Overview: In this assignment, you will write a (3 pages) addre
Overview: In this assignment, you will write a (3 pages) addressing artificial intelligence according to the prompt below.
Instructions:
- Read and review the material attached
- Discern the similarities and differences between artificial intelligence, machine learning, and predictive analytics.
- Explain the relationship between artificial intelligence, machine learning, and predictive analytics.
Transactions of the SDPS: Journal of Integrated Design and Process Science XX (XXXX) XX-XX DOI 10.3233/JID200002 http://www.sdpsnet.org
1092-0617/$27.50© 2020 – Society for Design and Process Science. All rights reserved. Published by IOS Press
Convergence of Artificial Intelligence Research in Healthcare: Trends and Approaches
Thomas T.H. Wan *
Professor of Healthcare Administration and Medical Informatics, Kaohsiung Medical University, Taiwan and Professor Emeritus of the Department of Health Management and Informatics, University of Center Florida, Orlando, USA
Abstract A value-based strategy relies on the implementation of a patient-centered care system that will directly benefit patient care outcomes and reduce costs of care. This paper identifies the trends and approaches to artificial intelligence (AI) research in healthcare. The convergence of multiple disciplines in the conduct of healthcare research requires partnerships to be established among academic scholars, healthcare practitioners, and industrial experts in software design and data science. This collaborative work will greatly enhance the formulation of theoretically relevant frameworks to guide empirical research and application, particularly relevant in the search for causal mechanisms to reduce costly and avoidable hospital readmissions for chronic conditions. An example of implementing patient-centered care at the community level is presented and entails the influence of the context, design, process, performance and outcomes on personal and population health, employing AI research and informational technology.
Keywords: AI research, context-design-performance-outcomes framework, predictive analytics, shared decision support, patient-centered care
1. Introduction
The Institute of Medicine (IOM) of the National Academies of Science has estimated that 44,000 to 98,000 Americans die annually due to preventable mistakes in healthcare each year (Kohn, Corrigan, & Donaldson, 2000). The IOM has doggedly hounded the nation’s health care delivery system because it “…has fallen far short in its ability to translate knowledge into practice and to apply new technology safely and appropriately (Institute of Medicine, 2001)”. The IOM (2003) has made continuity of care a primary goal of its comprehensive call for transforming the quality of care in the United States. In 2006, the American College of Physicians (ACP) established continuity of care as a central theme for restructuring or reengineering healthcare. Recent research of life-limited patients receiving patient-centered care management showed a notable 38% reduction of hospital utilizations and a 26% reduction of overall costs with high patient satisfaction (Sweeney, Waranoff, & Halpert, 2007). Thus, it is imperative to establish scientific evidence in support of the need for adopting healthcare technologies/devices (Reckers-Droog et al., 2020) and expanding home care monitoring as part of the patient-centric care management technology (Williams & Wan, 2015). The current status of the healthcare system is evolving from a provider-centric to a patient-centric care modality.
* Corresponding author. Email: [email protected] Tel: 407-823-3678.
2 Thomas T.H. Wan. / Convergence of Artificial Intelligence Research in Healthcare: Trends and Approaches
The changes in ecology of medical care are greatly facilitated by the availability of advanced health technology and informatics (Rav-Marathe et. al, 2016), particularly related to chronic disease and self-care management. For instance, design and process science plays a pivotal role in reshaping the service delivery system for improving the efficiency and quality of patient care safety through the adoption of usable information technology tools. Furthermore, the workflow of health services begins to be more standardized and routinized. Important clinical and personal care data are often used to assess the performance of healthcare system.
