Part of your task as a scholar-practitioner is to act as a critical consumer of research and ask informed questions of published material. Sometimes, claims
Part of your task as a scholar-practitioner is to act as a critical consumer of research and ask informed questions of published material. Sometimes, claims are made that do not match the results of the analysis.
Assignment: Evaluating Significance of Findings
Part of your task as a scholar-practitioner is to act as a critical consumer of research and ask informed questions of published material. Sometimes, claims are made that do not match the results of the analysis. Unfortunately, this is why statistics is sometimes unfairly associated with telling lies. These misalignments might not be solely attributable to statistical nonsense, but also “user error.” One of the greatest areas of user error is within the practice of hypothesis testing and interpreting statistical significance. As you continue to consume research, be sure and read everything with a critical eye and call out statements that do not match the results.
For this Assignment, you will examine statistical significance and meaningfulness based on sample statements.
To prepare for this Assignment:
· Review the Week 5 Scenarios found in this week’s Learning Resources and select two of the four scenarios for this Assignment.
· For additional support, review the Skill Builder: Evaluating P Values and the Skill Builder: Statistical Power, which you can find by navigating back to your Blackboard Course Home Page. From there, locate the Skill Builder link in the left navigation pane.
For this Assignment:
Critically evaluate the two scenarios you selected based upon the following points:
· Critically evaluate the sample size.
· Critically evaluate the statements for meaningfulness.
· Critically evaluate the statements for statistical significance.
· Based on your evaluation, provide an explanation of the implications for social change.
Use proper APA format and citations, and referencing.
https://www.amstat.org/asa/files/pdfs/p-valuestatement.pdf
Frankfort-Nachmias, C., Leon-Guerrero, A., & Davis, G. (2020). Social statistics for a diverse society (9th ed.). Thousand Oaks, CA: Sage Publications.
· Chapter 8, “Testing Hypothesis: Assumptions of Statistical Hypothesis Testing” (pp. 241-242)
Wagner, III, W. E. (2020). Using IBM® SPSS® statistics for research methods and social science statistics (7th ed.). Thousand Oaks, CA: Sage Publications.
· Chapter 6, “Testing Hypotheses Using Means and Cross-Tabulation”
Walden University, LLC. (Producer). (2016f). Meaningfulness vs. statistical significance [Video file]. Baltimore, MD: Author.
Note: The approximate length of this media piece is 4 minutes.
In this media program, Dr. Matt Jones discusses the differences in meaningfulness and statistical significance. Focus on how this information will inform your Discussion and Assignment for this week.
Skill builder: Evaluating P Values
Hypothesis Testing
In doing research, one of the most common activities is testing hypotheses. The Afrobarometer data set below is a survey of African citizens’ attitudes on democracy, governance, the economy, and other related topics ( www.afrobarometer.org ). Using this data set, you might want to examine hypotheses related to whether rural and urban citizens differ, on average, in how much they trust the government. The tables below present results from an independent samples t-test to examine these hypotheses using a random sample of 44 participants from the complete data set. Each respondent’s score is a value between 0 and 15 with a higher score indicating greater trust. You can see that the mean for the urban group is 7.00 ( SD = 4.17) and the mean for the rural group is 7.74 ( SD = 4.38). The observed value of the t-statistic is -.564 and the p-value equals 0.576 (see the column labeled “Sig. (2-tailed)”).
African Citizens' Attitudes on Democracy
The tables below present results from an independent samples t-test to examine these hypotheses using a random sample of 44 participants from the complete data set. Each respondent’s score is a value between 0 and 15 with a higher score indicating greater trust. You can see that the mean for the urban group is 7.00 ( SD = 4.17) and the mean for the rural group is 7.74 ( SD = 4.38). The observed value of the t-statistic is -.564 and the p-value equals 0.576 (see the column labeled “Sig. (2-tailed)”).
t |
df |
Sig. (2-tailed) |
Mean Difference |
Std. Error Difference |
|
Trust in Government Index (higher scores = more trust) |
-.564 |
41 |
.576 |
-.73913 |
1.30978 |
Group Statistics
Urban or Rural Primary Sampling Unit |
N |
Mean |
Std. Deviation |
Std. Error Mean |
|
Trust in Government Index (higher scores = more trust) |
Urban |
20 |
7.000 |
4.16754 |
.93189 |
Rural |
30 |
7.7391 |
4.38196 |
.91370 |
The p-value is the probability of obtaining a value more extreme than .564 (less than -.564 or greater than +.564) if you were to repeat the test with a new sample of data and if the null hypothesis is true. You will see in this Skill Builder that the p-value can easily be used to make statistical decisions in hypothesis testing. However, while the p-value is important in determining statistical significance, it does not tell the whole story.
