ACCT 597: Tax Transformation and Technology Final Project
Please note that: I need the Initial Draft by April 6th, 2025. The full and complete paper by April 30th, 2025. Please see the attached files for the prompt & requirements of the paper and also the sample research paper of my class. Thank you.
ACCT 597: Tax Transformation and Technology Final Project
Spring 2025
Research Topic: How Artificial Intelligence Will Transform the Role of the Tax Professional
Introduction
o Importance of the tax professional in a corporate environment
o The emergence of artificial intelligence in tax processes
o Objectives and scope of the paper
The Traditional Role of the Tax Professional
o Key responsibilities (compliance, reporting, advisory)
o Challenges in the traditional role (time-intensive tasks, manual errors)
o Skills traditionally required (technical expertise, knowledge of tax law)
New Skills and Expertise Required for Tax Professionals
o Data analytics and AI literacy
o Problem-solving in an AI-assisted environment
o Communication skills for explaining AI-generated results to stakeholders
o Ethical considerations in AI use
The Integration of Artificial Intelligence in Tax
o Overview of AI technologies in tax (RPA, machine learning, NLP)
o Examples of AI tools and their functions (tax compliance automation, data analysis)
o Current adoption trends in the tax profession
Impacts of AI on the Role of Tax Professionals
o Automation of repetitive tasks (e.g., data entry, reconciliation)
o Shift toward strategic and advisory roles
o Increased focus on interpreting AI-generated insights
o Collaboration with AI tools in decision-making
Opportunities for Tax Professionals
o Providing higher-value advisory services
o Enhanced efficiency and productivity
o Leadership in implementing AI-driven tax solutions
o Addressing global tax challenges with AI tools (e.g., BEPS, OECD compliance)
VII. Challenges for Tax Professionals in an AI-Driven Future
o Bridging the skill gap
o Managing resistance to AI adoption
o Ensuring ethical and accurate AI use
o Navigating regulatory changes related to AI
VIII. Examples: AI and the Tax Professional
o Examples of successful AI integration in multinational corporations
o Lessons learned and best practices
IX. Future Trends in the Role of Tax Professionals
o A. AI as a partner, not a replacement
o B. Continuous learning and skill development
o C. Evolution of tax advisory to align with digital transformation
o Global harmonization facilitated by AI
X. Conclusion
o A. Summary of AI’s impact on the tax profession
o B. Recommendations for professionals to adapt and thrive
o C. Future outlook for the profession
XI. References
Include academic sources, industry reports, and real-world examples
Properly cite all sources, including academic journals, books, case studies, and reports relevant to AI in taxation.
Additional Notes: • Paper must be a minimum of 20 pages – this does not include any appendices • MLA format is required. MLA requirements. Citing Generative AI ChatGPT
· Due Date – Initial Draft is Due: April 7th, 2025 by 5:00 PM PST
· Due Date: May 5th, at 5:00 pm PT
· Body of Paper
· 20 Pages
· Doubles spaced
· Ariel 12 pitch font
· Footnotes within body of the paper
· A Table of Contents is required
· Minimum of 20 references
· Title Page
· Index
· Body of Paper – outline
· Appendices
· A Works Cited page is required at the end of the paper which will list the resources cited.
