Include previous studies and application in open science that relates to communication (particularly mobile and internet) field
Include previous studies and application in open science that relates to communication (particularly mobile and internet) field. literature (studies, projects(Apple, google…), conferences) have to be international .. .and related to communication. mention at least 3 examples as (studies, projects, conferences) 3 examples for each one of them. Example from Youtube, projects, websites, …. and make a proposal for new idea in open science. it will be proposal and capstone project. EO Open Science.
1
Do You Speak Open Science? Resources and Tips to Learn the Language.
Paola Masuzzo1, 2 – ORCID: 0000-0003-3699-1195, Lennart Martens1,2 – ORCID: 0000- 0003-4277-658X
Author Affiliation 1 Medical Biotechnology Center, VIB, Ghent, Belgium 2 Department of Biochemistry, Ghent University, Ghent, Belgium
Abstract
The internet era, large-scale computing and storage resources, mobile devices, social media, and their high uptake among different groups of people, have all deeply changed the way knowledge is created, communicated, and further deployed. These advances have enabled a radical transformation of the practice of science, which is now more open, more global and collaborative, and closer to society than ever. Open science has therefore become an increasingly important topic. Moreover, as open science is actively pursued by several high-profile funders and institutions, it has fast become a crucial matter to all researchers. However, because this widespread interest in open science has emerged relatively recently, its definition and implementation are constantly shifting and evolving, sometimes leaving researchers in doubt about how to adopt open science, and which are the best practices to follow.
This article therefore aims to be a field guide for scientists who want to perform science in the open, offering resources and tips to make open science happen in the four key areas of data, code, publications and peer-review.
The Rationale for Open Science: Standing on the Shoulders of Giants
One of the most widely used definitions of open science originates from Michael Nielsen [1]: “Open science is the idea that scientific knowledge of all kinds should be openly shared as early as is practical in the discovery process”. With this in mind, the overall goal of open science is to accelerate scientific progress and discoveries and to turn these discoveries into benefits for all. An essential part of this process is therefore to guarantee that all sorts of scientific outputs are publicly available, easily accessible, and discoverable for others to use, re-use, and build upon.
As Mick Watson has recently wondered, “[…] isn’t that just science?” [2]. One of the basic premises of science is that it should be based on a global, collaborative effort, building on open communication of published methods, data, and results. In fact, the concept of discovering truth by building on previous findings can be traced back to at least the 12th century in the metaphor of dwarfs standing on the shoulders of giants: “Nanos gigantum humeris insidentes”1.
While creativity and intuition are contributed to science by individuals, validation and confirmation of scientific findings can only be reached through collaborative efforts, notably peer- driven quality control and cross-validation. Through open inspection and critical, collective analysis, models can be refined, improved, or rejected. As such, conclusions formulated and validated by the efforts of many take prominence over personal opinions and statements, and this
1 Metaphor attributed to Bernard of Chartres, and better known in its English form as found in a 1676 letter of Isaac Newton: “If I have seen further, it is by standing on the shoulders of giants”
PeerJ Preprints | https://doi.org/10.7287/peerj.preprints.2689v1 | CC BY 4.0 Open Access | rec: 3 Jan 2017, publ: 3 Jan 2017
2
is, in the end, what science is about. While science has been based for centuries on an open process of creating and sharing knowledge, the quantity, quality, and speed of scientific output have dramatically changed over time. The beginning of scholarly publication as we intend it today can be traced back to the 17th century with the foundation of the ‘Philosophical Transactions’. Before that, it was not at all unusual for a new discovery to be announced in an encrypted message (e.g., as an anagram) that was usually indecipherable for anyone but the discoverer: both Isaac Newton and Leibniz used this approach. However, since the 17th century, the increasing complexity of research efforts led to more (indirect) collaborations between scientists. This in turn led to the creation of scientific societies, and to the emergence of scientific journals dedicated to the diffusion of scientific research. Paradoxically however, knowledge diffusion has dramatically slowed down over the same time. In his review of Michael Neilsen’s book “Reinventing Discovery” [3], Timo Hannay describes science as “self-serving” and “uncooperative”, “replete with examples of secrecy and resistance to change”, and furthermore defines the natural state of researchers as “one of extreme possessiveness” [4]. Hannay might have a point: the majority of research papers are behind a paywall [5], researchers still fail at making data and metadata available [6], reproducibility is hampered by the lack of appropriate reporting of methodologies [7], software is often not released [8], and peer-review is anonymous and slow [9].
