MatLab Question
Industrial Unione convention centre 102 23 10 days hrs min REGISTRATION HOME MANAGEMENT CALL FOR PAPERS DOCTORAL SYMPO PROGRAM VENUE SPONSORS CONTACTS DATA CHALLENGE 2020 NEW: We have added a FAQ to help candidates: link. The PHM Society invites you to join other participants around the world from academia and industry in the PHME20 Data Challenge. An experimental filtration system is the subject of the competition and the challenge is to create high-performance prognostic models to estimate Remaining Useful Life (RUL). Model adaptability and sensitivity are key performance criteria in this competition as prediction capability will be tested and assessed as a function varying experimental parameters and number run-to-failure data sets. Application of Data-Driven as well as Physics-based modelling approaches are encouraged. Winning teams will be invited to publish their work in the conference proceedings of the 5th European Conference of the PHM Society and a representative will be expected to make an oral presentation at the event. Collaboration is encouraged and teams may comprise students and professionals from single or multiple organizations. Competition Open February February 14, 2020 Validation Data Posted Submission Portal Open April 24, 2020 Competition Closed May 15, 2020, 11:59:59 GMT Winners Announced May 29, 2020 Final Papers Due June 7, 2020 PHM Conference Date July 1-3 2020 Objective Participants are challenged to develop models to provide estimates of Remaining Useful Life (RUL) of a ltration system. ‘Run-to-Failure’ data is provided with key condition monitoring parameters: ow rate, upstream pressure (before lter) and downstream pressure (after lter). Data is generated under controlled conditions, with key experimental variables of contamination particle size and concentration, i.e. solid-to-liquid ratio. Model adaptability and sensitivity are key performance criteria in this competition as prediction capability will be assessed as a function of varying contamination particle size, concentration, and number of run-to-failure experiments. Training datasets will be made available immediately after the competition is launched and validation datasets will be provided prior to closing of the competition. Application of DataDriven as well as Physics-based modelling approaches are encouraged. The winning teams will be asked to prepare a full manuscript which will be featured in the PHM conference proceedings and a representative will be expected to make an oral presentation at the event. The prize will be awarded at the conference banquet. For any questions about the competition, please contact us. Teams Collaboration is encouraged and teams may comprise students and professionals from single or multiple organisations. There is no requirement on team size. Register your team’s entry by filling the form. IMPORTANT: You can register to the Data Challenge (free of charge) without register to the conference and decide later to attend or not the PHM Europe 2020. The registration to the conference is not mandatory. The winning teams will be selected and awarded contingent upon: Having at least one member of the team register and attend the PHM 2020 [RKM3] Conference. Submitting a peer-reviewed conference paper. Presenting the analysis results and technique employed at the conference. The organizers of the competition reserve the right to modify these rules and disqualify any team for any e orts it deems inconsistent with fair and open practices. System Description An experimental rig to demonstrate lter clogging failure has been constructed and consists of the following major components: Pump, liquid tanks, tank stirrer, pulsation dampener, lter, pressure and ow rate sensors, data acquisition system connected to a computer. The experiment rig is a circuit composed of a pump owing a liquid from a tank to another through a lter. The circuit is instrumented with sensors able to monitor the flow rate, and the liquid pressure before and after the lter. The uid injected in the system is a suspension composed by Polyetheretherketone (PEEK) particles and water with di erent concentrations. To eliminate possible pulsations in the ow the circuit includes a dampener. Figure 1 depicts the employed experimental rig. Figure 1: System of the experimental rig The main components applied to construct this experimental rig are the following: Pump: Since the system will involve contaminants in the uid, a peristaltic pump has been used as its mechanism is more tolerant to particles in the liquid. A Master ex® SN-77921-70 (Drive: 07523-80, Two Heads: 77200-62, Tubing: L/S© 24) model peristaltic pump was installed in the system to maintain the ow of the prepared suspension. The pump is a positive displacement source, providing a ow rate ranging from 0.28 to 1700 ml/min (i.e. from 0.1 to 600 RPM). Dampener: The aim of using rigid tubing is to prevent the system from unwanted tube expansion due to pressure build up, which a ects the actual pressure build up generated from lter clogging. A Master ex® pulse dampener is installed on the downstream side of pump to eliminate any pulsation in ow.A majority of the system is furnished with a rigid polypropylene tubing, whereas the pump side is covered with a exible Tygon® LFL pump tubing. Particles: The suspension is composed of Polyetheretherketone (PEEK) particles and water. PEEK particles have a density (1.3g/cm3) close to that of room temperature water and have signi cantly low water absorption level (0.1% / 24 hours, ASTM D570). Having a low water absorption level will prevent particles expanding when they mix with water. Subsequently, closer density with water allows particles to suspend longer in water. Flow Rate Sensor: A GMAG100 series electromagnetic ow meter (measurement range: 3 – 25,000 millilitres per minute) is installed in the system to keep track of the ow rate in the system. Pressure Sensors: Upstream and downstream Ashcroft® G2 pressure transducers (measurement range: 0 – 100 PSI) are installed in the system to capture the pressure drop (i.e. ‘ΔP’) across the lter, which is considered as the main indicator of clogging. Filter: the lter has a pore mesh size of 125µm as shown in Figure 2. Figure 2. Filter under study With this experimental rig, several experiments have been run with suspensions of di erent particle size and concentration. As concentration, for each particle size we perform 4 experiments with the concentration reported in Table 1. Table 1: Suspension pro le details Pro le Number 1 2 3 4 7968 7497 7079 6704 32 32 32 32 0.0045 0.00475 (0.45%) (0.48%) Water (g) in the suspension tank Particle (g) 0.004 Solid ratio (0.40%) 0.00425 (0.43%) To create the suspensions we add particles of three possible sizes: small, medium, large. Details about the particle size are reported in Table 2. Table. 