Describe what you learned from the Course Describe how you may appl
- Describe what you learned from the Course.
- Describe how you may apply the information in your current or future career.
Certified Specialist Business
Intelligence (CSBI) Reflection
Part 5 of 6
CSBI Course 5: Business Intelligence and Analytical and Quantitative Skills
● Thinking about the Basics
● The Basic Elements of Experimental Design
● Sampling
● Common Mistakes in Analysis
● Opportunities and Problems to Solve
● The Low Severity Level ED (SL5P) Case Setup as an Example of BI Work
● Meaningful Analytic Structures
Analysis and Statistics
A key aspect of the work of the BI/Analytics consultant is analysis. Analysis can be defined as how the data is turned into information. Information is the outcome when the data is analyzed correctly.
Rigorous analysis is having the best chance of creating the sharpest picture of what the data might reveal and is the product of proper application of statistics and experimental design.
Statistics encompasses a complex and detailed series of disciplines. Statistical concepts are foundational to all descriptive, predictive and prescriptive analytic applications. However, the application of simple descriptive statistical calculations yields a great deal of usable information for transformational decision-making. The value of the information is amplified when using these same simple statistics within the context of a well-designed experiment.
This module is not designed to teach one statistic. It is designed to place statistical work within the appropriate context so that it can be leveraged most effectively in driving organizational performance..
An important review of the basic knowledge for work with descriptive and inferential statistics.
The Basic Elements of Experimental Design
Analytic tools also can provide an enhanced ability to conduct experiments. More than just allowing analysis of output of activities or processes, experiments can be performed on processes and the output of processes. Experimenting on processes is a movement beyond the traditional realm of report-writing analysis(collection and analysis of data without applying changes to factors to find differences that are not random variations) and observational studies. This leads to performance improvements, as this enables decision management recommendations and guidance on future actions to undertake.
In experiments the focus is on carrying out specific orderly procedures to verify/refute/establish the validity of one or more thoughts(hypotheses) on what might happen in each situation. For example, what happens to collections when a method or process like accelerating denial review is manipulated. We think we know, of course, as we have observed changes implemented in the past.
However, an experiment is needed to ensure the change is indeed significant, the improvement is not just random positive variation and the new procedure is not a waste of resources. The experiment provides insight into true cause-and-effect by demonstrating what outcome occurs when a factor is manipulated. This is greatly enhanced through the power of predictive analytics.
Prediction allows the performance of what if and off-line scenarios. Subsequently the parameters in each positive what-if can be tried in real-time to look for proof of the effect.
Experimental Design
Then: ● Better targets, metrics or KPIs can be established, as what is possible from a process is
now more fully understood within agreed levels of confidence. ● Parameters, rules or recommendations can be implemented to guide decisions in
real-time dynamics to achieve desired, favorable or anticipated results in a situation or as a result of a process toward attainment of targets or goals.
● Decisions/decision-making attains greater precision and speed.
Because there is natural variation that must be considered and dealt with, bias must be eliminated, and working one time is not enough. The goal is to implement things that work, remember that things that work are real and real things are replicable.
Here is a 3 step process:
1. Consider the question that should be answered and possible ideas about what these answers might be-the hypothesis. For example, a new methodology to speed
collections might be needed because an organization is not up to the benchmark they track.
2. Consider the sample to be tested and data collection. 3. Design a proper experiment, taking into consideration the variation, bias and replications
needed in collecting the data.
Example
There are four new process ideas by which to engage collections activity for accounts. From the general population of accounts, accounts that fit the desired sample size have been randomly selected. Credit score groups are created, and parties responsible for accounts are assigned to them-the lowest four scores in group one, and so on through the highest score. Then each member of each credit score group is randomly assigned to use one of the new processes in collecting their account. A control group in which current collection processes are continued should also be maintained.
Sampling
Correcting Targeting
The objective of the experience is to make inferences about the population. However, the population may be too large to test in its entirety. If so, a sample is needed. A sample is the data set used to make inferences about the entire population. Again, it is important to have a firm grasp on what one is trying to answer and how this might be emphasized, as without this understanding, an incorrect population might be targeted-for example, is the effort focused on speeding collections overall, then some sort of continuous sampling of all accounts is in order. If it’s only slow-pay accounts, then a very different sample is needed and maybe a different approach and experiment time frame.
Representative Samples
Be sure that samples are representative of the population. If not representative of the population, conclusions cannot be drawn, since the results would be different than for the entire population. This leads to the idea of sampling risk.
There are two types of sampling risks:
1. The risk of incorrect acceptance of the research hypothesis. The sample can yield a conclusion that supports a theory about the population when it is not existent in the population.
2. The risk for incorrect rejection. The sample can yield a conclusion that rejects a theory about the population when the theory holds true in the population.