Steps of Hypothesis Testing
To interpret p-values, let's review the key steps in hypothesis testing. Use the < and > icons to navigate between the steps.
Step 1
State the null and alternative hypotheses
Recall that hypotheses are statements about population parameters. For the Trust in Government example from the Afrobarometer data set, the null (HO) and alternative hypotheses (HA) is seen in the above image.
The Greek letter, µ, indicates a population mean, and the subscripts indicate levels of the independent variable (“urban” and “rural”). Here the null is saying that the mean for the urban population on the Trust In Government variable is the same as the mean for the rural population. The alternative hypothesis states that these means are not the same.
Step 2
Set alpha , the probability of a type I error
Frequently, the value of alpha is set equal to 0.05, although researchers are free to use other values. If using an alpha of .05, then researchers are specifying that there is a 5% chance that they will reject the null when, in fact, it should not be rejected. Setting alpha at .05 is popular because there is relatively minimal risk of making a type I error, and alpha is not so small that researchers greatly increase their risk of not rejecting the null when they actually should (a type II error). So in setting alpha, researchers have to be aware of both the risk of rejecting the null erroneously and of not rejecting it when they actually should. For our Afrobarometer example, we will set alpha at .05.
Step 1
State the null and alternative hypotheses
Recall that hypotheses are statements about population parameters. For the Trust in Government example from the Afrobarometer data set, the null (HO) and alternative hypotheses (HA) is seen in the above image.
The Greek letter, µ, indicates a population mean, and the subscripts indicate levels of the independent variable (“urban” and “rural”). Here the null is saying that the mean for the urban population on the Trust In Government variable is the same as the mean for the rural population. The alternative hypothesis states that these means are not the same.
Step 2
Set alpha , the probability of a type I error
Frequently, the value of alpha is set equal to 0.05, although researchers are free to use other values. If using an alpha of .05, then researchers are specifying that there is a 5% chance that they will reject the null when, in fact, it should not be rejected. Setting alpha at .05 is popular because there is relatively minimal risk of making a type I error, and alpha is not so small that researchers greatly increase their risk of not rejecting the null when they actually should (a type II error). So in setting alpha, researchers have to be aware of both the risk of rejecting the null erroneously and of not rejecting it when they actually should. For our Afrobarometer example, we will set alpha at .05.
Step 3
Decide on a test statistic
Because of a desire to compare two groups (rural and urban), a t-test for two independent samples is being used.
Step 1
State the null and alternative hypotheses
Recall that hypotheses are statements about population parameters. For the Trust in Government example from the Afrobarometer data set, the null (HO) and alternative hypotheses (HA) is seen in the above image.
The Greek letter, µ, indicates a population mean, and the subscripts indicate levels of the independent variable (“urban” and “rural”). Here the null is saying that the mean for the urban population on the Trust In Government variable is the same as the mean for the rural population. The alternative hypothesis states that these means are not the same.
Step 2
Set alpha , the probability of a type I error
Frequently, the value of alpha is set equal to 0.05, although researchers are free to use other values. If using an alpha of .05, then researchers are specifying that there is a 5% chance that they will reject the null when, in fact, it should not be rejected. Setting alpha at .05 is popular because there is relatively minimal risk of making a type I error, and alpha is not so small that researchers greatly increase their risk of not rejecting the null when they actually should (a type II error). So in setting alpha, researchers have to be aware of both the risk of rejecting the null erroneously and of not rejecting it when they actually should. For our Afrobarometer example, we will set alpha at .05.
Step 3
Decide on a test statistic
Because of a desire to compare two groups (rural and urban), a t-test for two independent samples is being used.
7
Step 4
Collect the data and examine the model assumptions
Before calculating the value for your test statistic, be sure you have checked assumptions, like homogeneity of variance and the absence of outliers.