As a reaction, the open science movement was born, almost as a counterculture to the too- closed system that re-emerged over the past few decades. More and more academic and research institutions are currently opening up the science they produce, making the scientific research, produced data and associated papers accessible to all levels of an ever more inquiring society, amateur or professional. And increasingly, major funding agencies are mandating the same. For example, the European Commission requires participants of the H2020 funding framework to adhere to the Open Access mandate and the Open Research Data Pilot. Furthermore, both the National Institutes of Health (NIH) and the Wellcome Trust have developed specific mandates to enforce more open and reproducible research. As a result, practicing open science is no longer only a moral matter, but has become a crucial requirement for the funding, publication, and evaluation of research.
Because the many benefits of open science have already been extensively studied and reported [10–16], this article instead intends to be a user guide for open science. The next sections of this article therefore provide an overview of the key pillars of open science, along with resources and tips to make open science happen in everyday research practices. This collection of resources can then serve as an open science guidebook for early-career researchers, research laboratories, and the scientific community at large.
Four Pillars of Open Science
Almost all scientists today will have bumped into the expression “open science”. As an umbrella term used to cover any kind of change towards availability and accessibility of scientific knowledge, “open science” evokes many different concepts and covers many different fronts, from the right to have free access to scholarly publications (dubbed “open access”), over the demand for a wider public engagement (typically referred to as citizen science), to the development of free tools for collaboration and open peer-review (as implemented in science-oriented social media platforms).
This diversity and perhaps even ambiguity of open science can be explained by the many stakeholders that are directly affected by a changing scientific environment: researchers,
PeerJ Preprints | https://doi.org/10.7287/peerj.preprints.2689v1 | CC BY 4.0 Open Access | rec: 3 Jan 2017, publ: 3 Jan 2017
3
administrators, funders, policy makers, libraries, publishing companies, and even the general public. Five different schools of thought on open science have been identified2, each with their stakeholder groups, their aims, and their tools and methods to achieve and promote these aims [12]. While these schools depict the whole scope of open science, their fundamental aim is to enhance openness in the four widely recognized thematic pillars: open research data, open software code, open access to papers, and open peer-review (Figure 1). The following sections will briefly introduce the rationale for each of these pillars, and will then provide resources for their adoption in daily research practice.
Figure 1: The four pillars of open science discussed in this article. Image adapted from [17], distributed under a CC BY 4.0 International license (http://creativecommons.org/licenses/by/4.0/).
Open Data: Sharing the Main Actor of a Scientific Story
By open data in science we mean data that are freely available on the public internet permitting any user to download, copy, analyze, re-process, or use these for any other purpose without financial, legal, or technical barriers other than those inseparable from gaining access to the internet itself3.
In the digital era, data are more and more considered to be the main part of a scientific publication, while the paper serves the secondary role of describing and disseminating scientific results. This because open data tend to outlive the associated paper. In fact, others (professional researchers as well as interested members from the general public) can conduct re-analyses on these data, and can do so within the context of new questions, leading to new scientific discoveries. In 2015 Borgman identified four rationales for sharing research data: to reproduce research, to make those data that can be considered public assets, available to the public4, to leverage investments in research, and to advance research and innovation [18]. Several studies have furthermore reported that scientific papers accompanied by publicly available data are on average cited more often [19,20], and are moreover characterized by fewer statistical errors and a greater degree of robustness [21].
