Describe the application of AI and Machine Learning in the two contexts (wildlife and park ranger) what do they have in common, how were they used, what worked and what didn’t? (2) Nam
(1) Describe the application of AI and Machine Learning in the two contexts (wildlife and park ranger) – what do they have in common, how were they used, what worked and what didn’t? (2) Name at least one other area where these same tools/techniques could be used successfully.
https://hbr.org/podcast/2023/03/can-ai-and-machine-learning-help-park-rangers-prevent-poaching
Only 100 Words. I have attached the article below.
9-323-036 R E V : D E C E M B E R 6 , 2 0 2 2
Senior Lecturer Brian Trelstad and Researcher Bonnie Yining Cao (Asia-Pacific Research Center) prepared this case. It was reviewed and approved before publication by a company designate. Funding for the development of this case was provided by Harvard Business School and not by the company. HBS cases are developed solely as the basis for class discussion. Cases are not intended to serve as endorsements, sources of primary data, or illustrations of effective or ineffective management. Copyright © 2022 President and Fellows of Harvard College. To order copies or request permission to reproduce materials, call 1-800-545-7685, write Harvard Business School Publishing, Boston, MA 02163, or go to www.hbsp.harvard.edu. This publication may not be digitized, photocopied, or otherwise reproduced, posted, or transmitted, without the permission of Harvard Business School.
B R I A N T R E L S T A D
B O N N I E Y I N I N G C A O
SMART: AI and Machine Learning for Wildlife Conservation
On a sunny summer afternoon in 2022, Jonathan Palmer, Executive Director of Conservation Technology for the Wildlife Conservation Society, paused. In advance of a meeting with the nine conservation organizations who had collaborated to develop the Spatial Monitoring and Reporting Tool, known as SMART, Palmer wondered how far to promote the integration of a new predictive analytic tool being developed at Harvard University, called the Protection Assistant for Wildlife Security (PAWS). While the SMART coalition had developed software to enable conservationists to record, store and query historical data about poaching in over 1,000 nature reserves around the world, PAWS promised to use artificial intelligence (AI) and machine learning (ML) to predict where poachers were likely to strike in the future.
In the global struggle to protect endangered species from poachers, the deterrence of poachers had long been viewed as more effective, and safer, than catching poachers in the act. Palmer wondered whether the data that PAWS gathered from the parks and wildlife reserves would be reliable enough for AI and ML to work effectively, and whether the park rangers, with their own deep knowledge of the protected areas in which they would value an external high-tech tool that claimed to be able to predict where the locations of poachers.
SMART had already demonstrated significant improvements in patrol coverage, with observed reductions in poaching and growth in wildlife populations, and the question was whether PAWS was a valuable analytical tool to be added to the SMART platform. Palmer thought back to the hundreds of one-off projects he had seen in his career in the non-profit sector that tried out the latest technology, with only a few of them working and everyone moving on to try the next new thing the following year. This consistent preference for the shiny new technology often meant that no one was investing in core products that could deliver value at scale.
Just as SMART was starting to gain widespread adoption, Palmer and his colleagues had to decide whether adding PAWS to SMART would add value and scale? As he reviewed the latest PAWS data from Srepok Wildlife Sanctuary in Cambodia, he wondered whether AI and ML were just more hype, or whether it could help his colleagues around the world achieve their mission: to collect and evaluate data to improve the effectiveness of wildlife conservation efforts.
This document is authorized for use only by melissa markley ([email protected]). Copying or posting is an infringement of copyright. Please contact customerse[email protected] or 800-988-0886 for additional copies.