Innovative collaboration in establishing academia-industry partnerships for artificial intelligence (AI) research and development in healthcare is essential to the improvement of quality and efficiency in care management practice. An evidence-based approach for doing the right thing right in healthcare is the fundamental step to establish performance guidelines and enhance the productivity of healthcare workforces. Since 2019 the Centers for Medicare and Medicaid Services (CMS) has launched the projects for AI Health Outcomes Challenge and offered federal grants and contracts to innovators to demonstrate how AI tools –- such as deep learning and neural networks – can be used to predict unplanned hospital and skilled nursing facility admissions and adverse events. By partnering with the American Academy of Family Physicians and Arnold Ventures, CMS challenges researchers and practitioners to harness AI solutions to predict health outcomes for potential use in CMS Innovation Center’s innovative payment and service delivery models.
In order to optimize the effectiveness of care management strategies we need to pay special attention to human factors in delivering patient-centered care. Professor Barbara Huelat, a renowned healing environment designer, often says that we should include human centric or patient-centered factors in the design of a system to optimize the healthcare delivery systems (Huelat and Wan, 2011). Hence, we should use information technology to identify and target population subgroups who are most likely to benefit from the use of innovative techniques. Most importantly, we have to utilize the knowledge-based information system and technology to guide shared decision making for patient care. Thus, human factors influencing the quality and efficiency of care can be effectively incorporated into the design and implementation of AI in healthcare.
A report on the rankings of health for more than 3,000 counties in the U.S. has documented the need for recognizing four categories of predictors of the variability in population health and performance in 2019 (www.countyhealthrankings.org). The first category is physical environmental and ecological factors, which account for 10% of the total health variation. The second category is medical care, accounting for 20% of the variation. The third category is health behavioral factors, accounting for 30% of the variation. The fourth category is related to socio-economic factors or disparities, accounting for 40% of the variation in county health. So, if one would like to improve health status or reduce health disparities, it is necessary to pay greater attention to health behavioral and socioeconomic factors that may influence the health and health care of the population. Naturally, healthy habits and lifestyles are important components of promoting health and wellbeing for the people. Therefore, to actualize the power of AI or technology- oriented decision support systems in healthcare we should prioritize healthcare research on identifying the determinants of personal and population health. The past, current, and future interests in pursuing AI research are relatively centered in employing machine-learning methods (i.e., classic support vector machine, neural network and deep learning) for structured data and the natural language processing methods on unstructured health data (Jiang et al., 2017). The opportunities for understanding human emotions and behavioral responses to care rendered should be thoroughly explored by AI researchers and software developers.
The use of theoretically informed frameworks to guide machine learning and deep learning explorations in healthcare data is important for generating causal inferences derived from specified and justifiable assumptions in the empirical investigation of healthcare outcomes. The proper design and implementation of an innovative patient-centered care system has to pay attention to the collection of the right kind of clinical and patient-reported data. If the data are not correctly specified or quantified, they will not be used properly no matter how much data you have generated. In other words, data driven activities will not be fruitful without the determination of their theoretical relevance. It is the integration of inductive and
Thomas T.H. Wan. / Convergence of Artificial Intelligence Research in Healthcare: Trends and Approaches 3
deductive logics in the conduct of scientific inquiry that enables us to develop some forms of predictive medicine or precision medicine. The confirmatory nature of data-driven effort could solidify supportive and foundational theories to guide us in designing more efficacious or efficient delivery system. Hence, we could formulate clinical and administrative decision support products for enhancing patient care management.
2. Current Trends in AI Healthcare Research
AI research in healthcare emerges into a high-growth area of medical enterprises. Attention to practice standards and self-reported care outcomes in both inpatient and outpatient care settings offers rewarding benefits for improving the quality of care.
A few trends in AI healthcare and applications are worthy of noting here. First, the world’s population is aging at a rapid rate. The compression of morbidity and mortality has signified the need to design useful care management strategies for the chronically ill. The call for attention to population health management for poly chronic conditions as a systematic approach is timely in response to the potential needs of the aging population (Wan, 2018). Second, the decline in population growth engenders a significant dilemma for future economic development and growth as it is manifested in the shortage of labor. The shift of caregiving responsibilities towards finding formal caregivers to take care of our elderly is a modern phenomenon. Third, it is very fashionable to advocate the need for delivering patient-centered care, but the substantive meaning of patient-centered care has yet to be better understood. The three-prong questions are: 1) What is patient-centric care? 2) How do we incorporate the principles of considering personal or patient experiences into the design of AI products for healthcare? 3) What types and generations of information technology are available for supporting healthcare organizations in solving the delivery problems?