2 Democratic, Pragmatic, Infrastructure, Public and Measurement 3 see the full Open Definition at: http://opendefinition.org/od/2.0/en/ and the Panton Principles for Open Data in Science at http://pantonprinciples.org 4 Privacy sensitive data for instance, do not belong to this category.
PeerJ Preprints | https://doi.org/10.7287/peerj.preprints.2689v1 | CC BY 4.0 Open Access | rec: 3 Jan 2017, publ: 3 Jan 2017
4
Releasing data, however, is not sufficient by itself. For re-use to happen efficiently, which is ultimately the goal of open data, data sharing needs to become a custom routine, should encompass the full research cycle, and needs to assure long-term preservation. Furthermore, data sharing requires some amount of manual work, and a specific shift in research habits, for which the current credit system in research should accommodate. A nice example of this shift is provided by the journal Psychological Science, which adopted such an incentive for open research data in January 2014, by offering “badges” to acknowledge and signal open practices in publications. To receive an ‘open data’ badge, authors must make all digitally shareable data relevant to the publication available on an open access repository. Similarly, to earn an ‘open materials’ badge, authors must make all digitally shareable materials available on an open access repository. Those who apply for a badge and meet open data or open materials specifications receive the corresponding badge symbol at the top of their paper and provide an explicit statement in the paper including a URL to the data or materials at an open repository. A recent study has shown that these badges are effective incentives to improve the openness, accessibility, and persistence of data and materials that underlie scientific research [22].
Finally, for data sharing to encourage re-use, data curation and metadata annotations are key factors, together with reliable basic infrastructure for data sharing: the availability of data infrastructures that are well curated and well maintained in the long-term, and a rich catalogue of standards and formats that are moreover continuously updated to keep up with shifts in technology and knowledge.
Where to Submit Research Data? General-Purpose and Domain-Specific Repositories
As a general rule, data should be submitted to a repository prior to submission of a relevant manuscript that describes these data. Thus, the authors can point the readers to the location of the data in the manuscript itself, increasing transparency, reproducibility and validation of the results, and aiding efficient peer-review. Two types of such data repositories exist: general-purpose and domain-specific repositories. The former are inter-disciplinary repositories meant to host data for which domain-specific repositories do not exist, as well as general research output (such as posters, presentations, code). The latter on the other hand, are well-established subject or data-type specific repositories that typically serve specific fields. Table 1 lists the most widely used repositories across both types. Although not exhaustive, this list provides a good cross-section of repositories that should be considered both for publication of data, and for the location and retrieval of relevant data for (re)use in research.
A global registry of research data repositories for different scientific disciplines can be found at the Registry of Research Data Repositories (http://www.re3data.org). Furthermore, NCBI and EBI online databases can be found at http://goo.gl/0KwIq8 and http://goo.gl/j3stqD, respectively. Biomed Central suggests a list of possible repositories at https://goo.gl/dBHeZf, while another interesting list, maintained by Nature Scientific Data, can be found at https://goo.gl/G7cLFp. Finally, the Biosharing catalogue includes bioscience databases described according to domain guidelines and standards (https://biosharing.org/databases/, 798 databases listed at the time of writing).
PeerJ Preprints | https://doi.org/10.7287/peerj.preprints.2689v1 | CC BY 4.0 Open Access | rec: 3 Jan 2017, publ: 3 Jan 2017
5
Table 1: A list of general-purpose and domain-specific data repositories (in alphabetical order).
