Jane, a military psychologist, wants to examine two types of treatments for depression in a group of military personnel who have suffered the loss o
Jane, a military psychologist, wants to examine two types of treatments for depression in a group of military personnel who have suffered the loss of their legs. She has only 20 men to work with.
- What would be the best research design for the study and why?
- What are some issues that Jane needs to consider before starting the study?
- What is a longitudinal study? What are the benefits and challenges associated with a longitudinal study?
- Using the Online Library find two peer-reviewed articles (one that has used a between study design and one that has used a within study design) Summarize both of these articles. Make sure you discuss the research design specifically.
- Explain what practice and carryover effects are in the context of the within subjects design study that you found. What steps did the researchers take to reduce these effects?
Justify your answers with appropriate reasoning and research
Methods for Policy Analysis Rebecca A. Maynard, Editor
Kenneth A. Couch, Guest Editor
Authors who wish to submit manuscripts for all sections except Book Reviews should do so electronically in PDF format through Editorial Express.
STRENGTHENING THE REGRESSION DISCONTINUITY DESIGN USING ADDITIONAL DESIGN ELEMENTS: A WITHIN-STUDY COMPARISON
Coady Wing and Thomas D. Cook
Abstract
The sharp regression discontinuity design (RDD) has three key weaknesses compared to the randomized clinical trial (RCT). It has lower statistical power, it is more de- pendent on statistical modeling assumptions, and its treatment effect estimates are limited to the narrow subpopulation of cases immediately around the cutoff, which is rarely of direct scientific or policy interest. This paper examines how adding an un- treated comparison to the basic RDD structure can mitigate these three problems. In the example we present, pretest observations on the posttest outcome measure are used to form a comparison RDD function. To assess its performance as a sup- plement to the basic RDD, we designed a within-study comparison that compares causal estimates and their standard errors for (1) the basic posttest-only RDD, (2) a pretest-supplemented RDD, and (3) an RCT chosen to serve as the causal bench- mark. The two RDD designs are constructed from the RCT, and all analyses are replicated with three different assignment cutoffs in three American states. The re- sults show that adding the pretest makes functional form assumptions more trans- parent. It also produces causal estimates that are more precise than in the posttest- only RDD, but that are nonetheless larger than in the RCT. Neither RDD version shows much bias at the cutoff, and the pretest-supplemented RDD produces causal effects in the region beyond the cutoff that are very similar to the RCT estimates for that same region. Thus, the pretest-supplemented RDD improves on the standard RDD in multiple ways that bring causal estimates and their standard errors closer to
Journal of Policy Analysis and Management, Vol. 32, No. 4, 853–877 (2013) C© 2013 by the Association for Public Policy Analysis and Management Published by Wiley Periodicals, Inc. View this article online at wileyonlinelibrary.com/journal/pam DOI:10.1002/pam.21721
854 / Methods for Policy Analysis
those of an RCT, not just at the cutoff, but also away from it. C© 2013 by the Association for Public Policy Analysis and Management.
INTRODUCTION
A carefully executed regression discontinuity design (RDD) is now widely considered a sound basis for causal inference. The design was introduced in Thistlewaite and Campbell (1960), and Goldberger (1972a, 1972b) showed that RDD produces causal estimates that are unbiased, but less efficient than those produced by a comparable randomized clinical trial (RCT). Recent work has clarified the assumptions that support parametric and nonparametric identification in the RDD (Hahn, Todd, & Van der Klauuw, 2001; Lee, 2008), and has examined the statistical properties of common estimators (Lee & Card, 2008; Porter, 2003; Schochet, 2009). In addition, a growing literature compares RDD estimates to benchmark estimates from an RCT, and these within-study comparisons show that RDD and RCT estimates have been similar in various applied settings (Cook & Wong, 2008; Green et al., 2009; Shadish et al., 2011). Despite this recent work, the basic elements of the design have not changed. An RDD requires an outcome variable, a binary treatment, a continuous assignment variable, and a cutoff-based treatment assignment rule. The assignment rule is crucial: In a successful RDD, individuals with assignment scores on one side of the cutoff receive one treatment and individuals on the other side receive another treatment, usually a no-treatment control condition. An RDD is sharp when all individuals receive the intended treatment, and it is fuzzy when compliance is partial. This paper deals only with sharp RDD studies.
