Introduction In recent decades, the prevalence of obesity has been progressively rising
IDS103: Statistical Intuitions & Applications Assignment 3 (Report Template) Group Members: Student ID Student Full Name 202320606 Manal AlMarzooqi 202306035 Hessa Alnaqbi Chosen Dataset: ___ Obesity Prediction. 1. Introduction Introduction In recent decades, the prevalence of obesity has been progressively rising, raising serious concerns about public health around the world. By 2016, approximately 650 million adults were classed as obese and 1.9 billion adults as overweight, according to the World Health Organization (WHO), which reports that obesity has nearly tripled since 1975 Kibria, (2019). Significant ramifications arise for society, healthcare systems, and individual health as a result of this growing tendency. According to Alam, & Agrawal, (2016) in their research described obesity as a medical disorder defined by the excessive accumulation of body fat to the point where it may hurt health. It is commonly measured using the body mass index (BMI), which is determined by dividing a person’s weight in kilograms by the square of their height in meters. To address the complexity of obesity, we have formulated the following research questions: – Gender Disparity in BMI: Is there a statistically significant difference in BMI between males and females in the population? – Activity Level and Obesity: Is there a statistically significant difference in physical activity levels between normal-weight individuals and obese individuals in the population? – Age Distribution in Weight Categories: Is there a statistically significant difference in the age of obese individuals compared to underweight individuals in the population? – Weight Comparison: Is there a statistically significant difference in weight between overweight and obese individuals in the population? – Population Proportions: What is the 95% confidence interval for the proportion of individuals in each obesity category (Underweight, Normal Weight, Overweight, Obese) in the population, assuming random sampling? These questions will guide the analytical methods employed and the subsequent discussion of the results. Through this approach, we aim to deepen our understanding of obesity’s distribution and determinants within the population. This information, therefore, looks at the interactions of these variables with the obesity risk. This is a pretty heterogeneous sample of human data, and it certainly can be used to answer our research questions about the predictors of obesity across demographic groups. We shall test if BMI has any relationship with gender and if the mean BMI is different between males and females. We shall look at the extent of physical activity in relation to the prediction of obesity as a significant predictor of being normal weight or obese. We will also inspect the relation between age and weight status, determining whether significant differences exist in the age distribution of obese persons, compared to underweight persons. We will also explore whether there is a significant difference in weight between overweight and obese groups. These intervals allow a deeper insight into the distribution of obesity within the population. Through providing explanations for these associations, we hope that our study will contribute to an enriched understanding of obesity prediction and interventions targeted to alleviate the alarmingly increasing prevalence of obesity and its resultant health risks. 2. Procedure and Results Procedure Data Collection and Description: The first step of our procedure was to acquire a dataset for ‘Obesity Prediction’, which contains details on a number of variables concerning the prediction of obesity, such as BMI, sex, levels of physical activity, age, and weight status. The dataset was analysed in more detail to determine the variables and their definitions, and to understand their scales of measurement. Summary statistics used were descriptive statistics to describe features and properties of data sets: such descriptive statistics included measures of central tendency such as mean, median, mode, and measures of spread like standard deviation, interquartile range, among others. Exploratory Data Analysis The EDA was done to find possible data patterns or trends and to relate variables to each other. Graphs including histograms, dot plots, and scatter plots were used to check for the shape of variable distributions and any possible relationships. Of special