*Please note: The risk of incorrect rejection(ii) is more concerning than the risk of incorrect acceptance(i).
Consider this example: An experimental drug was tested for its debilitating side effects( hypothesis= the drug has debilitating side effects).
With incorrect rejection, the researcher will conclude that the drug has no negative side effects. The entire population will take the drug believing that it has no side effects, yet members of the population will suffer consequences of the mistake of the researcher.
While the risk of incorrect acceptance, the researcher will conclude that the drug has no negative side effects (yet the truth is that it doesn’t). The entire population will then abstain from taking the drug and no one is harmed.
Practicability
Practicability of the sampling must be considered. Statistical sampling techniques allow one to estimate the number of samples needed, which speaks to availability of the subjects/samples, the duration of the study, the workforce that the study demands, the materials, tools/equipment, ethical concerns and maybe the need for the study if these costs are too high.
Modeling Methods
When determining sample size, remember first that each situation is different and calls for differing statistical modeling methods. Every statistics modeling method has its own sampling rule. These rules intersect in designing your experiment, as one must match the modeling methods that address the question being asked and develop an appropriate sample for it.
Example
A t test is used when you cannot know the result of a total population yet want a level of confidence that what your sample is indicating is true or applicable (scales) to the entire population. A two-sample t test (a test regularly used in comparative testing experiments in healthcare) allows for a sample size as small as six and calls for 920 depending on the confidence level desired in the result -25% confidence level with six and 99% confidence with 920.
Common Mistakes in Analysis
Sophisticated Compensates
Sophistication compensates for lack of data and/or business understanding. The convenience of available applications can lead to a temptation to supplement lack of data or business understanding with sophisticated statistics. This will result in incorrect approaches to analytics and problem-solving. It is necessary to understand the business, the problem being addressed, the process, the data underneath the process and then apply analytics tools. Tool selection is important as well. Do not use tools that are inappropriate to the task for example, using linear programming to fix resource use in relation to a “fixed average volume demand,” when the clinical unit, for which this information is to improve decision-making, is one where volume demand is naturally quite variable. This model will continually require adjustment (likely daily) to be accurate. Meanwhile pick a department for which demand is quite stable- say outpatient therapy. In these departments, healthcare users are waiting or booked to start many weeks into the future. This Is stable demand and linear programming might work in relation to some resources and situations, but not others. One needs to determine whether a decision is about planning a time frame to vary it (increase or decrease). If this is the case, one needs to ascertain how well linear programming will aid making long-term adjustments.
In advance, it is important to run tests (experiments not observational studies) comparing one’s models with actual results, and to use actual numbers in the models. If off by 5% to 10%, then rethink the model application. It is crucial to focus on developing business and data understanding before getting started and to experiment along the way to develop and ensure appropriate use of tools and techniques.
Isolating and Explaining Meaningful Patterns
It is often difficult to isolate and explain the meaningful patterns shown by the data. When attempting to explain everything detected, there is a tendency to consider randomness or “noise” in the system. Suppose there is descriptive information showing volume increasing over the last eight months. Does this mean expansion is required? In the quest for growth, some might look quickly in the direction of expansion. Is this a random situation? Is it part of a long-term long-wave cycle? Is it a temporary shift due to external economic factors, as an area bounces back from recession? None of these would call for expansion. Here knowledge of the data, the environment, statistics and use of appropriate tools are crucial, along with willingness to move beyond the intuitive guess.
Correlation vs. Causation
When correlation is shown, it does not mean the independent variable is the driver or cause. The data may show that healthcare user inpatient admissions with chronic heart failure(CHF) diagnosis rise a few days after every holiday. Are holidays the cause? They may be. However, one must isolate all the reasons the CHF admission increases and test to prove if these factors are more prevalent during holidays. Has one considered all things occurring around holidays? Even if a full moon correlated with certain activity, would it really be the
cause? How could it be proven? These examples bringup the topic of hypothesis testing. When considering causation, one must develop a list of possible reasonable business predictions (hypothesis) for the results being studied.
1. Being unaware of the need for experiments 2. Engaging use of wrong tool:
● Making do with one-size fits-all ● Using visualization tools and thinking this will address analytic needs ● Utilizing tools that require known and/or stable demand ● Using non-predictive tools for predictions
3. Improper consideration of systems dynamics ● Volume demand fluctuations ● Dependencies ● Resource demand and supply ● Long-term and short-term frames ● Seasonality ● Time of day and day of week ● Environmental trends
4. Not understanding the business
Overfitting/Underfitting
In statistics overfitting is “the production of an analysis that corresponds to closely or exactly to a particular set of data, and may therefore fail to fit additional data or predict future observations reliably.” An overfitted model is a statistical model that contains more parameters than can be justified by the data. The essence of overfitting is to have unknowingly extracted some of the residual variation (i.e. the noise) as if that variation represented underlying model structure.