The analysis of an RDD is not complicated in principle. Researchers estimate treat- ment effects by comparing mean outcomes among people with assignment scores immediately below and immediately above the cutoff. The difference between these two conditional means can be understood as a discontinuity in the regression func- tion that links average outcomes across subpopulations defined by the assignment variable. A basic assumption in the RDD is that in the absence of a treatment effect, the regression would be a smooth function near the cutoff; conversely, a sudden break or discontinuity at the cutoff is evidence of a treatment effect. The size of the discontinuity measures the magnitude of the effect.
The RDD has at least three important limitations relative to an RCT. The first involves the amount of statistical modeling required to identify and estimate causal effects. In an RCT, treatment effects are nonparametrically identified so that as- sumptions about the underlying statistical model are not required to interpret the data. Moreover, there is usually a close connection between the research design and the statistical tools used to perform the analysis.1 In RDD, on the other hand, treatment effects are nonparametrically identified, but fully nonparametric anal- ysis requires very large sample sizes that cannot always be attained. In practice, researchers often proceed by specifying a parametric or semiparametric functional form of the regression and allowing for an intercept shift at the cutoff (Lee & Card, 2008). Choosing the wrong functional form can lead to biased treatment effect estimates, so it is good practice for analysts to use flexible methods to estimate functional forms before evaluating how sensitive the results are to alternative speci- fications. Although many techniques for sensitivity analysis exist, it would be a boon
1 Of course, analysts often employ parametric regression models in the analysis of experimental data either to improve the statistical precision of the treatment effect estimates or to adjust for chance imbalances in observable covariates. But this additional modeling is usually not central to the study’s findings.
Journal of Policy Analysis and Management DOI: 10.1002/pam Published on behalf of the Association for Public Policy Analysis and Management
Methods for Policy Analysis / 855
in RDD studies to have better methods for validating functional form assumptions. We present one such method here.
A second limitation of standard RDD is that treatment effect estimates are less statistically precise than in an RCT, reducing the statistical power of key hypothesis tests (Goldberger, 1972b; Schochet, 2009). Some of the efficiency loss is due to the multicollinearity between assignment scores and treatment variable that is inherent in the RDD assignment rule. RDD estimates that rely on nonparametric estima- tion methods may also have lower power because they employ a bandwidth that decreases the study’s effective sample size. Lower statistical power is a secondary concern in RDD studies with large administrative databases, but it is more central when investigators prospectively design a study and collect their own data directly from respondents. In this last circumstance, adding more cases may be costly and tempt researchers into favoring alternative designs with greater power, but a weaker identification strategy (Schochet, 2009).
A third limitation concerns the generality of RDD results. RCTs produce treatment effect estimates averaged across all members of the study population. In contrast, RDD estimates are limited to average treatment effects among members of the narrow subpopulation located immediately around the cutoff. For example, if a treatment is given to students scoring above the 75th percentile on an achievement test, then RDD results can only be generalized to students near that point. Unfor- tunately, social science and public policy debates usually are concerned with the effects of treatments in broader subpopulations, such as all students, or all students in the upper quartile of the test score distribution. Constructing estimates of these more general parameters in an RDD setting requires making extrapolations beyond the cutoff score. Researchers often are reluctant to make such extrapolations be- cause there is rarely a firm theoretical basis for the assumption that the functional form of the regression is stable beyond the range of the observed data. The crux of the problem is that no one knows what the treatment group functional form would have looked like in the absence of the treatment. The absence of this counterfactual regression function is why it is standard practice to limit causal inferences to the cutoff subpopulation, even though this narrow applicability of the estimates reduces the value of the standard RDD as a practical method for policy analysis (Manski, 2013).