Strategically speaking, we should start our exploratory journey in search of AI solutions by looking for low-hanging fruits. By employing low-tech strategies in the initial phase, we could find out what's known about the effects of human experience in the healing process. For example, a large hospital in Florida faces a situation of paying millions of dollars in annual fines as a penalty for having higher readmission rates than the national average for heart failure and other chronic conditions. The Centers for Medicare and Medicaid Services (CMS) uses the annual average rate at 15% of hospital readmissions for heart failure as a standard. Higher than the national average rates are therefore liable to pay the penalty in an average of 2 to 5% reduction in reimbursement or payment, depending upon the categories of clinical diagnosis. Under the threat of reducing revenues, all hospitals are very concerned about how to reduce avoidable readmissions for chronic conditions. Naturally, a proper care management strategy is to focus on the determinants of hospital readmission. The literature also suggests that multiple causal factors for readmissions exist. The relative influence of personal, health provider, and institutional factors on hospital readmission has yet to be determined (Wan, 2018). Interestingly enough, empirical studies have also documented that provider characteristics and practice factors (e.g., primary care or clinical integration) may contribute to the variations in hospital readmissions. However, limited research has been focused on in- patient-centric care modalities and their effects on patient readmission.
In response to the need for conducting a thorough investigation on patient or personal care factors influencing the variability in hospitalization or re-hospitalization, a systematic analysis was performed along with meta analysis on the data derived from high-quality published clinical trial studies on heart failure admissions (Wan et al., 2017). A well-trained group of graduate students conducted the systematic review on personal determinants of heart failure and found magic bullets for eliminating or reducing the readmission problem. They identified important personal factors affecting patient variations in heart failure readmission. They learned that human factors involved with patients would help with redesigning or improving care management. Finally, they classified patient-centered factors into an eight-character word, CREATION as an abbreviation of Choice (C), Restfulness (R), healing Environment (E), Activity (A), Trust (T), Interpersonal relations (I), Outlook (O), and Nutrition (N). They found that the Choice factor or self-efficacy has exerted a substantial influence on readmission. When the patient-centered care strategy focuses on a great deal of individual choice or preferences, heart failure patients will be able to reduce the
4 Thomas T.H. Wan. / Convergence of Artificial Intelligence Research in Healthcare: Trends and Approaches
likelihood of readmission in multi-fold than an average heart patient without practicing self-care. The conclusion is that higher priorities should be given to delivering patient educational interventions and raising patient awareness of self-care management, and understanding the interplay among multiple personal factors such as the knowledge (K), motivation (M), attitude (A), preventive practice (P) and patient care outcomes (O). Figure 1 is a behavioral change model with the KMAP-O framework for improving patient adherence levels (Wan et al., 2018). Health practices or preventive activities are directly influenced by improved knowledge, motivation, and attitude toward self-care via patient care education and, in turn, positively affect patient care outcomes. Thus, it confirms the validity in adopting a systematical review and meta-analytic approach to the low-hanging fruit for reducing or avoiding hospital readmissions. By searching for current literature and finding potential causal factors relevant to prevent avoidable hospitalization or re-hospitalization, one can then effectively design patient-centered interventions. Because there are many known multi-tiered approaches involving personal, provider, community, and policy factors, we should recognize the relative influences of determinants of health behavioral change properly when we launch a patient-centered care and educational initiative.
