From the article provided, answer the following questions. ? In your post, include the following: 1. Point out the experimental
Cross referenced through turnitin and coursehero.
From the article provided, answer the following questions.
In your post, include the following:
1. Point out the experimental question & purpose of study.
2. Point out the exact design utilized (example: non-concurrent multiple baseline)
3. Think about the visual display of data and describe the (each):
o Level
o Trend
o Variability
o Latency to change
4. Shortly summarise if the study showed control & evidence to support your decision .
Modeling external events in the three-level analysis of multiple-baseline across-participants designs: A simulation study
Mariola Moeyaert & Maaike Ugille & John M. Ferron & S. Natasha Beretvas & Wim Van den Noortgate
Published online: 12 December 2012 # Psychonomic Society, Inc. 2012
Abstract In this study, we focus on a three-level meta- analysis for combining data from studies using multiple- baseline across-participants designs. A complicating factor in such designs is that results might be biased if the depen- dent variable is affected by not explicitly modeled external events, such as the illness of a teacher, an exciting class activity, or the presence of a foreign observer. In multiple- baseline designs, external effects can become apparent if they simultaneously have an effect on the outcome score (s) of the participants within a study. This study presents a method for adjusting the three-level model to external events and evaluates the appropriateness of the modified model. Therefore, we use a simulation study, and we illus- trate the new approach with real data sets. The results indicate that ignoring an external event effect results in biased estimates of the treatment effects, especially when
there is only a small number of studies and measurement occasions involved. The mean squared error, as well as the standard error and coverage proportion of the effect esti- mates, is improved with the modified model. Moreover, the adjusted model results in less biased variance estimates. If there is no external event effect, we find no differences in results between the modified and unmodified models.
Keywords Multiple baseline across participants . Three-level meta-analysis . Effect sizes . External event effect
A multiple-baseline design (MBD) is one of the variants of single-subject experimental designs (SSEDs). SSED researchers observe and measure a participant or case re- peatedly over time. Observations are obtained during at least one baseline phase (when no intervention is present) and at least one treatment phase (when an intervention is present). By comparing scores from both kinds of phases, SSED researchers can assess whether the outcome scores on the dependent variable changed, for instance, in level or in slope when the treatment was present (Onghena & Edgington, 2005).
In an MBD, an AB phase design (with one baseline phase, A, and one treatment phase, B) is implemented simultaneously to different participants, behaviors, or settings (Barlow & Hersen, 1984; Ferron & Scott, 2005; Onghena, 2005; Onghena & Edgington, 2005). MBDs are popular among SSED researchers (Shadish & Sullivan, 2011) because the interven- tion is introduced sequentially over the participants (or settings and behaviors), which entails the advantage that the researchers can more easily disentangle effects of the intervention and effects of some external events, such as the illness of a teacher, an exciting class activity, the presence of a foreign observer, or
M. Moeyaert : M. Ugille University of Leuven, Leuven, Belgium
J. M. Ferron University of South Florida, Tampa, FL, USA
S. N. Beretvas University of Texas, Austin, TX, USA
W. Van den Noortgate University of Leuven, Leuven, Belgium
M. Moeyaert (*) Faculty of Psychology and Educational Sciences, University of Leuven, Andreas Vesaliusstraat 2, Box 3762, 3000 Leuven, Belgium e-mail: [email protected]
Behav Res (2013) 45:547–559 DOI 10.3758/s13428-012-0274-1
a teacher intern (Baer, Wolf, & Risley, 1968; Barlow & Hersen, 1984; Kinugasa, Cerin, & Hooper, 2004; Koehler & Levin, 2000). This is because, if an external event occurs at certain points in time, the outcome scores for all participants in that study might be simultaneously influenced. Figure 1 gives a graphical presentation of possible consequences for the occur- rence of an external event in a multiple-baseline across- participants design. In Fig. 1a, the external event has a constant effect on the dependent variable on subsequent measurements—for instance, the teacher is ill during subse- quent days, or there is a foreign observer during some measure- ment occasions. Figure 1b illustrates a gradually fading away external event effect. For instance, the influence of a teacher intern on the behavior of the students may be reduced over time.