This paper explores an RDD variant that can improve on all three of these limita- tions. It requires supplementing the conventional posttest-only RDD with a pretest measure of the outcome variable. In what follows, we refer to the conventional RDD as a “posttest RDD” because it only requires posttest information. We refer to the pretest-supplemented design as a “pretest RDD,” noting that it makes use of both pretest and posttest outcome data. The key idea is that the pretest data provides information about what the regression function linking outcomes and assignment scores looked like in the absence of the treatment in an earlier time period. If the functions are stable over time, then the pretest data can inform the analysis of the posttest data. Minor differences between the pretest and posttest functional forms in the untreated part of the assignment variable, such as intercept differences, are easily accommodated. But functional forms that are observed to be very dissimilar over time in the untreated part of the assignment variable would cast doubt on the results of a pretest-supplemented RDD.
The core of this paper is a within-study comparison that evaluates the performance of the pretest and posttest RDDs relative to each other and to a benchmark RCT. LaLonde (1986) and Fraker and Maynard (1987) were the first to use this method to examine whether econometric adjustments for selection bias in an observational study could reproduce the results of job-training RCTs. Since then, researchers have used the method to study the performance of RDD (Green et al., 2009; Shadish et al., 2011), intact group and individual case matching (Bifulco, 2012; Cook, Shadish, &
Journal of Policy Analysis and Management DOI: 10.1002/pam Published on behalf of the Association for Public Policy Analysis and Management
856 / Methods for Policy Analysis
Wong, 2008; Wilde & Hollister, 2007), and alternative strategies for covariate se- lection (Cook & Steiner, 2010). The implementation details of within-study com- parisons vary, but the basic idea is always to test the validity of a nonexperimental method by comparing its estimates to a trustworthy benchmark from an RCT. Meth- ods for conducting a high-quality within-study comparison have evolved over time, and Cook, Shadish, and Wong (2008) describe the current best practices that we follow in this paper.
Our within-study comparison is based on data from the Cash and Counseling Demonstration Experiment (Dale & Brown, 2007). In the original study, disabled Medicaid beneficiaries in Arkansas, Florida, and New Jersey were randomly as- signed to obtain home- and community-based health services through Medicaid (the control group), or to receive a spending account that they could use to procure home- and community-based services directly (the treatment group). The origi- nal study examined the effects of the program on a variety of health, social, and economic outcomes. But for the purposes of our within-study comparison, the outcome variable we focus on is a measure of individual Medicaid expenditures in the 12 months after the study began.
To construct pretest and posttest RDDs from the RCT, we used baseline age as the assignment variable and sorted the RCT treatment-group and control-group cases by baseline age. Then, we defined a cutoff age for treatment assignment, selecting three of them for replication purposes—ages 35, 50, and 70. Next, we systematically deleted control cases from above the cutoff and treatment cases below the cutoff. Since we had data from Florida, New Jersey, and Arkansas, a total of nine posttest and nine pretest RDDs resulted—three age cutoffs crossed with three states. At each age cutoff, we compared the pretest and posttest RDD estimates to each other and to the corresponding RCT estimate. In the pretest RDD, we also used the comparison data to compute an estimate of the average treatment effect for everyone older than the cutoff, which is the average treatment effect on the treated (ATT) parameter that is often of interest in program evaluation research and is usually out of reach in RDD studies. We compared these extrapolated estimates to the corresponding RCT benchmarks.
The results of our analysis indicate that the pretest RDD can shore up all three key weaknesses of the posttest RDD. First, our comparisons show that the pretest and posttest functional forms are similar below the cutoff, thus providing some support for the proposition that the pretest data could be informative about the counterfactual untreated regression function in the posttest period. Second, we found that adding the pretest led to more statistically precise estimates than the conventional posttest RDD, although the estimates are still not quite as precise as in the RCT. And finally, the pretest RDD produced unbiased treatment effects relative to the RCT, not only at the cutoff, but also beyond the cutoff. In the within-study comparison considered in this paper, the multidimensional superiority of the pretest RDD over the posttest RDD is clear.
