Please use the attached sample and Project reports.? How to Prepare the Portfolio The portfolio report must be typewritten and s
Please use the attached sample and Project reports.
How to Prepare the Portfolio
The portfolio report must be typewritten and should be a minimum of 3 complete pages in length. A project report that is two and a half pages (2.5 pages) is not acceptable. Large margins that have been increased to meet the length requirement are also not acceptable. If your report is not submitted according to the stated specifications, you will be asked to re-write it.
Do not submit the original project; the report is meant to capture the project highlights and significant points of the original project.
You will write a report on the project that includes:
- Introduction
- Explanation of the solution
- Description of the results
- Description of your contributions to the project
- Explanation of what new skills, techniques, or knowledge you acquired from the project and if it was a group project, you should also include a list of team members who worked on the project.
- A reference section with at least 4 references and citations to those references inside the text. Use the IEEE Referencing Style Sheet for citation guidelines.
1
Individual Contribution Report
Pradeep Peddnade Id: 1220962574
2
Reflection:
My overall role in the team was Data Analyst where I was responsible for combining
theory in the group and practices to make and communicate data insights that enabled my
team to make informed inferences regarding the data. Through skills such as data analytics and
statistical modeling, my role as a data analyst was crucial in mining and gathering data. Once
data is ready, performed exploratory analysis for native-country, race, education, and work
class variables of the dataset.
The other role was charged with as a data analyst in the group was to apply statistical
tools to construe the mined data by giving specific attention to the trends and the various
patterns which would lead to predictive analytics to enable the group to make informed
decisions and predictions.
Another role that I did for the group was to work on data cleansing. The specific role
involved managing data though procedure that ensures data us properly formatted and
irrelevant data points are removed.
Lessons Learned:
The wisdom that I would share with others regarding research design is to ensure that
the design is straightforward and aimed towards answering the research question. Having an
appropriate research design will assist the group to answer the research question effectively. I
would also share with the team that it is very appropriate to consider at the time of data
collection from sources and analyze the data into something that the researcher the team
would want to consider. On how to best apply them is to consider that it is appropriate for the
team to ensure that the data is analyzed appropriately and structured appropriately. Make sure
data is cleansed and outliers are removed or normalized.
From the group, we can conclude that the research was an honest effort that was
established to identify how the lessons learned are beyond the project. The data analytics skills
ensured that the analyzed data was collected from the primary sources of data, this prevent
3
the group from the biasedness of another research that was previously conducted. In this, data
world there is unlimited data choosing right variable among the data to answer the research
questions is very important by using correlation and other techniques.
Assessment:
Additional skills that I learned from the course and during the project work is choosing
the visualization type and variables from data set, which is a very important in the analysis of
data. Through this skill, I was able to conceptualize and properly analyze and interpret big data
that requires data modeling and management. Despite that is through the group that I was able
to develop my communication skills since the data analytic role needed an excellent
communicator who would interpret and explain the various inferences to my group.
Group members are in different time zones, scheduling a time to meet was
strenuousness. Everyone in the team was accommodating.
Future Application:
In my current role, I will analyze the metrics of the cluster and logs to monitor the health
of the different services using Elasticsearch Kibana and Grafana. The topics I learned in this
course will be greatly useful and I can apply it in building metrics based Kibana dashboard for
Management to see the usage and cost incurred for each service running in the cluster. And I
will use statistical methods on picking the fields interested among thousands of available fields.