Van den Noortgate and Onghena (2003) proposed the use of multilevel models to synthesize data from multiple SSED studies, allowing investigation of the generalizability of the results and exploration of potential moderating effects. In previous research evaluating this multilevel meta-analysis of MBD data (Ferron, Bell, Hess, Rendina-Gobioff, & Hibbard, 2009; Ferron, Farmer, & Owens, 2010; Moeyaert, Ugille, Ferron, Beretvas, & Van den Noorgate, 2012a, 2012b; Owens & Ferron, 2012), the data were typically simulated with a treatment effect and random noise only. Potential
confounding events that could have a simultaneous ef- fect on all participants within a study were not taken into account. In this study, we evaluate the performance of the basic three-level model when there are effects of external events, as well as that of an extension of the model that tries to account for potential event effects. In the following, we first present the basic model and a possible extension to account for external events. Next, we evaluate the performance of both models, by means of a simulation study and an analysis of real data.
Three-level meta-analysis
A meta-analysis combines the results of several studies addressing the same research question (Cooper, 2010; Glass, 1976). Study results are typically first converted to a com- mon standardized effect size before meta-analyzing them. The effect sizes may be reported in the primary studies or can be calculated afterward, using reported summary and/or test statistics.
One possible way to calculate effect sizes when SSEDs are used is to analyze the data using regression models and to use the regression coefficients as effect sizes. A
Fig. 1 Graphical display of a constant external event effect (a) and a gradually fading away external event effect (b) affecting the score on four subsequent moments (day 17, day 19, day 21, and day 23) for a
multiple-baseline design across 3 participants, with the treatment start- ing on day 6, day 16, and day 24, respectively
548 Behav Res (2013) 45:547–559
regression model of interest here is the one proposed by Center, Skiba, and Casey (1985–1986):
Yi ¼ b0 þ b1Ti þ b2Di þ b3T 0iDi þ ei: ð1Þ
The score of the dependent variable on measurement occasion i (Yi ) depends on a dummy coded variable (Di) indicating whether the measurement occasion i belongs to the baseline phase (Di 0 0) or the treatment phase (Di 0 1); a time-related variable Ti that equals 1 on the first measure- ment occasion of the baseline phase; and an interaction term between the centered time indicator and the dummy vari- able, T 0iDi, where T
0 i is centered such that T
0 i equals 0 on the
first measurement occasion of the treatment phase. b0 indi- cates the expected baseline level b1 is the linear trend during the baseline, b2 refers to the immediate treatment effect, and b3 refers to the effect of the treatment on the time trend.
Van den Noortgate and Onghena (2003) proposed using the ordinary least squares estimates for b2 and b3 from Eq. 1 as effect sizes in the three-level meta-analysis. At the first level, the estimated effect sizes of the immediate treatment effect, b2jk, and the treatment effect on the time trend, b3jk, for participant j from study k are equal to the unknown population effect sizes, β2jk and β3jk, respectively, plus ran- dom deviation s,r2jk and r3jk, that are assumed to be normal- ly distributed with a mean of zero:
b2jk ¼ b2jk þ r2jk with r2jk � N 0; σ2r2jk � �
b3jk ¼ b3jk þ r3jk with r2jk � N 0; σ2r3jk � �
: ð2Þ
The sampling variances of the observed effects, σ2r2jk and
σ2r3jk ; are the squared standard errors that are typically
reported by default when a regression analysis is performed. These variances depend to a large extent on the number of observations and the variance of these observations and, therefore, can be participant and study specific. At the second level, the population effect sizes b2jk and b3jk from Eq. 2 can be modeled as varying over participants around the study-specific mean effect, θ20k and θ30k (Eq. 3):
b2jk ¼ θ20k þ u2jk with u2jk � N 0; σ2u2jk � �
b3jk ¼ θ30k þ u3jk with u3jk � N 0; σ2u3jk � �
: ð3Þ
The population effects for studies can vary between studies (third level, Eq. 4):
θ20k ¼ g200 þ v20k with v20k � N 0; σ2v20k � �
θ30k ¼ g300 þ v30k with v30k � N 0; σ2v30k � �
: ð4Þ
The model parameters that we are typically interested in when using a multilevel model are the fixed effects regression coefficients (i.e., g200 , referring to the average immediate treatment effect over participants and studies, and g300, refer- ring to the average treatment effect on the linear trend over participants and studies in Eq. 4) and the variances (i.e., σ2v20k ,
referring to the between-study variance for the estimated im- mediate treatment effect; σ2v30k , indicating the between-study
variance for the estimated treatment effect on the time trend; σ2u2jk , the between-case variance for the estimated immediate
treatment effect; and σ2u3jk , referring to the between-case vari-
ance of the estimated treatment effect on the time trend).