323-036 SMART: AI and Machine Learning for Wildlife Conservation
2
Global Poaching
Poaching, the illegal hunting or killing of wildlife, was a pressing issue globally. The illegal wildlife trade—the fourth biggest illegal trade in the world—was estimated to be worth over $18.25 billion
annually.1 The number of reported seizures of wildlife worldwide surged more than six times to 20,762 from 3,317 in 1999.2 (See Exhibit 1 for global poaching trends). Some animals, such as birds, were captured and sold as exotic pets, while others were slaughtered for commercial value, causing certain species to be at higher risks of population decline or even extinction.3 For example, about 20,000 African elephants4 were estimated to be killed by poachers each year, fueled by the global ivory trade. Poaching also had devastating consequences for rhinoceros. In Africa alone, rhino poaching was estimated to increase by about 356% from 2015 to 2030.5 Poaching also posed threats to smaller creatures, such as lizards and monkeys,6 which were made into jewelry or traditional medicine, or sold
as pets or for their wild meat.7
Types of Poachers
Commercial poachers were those who made a career out of illegal hunting and trading of wildlife animals as they sold animal meat for human consumption or animal parts for souvenirs.8 Consumers, including locals or tourists, were often unaware of the origin of the animals. Commercial poachers sometimes also conducted the low-level groundwork for large-scale poaching syndicates. In some parts of Africa and Asia, regional syndicates operated across borders and funded illegal hunting of high- value wildlife trafficking.9 During 2016-2018, the annual illicit income from ivory and rhino horn trafficking was estimated to be $400 million and $230 million respectively.10 In some unstable parts of the world, poaching activities were linked with armed militia and extremist groups to fund their battles.11
Most poaching today occurred in lower-income countries where it tended more towards subsistence than the commercial trade, as an option to support a family’s livelihood or access protein for consumption often came from hunting protected wildlife.12 In countries such as Cambodia, rural residents with limited access to livestock killed wildlife for protein or to trade in order to purchase other necessities.13 The annual household income of Cambodia per capita was $1,540, about 4.6% of that of the U.S. as of 2020.14 About 17.8% of Cambodians lived below the national poverty line in 2019.15
CITES
Convention on International Trade in Endangered Species of Wild Fauna and Flora (CITES), which was adopted at a meeting of the International Union for the Conservation of Nature (the IUCN) in 1963 and entered into force in 1975, was an international agreement between governments with a goal to “ensure that international trade in specimens of wild animals and plants did not threaten the survival of the species.”16 It was difficult to calculate the value of animals that were illegally traded because sellers would not report such trades. However, legal trades were tracked by the CITES trade database.17
(CITES introduced a system to report illegal trades in 2017.18)
As of 2022, CITES had 184 member countries and regions.19 Over 38,700 species—including about 5,950 species of animals—were covered under CITES against over-exploitation through international
trade.20 The convention categorized species into three levels of appendixes to ensure sustainable trades. Appendix I listed species threatened with extinction, so international trade was prohibited in all but exceptional circumstances. Appendix II included species not necessarily threatened with extinction, but in which trade must be controlled.21 Appendix III listed species of which individual countries were
This document is authorized for use only by melissa markley ([email protected]). Copying or posting is an infringement of copyright. Please contact customerse[email protected] or 800-988-0886 for additional copies.
SMART: AI and Machine Learning for Wildlife Conservation 323-036
3
struggling to regulate domestic trade and required the assistance of other CITES Parties to help control trade.22 (See Exhibit 2 for a list of species on the respective CITES Appendices).
The CITES Secretariat, administered by the United Nations Environmental Program, was based in Geneva, Switzerland,23 while the convention was financed by a core fund (which was replenished from the contribution of members), as well as an external fund.24 The core fund and external fund had a surplus of $1.5 million25 and $2.2 million,26 as of the end of 2020, respectively. The enforcement of CITES faced many challenges. For many species, experts lacked basic data on population estimates, especially in developing countries, making it hard to know the sustainable trade level for such species.27
Some analysts argued that since the convention was signed and enforced based on the
understanding of trade in the 1970s, it was outdated.28 The CITES Secretariat had only very recently started to use trade sanctions to address serious noncompliance issues, but still did not deploy such measures widely, perhaps because of geopolitical considerations and economic ties between signatory countries.29 Other contemporary critics pointed out that CITES, despite its critical role, over-relied on regulation but “failed to contend with the complex social, cultural, and economic nature of the wildlife trade, especially the role of local communities in the developing world.”30 As CITES focused on trade, poaching remained an issue to be solved.