2: Particle size data Particle Size (μm) Small 45-53 Medium 53-63 Large 63-75 Datasets and Challenge The experimental dataset is composed of the flow rate (Flow_Rate) of the liquid, and the pressure before (Upstream_Pressure) and after (Downstream_Pressure) the lter, acquired at 10 Hz. To identify when the system fails, we compute the Pressure Drop (Upstream Pressure – Downstream Pressure), and we identify the lter as clogged whenever the pressure drop is higher than 20 psi. The objectives of this data challenge are: (i) to predict when the filter is fully clogged creating models able to determine accurately the Remain Useful life of the filter, (ii) to assess the capability to create a model with performance that do not degrade when a smaller portion of the available dataset is used to create the model itself. For these two sets of data are provided in different moments of the challenge. Model training: 24 experiments describing the run to failure of the lter are used as training datasets. The dataset includes experiments with small and large particle sizes and di erent particle concentrations. For each particle size and concentration 4 experiments are reported. Table 3 describes the training dataset. Model validation: 8 experiments describing the run to failure of the lter are used as validation datasets. The datasets include concentrations that were not available during the training phase. For each particle size and concentration 4 experiments are reported. Table 3: Training set – 24 samples Pro le Number Particle Size Solid Ratio (%) Sample Size 1 45-53 0.4 4 2 small 0.425 4 0.45 4 3 1 63-75 2 large 3 0.4 4 0.425 4 0.45 4 Performance evaluation Participants are expected to generate 4 models to predict accurately the maximum useful life of a lter, and estimate the remaining useful life of the lter in di erent moments. Each team has to build the following models: 1. Using all the available experiments (i.e., Model training + Model validation), to create the model 2. Using 75% of the available experiments (i.e., Model training + Model validation), to create the model 3. Using 50% of the available experiments (i.e., Model training + Model validation), to create the model 4. Using 25% of the available experiments (i.e., Model training + Model validation), to create the model The performance of each model will be evaluated based on an error function, evaluating the accuracy of the model with: the training set and validation set together a test set composed by experiment not available for training and validation. Testing on an independent dataset is performed to assess the generality of the deployed models. Fig. 3 reports an example of the error function trend based on the amount of data used to create the model (x-axis), and the datasets used to evaluate the performance. Figure 3: Example of performance trend A penalty score is used to combine the accuracy of each model and the performance degradation introduced by reducing the amount of data used to build the model. Details of the error function and the penalty score are provided on the « Penalty Score » tab. In addition, a spreadsheet with the calculations has been provided in the « Downloads » tab (for logged-in contestants). Submission les For the submission participants are required to submit a Jupyter Notebook describing the performed process, the 4 created models (e.g., pickle le), usable for the error function evaluation, and a short paper (maximum 4 pages) describing the applied methodology. Penalty score Mi = Model generated with i % experiments TV = Training + Validation datasets TE = Test dataset For the Error Function we use the Mean Absolute Error (MAE) Penalty Score: Penalty(TV) = ∑MAE(Mi(TV)) i ∈ {25,50,75,100} Penalty(TE) = ∑MAE(Mi(TE)) i ∈ {25,50,75,100} Penalty Score = 1.5*Penalty(TE) + Penalty(TV) Downloads Remind that to register to the Data Challenge is free of charge. After completing your registration form, the downloadable les will be available on your profile page. SECRETARY PHME20 KEY DATES For any request, please contact: Abstracts submission EXTENDED deadline: +33 (0)2 47 27 33 30 [email protected] 22 Feb 2020 Paper and poster submissions due: 15 Apr 2020 Paper review feedback: 15 May 2020 Final paper and poster due: 31 May 2020 Propulsed by VERT COM agency
Collepals.com Plagiarism Free Papers
Are you looking for custom essay writing service or even dissertation writing services? Just request for our write my paper service, and we'll match you with the best essay writer in your subject! With an exceptional team of professional academic experts in a wide range of subjects, we can guarantee you an unrivaled quality of custom-written papers.
Get ZERO PLAGIARISM, HUMAN WRITTEN ESSAYS
Why Hire Collepals.com writers to do your paper?
Quality- We are experienced and have access to ample research materials.
We write plagiarism Free Content
Confidential- We never share or sell your personal information to third parties.
Support-Chat with us today! We are always waiting to answer all your questions.
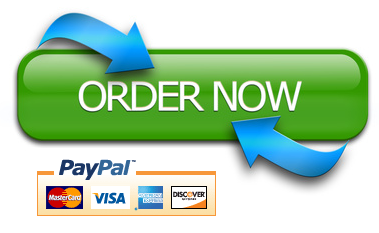