4
,
2/26/22, 9:04 PM CSE578Project
localhost:8888/nbconvert/html/CSE578Project.ipynb?download=false 1/13
In [71]: import pandas as pd import numpy as np from collections import Counter import matplotlib.pyplot as plt import numpy from statsmodels.graphics.mosaicplot import mosaic from sklearn.preprocessing import MinMaxScaler from sklearn.linear_model import LogisticRegression from sklearn.neighbors import KNeighborsClassifier from sklearn.metrics import accuracy_score, precision_score, recall_scor e, f1_score from sklearn.preprocessing import MinMaxScaler from sklearn.model_selection import GridSearchCV, RandomizedSearchCV, tr ain_test_split import warnings %matplotlib inline df = pd.read_csv("data/adult.data", header=None, sep=", ") df.columns = ["age", "workclass", "fnlwgt", "education", "education-num" , "marital-status", "occupation", "relationship", "race", "sex", "capita l-gain", "capital-loss", "hours-per-week", "native-country", "class"] df = df[df["workclass"] != '?'] df = df[df["education"] != '?'] df = df[df["marital-status"] != '?'] df = df[df["occupation"] != '?'] df = df[df["relationship"] != '?'] df = df[df["race"] != '?'] df = df[df["sex"] != '?'] df = df[df["native-country"] != '?'] below = df[df["class"] == "<=50K"] above = df[df["class"] == ">50K"]
<ipython-input-71-d873bf4dac12>:19: ParserWarning: Falling back to the 'python' engine because the 'c' engine does not support regex separator s (separators > 1 char and different from 's+' are interpreted as rege x); you can avoid this warning by specifying engine='python'. df = pd.read_csv("data/adult.data", header=None, sep=", ")
2/26/22, 9:04 PM CSE578Project
localhost:8888/nbconvert/html/CSE578Project.ipynb?download=false 2/13
In [61]: above_50k = Counter(above['native-country']) below_50k = Counter(below['native-country']) print('native-country') fig, axes = plt.subplots(ncols=1, nrows=2, figsize=(5,10)) axes[0].pie(above_50k.values(), labels=above_50k.keys(), autopct='%1.0f% %') axes[0].set_title(">50K") axes[1].pie(below_50k.values(), labels=below_50k.keys(), autopct='%1.0f% %') axes[1].set_title("<=50K") plt.show()
native-country
2/26/22, 9:04 PM CSE578Project
localhost:8888/nbconvert/html/CSE578Project.ipynb?download=false 3/13
In [62]: above_50k = Counter(above['race']) below_50k = Counter(below['race']) print('race') fig, axes = plt.subplots(ncols=1, nrows=2, figsize=(5,10)) axes[0].pie(above_50k.values(), labels=above_50k.keys(), autopct='%1.0f% %') axes[0].set_title(">50K") axes[1].pie(below_50k.values(), labels=below_50k.keys(), autopct='%1.0f% %') axes[1].set_title("<=50K") plt.show()
race
2/26/22, 9:04 PM CSE578Project
localhost:8888/nbconvert/html/CSE578Project.ipynb?download=false 4/13
In [63]: above_50k = Counter(above['education']) below_50k = Counter(below['education']) print('education') fig, axes = plt.subplots(ncols=1, nrows=2, figsize=(5,10)) axes[0].pie(above_50k.values(), labels=above_50k.keys(), autopct='%1.0f% %') axes[0].set_title(">50K") axes[1].pie(below_50k.values(), labels=below_50k.keys(), autopct='%1.0f% %') axes[1].set_title("<=50K") plt.show()
education
2/26/22, 9:04 PM CSE578Project
localhost:8888/nbconvert/html/CSE578Project.ipynb?download=false 5/13
In [64]: above_50k = Counter(above['workclass']) below_50k = Counter(below['workclass']) print('workclass') fig, axes = plt.subplots(ncols=1, nrows=2, figsize=(5,10)) axes[0].pie(above_50k.values(), labels=above_50k.keys(), autopct='%1.0f% %') axes[0].set_title(">50K") axes[1].pie(below_50k.values(), labels=below_50k.keys(), autopct='%1.0f% %') axes[1].set_title("<=50K") plt.show()
workclass
2/26/22, 9:04 PM CSE578Project
localhost:8888/nbconvert/html/CSE578Project.ipynb?download=false 6/13
In [65]: fig, axes = plt.subplots(ncols=2, nrows=3, figsize=(8,8)) fig.subplots_adjust(hspace=.5) x = below['capital-gain'] y = below['age'] axes[0, 0].scatter(x,y) axes[0, 0].set_title("<=50K") axes[0, 0].set_xlabel('capital-gain') axes[0, 0].set_ylabel('age') x = above['capital-gain'] y = above['age'] axes[0, 1].scatter(x,y) axes[0, 1].set_title(">50K") axes[0, 1].set_xlabel('capital-gain') axes[0, 1].set_ylabel('age') x = below['age'] y = below['hours-per-week'] axes[1, 0].scatter(x,y) axes[1, 0].set_title("<=50K") axes[1, 0].set_xlabel('age') axes[1, 0].set_ylabel('hours-per-week') x = above['age'] y = above['hours-per-week'] axes[1, 1].scatter(x,y) axes[1, 1].set_title(">50K") axes[1, 1].set_xlabel('age') axes[1, 1].set_ylabel('hours-per-week') x = below['hours-per-week'] y = below['capital-gain'] axes[2, 0].scatter(x,y) axes[2, 0].set_title("<=50K") axes[2, 0].set_xlabel('hours-per-week') axes[2, 0].set_ylabel('capital-gain') x = above['hours-per-week'] y = above['capital-gain'] axes[2, 1].scatter(x,y) axes[2, 1].set_title(">50K") axes[2, 1].set_xlabel('hours-per-week') axes[2, 1].set_ylabel('capital-gain') plt.show()
