was a significant range of values for the majority of the variables being examined. This extent of variation confirms that a model is absolutely necessary in order to best capture all of the data as well as to predict which variables have an influence on the median home value. Evaluating Predictor Variables I then decided to investigate individual variables that I felt would hold a greater influence on the median home value in Boston, as well as those that I felt are the most relevant when evaluating a residential property investment. The three variables that I chose to investigate were: crim, age, and tax.
Figure 2. Histogram of the per capita crime rate by town in the Boston area.
Figure 3. Histogram of the proportion of owner-occupied units built prior to 1940. Figure 4. Histogram of the full-value property-tax per $10,000.
I created a histogram for each of the three determined variables using the following R code: hist(Boston$crim, c = “blue”), hist(Boston$age, c = “blue”), hist(Boston$tax, c = “blue”). From the three figures above, I was able to draw conclusions about the range of the variables as well as the relative frequencies of their values. Figure 1 demonstrates that the per capita crime rate per town most commonly falls between the range of 10 and 20, with very few responses having a value above 20. Figure 2 demonstrates that the proportion of owner-occupied units built prior to 1940 ranges from a proportion of 0 to a proportion of 100, with the most common segment being a proportion between 90 and 100. Finally, Figure 3 demonstrates that the full-value property-tax per $10,000 does not have any data that falls between the range of 500 and 650, and that the most frequent value fall between the range of 650 and 700. Evaluating the Response Variable When looking at the response variable, medv, I used a boxplot in order to spot potential outliers and gain a visual representation of the data using R code boxplot(Boston$medv). I also made a histogram with the median home value data in order to get a different visual representation of the data using R code hist(Boston$medv, c = “blue”).
Figure 5. Boxplot of the median value of owner-occupied homes in the Boston area.
Figure 6. Histogram of the median value of owner-occupied homes in the Boston area.
Figures 5 above shows the extensive number of outliers that exist in the median value data. The median of the median value data is around 22, with values approximately 38 or greater being considered outliers in the data set. Figure 6 confirms these findings. The histogram of the median value of owner-occupied homes shows that the most frequent value is between 20-25, with the second most frequent between 15- 20.
Predictor Variable Associations After evaluating the three predictor variables that I felt were most significant (crim, age, tax) and the response variable (medv) on their own, I evaluated the correlations that exist between those variables in order to gain additional insight into the data set. To explore the associations between these variables and the median home value, I decided to utilize scatterplots as well as calculate the correlation coefficient that exists between the two variables using R code plot(Boston$medv~Boston$crim, main="Scatterplot of medv Against crim", xlab = "crim", ylab = "medv", pch=16) and cor(Boston$medv, Boston$crim).
Figure 7. Scatterplot of median value of owner- occupied homes and crime rate per capita by town. Correlation coefficient: -0.388 Figure 8. Scatterplot of median value of owner- occupied homes and proportion of owner-occupied units built prior to 1940. Correlation coefficient: -0.378 Figure 9. Scatterplot of median value of owner- occupied homes and full-value property-tax rate per $10,000. Correlation coefficient: -0.469
Figure 7 represents the relationship between the median home value and the per capita crime rate per town. With a correlation coefficient of -0.388, the variables can be said to have a negative correlation. Having a negative correlation signifies that as the median home value decreases, the crime rate increases, or that as the median home value increases, the crime rate decreases. The scatterplot demonstrates the trend of negative correlation. Figure 8 represents the relationship between the median home value and the proportion of owner-occupied homes built prior to 1940. With a correlation of coefficient of -0.378, the variables can be said to have a negative correlation. The scatterplot demonstrates the relationship of negative correlation because as the age range increases, the median home value generally decreases. Figure 9 represents the relationship between the median home value and the full-value property-tax rate. With a correlation coefficient of -0.469, the variables can be said to have a negative correlation that is even stronger than that of the crim and age variables. The scatterplot demonstrates this negative correlation because as the property-tax rate increases, the median home value generally decreases.