THE RCT DATA
The Cash and Counseling Demonstration and Evaluation is described in detail else- where (Brown & Dale, 2007; Dale & Brown, 2007a; Doty, Mahoney, & Simon- Rusinowitz, 2007; Carlson et al., 2007). Study participants were disabled elderly and nonelderly adult Medicaid beneficiaries who agreed to participate and lived in Arkansas, New Jersey, or Florida from 1999 to 2003. The study employed a rolling enrollment design in which new enrollees completed a baseline survey and then were randomly assigned to treatment or control status, after which the state agency was informed of the assignments. The treatment condition was a “consumer-directed
Journal of Policy Analysis and Management DOI: 10.1002/pam Published on behalf of the Association for Public Policy Analysis and Management
Methods for Policy Analysis / 857
Table 1. Descriptive statistics for the variables and samples used to form within-study comparisons.
Arkansas Florida New Jersey
Variable Control Treatment Control Treatment Control Treatment
Pretest Medicaid expenditures
$6,358 $6,439 $14,300 $14,377 $18,779 $18,215
Posttest Medicaid expenditures
$7,583 $9,443 $18,088 $19,944 $20,100 $21,299
Mean age 70 70 55 55 62 63
N 1,004 1,004 906 907 869 861
budget” program. It allowed disabled Medicaid beneficiaries to procure their own home- and community-based support services and providers using a Medicaid- financed spending allowance. The control group received home- and community- based support services procured by a local Medicaid agency from Medicaid certified providers, which is the status quo policy. In both groups, Medicaid pays for the ser- vices. The key difference is whether the Medicaid enrollee or the Medicaid agency makes the micro-level spending decisions. In the new program, the personal al- lowance was set to the amount the agency would have allocated in the absence of the new program because the intervention was meant to be revenue neutral. So, the study outcome we analyze—how much was actually spent for services—tests whether individuals or Medicaid officials spent more of the same allocated total.
Our methodological study used a small subset of the measures collected in the original study. For each member of the study, we retained information on age at baseline, state of residence, and randomly assigned treatment status. We created a measure of annual Medicaid expenditures by adding up six categories of monthly expenditures across the 12 months before random assignment (pretest) and after random assignment (posttest). The six expenditure categories were Inpatient Ex- penditures, Diagnosis-Related Group Expenditures, Skilled Nursing Expenditures, Personal Assistance Services Expenditures, Home Health Services Expenditures, and Other Services Expenditures.2 Throughout, we refer to this six-item index as “Medicaid expenditures,” and it is the sole outcome in our study.
The summary statistics in Table 1 show that in the RCT, Arkansas had 1,004 par- ticipants in each of the treatment and control arms, Florida had 906 control and 907 treatment participants, and New Jersey had 869 control and 861 treatment-group members. In Arkansas, the average participant was 70 years old, compared to 55 in Florida, and 62 in New Jersey. Within each state, average pretest expenditures were similar in the treatment and control groups, but the level of spending varied by state. The average person in Arkansas had pretest expenditures of $6,400 compared to $14,300 in Florida and $18,500 in New Jersey. Mean posttest expenditures were consistently higher in the treatment groups. Simple intent-to-treat (ITT) compar- isons imply that the intervention increased average expenditures by about $1,860
2 The claims data included a small number of cases with very high levels of expenditures that could be either real or data entry errors. To reduce concerns that these outliers would skew our regression estimates, we top coded the pretest and posttest Medicaid expenditures variable at the 99th percentile of the pooled distribution of posttest expenditures, which was equal to $78,273. The top coding procedure affected 89 posttest observations and 79 pretest observations.