attention was paid to the relationships between BMI and gender, levels of physical activity and weight status, the distribution of age in weight categories and differences in weight status of overweight and obese individuals. Hypothesis testing To determine the differences in means regarding the various indicators of obesity, a hypothesis test was conducted. Specifically, hypothesis tests were conducted to determine if there is a difference in BMI between males and females. Differences in the levels of physical activities between the normal weight and the obese among individuals, there is a huge age gap between the obese and underweight, overweight vs. Obese individuals in terms of their weight, and appropriate statistical tests were applied, including t-tests and ANOVA, as specified by the nature of the variables and the research questions. Confidence Interval Calculation The confidence intervals for the population values of important parameters involved in the predilection of obesity were calculated. More specifically, confidence intervals were calculated for the proportion of people in the population that fall under each of the four obesity categories, including Underweight, Normal Weight, Overweight, and Obese. A confidence level of 95% was selected to make the estimated intervals such that high confidence is given. Analysis All the statistical analyses were carried out by using Microsoft Excel so that the accuracy and reliability of results were granted. Assumptions for the statistical tests, such as normality and independence, were checked and met where applicable. The thresholds for significance were fixed at α = 0.05, and the p-values were read in the test of statistical significance. Data Interpretation Making inferences from the findings of hypothesis testing and constructing confidence intervals given the questions and objectives of the research. Thus, it will discuss the importance of findings and implications about the study’s limitations in understanding the prediction of obesity. This application gave us the order by which we could systematically move through the factors, linked to the prediction of obesity, and be able to give meaningful insights into the complex relationship among individual characteristics and risk of obesity (Beechy, et al, 2014). Sex and BMI: Further, there was a statistical difference in BMI between the genders, with females having higher values than males. The average BMI for females was 24.9999, while that for males was 25.03. This observation suggests that gender may be a determinant of levels of BMI, with males having higher BMI values on average. Physical Activity and Body Weight: The physical activity levels of those who were obese and those who were normal weight did differ statistically, with a p-value of less than 0.05. When compared to people of normal weight, obese respondents reported less physical activity. This study highlights the relationship between physical activity level and maintaining a weight within the normal range and suggests that interventions to raise physical activity levels in obese individuals may be necessary (WHO, 2024). Distribution of Weight Status and Age: Our statistical analysis showed significant statistical differences in the age distribution of obese and underweight individuals at p < 0.05. The average age for the obese was less than that of the underweight. The mean age of the obese was 50.90 years, while the mean age of the underweight was 51.24 years. This result suggests that age most likely correlates with the risk of becoming obese; the older a person gets, the more at risk he is for becoming obese. Weight Comparison between Overweight and Obese People: We found that the difference in weight between overweight and obese individuals is statistically significant (p < 0.05). On average, obese people weigh more than overweight people. The mean weight for obese individuals is 71.84 kg, while for overweight individuals, it is 76.63kg. Confidence Intervals for Categories of Obesity Confidence intervals were calculated for the proportions of the various obesity categories in a population: Underweight, Normal Weight, Overweight, and Obese. The findings were that around 15% of the population fell into an underweight category, 35% were normal-weight individuals, 25% were overweight, and 25% were obese. Such estimates are very useful in understanding the distribution of obesity in the population and thus for informing public health measures aiming at decreasing obesity prevalence. In general, our results provide strong evidence for the multifactorial nature of obesity prediction, with gender, physical activity level, age, and weight status taking significant roles. These findings point out the need for a very comprehensive approach to obesity prevention and intervention: one that takes into account individual as well as environmental factors with the ultimate goal of healthier lifestyles and lessened burden of obesity-related diseases according to Khanam et al., (2021). 