Step 5
Calculate the observed value of the test statistic
Once the data have been collected, the observed value of the test statistic will be used to make a statistical decision. In the Afrobarometer example, the observed value of the test statistic is -.564, sometimes written as tobserved(41)= −.564 where the 41 is the number of degrees of freedom associated with the test.
Step 1
State the null and alternative hypotheses
Recall that hypotheses are statements about population parameters. For the Trust in Government example from the Afrobarometer data set, the null (HO) and alternative hypotheses (HA) is seen in the above image.
The Greek letter, µ, indicates a population mean, and the subscripts indicate levels of the independent variable (“urban” and “rural”). Here the null is saying that the mean for the urban population on the Trust In Government variable is the same as the mean for the rural population. The alternative hypothesis states that these means are not the same.
Step 2
Set alpha , the probability of a type I error
Frequently, the value of alpha is set equal to 0.05, although researchers are free to use other values. If using an alpha of .05, then researchers are specifying that there is a 5% chance that they will reject the null when, in fact, it should not be rejected. Setting alpha at .05 is popular because there is relatively minimal risk of making a type I error, and alpha is not so small that researchers greatly increase their risk of not rejecting the null when they actually should (a type II error). So in setting alpha, researchers have to be aware of both the risk of rejecting the null erroneously and of not rejecting it when they actually should. For our Afrobarometer example, we will set alpha at .05.
Step 3
Decide on a test statistic
Because of a desire to compare two groups (rural and urban), a t-test for two independent samples is being used.
Step 4
Collect the data and examine the model assumptions
Before calculating the value for your test statistic, be sure you have checked assumptions, like homogeneity of variance and the absence of outliers.
Step 5
Calculate the observed value of the test statistic
Once the data have been collected, the observed value of the test statistic will be used to make a statistical decision. In the Afrobarometer example, the observed value of the test statistic is -.564, sometimes written as tobserved(41)= −.564 where the 41 is the number of degrees of freedom associated with the test.
Step 6
Make a statistical decision using the observed value
This decision requires examining the distribution of the test statistic under the assumption the null hypothesis is true. Practically, the area in the tail of the distribution beyond the observed value of the test statistic, called the p-value, needs to be determined (see the figure above). Fortunately, computer programs can do the calculation of the area quickly and easily. If the probability is less than alpha (e.g., .05), we will reject the null hypothesis. Thus, if you set alpha equal to .05 and the p-value for your test statistic is any value less than .05, you will reject the null hypothesis. Otherwise, retain the null.
Step 7
Make a real-world decision
The statistical decision is focused on the abstract hypothesis test. The final step is to examine the implications of the statistical decision in the real world. You will need to consider whether your results are practically significant. It turns out that not all statistically significant results are important in the real world. We will discuss more about this later in the Skill Builder.
Skill Builder: Statistical Power,
Statistical Power
Statistical power is the probability of rejecting a null hypothesis if the null is false (i.e., the alternative is true). It is the degree to which the researcher is able to detect an effect if there actually is one. With low statistical power, a researcher may struggle to detect an effect (to reject the null), even if an effect actually occurs in the population.
Suppose you are planning an experiment involving stereotype threat. Stereotype threat is defined as a tendency to behave in a manner consistent with negative beliefs that others have about a racial or gender group. For example, if some black test takers are told that as a group, black test takers do not perform well on math tests, performance among those black test-takers is worse than for black test takers for whom the stereotype is not evoked. One question you will need to answer is how many participants should you include in your study to be confident in identifying the effect? In other words, how many participants do you need in order to have adequate statistical power in your study?
The Affect of Statistical Power
Understanding how several factors affect the statistical power of a study will help you to understand and critique research findings and will also lead to greater satisfaction with your own research. When conducting your own research studies, you should do a power analysis prior to collecting data to make sure you have a good chance of demonstrating the effect you are looking for.
There are three main factors that affect how much statistical power you have in your study:
· 1
1
Alpha (i.e., the probability of a type I error)
· 2
2
Effect size (i.e., the difference between the population means for the experimental and control groups)
· 3
3
Sample size (i.e., n )
As a researcher, you have control over alpha and sample size. The effect size, however, is not under your control and is predetermined. What will be important to you is having an idea about how great the effect may be. This Skill Builder is concerned with how alpha, effect size, and sample size are related to statistical power.
A Review of Hypothesis Testing
Before discussing power, let’s review the basics of hypothesis testing:
· bullet
The null hypothesis is the statement of no effect.