· NO PLAGARISM
,
BRIDGING THE GAP: AI'S TRANSFORMATIVE ROLE IN
MODERN TAXATION Fall 2024 – 597 Final Paper
Dai, Annie [email protected]
Presented to Professor Jeffrey Bogan at California State University, Fullerton
In Partial Fulfillment of the Requirements for the Degree Master of Science In Taxation
1
Contents I. Introduction ………………………………………………………………………………………. 3
Objectives of the Paper ……………………………………………………………………………………………. 3 Overview of Artificial Intelligence (AI) …………………………………………………………………………. 3 What is AI? …………………………………………………………………………………………………………….. 4 The Components and Branches of AI …………………………………………………………………………. 5
1. 1. Learning …………………………………………………………………………………….. 5
2. 2. Reasoning and Decision Making …………………………………………………….. 5
3. 3. Problem-Solving ………………………………………………………………………….. 6
4. 4. Perception ………………………………………………………………………………….. 7
5. 5. Language-Understanding ……………………………………………………………… 7
The History of Artificial Intelligence and Its Impact Today ………………………………………………. 8 II. Theoretical Foundations of AI in Tax ……………………………………………………. 10
AI and Its Technologies in Taxation ……………………………………………………………………………10
6. Machine Learning ………………………………………………………………………….. 10
7. Data Mining ………………………………………………………………………………….. 12
8. Artificial intelligence ……………………………………………………………………….. 16
9. Natural Language Processing (NLP) …………………………………………………. 17
AI in the Context of Digital Transformation ………………………………………………………………….18
10. The role of AI in the broader context of digital transformation in tax ………… 19
11. Comparison with Traditional Tax Technologies …………………………………… 19
Legal and Ethical Considerations ………………………………………………………………………………21
12. Legal Frameworks Governing AI in Tax …………………………………………….. 22
13. Ethical Considerations and Challenges ……………………………………………… 23
III. AI Applications in Taxation …………………………………………………………………. 25
14. AI in Tax Compliance and Reporting …………………………………………………. 26
15. Automation of Tax Filing and Reporting Processes ……………………………… 29
16. AI-Powered Tax Preparation Software ………………………………………………. 29
17. Real-Time Data Analytics for Compliance Monitoring …………………………… 30
AI in Tax Planning and Strategy ………………………………………………………………………………..33
18. Predictive Analytics for Tax Planning ………………………………………………… 33
19. AI Tools for Optimizing Tax Strategies ………………………………………………. 36
2
AI in Tax Audits and Investigations …………………………………………………………………………….37
20. AI in Detecting Tax Fraud and Evasion ……………………………………………… 37
21. Use of AI in Risk Assessment and Audit Selection ………………………………. 38
22. Examples of AI Applications in Government Tax Agencies ……………………. 38
IV. Impact of AI on the Role of Tax Professionals ……………………………………….. 39
Changing Skill Sets and Competencies ………………………………………………………………………40 AI as A Tool for Enhanced Decision-Making ………………………………………………………………..42 The Future of Tax Jobs ……………………………………………………………………………………………42
V. Challenges and Risks of AI in Taxation…………………………………………………. 43
Technical Challenges ………………………………………………………………………………………………43 Legal and Regulatory Challenges ………………………………………………………………………………44 Ethical and Social Risks …………………………………………………………………………………………..45
VI. The Future of AI in the Tax Profession ………………………………………………….. 47
Emerging Trends in AI and Taxation ………………………………………………………………………….48 Preparing for the Future …………………………………………………………………………………………..49
VII. Conclusion ………………………………………………………………………………………. 50
Implications for the Future of Tax Professionals …………………………………………………………..50
23. Long-Term Implications of AI in the Tax Field …………………………………….. 50
Final Thoughts ………………………………………………………………………………………………………..51 Bibliography ……………………………………………………………………………………………………. 52
3
I. Introduction
In today’s rapidly evolving landscape, the integration of artificial intelligence (AI)
in tax has become a transformative force, reshaping the interplay between business,
data, and taxation. This paper aims to explore the complex challenges tax professionals
face in leveraging AI technologies, providing an in-depth examination of the technical
and operational limitations encountered in practical scenarios.
Objectives of the Paper
By delving into these challenges, the paper will propose strategic advancements
and AI-driven enhancements to navigate the intricate and dynamic world of tax
compliance, planning, and reporting. The objective is to offer actionable insights and
innovative solutions that empower tax professionals to address nuanced, multifaceted
issues with greater accuracy, efficiency, and foresight, ensuring alignment with the ever-
changing demands of the global tax environment.
This exploration seeks to bridge the gap between traditional tax methodologies
and cutting-edge AI capabilities, paving the way for a more effective and data-driven
approach to taxation.