Poaching and Anti-Poaching in Cambodia
Cambodia, one of the poorest countries in the world, was also among the fastest-growing economies with an average annual growth rate of 7.7% in the period 1998-2019, driven mostly by garment exports and tourism.31 Historically, agriculture was one of the major growth engines of the economy;32 it accounted for 22.8% of the GDP in 2021.33 Most Cambodians were descendants of the Angkor Empire, which existed from the 9th to 15th centuries34 and occupied much of the present day Thailand, Laos and Vietnam at its height.35 It was colonized by France since 1863 till its independence in 1953.36 The
Japanese occupied the country during World War II.37
In one of the world’s worst genocides in the last century, Pol Pot, the leader of the communist party Khmer Rouge, killed as many as 25% of the population in less than four years between 1975 and 1979.38 In 1978, the Vietnamese invaded Cambodia to drive the Khmer Rouge out, into the countryside, and they ended up occupying Cambodia for 10 years. Twenty years of civil war ensued,39 as the Khmer Rouge activists persisted until 1997.40 In 1985, Hun Sen, a senior Cambodia Peoples’ Party leader, became the Prime Minister and remained in leadership ever since, making him one of the world’s longest serving PMs. 41 The COVID-19 pandemic dampened the country’s economy to its worst
performance since 1994, declining by 3.1% in 2020.42
Poaching and illegal wildlife trafficking were pressing issues in Cambodia, which was both a wildlife source and transit country for trafficking. Between 2001 and mid-2018, an estimated 125,445 animals were confiscated in 10,829 incidents throughout Cambodia.43 Corruption and the limited governmental capacity and funding further exacerbated the issue.44 (Cambodia ranked 157th out of 180 countries globally by Transparency International as of 2021.45)
In 1997, Cambodia joined CITES.46 In 1998, The World Wild Fund for Nature (WWF) and the Cambodia government established a taskforce as a part of the organization’s Greater Mekong Program to coordinate conservation efforts across the region.47 In 2001, another NGO called the Wildlife Alliance and the Cambodian government established the Wildlife Rapid Rescue Team (WRRT), “the only wildlife trade enforcement unit with a national mandate and judicial police authority to arrest
This document is authorized for use only by melissa markley ([email protected]). Copying or posting is an infringement of copyright. Please contact customerse[email protected] or 800-988-0886 for additional copies.
323-036 SMART: AI and Machine Learning for Wildlife Conservation
4
traffickers and seize smuggled wildlife.”48 As of 2022, WRRT had rescued over 69,000 live animals and apprehended over 7,700 traders.49 In 2021, rangers confiscated 758 weapons, another threat to the wildlife, similar to the number in 2020.50 In Cambodia, poaching endangered wildlife could result in a prison sentence of up to 5 years and a fine of 15-100 million riels ($3,620-$24,137)a.51
Srepok Wildlife Sanctuary
Srepok Wildlife Sanctuary, established in 2016, 52 was a protected area of 372,971 hectares (3729.71km2) located in the Eastern Plains Landscape (EPL) of Mondulkiri province, in Cambodia.53
(See Exhibit 3 for a map of Srepok.) Srepok was about the same size of Rhode Islandb in the U.S. It was currently under the management of Cambodia’s Ministry of Environment, with technical support from
WWF.54
Srepok, home to many wildlife species of the Lower Mekong Dry Forest region within the Eastern Plains, included many critically endangered species like the Siamese crocodile and red-headed vulture.55 However, from 2010 to 2020 in the Srepok core, three main species of ungulatec—banteng, red muntjac and wild pig—declined significantly due to the historically high hunting levels and increasing poaching activities. 56 Srepok’s location on the international border with Vietnam also enabled a high influx of poachers, with easier access to trafficking.57 “It used to be a site for illegal logging. It is right on the border with Vietnam, you would have foreigners just come in. That then shifted to larger value species like tigers,” said Alex Wyatt, Wildlife Crime Program Coordinator at WWF. Due to extensive poaching, the last tiger that was sighted in Srepok was in 2007,58 and now believed to be extinct in all of Cambodia. “The majority of the trade now is to feed bushmeat markets and some subsistence, not just locally but also regionally. It’s very illegal but very popular,” Wyatt added.