Journal of Policy Analysis and Management DOI: 10.1002/pam Published on behalf of the Association for Public Policy Analysis and Management
858 / Methods for Policy Analysis
Table 2. Sample sizes in the nine constructed posttest regression discontinuity designs.
State Age cutoff Below the cutoff Above the cutoff Total
Arkansas 35 59 944 1003 Florida 35 296 609 905 New Jersey 35 106 770 876
Arkansas 50 143 868 1011 Florida 50 417 496 913 New Jersey 50 224 650 874
Arkansas 70 361 623 984 Florida 70 555 359 914 New Jersey 70 491 387 878
(P < 0.01) in Arkansas, $1,856 (P = 0.01) in Florida, and $1,200 (P = 0.09) in New Jersey. Thus, the Cash and Counseling treatment increased Medicaid expenditures relative to when Medicaid officials controlled the expenditures.
WITHIN-STUDY RESEARCH DESIGN
To implement the within-study comparison, we created 21 different subsets of the original RCT data. The first three are the state-specific RCT treatment and control groups, for which sample sizes and basic descriptive statistics are in Table 1. The next nine subsets represent state and age specific posttest RDDs based on three states and three age cutoffs (35, 50, and 70). To create the posttest RDD samples, we removed from the RCT data all treatment group members younger than the rel- evant age cutoff and all control group members at least as old as the cutoff. Table 2 shows the sample sizes for the nine posttest RDD subsets. The number of obser- vations below the cutoff increases with cutoff age. With the cutoff set at 35, there are many more observations above the cutoff than below; at age 50, observations are more balanced; and at age 70 balance is best overall. The different age cut- offs also determine how much extrapolation is required to compute average effects for everyone above the cutoff. For example, estimating the average effect among everyone older than 35 requires an extrapolation from 36 to 90. In contrast, esti- mating the average effect for people over 70 only requires an extrapolation from 71 to 90.
Next, we used Medicaid expenditures from the pre-randomization year to create nine pretest RDD data subsets based on the same cutoff values and states. With the pretest and posttest RDD subsets in hand, we created a long-form data set by stacking the pretest and posttest RDD data, and defined an indicator variable to identify which observations were from each time period. These stacked data sets form the pretest RDD. They combine data from the pretest period when no one of any age had received the treatment, with data from the posttest period when treatment was available above a specified age cutoff. Stacking the data in this way results in twice as many observations in the pretest RDD compared to the posttest RDD because each participant is observed twice.
These procedures resulted in an RCT, a posttest RDD, and a pretest RDD, each replicated across three states and three age cutoffs. The basic goal of our analysis is to construct estimates of the same causal parameters using each of these research designs. Interpreting the RCT estimates as internally valid allows us to measure the performance of the RDD estimates relative to each other and to the best estimate of the true effect.
Journal of Policy Analysis and Management DOI: 10.1002/pam Published on behalf of the Association for Public Policy Analysis and Management
Methods for Policy Analysis / 859
METHODS
Implementing the within-study comparison requires (1) defining treatment effects of interest, (2) specifying estimators for each effect in each design, and (3) developing measures of performance by which to judge the strengths and weaknesses of each design.
Parameters of Interest
Throughout the paper, we use i to index individuals, and t = [0, 1] to denote the pretest and posttest periods. Ai is a person’s (time invariant) age at baseline, and Preit = 1(t = 0) is a dummy variable that identifies observations made during the pretest time period. We adopt a potential outcomes framework in which Y(1)it denotes the ith person’s treated outcome at time t, and Y(0)it denotes the person’s untreated outcome at time t. The outcome variable in all of our analysis refers to the person’s Medicaid expenditures over the 12 months prior to period t.Dit is an indicator set to 1 if the person has received the treatment at time t. In the Cash and Counseling data, a person is treated if she has the option to control her own Medicaid-financed home care budget. Since no one received the treatment in the pretest time period, Di0 = 0 for everyone in the sample. A person’s realized outcome is Yit = Y(1)it Dit + Y(0)it (1 − Dit ).