Overview of Artificial Intelligence (AI)
Artificial Intelligence (AI) refers to the simulation of human intelligence in
machines designed to perform tasks that typically require human cognitive abilities,
such as learning, reasoning, problem-solving, perception, and decision-making. By
leveraging advanced algorithms, machine learning, and vast amounts of data, AI
systems can adapt and improve over time, driving innovation across industries. From
4
automating mundane tasks to enabling predictive insights and fostering creativity, AI is
transforming how we interact with technology and navigate the complexities of modern
life.
What is AI?
Before diving into the topic, it’s important to understand what AI, or artificial
intelligence, truly means. According to the Collins English Dictionary, "artificial" refers to
something created by humans rather than occurring naturally, often designed to imitate
a natural product. (Collins Dictionaries) "Intelligence," on the other hand, is defined as
the capacity for learning, reasoning, understanding, and other mental activities. (Collins
Dictionaries) Combining these definitions, we can understand AI as the effort to create
human-made systems or products endowed with intelligence designed to mimic human
thought processes.
According to Google Cloud, artificial intelligence (AI) refers to a collection of
advanced technologies that empower computers to perform complex tasks that typically
require human intelligence. These tasks include visual perception, language
understanding and translation, data analysis, and generating actionable
recommendations. AI is not a standalone concept; it is the result of integrating multiple
disciplines, such as computer science, data analytics, statistics, software engineering,
and many other fields, working together to create intelligent systems capable of solving
real-world problems.
5
The Components and Branches of AI
After learning the history and definition of AI, we can take a deep dive into the AI
world, where we first need to understand what the 5 important components of artificial
intelligence are.
1. Learning
Learning in AI resembles how humans acquire knowledge, where individuals
memorize information and apply it when needed. As stated, “One of the essential
components of AI, learning for AI includes the trial-and-error method. The solution keeps
on solving problems until it comes across the right results.” (Bansal) The learning
process involves memorizing various aspects of information, such as solutions to
problems, vocabulary, and terms from different languages. This process, known as rote
learning, is foundational and often evolves into the generalization method. “This learning
method is later implemented using the generalization method.” (Bansal)
2. Reasoning and Decision Making
AI understands information and makes decisions using reasoning. This means it
figures things out based on what it knows. There are two types of reasoning: inductive
and deductive. Deductive reasoning gives clear and definite answers, while inductive
reasoning is used in situations where the results are uncertain and not guaranteed.
6
3. Problem-Solving
AI solves problems using techniques such as planning, searching, and
optimization. These methods help AI find the best way to reach a solution. Some
techniques are special-purpose methods, which are designed to handle specific types of
Figure 1
Components of AI. Image from "What are the Components of AI?" ellow.io, 11 Jan. 2024,
https://ellow.io/components-of-ai/.
7
problems by focusing on their unique features. Others are general-purpose methods,
which are more flexible and can be applied to a variety of different problems. Problem-
solving in AI often works by breaking the task into smaller steps. This process involves
gradually reducing the differences between the current situation (where things are now)
and the goal (the desired outcome) until the problem is completely solved.
4. Perception
AI understands its surroundings by collecting and interpreting information, similar
to how humans use their senses. It processes this data to study scenes, recognize
objects, and figure out how they relate to each other and their features. This ability,
called perception, is very important for tasks like understanding images and recognizing
speech.
5. Language-Understanding
"In simpler terms, language can be defined as a set of different system signs that
justify their means using convention. As one of the widely used artificial intelligence
components, language understanding uses distinctive types of language over different
forms of natural meaning, exemplified overstatements.” (Bansal) AI processes
language by understanding and creating natural language. It uses techniques such as
natural language understanding, machine translation, and text generation to work with
language effectively. These capabilities power tools like chatbots, translation apps, and
sentiment analysis, allowing them to perform smoothly and interact naturally.