· bullet
The alternative hypothesis is a statement that an effect exists in the population.
· bullet
Obtaining a significant result means that you have rejected the null hypothesis and have concluded that it’s likely that there is an effect in the population.
· bullet
A type I error happens when the null hypothesis is true but you reject it erroneously. This is referred to as a false positive.
· bullet
A type II error happens when the null hypothesis is false but you fail to reject it. This is referred to as a false negative.
Reviewing Type I and Type II Errors
Type I and type II errors and their probabilities are important concepts when thinking about hypothesis testing. These error events are called “conditional,” meaning that the events can only occur under certain conditions.
The following is the language that is used to talk about these conditional events:
· Alpha (α) = P(type I error) = P(Reject H 0 |H 0 is true) which is read as the probability of a type I error equals the probability of rejecting the null hypothesis given the null is true.
· Beta (β) = P(type II error) = P(Retain H 0 |H A is true) which is read as the probability of a type II error equals the probability of retaining the null hypothesis given the alternative hypothesis is true.
Table 1 shows the possible outcomes for a hypothesis test.
Table 1: Possible Outcomes for a Hypothesis Test
D |
True State of Nature |
|
Decision |
Ho is true |
Ho is false |
Retain Ho |
Correct decision |
Type II error |
Reject Ho |
Type 1 error |
Correct decision |
Power Analysis
Power analysis is the process of examining a test of the null hypothesis to determine the chances of rejecting it and placing belief in the alternative hypothesis.
Researchers typically want to get a sense of how much statistical power they will have in their study before collecting data. In order to do so, they usually conduct a power analysis. Suppose you design a study, and a part of it is to demonstrate stereotype threat involving females. Nguyen and Ryan (2008) provide results that indicate the average Cohen’s d in previous studies of gender-based stereotype threat for cognitive tests is about .21. This means that over many studies, females who are NOT made aware of a gender stereotype (NOT primed) score about 0.2 standard deviations higher on cognitive tests than females who are made aware of a gender effect (primed). To demonstrate this effect in your study, you will test the following null hypothesis:
HA : μNOT primed − μprimed ≤ 0
If you reject the null, you will place your confidence in the following alternative hypothesis:
HA : μNOT primed − μprimed > 0
μNOT primed Indicates the population mean for the “not primed” condition.
μprimed Indicates the population mean for the “primed” condition.
HA : μNOT primed − μprimed > 0 The alternative hypothesis specifies that the “not primed” condition will score higher than the “primed” condition.
To test this null hypothesis, you would examine a test statistic distribution and note the area in the upper tail of the distribution equal to alpha. Suppose you plan to test this hypothesis with a t-test with 50 participants in each condition (primed or NOT primed).
Figure 1 sampling distribution shows what you should expect for the values of the test statistic if the null hypothesis is true. In order to reject the null hypothesis, the t value would need to be greater than 1.66055.
Figure 1
Because the test statistic is a continuous variable, the curve shows probability density, and probability is found by determining the area under the curve.
The entire area under the curve, between – ∞ and + ∞ , is 1.00.
To find the probability of a statistic taking on a value within a certain range, you need to find the area under the curve within the range. For example, there are tables that will tell you that the area under the curve between t = 0 and t = +1 corresponds to a probability of about .34. Most importantly, because alpha has been set equal to .05, the area b
Collepals.com Plagiarism Free Papers
Are you looking for custom essay writing service or even dissertation writing services? Just request for our write my paper service, and we'll match you with the best essay writer in your subject! With an exceptional team of professional academic experts in a wide range of subjects, we can guarantee you an unrivaled quality of custom-written papers.
Get ZERO PLAGIARISM, HUMAN WRITTEN ESSAYS
Why Hire Collepals.com writers to do your paper?
Quality- We are experienced and have access to ample research materials.
We write plagiarism Free Content
Confidential- We never share or sell your personal information to third parties.
Support-Chat with us today! We are always waiting to answer all your questions.
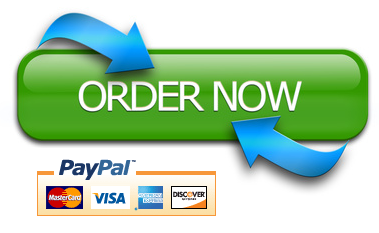