Name Description Domain Website
Cell Image Library
public repository of reviewed and annotated images, videos, and
animations of cells from a variety of organisms
biological imaging http://www.cellimagelib
rary.org
Coherent X-ray Imaging Data
Bank open repository for X-ray images
macromolecular structures
http://www.cxidb.org/id -2.html
Crystallograph y Open
Database
open-access collection of crystal structures of organic, inorganic, metal-organic compounds and
minerals, excluding biopolymers
macromolecular structures
http://www.crystallogra phy.net
DataOne a framework and infrastructure for
Earth observational data environmental and
ecological data https://www.dataone.or
g
Dryad
a resource that makes the data underlying scientific publications discoverable, freely reusable, and
citable
general-purpose http://datadryad.org
Figshare
a repository where users can make all of their research outputs available in
a citable, shareable and discoverable manner
general-purpose https://figshare.com
GenBank the NIH genetic sequence database,
an annotated collection of all publicly available DNA sequences
sequence and omics data
http://www.ncbi.nlm.ni h.gov/genbank/
GEOSS portal a portal for Earth science data environmental and
ecological data www.geoportal.org
Global Biodiversity Information
Facility
a repository containing data about all types of life on Earth, published
according to common data standards
environmental and ecological data
http://www.gbif.org
JCB Data Viewer
a platform to view, analyze and share image data associated with articles published in The Journal of Cell
Biology
biological imaging http://jcb-
dataviewer.rupress.org
Morphbank
an image database documenting a range of specimen-based research,
including comparative anatomy and taxonomy
biological imaging http://www.morphbank.
net
Movebank an online database of animal tracking
data environmental and
ecological data https://www.movebank.
org
NERC data centers
seven centers for: marine, atmospheric, Earth observation, solar
and space physics, terrestrial and
environmental and ecological data
http://www.nerc.ac.uk/r esearch/sites/data/
PeerJ Preprints | https://doi.org/10.7287/peerj.preprints.2689v1 | CC BY 4.0 Open Access | rec: 3 Jan 2017, publ: 3 Jan 2017
6
freshwater, geoscience, and polar and cryosphere data
NeuroVault a repository for statistical maps,
parcellations, and atlases produced by MRI and PET studies
neuroimaging data http://neurovault.org
NIH 3D Print Exchange
a repository with models for 3D printers and tools to create and share
3D-printable models related to biomedical science
3D-printable models http://3dprint.nih.gov
Open Energy Information
a crowdsourced collection of information, data and discussions around multiple aspects of energy
engineering http://en.openei.org
Open Science Framework
a research and workflow management tool and open
repository; allows for integration with several external tools like Dropbox, Github, and Zotero
general-purpose https://osf.io
OpenfMRI a project dedicated to the free and
open sharing of functional magnetic resonance imaging (fMRI) datasets
neuroimaging data https://openfmri.org
OpenTrials
a project to locate, match, and share all publicly accessible data and
documents on all trials conducted on all medicines and other treatments
health data http://opentrials.net
PANGAEA a repository for geospatial data environmental and
ecological data https://www.pangaea.de
PRIDE an archive of protein expression data as determined by mass spectrometry
sequence and omics data
http://www.ebi.ac.uk/pri de/archive/
Protein Data Bank
a databank for 3D protein structures macromolecular
structures http://www.rcsb.org/pdb
/home/home.do
The Knowledge Network for
Biocomplexity
an international repository intended to facilitate ecological and
environmental research
environmental and ecological data
https://knb.ecoinformati cs.org
Uniprot a comprehensive resource for protein sequence and functional annotation
data
sequence and omics data
http://www.uniprot.org
Worldwide Protein Data
Bank
a publicly available repository of macromolecular structural data
macromolecular structures
http://www.wwpdb.org
Zenodo
a repository that supports a wide variety of content including
publications, presentations, images, software (integration with GitHub),
and data
general-purpose https://zenodo.org
PeerJ Preprints | https://doi.org/10.7287/peerj.preprints.2689v1 | CC BY 4.0 Open Access | rec: 3 Jan 2017, publ: 3 Jan 2017
7
Submitting data: points to consider
The following section highlights some key aspects to keep in mind when submitting research data.
x Research materials in a broad sense (essentially any research output such as figures, posters, code, presentations, and media) are best deposited in general-purpose repositories. Domain- specific data on the other hand, are best submitted to a domain-specific repository (see Table 1). Recent surveys have shown that the majority of researchers still prefer to share data as supplementary material to an article, but this is certainly not an optimal solution, because it is essentially a very static representation of data (often also formatted in document rather than data mark-up formats, such as PDF) and therefore does not allow for dynamic inspection and re-use of the data. It may also not represent a long-term data storage solution.
