Discussion Thread: Variables, Z Scores, Population and Output reply to 1 of your peers’ responses to D2.1 and D2.2? ? The student must then post 1 reply to another student’s post. The reply
Discussion Thread: Variables, Z Scores, Population and Output reply to 1 of your peers' responses to D2.1 and D2.2
The student must then post 1 reply to another student’s post. The reply must summarize the
student’s findings and indicate areas of agreement, disagreement, and improvement. It must be
supported with scholarly citations in the latest APA format and corresponding list of references.
The minimum word count for Integrating Faith and Learning discussion reply is 250 words.
Herve Ngate
Quantitative Research Methods, BUSI 820-B05
May 23, 2024
Discussion Week 2: Variables, Z Scores, Population and Output
D2.3.1 “If you have categorical, ordered data (such as low income, middle income, high income) what type of measurement would you have? Why?”
According to Morgan et al. (2020), if you have categorical, ordered data such as low income, middle income, and high income, you will have an ordinal level of measurement because ordinal data is a type of categorical data with a natural order or ranking. In this case, the income categories have a clear order from low to middle income and then to high income. This type of measurement allows for a meaningful comparison of the income categories based on their relative positions in the order.
D2.3.2 (a) Compare and contrast nominal, dichotomous, ordinal, and normal variables.
– Nominal variables are categorical variables with no inherent order or ranking. Examples include gender, ethnicity, or marital status.
– Dichotomous variables are a specific type of nominal variable with only two categories. Examples include yes/no responses, true/false answers, or presence/absence of a characteristic (Shreffler and Huecker, 2023).
– Ordinal variables are categorical variables with a natural order or ranking. Examples include Likert scale responses (e.g., strongly disagree, disagree, neutral, agree, strongly agree) or educational levels (e.g., high school, college, graduate school).
– Normal variables are measured on a scale with equal intervals between values and have a meaningful zero point. These variables can take any numerical value and are typically used for quantitative measurements such as height, weight, or temperature.
D2.3.2 (b) In social science research, why isn't it important to distinguish between interval and ratio variables?
In social science research, it is not always essential to distinguish between interval and ratio variables because they involve quantitative measurement. In addition, both variables can often be treated similarly in statistical analysis when using them with the term scale in SPSS (Morgan et al., 2020). Both interval and ratio variables can be subjected to statistical techniques such as mean, standard deviation, correlation, and regression analysis.
D2.3.3 What percent of the area under the standard normal curve is within one standard deviation of (above or below) the mean? What does this tell you about scores that are more than one standard deviation away from the mean?
The standard normal distribution follows a bell-shaped "normal curve". In this distribution, based on the Empirical Rule around 68% of the zone “under the curve falls within one standard deviation of the mean” (Ross, 2021).
Therefore, considering the area under the standard normal curve, approximately 34% is within one standard deviation above the mean, and approximately 34% is within one standard deviation below the mean. These two percentages sum up to a total of roughly 68%.
D2.3.4 (a) How do z scores relate to the normal curve?
The normal curve, also known as the standard normal distribution or Z-distribution, is a specific type of distribution with a mean of 0 and a standard deviation of 1. The z-scores correspond to specific percentiles on the normal curve, enabling us to determine the proportion of scores below or above a particular z-score. Therefore, Z-scores relate to the normal curve by indicating the number of standard deviations a specific score is from the mean.
D2.3.4 (b) How would you interpret a z score of -3.0?
A z-score of -3.0 indicates that the raw score is three standard deviations below the mean. In other words, it suggests that the score is significantly lower than the mean value. Since the normal distribution is symmetrical, a z-score of -3.0 indicates that the raw score is in the left tail of the distribution, far away from the mean.
D2.3.4 (c) What percentage of scores is between a z of -2 and a z of +2?
The Empirical Rule states that approximately “95% of the scores fall within two standard deviations of the mean in a normal distribution” (Ross, 2021). Therefore, between a z-score of -2 and a z-score of +2, we can expect approximately 95% of the scores to lie within this range. This means 95% of the scores are within two standard deviations above and below the mean.
D2.3.5 “Why should you not use a frequency polygon if you have nominal data? What would be better to use to display nominal data?”
A frequency polygon represents the connection of midpoints of each category's bar in a histogram. According to Morgan et al. (2020), you should not use a frequency polygon to display nominal data because nominal data represents categories or groups with no inherent order or ranking. However, since nominal data has no natural order, connecting the midpoints could create a misleading visual representation.
A better option is to use a bar chart to display nominal data (Morgan et al., 2020). This graphical representation uses rectangular bars to represent the frequencies or proportions of each category. This chart type is suitable for displaying nominal data as it allows for easy comparison of the frequencies or proportions between different categories.
References
Morgan, George A., Barrett, Karen C., Leech, Nancy L., & Gloeckner, Gene W. (2020). IBM SPSS for Introductory Statistics. Use and Interpretation. Sixth Edition. Routledge.