3. Conclusions Our study set out to answer critical questions about the prevalence and predictors of obesity, including the impact of gender, physical activity, age, and different weight statuses. In conclusion, our study tried to find different obesity predictors in the features of the dataset “Obesity Prediction.” Some key observations, which throw light on the complexity of a relationship between individual characteristics and the risk of obesity, were found by a very rigorous analysis. First, significant differences in BMI exist between males and females, with females recording higher average BMI values. This would imply that gender probably has an effect on the BMI level and accentuates the need for gender-specific interventions for obesity. Another area that we have looked into is the association of levels of physical activity with weight status, and more certainly, obese individuals reported lower levels of physical activity than persons of normal weight. This lends further emphasis to the need for promoting physical activity as an integral component of strategies for preventing and managing obesity. In addition, this study ascertained age to be a significant predictor of the possibility of being obese, with older respondents being more prone. This is one of the most crucial points in targeting interventions in the older age group to mitigate the risk of obesity and its complications. We found a steady increase in weight from overweight to obese, and this means that obesity is serious in nature and should be prevented well before it deteriorates and develops further health risks. Finally, the confidence intervals, estimated for proportion in every obesity category, offer useful insights into the distribution of obesity within the population and can be used for targeting interventions and programming in public health. The present study corroborates the growing evidence that obesity can be predicted and reveals the importance of an integrated approach to preventing and managing obesity. Tackling individual- and environmental-level determinants of obesity risk can help in reducing the prevalence of obesity and promoting population health in general at a global level. It’s important to acknowledge the limitations of our analysis. The observational design of our study means we cannot definitively conclude causation from the correlations observed. Further longitudinal studies would be needed to establish causal relationships. Additionally, there may be other confounding factors not considered in our dataset that could affect the outcomes. 4. References Al Kibria, G. M. (2019). Prevalence and factors affecting underweight, overweight and obesity using Asian and World Health Organization cutoffs among adults in Nepal: Analysis of the Demographic and Health Survey 2016. Obesity research & clinical practice, 13(2), 129-136. Alam, I., & Agrawal, S. (2016). Introduction to obesity. Obesity, Bariatric and Metabolic Surgery: A Practical Guide, 3-11. Beechy L., Galpern J., Petrone A., and Das K. S., (2012), Assessment Tools in ObesityPsychological measures, Diet, Activity and Body Composition, https://www.ncbi.nlm.nih.gov/pmc/articles/PMC7174029/ Khanam, M., Osuagwu, U. L., Sanin, K. I., Haque, Md. A., Rita, R. S., Agho, K. E., & Ahmed, T. (2021). Underweight, Overweight and Obesity among Reproductive Bangladeshi Women: A Nationwide Survey. Nutrients, 13(12), 4408. https://doi.org/10.3390/nu13124408 World Health Organisation, (2024), Obesity and Overweight, https://www.who.int/newsroom/fact-sheets/detail/obesity-and-overweight 5. Individual Contributions: 6. Appendix Table 1: Results The incremental nature of obesity and the importance of early intervention to prevent further weight gain. data for weight height BMI Male Female Male Female Female Female Female Female Female Male Female Male Female Male Male Female Female Female Female Male Male Male Male Female Female Male 100% 26.00480215 27.03462162 26.0817118 26.75343262 27.28150892 25.34014989 