x If researchers wish to publish data sets through a data article, they can target appropriate data journals. Rather than presenting any analysis, results, or conclusions on the data, such a data article focuses on detailed descriptions of these data, and presents arguments about the value of the data for future (re-)analysis. Notable examples of data journals are: GigaScience (BioMed Central, http://gigascience.biomedcentral.com), Scientific Data (Nature Publishing Group, http://www.nature.com/sdata/) and Data in Brief (Elsevier, http://www.journals.elsevier.com/data-in-brief/). A data journal will not normally host data itself but will instead recommend a suitable repository where the data set should be deposited, and then link to it.
x When targeting a particular journal to publish their research, scientists should check for any policies on data. In fact, journals are increasingly requiring authors to deposit the data underlying their articles in a recognized repository, to complement or even replace any in-house facility for supplementary materials. For example, Public Library of Science (PLOS) recommends repositories it recognizes as “trusted within their respective communities” and also points to re3data as a more general source.
x The following questions can assist a researcher in choosing the right repository for their data: o Is the repository well known?
Is it community-recognized (e.g., listed in the re3data registry)? Some repositories are certified, meaning that they have passed a check in terms of reliable and long-term access to the data collections they host, but one should keep in mind that some good repositories are not compliant yet, and this might remain the case for some time.
o Will the repository accept my data? With the obvious exception of general-purpose repositories, most online databases accept data sets that relate to a specific research topic or domain, typically also formatted in a specific way. Three key aspects therefore need to be taken into account: (1) the data must be of a specific data type (e.g., microarrays, or biological imaging); (2) the data must be submitted in a specific data format (most likely an open, standard format instead of proprietary ones); (3) specific legal terms and conditions need to be satisfied (e.g., informed consent forms must be collected for health data).
x Use a recognized waiver or license that is appropriate for data. The OpenDefinition project lists conformant licenses (both for content and data): http://opendefinition.org/licenses/. Importantly, licenses non-conformant to the open definition are also reported: http://opendefinition.org/licenses/nonconformant/. As a general rule, it is important to remember that the use of licenses which limit commercial re-use or limit the production of derivative works by excluding use for specific purposes is discouraged. This because these
PeerJ Preprints | https://doi.org/10.7287/peerj.preprints.2689v1 | CC BY 4.0 Open Access | rec: 3 Jan 2017, publ: 3 Jan 2017
8
licenses can make it quite a bit harder to effectively re-use datasets, and could also prevent (tangential) commercial activities that could be used to support data preservation in the long- term5.
x Share the metadata along with the data. As Gray has put it: “Data is incomprehensible and hence useless unless there is a detailed and clear description of how and when it was gathered, and how the derived data was produced” [23]. Clear metadata make it easier to understand if data are appropriate for a project; without clear metadata data sets can be overlooked or even go unused. Worse yet, such data sets may be misinterpreted. The recently released FAIR (Findability, Accessibility, Interoperability, and Reusability) guidelines are a good starting point to check for efficient metadata reporting [24].
x Whenever possible, use standard file formats. This applies for both data and metadata file formats. The Biosharing registry lists a comprehensive collection of standards for the life sciences (https://biosharing.org/standards/) (663 standards at the time of writing). To ensure that both data and metadata are reported accurately and compliant with community-established standards, use (semantic) validation tools, whenever available.