The current patrolling efforts were inadequate in Srepok and across the country. In 2021 Cambodia had around 1,200 rangers to guard endangered species on more than 7.3 million hectares (73,000 km2)
of protected forests nationwide, while in the critical 6,000-square-kilometer bioregion of Srepok and another sanctuary, there were just 51 rangers, 46 wildlife guards and 588 community patrol teams.59 The ranger density of less than 1/100 km2d was much lower than the optimal level of 2-10/100 km2 and that of tiger reserves in Nepal (12-107/100 km2) and India (12-127/100 km2).60 A ranger’s salary was about $150 per month in Cambodia, according to Palmer.
Cambodia’s National Committee for Prevention and Crackdown on National Resources had taken several measures to combat poaching in Srepok. In 2017, Cambodia started the recruitment of community rangers, with the support and training from WWF.61 Throughout 2018, the number of rangers in Srepok increased from 33 to 73, and by the end of June 2018, 92.5% of rangers had received appropriate training.62
a 1 USD=4,143 KHR
b Rhode Island was the smallest of the 50 states in the U.S. with a total area of 3,139 square kilometers. (www.city- data.com/states/Rhode-Island-Location-size-and-extent.html, accessed September 2022.)
c Ungulate: hoofed mammals
d The actual ranger density was 0.82/100 km2.
This document is authorized for use only by melissa markley ([email protected]). Copying or posting is an infringement of copyright. Please contact customerse[email protected] or 800-988-0886 for additional copies.
SMART: AI and Machine Learning for Wildlife Conservation 323-036
5
SMART
Our government partners need better tools, and the market isn’t going to provide these: optimize patrolling efforts in a way that empowers not only the organizations but also these rangers. We gathered as nine organizations and said, “Look, let’s leave our logos and egos outside the room. Let’s build a partnership around developing a solution that works for our partners on the frontline of conservation.”
— Jonathan Palmer
To better combat poaching and deploy limited resources, nine leading conservation organizations and zoological societies, including WCS and WWF, worked closely with the leadership of CITES, to develop a law enforcement management (LEM) tool called “Spatial Monitoring And Reporting Tool” (SMART) in 2011.63 (See Exhibit 4 for SMART partners.) With the goal of becoming the global leader in protected area management solutions, SMART offered “an integrated suite of tools and services to help conservation practitioners improve management effectiveness and better protect wildlife.”64 Law enforcement in the field was fundamental for successful conservation, but it was often costly with rarely monitored effectiveness.65 SMART was built mainly around the theory of deterrence, according to Palmer. “In these vast areas with very small patrol teams, the chances of you actually catching people red-handed are fairly small,” Palmer said.
Each of the nine member organizations had equal footing in SMART, which was led by a steering
committee and three governance councils. (See Exhibit 5 for SMART governance structure.) “There’s one member from each organization that makes every decision. It’s a good example of a very productive partnership,” said Wyatt. “We’re all using the same tool. We’re all singing the same song.” The core running costs of SMART came from a $30,000 contribution per member organization per year, according to Palmer.
The SMART partnership developed an open-sourced software bearing the same name which included a set of free tools with desktop, mobile and cloud-based components. It was a holistic approach to help conservatory managers and rangers through the monitoring of patrolling activities.66 The SMART platform analyzed, visualized, and mapped data. Specifically, it also contained several modules designed to support adaptive protected management, allowing for effective patrolling, rapid
ecological surveys, and key species monitoring.67
In the protected areas, rangers patrolling in the field logged data on hand-held devices and GPS units. For example, SMART Mobile was a data collection app that could be installed even on “rugged devices”e for rangers to collect data on incidents in the field.68 Rangers would record their observations, “confiscate animal traps, rescue live animals caught in snares, and arrest any poacher they
encounter.”69 The data was then synced to a centralized SMART database. Managers could find the routes rangers patrolled and the locations of carcasses. SMART turned all the logged information into “visually informative” maps, charts, and reports.70 “Protecting a park is like protecting a bank. One of the problems is that parks don’t have a wall and your cash is your animals and your trees,” said Rohit Singh, Director of Wildlife Enforcement and Zero Poaching at WWF. “What SMART does is tell you where the animals and the potential threats are, and how to deploy your security to protect the animals and your assets from poachers. And it does it in the simplest way of producing maps and tables that is understandable to park managers.”