Moreover, artificial Intelligence is divided into five main branches, each playing a
unique role in creating intelligent systems. Machine Learning (ML) focuses on enabling
8
machines to learn from data and algorithms without needing explicit programming,
improving adaptability and decision-making. Deep Learning (DL), a subset of ML, uses
neural networks inspired by the human brain to analyze complex data features and
perform tasks like language processing in tools like Alexa or Google Home. Natural
Language Processing (NLP) allows AI to understand and interpret spoken and written
language, making technologies like chatbots, virtual assistants, and sentiment analysis
possible. Robotics combines AI with physical machines to create robots capable of
performing tasks autonomously in industries like healthcare and manufacturing. Lastly,
Fuzzy Logic helps AI handle uncertainties by working with "if-then" rules, improving
decision-making in real-world scenarios, such as determining braking force in automatic
systems. Together, these branches build the foundation for AI’s versatile applications.
The History of Artificial Intelligence and Its Impact Today
AI has become a widely discussed topic today, but its origins trace back much
further, with foundational concepts emerging in the early 20th century. In 1921, Czech
playwright Karel Čapek introduced the term "robots" in his science fiction play,
presenting the idea of "artificial people" to the public. By the mid-20th century, the field
took a significant leap when Alan Turing published his seminal paper, "Computing
Machinery and Intelligence" (Turing), which introduced the concept of the Turing Test
(Oppy and Dowe), originally referred to as the "imitation game." This test became the
benchmark for assessing machine intelligence. It was during this period that the term
"artificial intelligence" was officially coined, marking the formal beginning of AI as a field
of study.
9
The evolution of AI has seen both highs and lows over the decades. The late
1980s, known as the "AI Boom," marked a period of rapid advancements bolstered by
government support and breakthroughs in research. However, this momentum was
short-lived, as the "AI Winter" (1987-1993) brought a decline in interest and funding due
to high costs and limited returns, along with setbacks in expert systems and strategic
computing projects. Despite this, the 1990s to early 2000s saw significant progress,
including AI’s entry into everyday life with innovations like speech recognition software
and the Roomba, as well as groundbreaking achievements such as an AI defeating a
world chess champion. From 2012 to the present, advancements in artificial general
intelligence have transformed AI into a common part of daily life, popularizing
technologies like virtual assistants, search engines, deep learning, and big data.
According to Wolters Kluwer, “Today, artificial intelligence is transforming the way
tax and accounting professionals do their work. Rapid advancements in AI are making it
easier than ever for accounting firms to speed up routine tasks, analyze massive
amounts of financial data, spot patterns and anomalies, reduce human error, and keep
up with changing laws and regulations.” (Wolters Kluwer) This transformation allows tax
and accounting professionals to focus more on strategic decision-making and advisory
roles rather than spending time on repetitive, manual tasks. AI-powered tools not only
enhance efficiency but also improve accuracy, enabling firms to provide better insights
and value to their clients. As regulations become increasingly complex and data
volumes grow, integrating AI into tax and accounting workflows is becoming essential
for staying competitive and ensuring compliance in a rapidly evolving industry.
10
II. Theoretical Foundations of AI in Tax
AI and Its Technologies in Taxation
When it comes to Tax world, we heard so much about data analytics, but in the details,
we need understanding the some of key components that make data analytics famous
which is Machine Learning, data mining, artificial intelligence and more.
Machine Learning
Over the past decade, Artificial Intelligence (AI) and Machine Learning (ML)
technologies have revolutionized the tax landscape, transforming how tax authorities
and businesses operate. These tools are not a threat but a significant opportunity to
enhance efficiency, reduce costs, and improve outcomes. Governments and
organizations worldwide are increasingly adopting AI and ML to modernize tax
compliance and fraud detection processes.