Correcting effect sizes for external events
External events in a multiple-baseline across-participants de- sign can have an effect on the outcome score(s) of all partici- pants within a study. These external event effects are common in SSEDs, because practitioners often implement these designs in their everyday setting (for example, in the home, school, etc.), where they cannot control for outside experimental factors (Christ, 2007; Kratochwill et al., 2010; Shadish, Cook, & Campbell, 2002). If we do not model these external events, the results might be biased. For instance, suppose that a re- searcher is interested in a change in challenging behavior and staggers the beginning of the treatment across 3 participants. The 3 participants receive the treatment at day 6, day 16, and day 24, respectively (see Fig. 1) and are observed every 2 days. On days 17, 19, 21, and 23, the teacher is ill, and as a conse- quence, a substitute teacher takes his or her place, and the participants exhibit more challenging behavior. In this situation, the estimated treatment effect for participants 1 and 2 will be smaller, and the estimated treatment effect for participant 3 will be larger, and therefore differences between participants in the treatment effects are also likely to be overestimated, unless we correct the effect sizes for possible external events.
A possible way to calculate effect sizes corrected for an external event in an SSED is by estimating effect sizes for participants per study, by performing a regression analysis with a model including possible event effects, and by as- suming that external events simultaneously affect all partic- ipants in a study. Thereafter, the corrected effect sizes can be combined over studies in the three-level meta-analysis.
For the first step, we propose to use an extension of the Center et al. (1985–1986) model, including dummy varia- bles for measurement occasions:
Yij ¼ b0j þ b1jTij þ b2jDij þ b3jDijT 0 ij
þ XI�1 m¼2
b mþ2ð ÞMmi þ eij: ð5Þ
Behav Res (2013) 45:547–559 549
The score on the dependent variable Y on measurement occasion i (0 1, 2, . . . , I) from participant j (0 1, 2, . . . , J) is modeled as a linear function of the dummy-coded variable (Dij) indicating whether the measurement occasion i from participant j belongs to the baseline phase (Dij 0 0) or the treatment phase (Dij 0 1); a time-related variable Tij, which equals 1 at the start of the baseline phase; an interaction term between the dummy variable indicating the phase and the time indicator centered around its value at the start of the
treatment phase, DijT 0 ij; and finally, dummy-coded variables
indicating the moment (Mmi 0 1 if m 0 i, zero otherwise). By including the effects of individual moments, coefficients β2j and β3j can be interpreted as the treatment effects, corrected for possible external events.
We do not include a dummy variable for one mea- surement moment in the baseline phase and one mea- surement moment in the treatment phase. This is to ensure that the model is identified; if we included these parameters as well, an increase in the effects for each moment in the baseline phase could be compensated for by a decrease of the intercept, illustrating that without constraining these parameters, there would be an infinite number of equivalent solutions. For our study, we select the first and last moments as the times at which to set the moment effects to zero, but different moments can be chosen if we suspect a moment effect during one of these times.