Modeling and Interpretation of Results In order to evaluate the significance that variables have in predicting the median home value in Boston, I began with a model that accounted for all 14 variables that I was given, using R code model_full <- lm(medv ~ ., data = Boston). One other model that I wanted to create was one only accounting for the three variables I felt were most impactful in investment selection: crim, age, and tax. I created this second model, called model1, using R code model_1 <- lm(medv ~ crim + age + tax, data = Boston). I received summary statistic information for both of these models using R code summary(model_full) and summary(model1).
Figure 10. Summary statistics of the full variable model, including all 14 predictor variables within the Boston Housing Data. Estimate coefficients and their significance as well as the coefficient of determination for the model are included.
Figure 11. Summary statistics of the model accounting for the previously selected crim, age, and tax variables within the Boston Housing Data. Estimate coefficients and their significance as well as the coefficient of determination for the model are included.
Between these two models, it can be interpreted that the full model is better than model1. The coefficient of determination, R2, for the full model is 0.7406, whereas the R2 value for model1 is 0.2624. When evaluating models, the model with the highest coefficient of determination is the better fitted model. Because the model1 R2 value is so low, it is unlikely that the three selected predictor variables carry heavy significance in predicting the median home value, holding all other variables constant. Determining the Best Model With my three selected predictor variables producing the worse model, I decided to determine the best model by beginning with all 14 of the predictor variables in the Boston Housing Data. My initial goal was to find the best model that accounted for 3 of the predictor variables, holding all other predictor variables constant. To determine which 3 size model was the best determinant of median home value, I used R code bestsub <- regsubsets(medv ~., data=Boston, nbest=3, nvmax = 14). I then created a plot to visualize the predictor variables using R code plot(bestsub)
Figure 12. A plot demonstrating the best subsets to create a size 3 model that best predicts the median home value in the Boston Housing Data.
Upon examining the figure above, I determined that the best size 3 model, holding all other variables constant, accounts for the variables rm, ptratio, and lstat. On the chart, these variables appear first with the lowest associated BIC, and the lower the BIC, the better the model. The R code for this model is model_best <- lm(medv ~ rm + ptratio + lstat, data = Boston). Using R code summary(model_best) I determined that the coefficient of determination for this model is 0.6786, indicating that there is a better model, though my model_best was the best model that existed when accounting for only 3 of the predictor variables. In order to find the model that best predicts the Boston Housing Data, I first established a null model to which I could compare my best fitted model to using R code nullmodel <- lm(medv~1, data=Boston). In order to determine the best model, I decided to proceed with the backward selection method, using R code model_step_b <- step(model_full, scope=list(lower=nullmodel, upper=model_full), direction = ‘backward’).
Figure 13. Data demonstrating the backward model selection method for the Boston Housing Data.
After evaluating the backward selection method, I determined that the best model for predicting the median value of homes in the Boston area is one that includes all of the predictor variables except for the age and indus variables. In the backward selection method, it is shown that removing age and indus will provide the lowest AIC value. Because the lower the AIC, the better the model, those two variables are the only two which need to be removed in order to further decrease the AIC. On the other hand, the backward selection method tells us that the worst variables to eliminate from the model, in order, are: lstat, rm, and dis. This finding is relatively consistent with the model that I made above which included only the three best predictor variables to use in a model. This means that the model which best predicts the median home value can be established using R code: bestmodel <- lm(medv ~ . – indus – age, data = Boston) After running the best model, the coefficients for each of the included variables were as follows: intercept 36.341, crim -0.108, zn 0.046, chas 2.72, nox -17.378, rm 3.802, dis -1.49, rad 0.300, tax -0.012, ptratio – 0.947, black 0.009, lstat -0.523. An example interpretation of the coefficient of rm would be that 1 additional room in a home impacts the median home value by a factor of 3.802, holding all other variables constant. Within this model, the p values for each of the coefficients are less than 0.05, indicating that the findings are statistically significant. This means that I reject the null hypothesis that there is no association between the predictor variables and the response variable. Additionally, the p value of the f- test is also smaller than 0.05, meaning that at least one of the predictor variables has an impact on the response variable and does not have a coefficient of 0.