Machine learning, often misunderstood due to its portrayal in popular media, is a
practical tool that uncovers hidden patterns in large datasets and progressively
improves its performance. Instead of mimicking human intelligence, its purpose is to
provide actionable insights, reducing fraud, waste, and abuse across various industries,
including tax administration. Tax agencies leverage machine learning techniques to
strengthen fraud detection, streamline audits, and reduce biases in taxpayer
evaluations. Supervised learning, for example, uses past cases of fraud and audits to
identify patterns and correlations, enabling systems to predict similar fraudulent
activities in the future. This approach helps auditors focus on high-risk areas while
11
learning from both successes and failures, improving the overall accuracy of audits and
reducing biases.
Unsupervised learning, on the other hand, explores unknown areas by identifying
outliers or anomalies without prior case data. Techniques like clustering group similar
tax returns, flagging outliers for further investigation. This approach is particularly
valuable in uncovering high-risk taxpayer segments or non-compliant activities that
traditional methods often overlook. Both supervised and unsupervised learning
Figure 2
Types of machine learning. (Oluwole, "What Are the Types of Machine Learning?")
https://python.plainenglish.io/what-are-the-types-of-machine-learning-540b15dc467f
12
complement each other, providing tax authorities with robust tools for fraud detection
and compliance. Supervised learning enhances the detection of known fraud patterns,
while unsupervised learning uncovers new, hidden risks.
Machine learning’s adaptability allows tax agencies to create dynamic models that
evolve with new data. This “feedback loop” continuously refines fraud detection
capabilities and ensures predictive models remain accurate over time. These systems
can even alert auditors when models lose accuracy, ensuring continuous optimization.
While machine learning cannot eliminate all fraud, it significantly strengthens the fight
against tax evasion and abuse. By reducing fraud, governments can reclaim funds that
rightfully belong to the public, ensuring they are used to improve programs and
services. As tax systems grow increasingly complex, machine learning provides a
powerful, evolving tool to close gaps and protect public funds.
Data Mining
Tax risk detection using data mining and AI technologies has emerged as a critical focus
area in modern tax administration. The ability to extract insights from vast datasets has
made data mining indispensable for improving tax compliance, identifying irregularities,
and mitigating risks. As illustrated in Fig. 3, the growing body of research highlights the
increasing interest among scholars and practitioners in leveraging data mining for tax
risk detection. Over the past two decades, the number of published studies has
exhibited a consistent upward trend, emphasizing the importance of this field in shaping
the future of tax administration.
13
This increased attention reflects the significant benefits data mining offers to tax
administrations. By employing advanced techniques, tax authorities and professionals
can streamline the process of identifying risks, understanding challenges, and
uncovering opportunities. These capabilities allow for more efficient and proactive tax
compliance strategies, reducing manual effort and improving accuracy. Moreover, the
application of data mining provides a clearer picture of taxpayer behavior, enabling
authorities to focus resources on high-risk cases while minimizing unnecessary audits.
Figure 3.
Research and development trends of tax risk detection.
(Zheng et al., "A Survey of Tax Risk Detection Using Data Mining Techniques")
14
Data mining in tax risk detection is divided into two primary branches, as illustrated in
Fig. 4: Non-Relationship-Based Methods and Relationship-Based Methods. Each
<p
Collepals.com Plagiarism Free Papers
Are you looking for custom essay writing service or even dissertation writing services? Just request for our write my paper service, and we'll match you with the best essay writer in your subject! With an exceptional team of professional academic experts in a wide range of subjects, we can guarantee you an unrivaled quality of custom-written papers.
Get ZERO PLAGIARISM, HUMAN WRITTEN ESSAYS
Why Hire Collepals.com writers to do your paper?
Quality- We are experienced and have access to ample research materials.
We write plagiarism Free Content
Confidential- We never share or sell your personal information to third parties.
Support-Chat with us today! We are always waiting to answer all your questions.
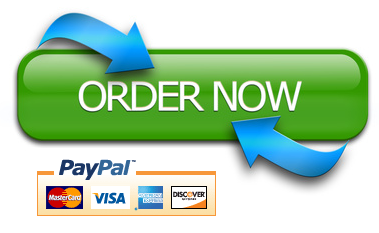