While the baseline level and slope (β0j and β1j) and both treatment effects (β2j and β3j) are participant specific, the moment effects are assumed to be the same for all partic- ipants from the same study and, therefore, have to be esti- mated for each study, using all data from that study. To this end, we propose to extend Eq. 5 by including a set of dummy participant indicators. For 2 participants, using dummy participant indicators P1 and P2, respectively, this results in Eq. 6:
Yij ¼ b01P1j þ b02P2j þ b11Ti1P1j þ b12Ti2P2j þ b21Di1P1j þ b22Di2P2j þ b31Di1T
0 i1P1j
þ b32Di2T 0 i2P2j þ
XI�1 m¼2
b mþ2ð ÞMmi þ eij: ð6Þ
After using Eq. 6 for each study to estimate the corrected effect sizes (β2j and β3j) for each participant, we can use the three-level meta-analysis (see Eqs. 2–4) to combine the corrected effect size estimates from multiple participants. In principle, we could also use a two-level model per study to estimate the participant- specific effects, but given the typically very small num- ber of participants per study, using a multilevel model might not be recommended.
A simulation study
Simulating three-level data
To evaluate the performance of the basic model and its extension, we performed a simulation study. We simulated raw data using a three-level model. At level 1, we used the following model:
Yijk ¼ b0jk þ b1jkTijk þ b2jkDijk þ b3jkTijkDijk þ eijk with eijk � N 0; σ2e
� � ;
ð7Þ
with measurement occasions nested within participants, which form the units at level two:
b0jk ¼ θ00k þ u0jk b1jk ¼ θ10k þ u1jk b2jk ¼ θ20k þ u2jk b3jk ¼ θ30k þ u3jk
8>>< >>:
with
u0jk u1jk u2jk u3jk
2 664
3 775 � N 0; @uð Þ: ð8Þ
The participants are, in turn, clustered within studies at the third level:
θ00k ¼ g000 þ v00k θ10k ¼ g100 þ v10k θ20k ¼ g200 þ v20k θ30k ¼ g300 þ v30k
8>>< >>:
with
v00k v10k v20k v30k
2 664
3 775 � N 0; @vð Þ: ð9Þ
Varying parameters
On the basis of a thorough overview of 809 SSED studies, Shadish and Sullivan (2011) enumerated some parameters that characterize SSEDs. On the basis of their results and our reanalyses of meta-analyses of SSEDs (Alen, Grietens, & Van den Noortgate, 2009; Denis, Van den Noortgate, & Maes, 2011; Ferron et al., 2010; Kokina & Kern, 2010; Shadish & Sullivan, 2011; Shogren, Faggella-Luby, Bae, & Wehmeyer, 2004; Wang, Cui, & Parrila, 2011), we decided to vary the following parameters that can have a significant influence on the quality of model estimation:
& g200 , represents the immediate treatment effect on the outcome and had values 0 (no effect) or 2.
& The treatment effect on the time trend, defined by g300, was varied to have values 0 (no effect) or 0.2.
& The regression coefficients of the baseline, g000 and g100 , did not vary and were set at 0, because the interest is in the overall treatment effects (e.g., the immediate treatment effect and the treatment effect on the time trend).
& The number of simulated participants, J, equaled 4 or 7. & The number of measurements within a participant, I, was
15 or 30. We chose to keep I constant for all participants within the same study.
550 Behav Res (2013) 45:547–559
& The number of studies, K, was 10 or 30. & The between-case covariance matrix: Covariances between
pairs of regression coefficients were set to zero. Therefore,u
is a diagonal matrix: P
u ¼ diag σ2u0; σ2u1; σ2u2; σ2u3 � �
¼ diag 2; 0:2; 2; 0:2ð Þ or Pu ¼ diag σ2u0; σ2u1; σ2u2; σ2u3
� � ¼
diag 0:5; 0:05; 0:5; 0:05ð Þ. & The between-study covariance matrix: Covariances between
pairs of regression coefficients were set to zero. Therefore, v
is a diagonal matrix: P
v ¼ diag σ2v0; σ2v1; σ2v; σ2v3 � �
¼ diag 2; 0:2; 2; 0:2ð Þ or Pv ¼ diag σ2v0; σ2v1; σ2v2; σ2v3
� � ¼
diag 0:5; 0:05; 0:5; 0:05ð Þ. & The moment of introducing a treatment effect was stag-
gered across participants within a study (see Table 1), depending on the number of measurements.