e Rugged device: a type of hardware designed especially for the exposure in the harshest environment from extreme temperatures to water or dust. (www.weareconker.com/technology/rugged-devices-and-ip-ratings, accessed September 2022.)
This document is authorized for use only by melissa markley ([email protected]). Copying or posting is an infringement of copyright. Please contact customerse[email protected] or 800-988-0886 for additional copies.
323-036 SMART: AI and Machine Learning for Wildlife Conservation
6
SMART had developed more than 10 languages of its desktop version and 150 languages in its app, a user-friendly move, according to Singh. The app also had over 1,000 icons representing wildlife and activities, as some rangers were illiterate. SMART’s adoption expanded from a few sites in a handful of countries to over 1,000 sites in 70 countries,71 becoming the most utilized LEM tool in the world.72 (See Exhibit 6 for SMART’s global footprint.) Palmer said, “Having a tool adopted in 1,000 protected areas globally is unheard of.”
Data officers and conservatory managers were mainly the ones who worked with the SMART software; the SMART training for data officers took five days to go through a manual of more than 300 pages,73 while rangers learned how to collect and enter data into the mobile app, according to Wyatt, who also led the SMART training program in Cambodia. More than 3,290 people worldwide had been trained in SMART as of 2020.74
Efficacy of SMART
The data on the effectiveness of SMART on anti-poaching was still “fairly limited,” according to Palmer. But a study based on the analysis of 5-year monitoring data in the Royal Manas National Park (RMNP), Bhutan, could shed some light on SMART’s efficacy. RMNP, established in 1966, was Bhutan’s oldest national park of 1,057 km2, about a third the size of Srepok and the tiger was also one of its prominent species.75 It was one of the country’s protected areas that adopted SMART in 2013. In 2013-2017, the annual patrol frequency and overall threat occurrence in RMNP were analyzed. The reduction in threat detection observed and increased patrol efforts between 2015 and 2017 suggested that targeted law enforcement monitoring powered by SMART could be effective in deterring illegal activity.76 (See Exhibit 7 for levels of patrolling and threat observations at RMNP.)
While SMART was adopted in over 1,000 sites, it only represented a small fraction of the 27,194 protected areas around the world as of September 2022.77 “Most protected areas literally don’t have the staff to use SMART, or the resources to keep those staff. The information is still gathered in various ways, but it’s not structured in the way that can be used with SMART,” said Wyatt. He predicted that out of the 1,000 sites that adopted SMART, only about 20% of the sites were using it effectively, citing the lack of funding and rangers as the main reasons.
PAWS
SMART used historical data to empower rangers to develop patrol strategies, but Palmer wondered whether AI and ML could generate data about future poaching patterns that could be relied on for more effective patrol routes. The Teamcore group at Harvard University, led by Milind Tambe, Professor of Computer Science at the John A. Paulson School of Engineering and Applied Sciences (SEAS), focused on advancing AI and multiagent systems research for social impact in areas such as wildlife conservation. It developed the PAWS (Protection Assistant for Wildlife Security) AI system with the aim of improving the patrolling strategy for rangers.78
Tambe and the team designed PAWS to be integrated into the SMART software and eventually be available to conservatory managers globally. PAWS automatically pulled the existing data of historic patrols and observed poaching activities from SMART. Occasionally, the PAWS team would request
organizations, including WWF and WCF, to provide Geography Information System (GIS) shapefilef
f Shapefile was a “nontopological format for storing the geometric location and attribute information of geographic features.”(https://desktop.arcgis.com/en/arcmap/10.3/manage-data/shapefiles/what-is-a-shapefile.htm, accessed September, 2022.)