Fig. 1. The KMAP-O framework as a patient-centered health education model
The fourth trend is related to market competition. Every company in AI design and application is trying to produce a device that could dominate the regional, national and/or global market. The Society for Design and Process Science (SDPS) sponsored the 24th International Conference on Navigating Innovative Design and Applications via Automation and Artificial Intelligence (SDPSnet.org) at the end of July of 2019 in Taichung, Taiwan. This conference exemplified the need for convergence of multiple disciplines in order to reshape market niches and facilitate collaborations among varying disciplines in their research and development initiatives. We hope that SDPS colleagues will lead the delivery of AI product design and process research to enable people to effectively adopt health information and knowledge management tools to solve healthcare problems such as hospital readmissions. Because the traditional technology-adoption model is limited in offering insightful ideas about how to improve the efficacy of patient-centered care modality, it is therefore imperative to search for the underlying reasons for those who do not use IT products for patient education and communication. Careful attention is needed to fully understand the reasons for the failure in effective use of health educational products.
The fifth trend relates to looking for ways to achieve multi-criteria optimization. By applying the KMAP-O model as specified for patient-centered care, we are able to collect the right kind of data with proven validity in its theoretical formulation of predictive domains of patient-centered care. Eventually, the data could be warehoused in a defined framework with populated variables in each major domain or conceptual formulation. The availability of big data enables investigators to employ effective data analytics to pursue both exploratory and confirmatory analysis of predictors of healthcare outcomes. Thus, we can maximize the power of knowing and confirming the predictor variables via multi-criteria optimization. Ultimately, decision support systems could be designed and incorporated into AI devices for improving
Thomas T.H. Wan. / Convergence of Artificial Intelligence Research in Healthcare: Trends and Approaches 5
personal health. Through innovation in design-process-outcome science, we hope that we can handle 80% of system problems with AI innovations in healthcare. It would be fascinating to see how clinical practice could be made more efficient and effective by using graphic-user interface (GUI) based decision support systems or other data visualization techniques in healthcare improvement.
The sixth trend is the increasing prevalence of chronic disease in the population. If you ask the elderly over 65 years or older, you may find that an average number of chronic conditions ranges from 2 to 5 chronic illnesses reported by them. Thus, how to target a high-risk population is a major task for researchers in population health management. The population health management perspective emerges as a new enterprise in health care management. By identifying high-risk groups for designing and implementing care management intervention using the AI technology to monitor and collect relevant data, health providers could design and adopt shared decision making apps for their patients in varying settings such as home based, community care, and/or institutional care settings (Wan, 2019).
The seventh trend is to learn how to enhance self-care ability. Patients discharged from an acute care facility should be coordinated and provided with adequate personal care information enabling them to take care of themselves during the post-hospital discharge period. Self-care management plays a very important role in reshaping the patient-first ideology and helping reduce the future health care expenditures.
The eighth trend is the adoption and use of varying health information technologies, particularly related to digital devices, cloud-based mechanisms and blockchain technologies to improving the design and process of healthcare delivery. Furthermore, the emerging data science applied to healthcare and enabled by advanced Internet technologies will greatly speed up data mining and analytics developments. Thus, researchers and practitioners can clearly understand how care management innovations and interventions will effectively impact patient care outcomes. The dose-response relationship between medical care interventions, such as the types and amounts of health education, and outcomes of care could be carefully delineated from the big-data-to-knowledge approach (National Institutes of Health, 2019). Addition, the cost-efficiency and quality of service delivery systems could be substantially improved when the system is able to achieve more effective coordination and timely process medical information or claims. AI via machine learning and optimization is capable to solve healthcare issues and then bend the cost and quality curve.
3. AI Healthcare Research: Directions and Strategies
Several directions and strategies for AI research in healthcare are suggested as follows: First, AI researchers in healthcare should utilize the results from predictive modeling of determinants of
personal health or outcomes. Predictive analytics should not just to rely on a single criterion. By identifying a few parameters parsimoniously, we would be able to optimize the performance and outcomes. In other words, the future is to look beyond the scope of design and process that will be directly influenced by the context or ecology of medical care. We should focus on outcomes and performance as well. This systems approach to healthcare also refers to the context-design-process-outcomes framework guiding the development of AI research.