In a first scenario, a constant external event was added to influence four subsequent scores of all the participants with- in a study (as in Fig. 1a). The moment was randomly generated from a uniform distribution for each study sepa- rately. Because we did not include a moment effect for the first and the last moments to make the model identified, the external event effect did not occur on these moments. The external event effect was 0 or 2, representing a null and a large external event effect, respectively.
In a second scenario, the effect of the external event effect was added, which fades away gradually (see Fig. 1b) for all the participants within a study. The effect across four time points was 3.5, 2.5, 1.5, 0.5, and 0, respectively, so that, on average, the overall effect was the same as in the first scenario. The start of the event effect was generated completely at random from a uniform distribution for each study separately, so that the external event effect did not occur on the first or last measurement occasion. Data were generated using SAS.
Analysis
We had a total of 29 (0 512) experimental conditions. We simulated 400 replications of each condition, resulting in 204,800 data sets to analyze. We analyzed the data twice and compared the results. First, we combined the uncorrected effect sizes in the three-level meta-analysis. Next, we analyzed the
three-level data by estimating the corrected effect sizes, β2j and β3j, using the regression analysis per study (see Eq. 5) before combining them in the three-level meta-analysis (see Eqs. 2–4).
In the two approaches, we used the SAS proc MIXED (Littell, Milliken, Stroup, Wolfinger, & Schabenberger, 2006) procedure to estimate the participant-specific effect sizes, β2jk and β3jk. In the first approach, the effect sizes were uncorrected for the external event effect, whereas the effect sizes in the second approach were corrected.
SAS proc MIXED was also used for the three-level meta- analysis. The Satterthwaite approach to estimating the degrees of freedom method was applied because this meth- od provides more accurate confidence intervals for estimates of the average treatment effect for two-level analyses of multiple-baseline data (Ferron et al., 2009).
In order to evaluate the appropriateness of both models, uncorrected and corrected for external events, we calculated the deviations of the estimated immediate treatment effect, bg200, from its population value, g200, and the deviations of the estimated treatment effect on the time trend, bg300 , from its population value,g300. The mean deviation gives us an idea of the bias. Next, we calculated the mean squared deviation (the mean squared error [MSE]), which gives information about the variance of both estimated treatment effects (bg200 andbg300) around the corresponding population effect (g200 and g300). Furthermore, we discuss the standard error and the 95 % confidence interval coverage proportion (CP) of the estimated immediate treatment effect and the treatment effect on the time trend. We also evaluate the bias of the point estimates of the between-study and between-case variances.
We used ANOVAs to evaluate whether there were signifi- cant effects (α 0 .01) of each model type (e.g., model using effect sizes corrected vs. uncorrected for external event effects) and of the simulation design parameters (g200, g300, K, I, J, σ
2 u2 ,
σ2v2) on the bias, the MSE, the standard error, and the CP.
Results of the simulation study
We present the results in two sections. In the first section, we discuss the constant external effect over four subsequent measurement occasions. The second section considers the case where the external effect gradually fades away over
Table 1 Time of introducing the treatment
Start of intervention
I Participant 1 Participant 2 Participant 3 Participant 4 Participant 5 Participant 6 Participant 7
15 5 6 7 8 9 11 13
30 5 8 11 14 17 20 23
Behav Res (2013) 45:547–559 551
four subsequent measurements. Each section presents the results of the three-level analysis of uncorrected and cor- rected effect sizes.
When there is no external event effect, the results of the three-level meta-analysis (i.e., bias in the fixed effects, MSE of the fixed effects, estimated standard errors of the fixed effects, CP for the fixed effects, and bias in the variance components) were found to be independent of the model type (corrected or not corrected for external events).
We found no significant bias for bg200 and bg300 when using the corrected or uncorrected model. Therefore, we discuss the results of the analyses of the data including only external event effects conditions.