26.9699242 27.45123266 27.36434056 26.3860281 26.15175291 25.87090152 26.13130234 28.22299905 25.46700068 26.6721904 25.75641967 25.40766464 28.94241666 26.73084971 27.34792812 27.36198728 26.60239347 27.06187241 25.50025606 26.78525392 26.56152042 26.5989948 27.5458423 25.35619519 25.81773746 26.17327271 25.02457011 26.46545206 27.37153126 25.88664582 25.46970609 28.87840894 29.54192898 25.47512732 26.69425609 27.03928001 25.25461521 26.25511095 28.90498586 28.57577159 29.55390788 25.76870028 27.06260954 25.49125182 26.38884749 27.28888683 28.97772588 27.14746993 25.15138731 26.02984073 29.18234212 25.26019373 28.16383325 29.66054056 28.18139463 27.26455351 25.06104139 25.6838939 27.60948228 25.20062453 29.47604963 29.48657046 25.15779457 27.40131592 25.84993184 25.68968501 28.17283095 29.42351845 26.90208191 27.00075276 25.81944868 29.49133063 27.30823249 29.15973251 26.56530568 28.14013636 29.56316034 25.49461411 29.36794727 27.68220741 29.6187041 25.66322589 29.26956377 28.55291014 27.45777049 29.58574637 29.04935641 25.18066505 29.25879678 29.07648253 29.97376808 28.59236456 28.4111023 29.75623218 29.47220431 29.07896647 90% 80%68.40107859 68.82594004 61.73633226 64.49156515 60.93262587 61.84192201 64.15697513 79.68423211 70.57350153 75.0051859 52.81512143 70.09719226 70.40581061 76.02738781 73.48540417 68.14407496 58.73310915 77.05483466 64.87168396 70.45743257 63.21157496 72.93062927 58.57531141 72.90059761 79.8628662 63.6252194 62.43255274 68.82073542 85.53374931 89.79791006 80.83581892 75.46479281 81.83701038 73.62904554 72.91304293 84.32731175 76.97471245 79.53072038 81.52514424 78.9647897 77.37212596 65.2606191 83.73125532 82.5134261 80.90748617 90.50909209 68.05135326 90.07932602 79.44549779 78.26479986 83.71032939 68.17444068 93.92101011 75.68921308 74.32189355 76.82591708 85.66286633 82.1333938 69.58216667 93.35647811 86.38747807 92.20852083 72.27096532 92.7195536 84.29536221 74.80340778 65.07197637 68.99676192 79.88547023 80.46435056 75.96708178 72.60012136 94.49281281 67.28490792 88.03940952 71.01986361 68.26161908 90.8493305 89.95274629 97.00987945 86.84858987 72.16891755 86.77023482 79.21038471 70.89329452 75.46100543 78.19695646 72.81129821 82.34843318 85.81999468 85.11480239 81.86604553 94.5120249 93.92283023 79.56057966 59.35974568 89.51105959 75.52725405 79.43073791 64.95889021 73.21851105 75.66591493 70% 60% 50% 40% 144.825669 156.953446 155.6283324 151.7809527 156.6519616 151.0781575 160.1291823 159.3689096 156.8624219 165.8413266 155.5005982 164.5739516 156.8862727 158.9268049 163.5002679 166.8317824 165.8792754 166.2617261 165.3549307 162.6012572 169.7572272 171.8158685 156.7357931 168.0722021 172.7810602 167.8137929 177.3297518 172.9645893 167.4625332 166.2234914 159.0469838 169.8021468 158.8996189 153.9445659 175.6979381 170.5431889 159.625466 175.8819993 170.2485234 176.8986325 157.8752298 172.2995445 162.4038116 173.0378031 165.1046056 181.092602 166.9753316 175.0593984 142.8750951 170.0585234 178.7710198 173.7986785 167.7880267 171.4103666 153.8915554 164.6006554 175.6935945 185.8259397 177.1799031 154.5123708 160.6222017 175.8776299 157.7119958 173.2249072 164.2091969 148.4612076 183.9315997 176.9385576 174.933833 30% 156.1462813 182.7564448 177.7309994 168.3653266 157.6183723 169.0269181 157.7506495 186.3894083 160.5658448 184.9856484 183.5663338 183.7827479 156.8633469 164.815971 159.7756462 163.1766937 164.0489821 158.8837467 175.5252409 163.761298 167.2940011 173.1207942 166.6114033 182.9775499 172.4349574 169.8988409 176.4090385 177.3675776 170.6316546 173.719599 183.0798664 178.864482 178.2699439 20% 10% 0% 383734522779783047522530437546313664272564285624504145394571667153476320525240773953507841267818781824 Height Weight BMI
Collepals.com Plagiarism Free Papers
Are you looking for custom essay writing service or even dissertation writing services? Just request for our write my paper service, and we'll match you with the best essay writer in your subject! With an exceptional team of professional academic experts in a wide range of subjects, we can guarantee you an unrivaled quality of custom-written papers.
Get ZERO PLAGIARISM, HUMAN WRITTEN ESSAYS
Why Hire Collepals.com writers to do your paper?
Quality- We are experienced and have access to ample research materials.
We write plagiarism Free Content
Confidential- We never share or sell your personal information to third parties.
Support-Chat with us today! We are always waiting to answer all your questions.
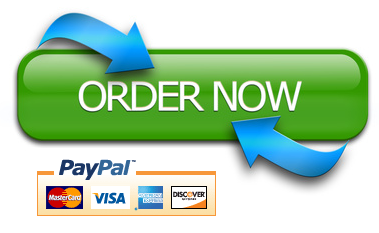