To estimate treatment effects at the conventional RDD cutoff and also beyond it, we define treatment effects conditional on specific ages and age ranges. In our notation, the average treatment effect in the posttreatment time period for people who are, say, 70 years old is written as �(70) = E[Y(1)it | Preit = 0, Ai = 70] − E[Y(0)it | Preit = 0, Ai = 70]. If the cutoff value in an RDD was set at age 50, then �(50) = �(RDD) is the average treatment effect in the cutoff subpopulation for that particular RDD.
In a conventional RDD, inference is limited to the average treatment effect at the cutoff. Since part of our analysis is concerned with extrapolating beyond the cutoff, it is also useful to describe average treatment effects in broader subpopulations. One way to do this is to consider averages effects across a range of age groups as relative frequency weighted averages of age-specific treatment effects. For example, If the cutoff value in an RDD was set at age 50 then would be the average treatment effect in the cutoff subpopulation for that particular RDD cutoff is
�(m ≥ 50) = M∑
m=50 �(m) × Pr( Ai = m| Preit = 0)
Pr( Ai ≥ 50 | Preit = 0) .
In a sharp RDD with a cutoff set at c, the parameter �(m ≥ c) represents the average treatment effect above the cutoff, which might also be called the ATT: �(m ≥ c) = �( AT T ). Estimating the ATT parameter requires extrapolation away from the cutoff, so the ATT parameter is not immediately identified in a standard RDD. The pretest RDD that we propose provides one mechanism for making credible extrapolations beyond the cutoff.
Estimation
To estimate the quantities of interest, we used regression methods that account for unknown functional forms either with kernel weighting or a polynomial series in the age variable—the two most common methods used in the modern RDD literature. The use of these flexible models meant that we could not specify a single polynomial model or a single bandwidth for all the designs and states in the analysis. Instead, we
Journal of Policy Analysis and Management DOI: 10.1002/pam Published on behalf of the Association for Public Policy Analysis and Management
860 / Methods for Policy Analysis
specified a method of selecting polynomial specifications and bandwidth parameters that was applied uniformly across the designs. In what follows, we describe the general approach to estimation employed with the RCT, posttest RDD, and pretest RDD. Then, we explain the model selection algorithm used to guide our choice of smoothing parameters like bandwidths and polynomial series lengths. The details regarding the bandwidths and polynomial specifications employed in the analysis are reported in the Appendix.3
Estimation in the RCT
We estimated age-specific treatment effects using two methods. First, we estimated local linear regressions of Medicaid expenditures on age separately for the treatment and control groups. Then, we computed age-specific treatment effects as point- wise differences in treatment and control regression functions for each age. To calculate average treatment effects above the cutoff, we weighted these age-specific differences according to the relative frequency distribution of ages among all of the treatment and control observations from each state. We computed the frequency weights separately for each state to account for differences in the age distribution of each state’s study population.
Since many applied researchers prefer to work with flexible polynomial specifica- tions rather than kernel-based regressions, we also estimated ordinary least squares (OLS) regressions of Medicaid expenditures on a polynomial series in age, a treat- ment group indicator, and interactions between the polynomial series and age for each state. Treatment effect estimates were computed using the coefficients on the treatment indicator and the appropriate interaction terms. Average treatment ef- fects above the cutoff were taken as weighted averages of age-specific differences with weights equal to the relative frequency of each age in the state sample.
Estimation in the Posttest RDD
We estimated treatment effects in the posttest RDDs using both kernel and poly- nomial series regression methods. To implement the kernel regression approach, we estimated treatment effects at the cutoff using local linear regressions applied separately to the data from above and below the cutoff in each state. Treatment effects at the cutoff were calculated using the difference in the estimates of mean Medicaid expenditures at the cutoff.