Constant external event over four subsequent measurement occasions
Overall treatment effect
Bias When we estimate g200 and the effect sizes are uncor- rected, the estimated treatment effect is, on average, signifi- cantly larger than the population value (g200 0 0 or 2). Over all conditions, the bias equals 0.032, t(51199) 0 17.32, p < .0001, whereas there is no significant bias for the corrected effect sizes (−0.0015), t(51199) 0 −0.96, p 0 .34. Table 2 presents the bias estimates for bg200, when g200 0 2 and g300 0 0.2.
Similar results are obtained for bg300. The bias is significantly negative for the uncorrected effect sizes and equals −0.20, t(51199) 0 −255.27, p < .0001, whereas the bias is not signif- icant for the corrected effect sizes, t(51199) 0 −0.00020, p 0 .79. Moreover, an analysis of variance on the deviations reveals a significant difference between the two different models, for both bg200 and bg300 [F(1, 102398) 0 192.06, p < .0001 for bg200, and F (1, 102398) 0 33,695.1, p < .0001 for bg300�. The differences are largest when there is a small number of measurement occasions (I 0 15) and studies (K 0 10). In the following condition, the
largest difference was identified: g200 0 2, g300 0 0, K 0 10, I 0 15, J 0 4, σ2u2 0 0.5, and σ
2 v2 0 2 (with a difference of 0.23).
MSE Similar to the bias, the MSE of the estimated treatment effect depends significantly on the model type; using an analysis of variance on the squared deviations, F(1, 102398) 0 882.77, p < .0001 for bg200 and F(1, 102398) 0 7,076.91, p < .0001 for bg300 . When using the corrected model, the MSE for bg200 and bg300 equals 0.12 and 0.028, respectively, whereas it is 0.18 and 0.070, respectively, for the uncorrected effect sizes. Differences between both mod- els are larger if the number of observations and the number of studies are small (see Table 3 for bg200; similar results are obtained for bg300 ). So especially in these conditions, the modified model is recommended.
Estimates of the standard errors In order to evaluate infer- ences regarding the treatment effects, we constructed confidence intervals around the estimated treatment effects, bg200 and bg300 Therefore, we needed to estimate the standard errors of the estimated treatment effects. Because we obtained 400 estimates of the effects in each condition, the standard deviations of the effect estimates can be regarded as a relatively good estimate of the standard deviation of the sampling distribution and can, therefore, be used as a criterion to evaluate the standard error. We looked at the relative standard error biases, which are the differences between the median standard error estimates and the standard deviation of the estimates of the effect divided by the standard deviation of the estimates ofbg200 andbg300. The relative differences are negative for bg200, which means that the median standard error estimates are smaller than expected. For bg300 , these differences are positive, referring to median standard error estimates larger than expected. The relative standard error biases for both bg200 and bg300 are, on average, larger across the conditions for the uncorrected effect sizes, in comparison with
Table 2 The bias of bg200 in the g200 0 2, and g300 0 0.2 conditions for the constant external event effect over four subsequent measurement occasions Corrected Unorrected
I 0 15 I 0 30 I 0 15 I 0 30
K J σ2u2 σ 2 v2 0 0.5 σ2v2 0 2 σ
2 v2 0 0.5 σ2v2 0 2 σ
2 v2 0 0.5 σ2v2 0 2 σ
2 v2 0 0.5 σ2v2 0 2
10 4 0.5 −0.003 0.007 0.025 −0.036 0.213 0.208 −0.027 0.027
2 0.015 0.002 −0.017 0.014 0.129 0.196 0.012 0.035
7 0.5 −0.026 −0.057 0.024 0.005 −0.093 −0.058 −0.019 −0.074
2 −0.028 −0.015 −0.011 −0.003 −0.099 −0.060 −0.016 −0.026
30 4 0.5 0.009 0.028 0.004 −0.005 0.219 0.185 −0.008 0.013
2 0.018 0.021 0.004 −0.011 0.210 0.222 0.008 0.035
7 0.5 0.023 0.005 0.002 −0.009 −0.075 −0.105 −0.004 −0.016
2 0.001 0.026 −0.006 −0.012 −0.077 −0.088 −0.003 0.006
“Corrected” and “uncorrected” refer, respectively, to corrected effect size and uncorrected effect size for external event effects
552 Behav Res (2013) 45:547–559
the corrected effect sizes. For bg200, the average relative standard error biases equal −1.8 % and −2.0 % for the corrected and uncorrected models, respectively. The average relative standard error biases difference forbg300 for the uncorrected model is 2 %, whereas it is substantial (more than 10 %; Hoogland & Boomsma; 1998) for the uncorrected model (25.7 %). So the difference between the model types becomes more apparent when g300 is estimated, F(1, 254) 0 38.9, p < .0001. The conditions with the largest relative standard error bias when the uncorrected model for bg300 was used tended to coincidence with the conditions where 30 studies, an immediate treatment effect of 2, and a treatment effect on the time trend of 0.2 were involved, with the bias mounting to 107 % in the condition where g200 0 2, g300 0 0.2, K 0 30, J 0 7, I 0 30, σ
2 v2 0 0.5, and
σ2u2 0 0.5.