Second, the convergence in systems science needs to employ causal inquiry approaches via the establishment of theoretical models containing the context-design-process-performance-outcome components of the healthcare system. This causal framework specifies that under specific contexts, a good design leads to a good process, good process leads to good performance, and then good performance helps achieve better patient care outcomes. This is an expanded model of the structural-process-outcome framework specified by Donabedian (1966) for quality improvement.
Third, a multi-tiered approach to healing environment design is suggested. Figure 2 displays a complex causal model of the determinants of health care outcomes. The endpoint is a holistic state of physical and mental wellbeing achievable through improving the healthcare delivery system and its performance. With adequate levels of inputs and outputs used in the healthcare system, the patient-centered care modality is integrated into the design. Evidence-based design in healing environments can exert important positive effects, including the reduction of stress and risk, improvement of patient safety, reduction of airborne
6 Thomas T.H. Wan. / Convergence of Artificial Intelligence Research in Healthcare: Trends and Approaches
pathogens and hospital acquired infections, avoidance of transfer patients induced errors, and enhancement of staff satisfaction and productivity (Ulrich et al., 2004; Douglas and Douglas, 2005; and Huisman et al, 2012). Furthermore, the systematic design has to consider the context or environment in which patient care is affected by cultural, political, social and physical environmental factors. The appropriate designs and processes of care management or population health management enable to maximize or optimize performance of a healthcare system.
Fig. 2. Holistic well-being affected by input- and output components of the healthcare system and person-
centric experience
Fourth, data science seeks the patterns and causal mechanisms associated with the observation (Ertas, Tanik, & Maxwell, 2000). We should effectively guide the development of theoretical foundations that enable the formulation of best practices in healing environment design. A transdisciplinary approach, combining micro- and macro-predictor variables, is highly recommended. This will widen the scope of research activities beyond the engineering or system domains. For instance, the empirical examination of personal and societal determinants of health should specify the relevance of micro- and macro-level predictors in a search for their causal influences on personal and population health. The micro-level factors may include KMAP-O components of health behavioral change, whereas the macro-level factors may consider the contextual, ecological, and organizational variabilities in the conduct of health services research. The big-data research in clinical practices could benefit from the integration of a multi-tiered approach with multi-level modeling and analysis (Wan, 2002). For instance, researchers can populate relevant micro- and macro-level predictor variables based on the conceptual formulation or model. Therefore, domain-specific information is organized and integrated into a theoretically sound data system defined by the investigators (Figure 3). Then, we will be able to tease out the relevance of system components in designing predictive analytics. The usefulness of exploratory and confirmatory approaches of data science should not be based on the hit and miss trials in search for important determinants of health, but they are theoretically guided investigations to identify action plans and directions of interventions. By considering predictive variables in a causal sequence, one can begin to develop useful predictive models in healthcare (Figure 3). We can then explain fully what we have gained from the data analysis via predictive
Thomas T.H. Wan. / Convergence of Artificial Intelligence Research in Healthcare: Trends and Approaches 7
modeling. Ultimately, we can design and implement decision support systems for optimizing health care outcomes, such as reduced hospital readmissions.
Fig. 3. Micro- and macro-level predictors and integration serving as a theoretical framework to guide the
design of predictive analytics
Fifth, the utilization of Internet-of-Things (IoT) technologies in healthcare offers researchers to connect with smart devices and data with Internet and identify relevant information for improving the healthcare quality (Dauwed and Meri, 2019). In a recent literature review, Naziv et al. (2019) examined varying sources of publications and workshops and identified concerns such as data connectedness, standardization, and security and privacy of data compiled by mobile health technologies. These issues are the challenges encountered by researchers as well as providers.
Sixth, value-based approaches to healthcare management are highlighted in prior research (Wan, 2002; Shortell et al., 2007; Lee and Wan, 2002; Wan, 2018). For instance, the increased technical efficiency of hospital care is positively associated with the improved quality of care. The relationship between efficiency and quality of care is a complimentary rather than a substitutive one. A recent hospital research report suggests that hospital standardization in the design of an automated care management system facilitates the effectiveness in targeting high-risk populations through a systematic risk identification (Shettian and Wan, 2018). Similarly, population health management could be enhanced by integrating activities such as risk identification, utilization, quality, and patient engagement management.