2/26/22, 9:04 PM CSE578Project
localhost:8888/nbconvert/html/CSE578Project.ipynb?download=false 7/13
2/26/22, 9:04 PM CSE578Project
localhost:8888/nbconvert/html/CSE578Project.ipynb?download=false 8/13
In [50]: fig, axes = plt.subplots(ncols=1, nrows=1, figsize=(15,10)) fig.subplots_adjust(hspace=.5) mosaic(df, ['occupation', 'class'], ax=axes, axes_label=False) plt.show()
2/26/22, 9:04 PM CSE578Project
localhost:8888/nbconvert/html/CSE578Project.ipynb?download=false 9/13
In [51]: fig, axes = plt.subplots(ncols=1, nrows=1, figsize=(15,10)) fig.subplots_adjust(hspace=.5) mosaic(df, ['marital-status', 'class'], ax=axes, axes_label=False) plt.show()
2/26/22, 9:04 PM CSE578Project
localhost:8888/nbconvert/html/CSE578Project.ipynb?download=false 10/13
In [54]: fig, axes = plt.subplots(ncols=1, nrows=1, figsize=(15,12)) fig.subplots_adjust(hspace=.5) mosaic(df, ['education-num', 'class'], ax=axes, axes_label=False) plt.show()
2/26/22, 9:04 PM CSE578Project
localhost:8888/nbconvert/html/CSE578Project.ipynb?download=false 11/13
In [90]: train = df train = train.drop("capital-loss", axis=1) train = train.drop("native-country", axis=1) train = train.drop("fnlwgt", axis=1) train = train.drop("education",axis=1) def get_occupation(x): if x in ["Exec-managerial", "Prof-specialty", "Protective-serv"]: return 1 elif x in ["Sales", "Transport-moving", "Tech-support", "Craft-repai r"]: return 2 else: return 3 def get_relationship(x): if x == "Own-child": return 6 elif x == "Other-relative": return 5 elif x == "Unmarried": return 4 elif x == "Not-in-family": return 3 elif x == "Husband": return 2 else: return 1 def get_race(x): if x == "Other": return 5 elif x == "Amer-Indian-Eskimo": return 4 elif x == "Black": return 3 elif x == "White": return 2 else: return 1 def get_sex(x): if x == "Male": return 2 else: return 1 def get_class(x): if x == ">50K": return 1 else: return 0 def get_workclass(x): if x == "Without-pay":
2/26/22, 9:04 PM CSE578Project
localhost:8888/nbconvert/html/CSE578Project.ipynb?download=false 12/13
return 7 elif x == "Private": return 6 elif x == "State-gov": return 5 elif x == "Self-emp-not-inc": return 4 elif x == "Local-gov": return 3 elif x == "Federal-gov": return 2 else: return 1 def get_marital_status(x): if x == "Never-married": return 7 elif x == "Separated": return 6 elif x == "Married-spouse-absent": return 5 elif x == "Widowed": return 4 elif x == "Divorced": return 3 elif x == "Married-civ-spouse": return 2 else: return 1 train['workclass'] = train['workclass'].apply(get_workclass) train['marital-status'] = train['marital-status'].apply(get_marital_stat us) train['occupation'] = train['occupation'].apply(get_occupation) train['relationship'] = train['relationship'].apply(get_relationship) train['race'] = train['race'].apply(get_race) train['sex'] = train['sex'].apply(get_sex) train['class'] = train['class'].apply(get_class)
Out[90]:
age workclass education-
num marital- status occupation relationship race sex
capital- gain
hours- per-
week cla
0 39 5 13 7 3 3 2 2 2174 40
1 50 4 13 2 1 2 2 2 0 13
2 38 6 9 3 3 3 2 2 0 40
3 53 6 7 2 3 2 3 2 0 40
4 28 6 13 2 1 1 3 1 0 40
2/26/22, 9:04 PM CSE578Project
localhost:8888/nbconvert/html/CSE578Project.ipynb?download=false 13/13
In [96]: test=pd.read_csv("data/adult.test", header=None, sep=", ") feature = train.iloc[:, :-1] labels = train.iloc[:, -1] feature_matrix1 = feature.values labels1 = labels.values train_data, test_data, train_labels, test_labels = train_test_split(feat ure_matrix1, labels1, test_size=0.2, random_state=42) transformed_train_data = MinMaxScaler().fit_transform(train_data) transformed_test_data = MinMaxScaler().fit_transform(test_data)
In [97]: t
In [114]: mod=LogisticRegression().fit(transformed_train_data,train_labels) test_predict=mod.predict(transformed_test_data) acc=accuracy_score(test_labels, test_predict) f1=f1_score(test_labels, test_predict) prec=precision_score(test_labels,test_predict) rec=recall_score(test_labels, test_predict)