Open Source: Sustainable Software for Sustainable Science
Open source refers to software that is made available under a license that permits anyone to use, change, improve, or derive from existing source code, and sometimes even to distribute the software6. The case for open source code is straightforward: the code researchers write and use to analyze data is a vital part of the scientific research cycle, and, similar to data, is not only necessary to reproduce and interpret the results and corresponding conclusions, but can also be used to answer novel research questions. Therefore, if researchers write code as a means to obtain results from data, then this code should be released as well [8]. Clear arrangements for the storage and preservation of the code should be made, instructions need to be provided that will allow the code to be compiled and run without issue, and the code should be accompanied by a description of the core functionalities and hard- and software requirements for its use. This in turn means that source code alone is not sufficient: the software environment needs to be described too, including for instance, any linked libraries, any runtime environments or virtual machines, The open source container engine Docker is intended to provide an efficient solution for computational reproducibility (see www.docker.com) [25,26].7
Researchers sometimes prefer not to share code because of a lack of complete and clear documentation. While documentation is undoubtedly essential for code validation and re-use, as a general rule, sharing undocumented code is preferable to not sharing code at all [27]. Another concern that might stop researchers from sharing their code is the fear that they will have to provide full user support afterwards. One solution to this problem is to setup a simple online mailing list (for example through Google), and point all users to ask questions through it. In this way, answers are searchable on the web and available to other users who might have the same issue/question. In fact, this system utilizes a core property of open source code, in that a community can come into being around useful code. This community can then maintain, support, and update this code even in the absence of the original author.
5 see also the Panton Principles for Open Data at: http://pantonprinciples.org 6 see the full Open Source definition at the Open Source Initiative webpage: https://opensource.org/docs/osd 7 see also: http://goo.gl/oba1qN
PeerJ Preprints | https://doi.org/10.7287/peerj.preprints.2689v1 | CC BY 4.0 Open Access | rec: 3 Jan 2017, publ: 3 Jan 2017
9
It should however, be noted that many of the issues with code quality and sharing can actually be addressed by following simple best practices in code organization and planning. For instance, a key tool that all research programmers should incorporate into their workflow is the use of a Version Control System (VCS) such as git [28] or subversion (SVN). A VCS provides a way for taking snapshots of evolving code that allow tracking of changes, and for reverting these if necessary (e.g., after making a change that ends up breaking the functionality of the code). A rapidly growing community of scientists use the Github platform (https://github.com), which is a freely available implementation of the git system, to contribute to collaborative projects, and to review and test code in a transparent and efficient way [29]. Interestingly, GitHub also promises to be a useful tool in assessing part of a researcher’s impact. For example, a repository can be forked (which means there will be adaptations of the code), starred (showing appreciation for the work), pull requests can happen (which show public engagement with the work and the degree of potential collaboration), as well as downloads (which may signal software installations or code use).
Another interesting way to make code available is by integrating it with tools that enable data interrogation and interactive visualization. This approach, known as literate programming [30], seamlessly integrates analysis code, visualization plots, and explanations in the form of narrative text. There are a number of tools available to support this style of research, including Jupyter (for R, Python and Julia, http://jupyter.org), R Markdown (for R, http://rmarkdown.rstudio.com), and matlabweb (for MATLAB, https://www.ctan.org/pkg/matlabweb). With these tools, researchers can create code files (in the case of Jupyter these are called Notebooks8) that can be then shared on Github, in turn allowing other people to directly run these …
Collepals.com Plagiarism Free Papers
Are you looking for custom essay writing service or even dissertation writing services? Just request for our write my paper service, and we'll match you with the best essay writer in your subject! With an exceptional team of professional academic experts in a wide range of subjects, we can guarantee you an unrivaled quality of custom-written papers.
Get ZERO PLAGIARISM, HUMAN WRITTEN ESSAYS
Why Hire Collepals.com writers to do your paper?
Quality- We are experienced and have access to ample research materials.
We write plagiarism Free Content
Confidential- We never share or sell your personal information to third parties.
Support-Chat with us today! We are always waiting to answer all your questions.
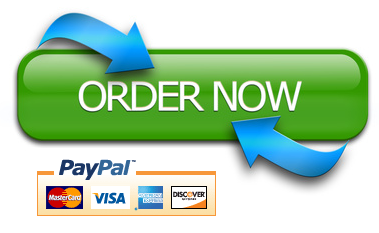