Conclusion After concluding my analysis, I have determined that the following model is the most significant in determining the median home value in Boston: medv ~ . – indus – age. I believe that the predictor variables indus and age should not be included in a model to determine the median home value in Boston because they increase the value of the AIC. The model accounting for all of the response variables has an AIC value of 1589.6, whereas the model without the indus and age variables has a lower AIC value of 1587.7. Additionally, in the best model, the R2 value is 0.7406, meaning that 74.06% of the variation in the data is explained by the model. Additionally, we can tell our clients that when considering their future residential investments in the Boston area, the three most important variables to consider are rm, ptratio, and lstat – if they are only wishing to consider the three most important variables.
,
Instruction of Lab 8 EBTM 350, Fall 2022, Professor: Xiaorui Zhu, Ph.D.
Purpose: I hope that by doing this lab, you will get a taste of how to prepare your final project, as well
as (1) practice your R coding; (2) get an idea of the entire process (Exploratory Data Analysis,
regression modeling, data mining, interpreting results, etc.); and (3) realize that data
understanding and result interpretation are not trivial tasks, and they are extremely important in
business projects. Submit a Word file with all the following sections and results well organized.
Requirements: 1. (5 points) Write an interesting story in the first section (Background or Introduction). In my class case, we
want to forecast the median home value in the Boston area (or the wine-tasting preference), but I would
refrain from offering you a specific context. I require you to think about in what situations, you may need to
forecast the median home price for your business/clients and write down your story.
a. For example, you can pretend you are a real estate buyer agent. Your decision is to give a
recommendation for your client (buyer) to make a reasonable offer for the house they are interested in.
So, you want to predict the median house price in a certain area with attributes so that your clients can
use it as a reference to make the offer.
b. Another simple example: From an analyst’s perspective, I want to understand how these predictors are
associated with the median home price. What are those major contributors? So, when I want to buy a
house, I should focus on these important attributes because they highly affect the market value of the
house.
c. Yours.
2. (10 points) Exploratory Analyses & Visualization.
a. (2 pts) Explore the data (use plots, summary statistics, etc.) and provide some findings and your
interpretation.
b. (2 pts) Explore the response variable: median house value (use visualization tools, check outliers, etc.).
c. (2 pts) Explore some of the predictors that you believe are important (use visualization tools, check
outliers, etc.).
d. (4 pts) Explore the associations between predictors and your response variable visually and
quantitatively.
3. (20 points) Modeling and Interpretation of your results.
a. (6 pts) Use multiple linear regression models we just learned. You may need to explore models with
different predictors and find the best one.
b. (6 pts) Use at least one variable selection method we learned this week (Best subset selection, Forward,
backward, stepwise selection) to find the best model.
c. (8 pts) Interpret the fitted model from multiple perspectives: find these significant predictors by t-test (2
pts), interpret the coefficient estimates (4 pts), and check whether the whole model is significant (F-
test) (2 pts).
4. (5 points) Summary or Conclusion:
a. (2 pts) Compare all the models you obtained (Use information criteria AIC/BIC, and goodness-of-fit R2 to
support your conclusion).
b. (2 pts) Provide a suggestion of the best model.
c. (1 pts) Conclude your findings: what predictors are useful, what is the performance of your model etc.