Ross, S. M. (2021). Descriptive statistics. In Elsevier eBooks, 11–61 . https://doi.org/10.1016/b978-0-12-824346-6.00011-9
Shreffler, J., & Huecker, M. R. (2023). Types of variables and commonly used statistical designs. StatPearls – NCBI Bookshelf. https://www.ncbi.nlm.nih.gov/books/NBK557882/
Discussion Board: Variables, Z Scores, Population and Output
Wendy Davis
BUSI820: Quantitative Research Methods (B05)
May 23, 2024
Discussion Board #2
School of Business, Liberty University
Author Note
Wendy Davis
I have no known conflict of interest to disclose.
Correspondence concerning this article should be addressed to Wendy Davis
Email: [email protected]
Discussion Board: Variables, Z Scores, Population and Output
Variables provide information that needs to be captured when completing research. Understanding the several types of data sets assists the research when displaying the results. The SPSS system provides the researcher with the formulas needed to display the data most beneficially. Understanding which type of data should be associated with a specific graph is vital to accurate research results.
If you have categorical, ordered data (such as low income, middle income, high income) what type of measurement would you have?
The categorical data set low, middle, and high income would be measured as Nominal variables. Morgan et al. (2021) explain that “nominal variables are two or more unordered categories” (p. 49). The three types of income have not been assigned a specific value that would change the type of measurement.
Why?
The nominal variables advise that the different incomes are measured the same. Each type of income would be assigned a number to assist with data collection (Morgan et al., 2021). To ensure data consistency, the researcher will use a codebook to explain how the numerical information relates to the variables.
Compare and contrast nominal, dichotomous, ordinal, and normal variables.
Nominal, dichotomous, ordinal, and normal variables are measurements for distinct categories of variables. Each type of measurement explains how the categories impact the data set. Research is dependent on constant measurements of data sets. Providing specific information about the data set explains to the audience what type of categories are being measured. The measurements are different based on what the researcher is explaining. Morgan et al. (2021) advise that ordinal measures three or more categories and determines if they are equal. Ordinal is different from nominal because the determination of the categories is not part of the measurement.
In social science research, why isn’t it important to distinguish between interval and ratio variables?
Morgan et al. (2021) explain interval variables as categories of data that are “different between levels are equal but have no true zero” (p. 49). Morgan et al. (2021) explain ratio variables as categories of data that are “different between levels are equal and there is a true zero” (p.49). Understanding the difference between interval and ratio variables is important in social science research to explain human behavior. Defining the type of measurement used to explain the behavior is important for the audience. Defining the type of measurement is how the researcher explains the research question and hypothesis.
What percent of the area under the standard normal curve is within one standard deviation of (above or below) the mean?
The percentage of the area under the standard normal curve is within one standard deviation of the mean is 68 percent. The percentage of the area under the standard normal curve that is within one standard deviation above or below the mean is 34 percent (Morgan et al., 2021).
What does this tell you about scores that are more than one standard deviation away from the mean?
The scores that are more than one standard deviation away from the mean need to have a conversion score associated with the value (Morgan et al., 2021). The conversions are referred to as z-scores.
How do z scores relate to the normal curve?
Morgan et al. (2021) explain that z-scores are referred to as the normal distribution based on the number of deviations. The z-score explains how the curve remains to the right of the z-score value.
How would you interpret a z score of –3.0?
A z-score of -3.0 is interpreted as – three standard deviations away from the mean.
What percentage of scores is between a z of –2 and a z of +2?
The percentage of the score that falls between a z of -2 and a z of +2 is 95 percent.
Why is this important?
Understanding that -1.0 and +1.0 must be equal because the curve has the same area on each side to represent a normal curve. Morgan et al. (2021) explain that values that fall beyond two standard deviations are rare.
Why should you not use a frequency polygon if you have nominal data?
Frequency Polygon connects two points between the categories of data (Morgan et al., 2021). Nominal data explains two or more categories, but they are unordered. The Frequency Polygon would not represent the data accurately due to the type of information this graph provides.
What would be better to use to display nominal data?
The best way to display nominal data is a bar graph.
References
Morgan, G., Leech, N., Gloeckner, G., Barrett, K. (2020). IBM SPSS for Introductory Statistics: Use and interpretation (6th ed.). Routledge.
Collepals.com Plagiarism Free Papers
Are you looking for custom essay writing service or even dissertation writing services? Just request for our write my paper service, and we'll match you with the best essay writer in your subject! With an exceptional team of professional academic experts in a wide range of subjects, we can guarantee you an unrivaled quality of custom-written papers.
Get ZERO PLAGIARISM, HUMAN WRITTEN ESSAYS
Why Hire Collepals.com writers to do your paper?
Quality- We are experienced and have access to ample research materials.
We write plagiarism Free Content
Confidential- We never share or sell your personal information to third parties.
Support-Chat with us today! We are always waiting to answer all your questions.
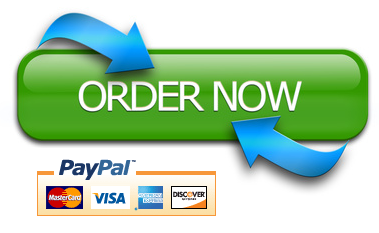