In [115]: print("%.4ft%.4ft%.4ft%.4ft%s" % (acc, f1, prec, rec, 'Logistic Regr ession'))
In [ ]:
<ipython-input-96-90f00b23459c>:1: ParserWarning: Falling back to the 'python' engine because the 'c' engine does not support regex separator s (separators > 1 char and different from 's+' are interpreted as rege x); you can avoid this warning by specifying engine='python'. test=pd.read_csv("data/adult.test", header=None, sep=", ")
0.8409 0.6404 0.7500 0.5588 Logistic Regression
,
Course Title Portfolio
Name Email
Abstract—This document This document This document This document This document This document This document This document This document This document This document This document This document This document This document This document This document This document This document This document This document This document This document This document This document This document This document This document This document This document This document This document.
Keywords—mean, standard deviation, variance, probability density function, classifier
I. INTRODUCTION This document This document This document This
document This document This document This document This document This document This document This document This document This document This document This document This document. [1].
This project practiced the use of density estimation through several calculations via the Naïve Bayes Classifier. The data for each equation was used to find the probability of the mean for. Without using a built-in function, the first feature, the mean, could be calculated using the equation in Fig. 1. The second feature, the standard deviation, could be calculated using the equation in Fig. 2. Utilizing the training set for digit 0, the mean of the pixel brightness values was determined by calling ‘numpy.mean()digit 0 or digit 1. The test images were then classified based on the previous calculations and the accuracy of the computations were determined.
The project consisted of 4 tasks:
A. Extract features from the original training set
There were two features that needed to be extracted from the original training set for each image. The first feature was the average pixel brightness values within an image array. The second was the standard deviation of all pixel brightness values within an image array.
B. Calculate the parameters for the two-class Naïve Bayes Classifiers
Using the features extracted from task A, multiple calculations needed to be performed. For the training set involving digit 0, the mean of all the average brightness values was calculated. The variance was then calculated for the same feature, regarding digit 0. Next, the mean of the standard deviations involving digit 0 had to be computed. In addition, the variance for the same feature was determined. These four calculations had to then be repeated using the training set for digit 1.
C. Classify all unknown labels of incoming data
Using the parameters obtained in task B, every image in each testing sample had to be compared with the corresponding training set for that particular digit, 0 or 1. The probability of that image being a 0 or 1 needed to be determined so it can then be classified.
D. Calculate the accuracy of the classifications
Using the predicted classifications from task C, the accuracy of the predictions needed to be calculated for both digit 0 and digit 1, respectively.
Each equation was used to find the probability of the mean for. Without using a built-in function, the first feature, the mean, could be calculated using the equation in Fig. 1. The second feature, the standard deviation, could be calculated using the equation in Fig. 2. Utilizing the training set for digit 0, the mean of the pixel brightness values was determined by calling ‘numpy.mean()of the data. These features helped formulate the probability density function when determining the classification.
II. DESCRIPTION OF SOLUTION This project required a series of computations in order to
successfully equation was used to find the probability of the mean for. Without using a built-in function, the first feature, the mean, could be calculated using the equation in Fig. 1. The second feature, the standard deviation, could be calculated using the equation in Fig. 2. Utilizing the training set for digit 0, the mean of the pixel brightness values was determined by calling ‘numpy.mean(). Once acquiring the data, the appropriate calculations could be made.