Seventh, longitudinal data and prospective study design are germane to the search for causal factors influencing care management effectiveness. Because the conventional approach to health data analysis does not observe patient states longitudinally in multiple time points with repeated measures, the static nature of patient care data is unable to reveal trajectory patterns of chronic disease and its complications. Sequential
8 Thomas T.H. Wan. / Convergence of Artificial Intelligence Research in Healthcare: Trends and Approaches
data of patient care status with both time-varying and time-constant variables together should capture any changes in the panel data system (Wan, 2017). Hence, we can develop meaningful and useful predictive analytics for identifying determinants of health or illness (Figure 4).
Fig. 4. Panel data needed in predictive analytics
4. Implementing Patient-Centered Care Management Technologies for Solving Problems in the Health Services Delivery System: A Proposal for AI Research
Body Text Wellness and preventive care may be improved through proper design and implementation of a patient-centered care management technology (PCCMT). Little is known about how an ideal care management technology can be applied to community-based wellness centers. Research has shown that increased patient-clinician communication is correlated to higher levels of patient satisfaction and improved health outcomes (Breen et al., 2009). The synergism of employing personal health records (PHR) and health information technology (HIT) in wellness centers may play a pivotal role for enhancing collaborative patient care and increasing patient safety and quality of care. It is also unclear if the PHR, augmented with a sound education training program, can reduce risks associated with medical errors in ambulatory care, improve patient-clinician communication, increase continuity of patient-centered care, and generate better proximal outcomes (patient and provider satisfaction, trust) and distal outcomes (health-related quality of life and health status).
In implementing the PCCMT, we need to identify barriers and benefits of PCCMT for participants, providers, wellness centers and the community. To evaluate the beneficial effects of the patient-centric care management technology (PCCMT) interventions, we propose to adopt the following: 1) Personal Health Records (PHR), 2) participant health education interventions, and 3) integration of PHR technologies with care coordination, lifestyle change and nutritional review, and preventive care processes and outcomes measured by indicators such as improvement of interpersonal continuity of care, patient-provider communication, patient adherence to prescribed treatment regimen, appropriate use of healthcare resources, participant satisfaction, adverse drug events detected by pharmacy consultation, health related quality of life (HRQOL), and health status measures.
Overall improvement in patient safety, using health information technologies (HIT) has been made (Bates and Singh, 2018; Bates and Bitton, 2010). However, the integration of electronic health records (EHR) into personal health records (PHR) has not been made to benefit the patient directly, particularly in the design of shared clinical decision making software. Relieving critical symptoms of the larger healthcare system failure requires a more comprehensive, dynamic intervention. Further protection of patient safety and ultimately, health system safety, requires attention to the broader scope of the root problem. Focus on better management and utilization of informatics must be employed at the heart of patient-centered delivery of care called PCCMT. This expanded approach to HIT is k
Collepals.com Plagiarism Free Papers
Are you looking for custom essay writing service or even dissertation writing services? Just request for our write my paper service, and we'll match you with the best essay writer in your subject! With an exceptional team of professional academic experts in a wide range of subjects, we can guarantee you an unrivaled quality of custom-written papers.
Get ZERO PLAGIARISM, HUMAN WRITTEN ESSAYS
Why Hire Collepals.com writers to do your paper?
Quality- We are experienced and have access to ample research materials.
We write plagiarism Free Content
Confidential- We never share or sell your personal information to third parties.
Support-Chat with us today! We are always waiting to answer all your questions.
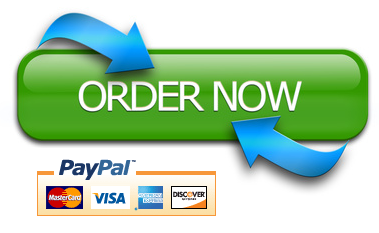