This document is authorized for use only by melissa markley ([email protected]). Copying or posting is an infringement of copyright. Please contact customerse[email protected] or 800-988-0886 for additional copies.
SMART: AI and Machine Learning for Wildlife Conservation 323-036
7
and GeoTIFF files g of each park. The team would then build an “imperfect observation-aware Ensemble model (iWare-E),” an innovative new method of modeling complex data that could take into account imperfect information and uncertainty to produce effective patrol routes in a computationally less expensive, faster, and more accurate way. 79 They integrated it with Gaussian processes h to
quantify the uncertainty in its predictions.80 “SMART only looks back at the data to say what happened in the past. And PAWS uses the same data to make predictions about the future rather than the past. That’s basically the value-added that PAWS brings,” said Tambe.
Dividing the conservatory area into one square kilometer sections, PAWS used algorithms to predict poaching hot spots, categorizing each segment into high, medium, or low risk. Its model considered factors like proximity to roads and towns, tree coverage, topography, and animal numbers.81 In the process of developing PAWS, Harvard PhD student at SEAS Lily Xu accompanied rangers on their patrols and was struck “by how much real time information they take in and process while moving at 60 kilometers per hour, if not faster, on motorbikes. They will see a footprint and guess somebody walked here five hours ago. Those are the kind of things we’ll never be able to mimic or even come close to replacing. At the same time there is still a lot of higher order things that we can help with.”
Srepok adopted PAWS in December 2018. Xu and the team visited Srepok and conducted a four- month field test in February 2019. According to Xu, they learned several things that they fed back into the system: for example, rangers followed clues like trodden grass to guide their patrol rather than stay on a designated path, so it was unrealistic for PAWS to set an exact path. Srepok rangers also often went on multi-day patrols and would camp overnight, so the team added appropriate campsite locations into its recommendations. 82 PAWS helped rangers detect and remove 1,000 snares, 42 chainsaws, 24 motorbikes, and one truck in one single month in Srepok.83 That was compared with a previous average removal of 200–300 snares a month.84 (See Exhibit 8 for PAWS prediction of poaching risks at Srepok.) However, in early January 2019, a ranger was shot at patrol (but survived) as part of poacher retaliation, according to Xu and Singh.
From Srepok and Scaling Beyond
Srepok was unique in that it had more sparse land and forest than the conservatories in Uganda and Malaysia where Tambe had previously worked. Rangers in Srepok often traveled on motorcycles, which meant moving in a different way and at a faster speed than if they were on foot patrol, rendering what they spotted different. The model also had to account for the seasonality of different locations: for example, in a dry season, rangers could cross a dried river, which would produce a different patrol route than in a wet season (monsoon season in Cambodia extended from May to October).85 “The prediction component about future and risks, based on the past data, perhaps is easier to transfer from one park to another. But the patrolling recommendations component of PAWS from park to park is more difficult to transfer, 
Collepals.com Plagiarism Free Papers
Are you looking for custom essay writing service or even dissertation writing services? Just request for our write my paper service, and we'll match you with the best essay writer in your subject! With an exceptional team of professional academic experts in a wide range of subjects, we can guarantee you an unrivaled quality of custom-written papers.
Get ZERO PLAGIARISM, HUMAN WRITTEN ESSAYS
Why Hire Collepals.com writers to do your paper?
Quality- We are experienced and have access to ample research materials.
We write plagiarism Free Content
Confidential- We never share or sell your personal information to third parties.
Support-Chat with us today! We are always waiting to answer all your questions.
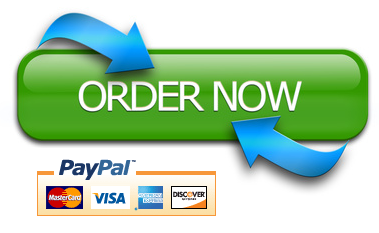