A. Finding the mean and standard deviation
The data was provided in the form of NumPy arrays, which made it useful for performing routine mathematical operations equation was used to find the probability of the mean for. Without using a built-in function, the first feature, the mean, could be calculated using the equation in Fig. 1. The second feature, the standard deviation, could be calculated using the equation in Fig. 2. Utilizing the training set for digit 0, the mean of the pixel brightness values was
determined by calling ‘numpy.mean()by calling ‘numpy.std()’, another useful NumPy function. These extracted features from the training set for digit 0 also had to be evaluated from the training set for digit 1. Once all the features for each image were obtained from both training sets, the next task could be completed.
Equ. 1. Mean formula
B. Determining the parameters for the Naïve Bayes Classifiers
To equation was used to find the probability of the mean for. Without using a built-in function, the first feature, the mean, could be calculated using the equation in Fig. 1. The second feature, the standard deviation, could be calculated using the equation in Fig. 2. Utilizing the training set for digit 0, the mean of the pixel brightness values was determined by calling ‘numpy.mean() and the array of the standard deviations created for digit 1.
Equ. 2. Variance formula
This equation was used to find the probability of the mean for. Without using a built-in function, the first feature, the mean, could be calculated using the equation in Fig. 1. The second feature, the standard deviation, could be calculated using the equation in Fig. 2. Utilizing the training set for digit 0, the mean of the pixel brightness values was determined by calling ‘numpy.mean()’ for each image in the set. In addition, the standard deviation of the pixel brightness values was calculated for each image by calling ‘numpy.std()’, another useful NumPy function. These extracted features from the training. This was multiplied by the prior probability, which is 0.5 in this case because the value is either a 0 or a 1.
This ]. Without using a built-in function, the first feature, the mean, could be calculated using the equation in Fig. 1. The second feature, the standard deviation, could be calculated using the equation in Fig. 2. Utilizing the training set for digit 0, the mean of the pixel brightness values was determined by calling ‘numpy.mean()’ for each image in the set. In addition, the standard deviation of the pixel brightness values was calculated for each image by calling ‘numpy.std()’, another useful NumPy function. These extracted features from the training.
This entire procedure had to be conducted once again but utilizing the test sample for digit 1 instead. This meant finding the mean and standard deviation of each image, using the probability density function to calculate the probability of the mean and probability of the standard deviation for digit 0, and calculating the probability that the image is classified as digit 0. The same operations had to be performed again, but for the training set for digit 1. The probability of the image being classified as digit 0 had to be compared to the
probability of the image being classified as digit 1. Again, the larger of the two values suggested which digit to classify as the label.
One aspect of machine learning that I understood better after completion of the project was Gaussian distribution. This normalized distribution style displays a bell-shape of data in which the peak of the bell is where the mean of the data is located [4]. A bimodal distribution is one that displays two bell-shaped distributions on the same graph. After calculating the features for both digit 0 and digit 1, the probability density function gave statistical odds of that particular image being classified under a specific bell- shaped curve. An example of a bimodal distribution can be seen in Fig. 7 below.
C. Determining the accuracy of the label
The mean for. Without using a built-in function, the first feature, the mean, could be calculated using the equation in Fig. 1. The second feature, the standard deviation, could be calculated using the equation in Fig. 2. Utilizing the training set for digit 0, the mean of the pixel brightness values was determined by calling ‘numpy.mean()’ for each image in the set. In addition, the standard deviation of the pixel brightness values was calculated for each image by calling ‘numpy.std()’, another useful NumPy function. These extracted features from the by the total number of images in the test sample for digit 1.
III. RESULTS mean for. Without using a built-in function, the first
feature, the mean, could be calculated using the equation in Fig. 1. The second feature, the standard deviation, could be calculated using the equation in Fig. 2. Utilizing the training set for digit 0, the mean of the pixel brightness values was determined by calling ‘numpy.mean()’ for each image in the set. In addition, the standard deviation of the pixel brightness values was calculated for each image by calling ‘numpy.std()’, another useful NumPy function. These extracted features from the also higher.
TABLE I. TRAINING SET FOR DIGIT 0
TTTTTTTT 000000
XXXXX 000000
When comparing the test images, the higher values of the means and the standard deviations typically were labeled as digit 0 and the lower ones as digit 1. However, this was not always the case because then the calculated accuracy would then be 100%.
The e. After classifying all the images in the test sample for digit 0, the total amount predicted as digit 0 was 899. This meant that the accuracy of classification was 0000%, which can be represented in Fig. 5.
Fig. 1. Accuracy of classification for digit 0
The total amount of images in the test sample for digit 1
0000. After classifying all the images in the test sample for digit 1, the total amount predicted as digit 00000. This meant that the accuracy of classification was 00000%, which can be represented in Fig. 6.