,
library(MASS) data("Boston") colnames(Boston) model_full <- lm(medv~., data = Boston) summary(model_full) model_1 <- lm(medv~crim+zn, data = Boston) # Manually select summary(model_full) summary(model_1) AIC(model_full); BIC(model_full) AIC(model_1); BIC(model_1) # Best Subset install.packages('leaps') library(leaps) # regsubsets only takes data frame as input subset_result <- regsubsets(medv~., data=Boston, nbest=2, nvmax = 14) summary(subset_result) plot(subset_result) # Backward Elimination nullmodel <- lm(medv~1, data=Boston) summary(model_full) # has fitted: model_full <- lm(medv~., data=Boston) model_step_b <- step(model_full, direction='backward') # Forward Selection model_step_f <- step(nullmodel, scope=list(lower=nullmodel, upper=model_full), direction='forward') # Stepwise Selection model_step_s <- step(nullmodel, scope = list(lower=nullmodel, upper=model_full), direction='both')
,
install.packages(MASS) library(MASS) data("Boston") summary(Boston) # Exploratory analysis # 1. Exploring the range and distribution hist(Boston$medv) hist(Boston$Cost) hist(Boston$Grad) hist(college$Debt) table(college$City) # 2. Exploring the association between Earnings and other numerical variables plot(Boston$medv ~ Boston$crim) cor(Boston$medv, Boston$crim) # 3. Exploring the association between Earnings and categorical variables tapply(college$Earnings, college$City, mean) boxplot(college$Earnings~college$City) # 4. Association between Earnings and Cost by City plot(college$Earnings~college$Cost, main="Scatterplot of Earnings against Cost", xlab = "Cost", ylab = "Earnings", pch=16, col=ifelse(college$City == 1, 20, 26)) legend("topright", legend=c("Other", "City"), pch=16, col=c(20, 26)) # Build models Model <- lm(Earnings ~ Cost + Grad + Debt + City, data = college) summary(Model) # Predict predict(Model, data.frame(Cost=25000, Grad=60, Debt=80, City=1)) predict(Model, data.frame(Cost=25000, Grad=60, Debt=80, City=1), level = 0.95, interval = "confidence") predict(Model, data.frame(Cost=25000, Grad=60, Debt=80, City=1), level = 0.95, interval = "prediction") # Model selection: goodness-of-fit measure Model1 <- lm(Earnings ~ Cost, data = college) Model2 <- lm(Earnings ~ Cost + Grad + Debt, data = college) summary(Model1) summary(Model2) Model3 <- lm(Earnings ~ Cost + Grad + Debt + City, data = college) summary(Model3) round(summary(Model3)$coefficients, digits = 4) # Lab 7 Q 15 library(readxl) myData <- read_excel("Labs/Ch7_Q15_V18_Data_File.xlsx") View(myData) model <- lm(Price ~ Sqft + Beds + Baths + Colonial, data = myData) summary(model) predict(model, data.frame(Sqft=2500, Beds=3, Baths=2, Colonial=1), level=0.95, interval="prediction") # Lab 7 Q 22 library(readxl) myData <- read_excel("Labs/Ch7_Q22_V11_Data_File.xlsx") View(myData) summary(myData) model <- lm(Property_Taxes ~ Size, data = myData) summary(model) # Lab 7 Q 33 library(readxl) myData <- read_excel("Labs/Ch7_Q33_V07_Data_File.xlsx") View(myData) summary(myData) model <- lm(Time ~ Miles + Load + Speed + Oil, data = myData) summary(model)
Collepals.com Plagiarism Free Papers
Are you looking for custom essay writing service or even dissertation writing services? Just request for our write my paper service, and we'll match you with the best essay writer in your subject! With an exceptional team of professional academic experts in a wide range of subjects, we can guarantee you an unrivaled quality of custom-written papers.
Get ZERO PLAGIARISM, HUMAN WRITTEN ESSAYS
Why Hire Collepals.com writers to do your paper?
Quality- We are experienced and have access to ample research materials.
We write plagiarism Free Content
Confidential- We never share or sell your personal information to third parties.
Support-Chat with us today! We are always waiting to answer all your questions.
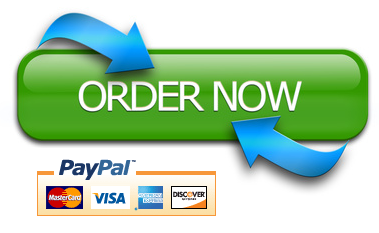