Underfitting occurs when a statistical model cannot adequately capture the underlying structure of the data. An underfitting model is a model where some parameters or terms that would appear in a correctly specified model are missing. Underfitting would occur, for example, when fitting a linear model to non-linear data. Such a model will tend to have poor predictive performance.
Resource application/allocation
The organization supplies fewer resources than are really needed for the task that is expected to be performed.
Opportunities and Problem to Solve
Opportunity Identification & Selection
Stephen R. Covey, the author of “The 7 Habits of Highly Effective People” pointed out that people often lose sight of what is important in the daily rush of taking care of urgent matters. Organizations are no different, and in complex ones such as hospitals and other large-scale healthcare providers, difficulties may be amplified. There are high volumes of activity, change and so much data to look at, what matters?
As discussed earlier in the course, incoming requests to the BI/A consultant/team can come in all at once, overwhelming the team’s capacity and lowering productivity and results. And of greater importance, the BI/A may be faced with many requests of debatable value, meaning time is taken up not surfacing those of real appreciable value. Here the BI/A consultant must undertake two tasks:
First Task 1. Develop and engage a process for surfacing meaningful high value analytic activity to
engage. In particular, the analytics team: ● Should focus on how to support the organization’s strategic initiatives and
direction(direction is a two-edged thought-organizations can have a strategic direction that is embedded in the process and between the specific written lines of the formal plan, yet documentation and actions point out a direction to be probed).
● Should ensure that a significant level of specific action is focused on things that are going well, so it can catapult forward and find new opportunities to move ahead.
● Should not always focus on poor performance areas(although they should not be ignored either) because doing so makes forward movement difficult.
Second Task 1. Develop and engage a work prioritization process. This process will involve a
combination of quantitative and qualitative factors that involve item weightings to reflect the organization’s differing situations and priorities for action. There is a specific call here for the process to include the expected dollar outcome resulting from the project and the information yielded by the work. This is vital to calculate, understand and report the value of the analytics work engaged. The specific value of analytic work is now being sought by senior decision-makers. One needs to be ready to report this in a meaningful manner.
Opportunity Identification
Opportunity Identification & Selection
Added considerations to the process are the following areas. ● Focus on patient safety ● Development of a high reliability are environment ● Process management improvements ● Bottlenecks, flow and throughout ● Government indicators ● What drives KPIs ● Triple aim activities ● Population health
Consider the following:
● processes(continuous, such as charge capture, billing or continuously repeated actions such registration, medication administration, staffing or supply replenishment)
● Decisions within the processes above, especially specific decision points having the greatest impact on the process or organization overall
● Organizational KPIs 1. Are they necessary 2. Are they really key indicators 3. Should they be changed to something forward-looking or something richer
in information or context Next-
● What is provided now(anything directly above) ties directly to the Driving Value Chart or Added considerations ideas
● What is the governing board and senior leadership team looking at in the monthly reports? Could these be used as leading indicators?
The idea here is to take a critical look at current output and move to work that has a high yield and is actionable in ways that stimulate forward progress/improved performance leveraging all three analytic viewpoints.
Sorting through opportunities-prioritization analysis
Now the opportunities to apply analytics have been identified, sortation/prioritization is necessary. This is a straightforward approach, yet it is designed with a level of detail that critically considers the complex variety of drivers at the foundation of success or disappointments.
The prioritization matrix chart(PMC) contains characteristics to be evaluated and categorized by focus of the analytic lens provided by the category.
This tool enables a prioritization that allows tradeoff decision-making about what to work on with limited resources. It also helps ensure that important items are not obscured by items of great weight or urgency.
A four step process will be engaged
Business Functional Areas of Focus
Finance ● Financial statements analysis
Collepals.com Plagiarism Free Papers
Are you looking for custom essay writing service or even dissertation writing services? Just request for our write my paper service, and we'll match you with the best essay writer in your subject! With an exceptional team of professional academic experts in a wide range of subjects, we can guarantee you an unrivaled quality of custom-written papers.
Get ZERO PLAGIARISM, HUMAN WRITTEN ESSAYS
Why Hire Collepals.com writers to do your paper?
Quality- We are experienced and have access to ample research materials.
We write plagiarism Free Content
Confidential- We never share or sell your personal information to third parties.
Support-Chat with us today! We are always waiting to answer all your questions.
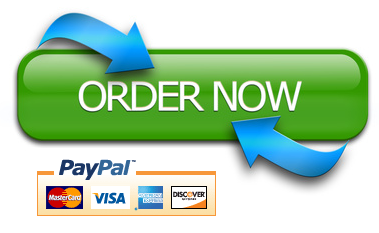