Coverage proportion We estimated the CP of the 95 % confi- dence intervals, which allowed us to evaluate the interval esti- mates of bg200 and bg300. The confidence intervals were estimated by using the standard errors and the Satterthwaite estimated degrees of freedom. The CP of these confidence intervals was estimated for each of the combinations. A positive significant difference between the corrected model and the uncorrected model in the CP is found for bg200, F(1, 254) 0 27.56, p < .0001 (see Table 4). Also, for bg300, the mean CP depends significantly on the model type, F(1, 254) 0 20.96, p < .0001 (see Table 4). The conditions with a CP less than .93 all have 15 measurements in common and occur when the effect sizes are uncorrected, for both bg200 and bg300. Moreover, for bg300, the CP is not only too small when I 0 15 and K 0 30, but also too
Table 3 The MSE of bg200 in the g200 0 2, and g300 0 0.2 conditions for the constant external event effect over four subsequent measurement occasions Corrected Unorrected
I 0 15 I 0 30 I 0 15 I 0 30
K J σ2u2 σ 2 v2 0 0.5 σ2v2 0 2 σ
2 v2 0 0.5 σ2v2 0 2 σ
2 v2 0 0.5 σ2v2 0 2 σ
2 v2 0 0.5 σ2v2 0 2
10 4 0.5 0.17 0.28 0.11 0.26 0.32 0.43 0.14 0.25
2 0.20 0.32 0.14 0.28 0.31 0.49 0.16 0.36
7 0.5 0.09 0.24 0.07 0.23 0.18 0.31 0.09 0.22
2 0.11 0.26 0.09 0.24 0.20 0.31 0.09 0.28
30 4 0.5 0.06 0.10 0.04 0.09 0.14 0.19 0.04 0.10
2 0.06 0.11 0.04 0.09 0.15 0.20 0.05 0.12
7 0.5 0.03 0.07 0.03 0.08 0.06 0.10 0.03 0.09
2 0.04 0.08 0.03 0.08 0.07 0.10 0.04 0.08
…
Collepals.com Plagiarism Free Papers
Are you looking for custom essay writing service or even dissertation writing services? Just request for our write my paper service, and we'll match you with the best essay writer in your subject! With an exceptional team of professional academic experts in a wide range of subjects, we can guarantee you an unrivaled quality of custom-written papers.
Get ZERO PLAGIARISM, HUMAN WRITTEN ESSAYS
Why Hire Collepals.com writers to do your paper?
Quality- We are experienced and have access to ample research materials.
We write plagiarism Free Content
Confidential- We never share or sell your personal information to third parties.
Support-Chat with us today! We are always waiting to answer all your questions.
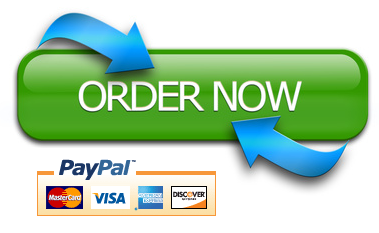