To implement the polynomial series methods, we pooled data from above and below the cutoff and estimated OLS regressions of Medicaid expenditures on a polynomial in age, a dummy variable set to 1 for observations above the cutoff, and interactions between the age polynomial series and the cutoff dummy variable. In these posttest RDD analyses, we computed treatment effects only at the cutoff. We did not make extrapolations based on the functional form implied by the polynomial regression coefficients because of the well-known tendency of polynomial series estimates to have very poor out-of-sample properties.
Estimation in the Pretest RDD
The pretest RDD combines pretest and posttest RDD data, and for our purposes the key idea is that information about the relationship between the assignment
3 All appendices are available at the end of this article as it appears in JPAM online. Go to the pub- lisher’s Web site and use the search engine to locate the article at http://www3.interscience.wiley.com/ cgi-bin/jhome/34787.
Journal of Policy Analysis and Management DOI: 10.1002/pam Published on behalf of the Association for Public Policy Analysis and Management
Methods for Policy Analysis / 861
variable and the outcomes during the pretest period may provide a sound basis for extrapolation beyond the assignment cutoff in the posttest time period. To put the idea into practice, we specify a flexible model of the untreated outcome variable in the pretest and posttest periods that accounts for simple nonequivalencies between the two periods. In particular, we consider models in which pretest and posttest untreated outcome regression functions differ by a constant across all ages:
Y(0)it = PreitθP + g( Ai ) + νit. In this model, θP represents the fixed difference in conditional mean outcomes across the pretest and posttest periods, and g(.) is an unknown smooth function that is assumed to be constant across the two periods. We assume that E[νit | Preit, Ait ] = 0. In essence, our model assumes that the difference between the mean untreated potential outcome in the pretest and posttest time periods does not vary across subpopulations defined by the assignment variable. The assumption that there is an assignment variable invariant time period effect is important. It implies that, after adjusting for the constant period effect, the underlying regression relationship between the outcomes and the assignment variable function can be recovered across the entire range of the assignment variable in the pretest time period, and then applied to the posttest period. This implication is what makes extrapolation possible.
Similar fixed effect restrictions are widely used in the analysis of longitudinal data (Wooldridge, 2011), though with standard panel data models the assumptions are somewhat stronger than in RDD because such models usually pair a fixed effects assumption with a specific functional form assumption for a vector of time-varying covariates. The point here is that the pretest RDD model is agnostic with respect to the functional form associated with the assignment variable, but it does impose the restriction that the shape of the function does not change across the two time periods except for a change in level that is attributable to the time period effect. Clearly, the accuracy of extrapolations away from the cutoff depends on the validity of the assumption that the time period effect is age invariant. In the next section, we present evidence that this particular assumption is credible in the Cash and Coun- seling data, so our within-study comparisons represent a test of the performance of the pretest RDD method in a situation where the core assumptions appear plausi- ble. Readers should note, of course, that applying our methods in situations where the constant period effect assumption is implausible would likely lead to very poor performance.
With the basic pretest RDD model of the untreated outcomes defined, we turn to methods for estimating treatment effects using the pretest RDD. The first task is to estimate the untreated outcom
Collepals.com Plagiarism Free Papers
Are you looking for custom essay writing service or even dissertation writing services? Just request for our write my paper service, and we'll match you with the best essay writer in your subject! With an exceptional team of professional academic experts in a wide range of subjects, we can guarantee you an unrivaled quality of custom-written papers.
Get ZERO PLAGIARISM, HUMAN WRITTEN ESSAYS
Why Hire Collepals.com writers to do your paper?
Quality- We are experienced and have access to ample research materials.
We write plagiarism Free Content
Confidential- We never share or sell your personal information to third parties.
Support-Chat with us today! We are always waiting to answer all your questions.
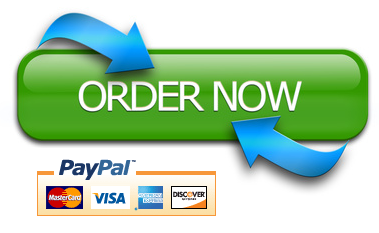