reply 2
1 reply for dr Mary Moore INSTRUCTOR MANAGER RE: Probability and Nonprobability Sampling COLLAPSE Hi Ghady, Great discussion! Excellent explanation of probability, non-probability, conditional, and unconditional sampling and the appropriateness of each. I would add that Bayes’s theorem and sensitivity/specificity are important considerations when we want to establish the presence of a disease. What would you recommend to reduce sampling bias so your findings could be applied to the population of interest? Thank you, Dr. Mary 2 my dis was Characteristics of Probability and Nonprobability Sampling These two ideas may appear to be similar to the layperson, but they vary since each individual in the demographic has a fair likelihood of being selected in probability sampling, but this is not the situation in non-probability sampling. The characteristics of probability sampling and nonprobability sampling are as follows. The first characteristic is that randomization is the foundation for probability sampling, which is why it is sometimes referred to as random sampling (Williams & Brown, 2019). On the other hand, nonprobability sampling does not apply the randomization strategy when selecting a sample. It is therefore viewed as non-random sampling. Secondly, in probability sampling, the individual picks the subject randomly to be a sample component, while in non-probability sampling, the individual picks the representative arbitrarily. Thirdly, there is a set and predetermined probability of selection in probability sampling. Unlike non-probability sampling, the probability is zero, implying it is not expressed. The fourth characteristic is that probability sampling does not include any influence, but non-probability sampling outcomes contain some partiality. In addition, the fifth characteristic is that probability sampling more accurately reflects the entire demographic than nonprobability sampling, as the participants are picked haphazardly by the 3 scientist. The outcomes of probability sampling may therefore be generalized to the overall demographic, but the results of non-probability sampling cannot. Last but not least, nonprobability sampling creates the hypothesis, whereas probability sampling tests it. Why Researchers Would Use Conditional Probability Instead Of Unconditional Probability When supplied knowledge or proof is more particular than what the original batch of findings can convey, conditional probabilities are expected to pay for themselves. Since it is practically omnipresent in both the technique (particularly the application of statistics and game theory) and the straightforward suggestions of the sciences, conditional probability is used by researchers. The effect size, potency, adequate data, regression methods, and Fisher information are among the fundamental statistical notions specified based on conditional probabilities. Game theorists employ conditional probabilities to estimate the anticipated payoffs in linked equilibrium and determine the Bayesian equilibrium in games with imperfect data (Abapour et al., 2020). It is also utilized in some forms of equilibrium selection in recurrent games that use Bayesian dynamic updating (Husák et al., 2018). Since an event’s likelihood to occur is independent of other occurrences, researchers avoid using unconditional probability. Marginal probability is another name for unconditional probability, a hypothesis that ignores other potential findings or current occurrences. Instead, whether or not alternative possibilities are available, an event can still occur, and a result can still be reached. Additionally, the results of earlier events do not influence the result of the current event; instead, they are independent of each other. 4 References Abapour, S., Nazari-Heris, M., Mohammadi-Ivatloo, B., & Tarafdar Hagh, M. (2020). Game theory approaches for the solution of power system problems: A comprehensive review. Archives of Computational Methods in Engineering, 27(1), 81–103. Husák, M., Komárková, J., Bou-Harb, E., & Čeleda, P. (2018). Survey of attack projection, prediction, and forecasting in cyber security. IEEE Communications Surveys & Tutorials, 21(1), 640-660. Kraschnewski, J. L., Keyserling, T. C., Bangdiwala, S. I., Gizlice, Z., Garcia, B. A., Johnston, L. F., Gustafson, A., Petrovic, L., Glasgow, R. E., & Samuel-Hodge, C. D. (2010). Optimized probability sampling of study sites to improve generalizability in a multisite intervention trial. Preventing chronic disease, 7(1), A10. Williams, B. K., & Brown, E. D. (2019). Sampling and analysis frameworks for inference in ecology. Methods in Ecology and Evolution, 10(11), 1832-1842. reply 1 5 RAWAN BAJUNAID Module 05: Discussion COLLAPSE Introduction Probability sampling requires randomly selecting individuals from a population, ensuring fairness and equal opportunity for inclusion. In contrast, non-probability sampling lacks randomization, resulting in unequal chances for selecting each individual from the