IV. LESSONS LEARNED The procedures practiced in this project required skill in
the Python programming language, as well as understanding concepts of statistics. It required plenty of practice to implement statistical equations, such as finding the mean, the standard deviation, and the variance. My foundational knowledge of mathematical operations helped me gain an initial understanding of how to set up classification problems. My lack of understanding of the Python language made it difficult to succeed initially. Proper syntax and built-in functions had to be learned first before continuing with solving the classification issue. For example, I had very little understanding of NumPy prior to this project. I learned that it was extremely beneficial for producing results of mathematical operations. One of the biggest challenges for me was creating and navigating through NumPy arrays rather than a Python array. Looking back, it was a simple issue that I solved after understanding how they were uniquely formed. Once I had a grasp on the language and built-in functions, I was able to create the probability density function in the code and then apply classification towards each image.
One aspect of machine learning that I understood better after completion of the project was Gaussian distribution. This normalized distribution style displays a bell-shape of data in which the peak of the bell is where the mean of the data is located [4]. A bimodal distribution is one that displays two bell-shaped distributions on the same graph. After calculating the features for both digit 0 and digit 1, the probability density function gave statistical odds of that particular image being classified under a specific bell- shaped curve. An example of a bimodal distribution can be seen in Fig. 7 below.
One aspect of machine learning that I understood better after completion of the project was Gaussian distribution. This normalized distribution style displays a bell-shape of data in which the peak of the bell is where the mean of the data is located [4]. A bimodal distribution is one that displays two bell-shaped distributions on the same graph. After calculating the features for both digit 0 and digit 1, the probability density function gave statistical odds of that particular image being classified under a specific bell-
shaped curve. An example of a bimodal distribution can be seen in Fig. 7 below.
One aspect of machine learning that I understood better after completion of the project was Gaussian distribution. This normalized distribution style displays a bell-shape of data in which the peak of the bell is where the mean of the data is located [4]. A bimodal distribution is one that displays two bell-shaped distributions on the same graph. After calculating the features for both digit 0 and digit 1, the probability density function gave statistical odds of that particular image being classified under a specific bell- shaped curve. An example of a bimodal distribution can be seen in Fig. 7 below.
Fig. 2. Bimodal distribution example [5]
Upon completion of the project, I was able to realize that
One aspect of machine learning that I understood better after completion of the project was Gaussian distribution. This normalized distribution style displays a bell-shape of data in which the peak of the bell is where the mean of the data is located [4]. A bimodal distribution is one that displays two bell-shaped distributions on the same graph. After calculating the features for both digit 0 and digit 1, the probability density function gave statistical odds of that particular image being classified under a specific bell- shaped curve. An example of a bimodal distribution can be seen in Fig. 7 below.
One aspect of machine learning that I understood better after completion of the project was Gaussian distribution. This normalized distribution style displays a bell-shape of data in which the peak of the bell is where the mean of the data is located [4]. A bimodal distribution is one that displays two bell-shaped distributions on the same graph. After calculating the features for both digit 0 and digit 1, the probability density function gave statistical odds of that particular image being classified under a specific bell- shaped curve. An example of a bimodal distribution can be seen in Fig. 7 below.
One aspect of machine learning that I understood better after completion of the project was Gaussian distribution. This normalized distribution style displays a bell-shape of data in which the peak of the the project was Gaussian distribution. This normalized distribution style the project was Gaussian distribution. This normalized distributio
Collepals.com Plagiarism Free Papers
Are you looking for custom essay writing service or even dissertation writing services? Just request for our write my paper service, and we'll match you with the best essay writer in your subject! With an exceptional team of professional academic experts in a wide range of subjects, we can guarantee you an unrivaled quality of custom-written papers.
Get ZERO PLAGIARISM, HUMAN WRITTEN ESSAYS
Why Hire Collepals.com writers to do your paper?
Quality- We are experienced and have access to ample research materials.
We write plagiarism Free Content
Confidential- We never share or sell your personal information to third parties.
Support-Chat with us today! We are always waiting to answer all your questions.
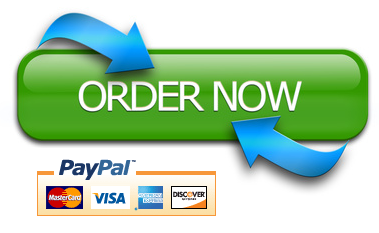