population. Researchers frequently favor conditional probability over unconditional probability because of its capacity to refine precision and relevance across diverse research domains (Sullivan, 2017). Characteristics of Probability Sampling Randomization governs the selection of a sample from the entire population, constituting the essence of probability sampling. This method involves randomly selecting individuals from the population to conduct tests or other research activities. Probability sampling boasts numerous features and attributes, primarily its considerable expense, time requirements, and complexity. Another characteristic of probability sampling is that it is used when there is required an accurate sample from the population (Castro-Martín et al., 2021). 6 In probability sampling, every individual possesses an equal chance of being chosen for the study, as the randomized principle guides the selection process, and individuals are selected randomly from the population. This method proves beneficial in environments containing multiple populations. Researchers opt for probability sampling to mitigate sampling bias. This sampling method is particularly favored in quantitative research. This sampling method is also helpful in identifying the appropriate population for research sampling, posing a challenge in probability sampling (Castro-Martín et al., 2021). Characteristics of Non-Probability Sampling Non-probability sampling involves selecting a sample from the population based on criteria that are not random, leading to unequal chances for individuals to be chosen for the research. With not every individual having an equal opportunity for selection, the risk of selection bias in the study increases. Non-probability sampling exhibits several characteristics, some of which are outlined below. It is easy to find individuals for the study from the population (Giovanni Di Franco, 2024). Non-probability sampling is characterized by its simplicity and cost-effectiveness. Another notable trait is its efficiency in terms of time. Thirdly, researchers employing non-probability sampling typically do not prioritize addressing selection bias concerns within the sample, given the absence of equal opportunity for every individual in the population to be selected for the research study. It is practical to use this sampling method when all the population has similar traits (Giovanni Di Franco, 2024). 7 Effectiveness of Conditional Probability Conditional probability refers to the likelihood of one event occurring when another has already happened. For example, when the occurrence of one event is dependent on the occurrence of another event, it falls under conditional probability. Conversely, unconditional probability calculates the likelihood of a single outcome among multiple potential outcomes, where one event does not influence the occurrence of others. Researchers favor conditional probability over unconditional probability due to its capacity to improve research findings’ precision, interpretability, and practicality. This preference makes conditional probability a valuable tool across various disciplines, including statistics, epidemiology, psychology, and economics (Castro-Martín et al., 2021). Conclusion It is concluded that grasping the inequalities between probability and non-probability sampling techniques is essential for researchers aiming to minimize biases and uphold the credibility of their conclusions. The inclination towards conditional probability highlights its significance in augmenting the precision and relevance of research outcomes (Sullivan, 2017). References 8 Castro-Martín, L., Rueda, M. del M., & Ferri-García, R. (2021). Combining Statistical Matching and Propensity Score Adjustment for inference from non-probability surveys. Journal of Computational and Applied Mathematics, 1(2), 113414. https://doi.org/10.1016/j.cam.2021.113414 Giovanni Di Franco. (2024). The return of non-probability sample: the electoral polls at the time of internet and social media. Quality & Quantity, 1(2). https://doi.org/10.1007/s11135-024-01835-8 Sullivan, L. M. (2017). Essentials of Biostatistics in Public Health (Essential Public Health) (3rd ed.). Jones & Bartlett Learning.
Collepals.com Plagiarism Free Papers
Are you looking for custom essay writing service or even dissertation writing services? Just request for our write my paper service, and we'll match you with the best essay writer in your subject! With an exceptional team of professional academic experts in a wide range of subjects, we can guarantee you an unrivaled quality of custom-written papers.
Get ZERO PLAGIARISM, HUMAN WRITTEN ESSAYS
Why Hire Collepals.com writers to do your paper?
Quality- We are experienced and have access to ample research materials.
We write plagiarism Free Content
Confidential- We never share or sell your personal information to third parties.
Support-Chat with us today! We are always waiting to answer all your questions.
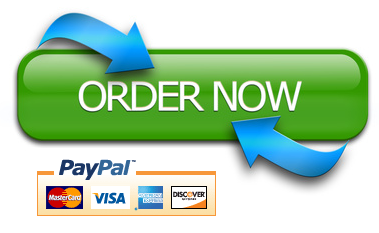