The PowerPoint slides for all the modules that were learned are attached.? Based on your experience and expectations, how would you characterize what you have learned in this cour
The PowerPoint slides for all the modules that were learned are attached.
- Based on your experience and expectations, how would you characterize what you have learned in this course? What were your significant learning moments of the different modules?
- What suggestions do you have to improve what was covered during the modules? Are there any gaps?
- What “ah-ha moments” have you experienced while working on the module materials?
- What have you learned about the concepts of data analysis in healthcare?
- How will you think differently about reported healthcare data since taking this course?
- To what extent have you been successful in achieving the learning outcomes listed in the modules? What is still unclear? What do you still need to work on?
- Compose a two-page paper in MS Word. Cite ALL sources according to APA format.
A Practical Approach to Analyzing Healthcare Data, Fourth Edition Chapter 1, Introduction to Data Analysis
Susan White, PhD, RHIA, CHDA
ahima.org
© 2019 AHIMA
ahima.org
Learning Objectives
Understand types of data analysis
Review types of data
Explore the skills required for a career in healthcare data analytics
© 2019 AHIMA
ahima.org
Data Analysis
Healthcare is a data driven business
Data collected
Diagnostic tests
Services provided
Costs and payment
Diagnosis and procedure codes
What is data analysis?
Task of transforming, summarizing or modeling data to allow the end user to make meaningful conclusions
© 2019 AHIMA
ahima.org
Data
Data
Data
Information
Primary vs Secondary Analysis
Primary data analysis is the use of data for its primary purpose
Example: Billing and claims data’s primary use it to determine services rendered and payment to from a patient or third-party payer
Performing an analysis of typical payment received from a payer for emergency visits is a primary use
Secondary data analysis is the use of data beyond its primary purpose
Example: ICD-10 diagnosis codes are assigned to a patient to record diseases present or discovered during an encounter
Using a profile of the most common ICD-10 diagnosis categories for the purposes of determining the patient load by service line is a secondary use.
Be aware of primary use and evaluate the secondary use is valid and reliable
© 2019 AHIMA
ahima.org
Types of Statistical Analysis
Descriptive statistics
Characterizes the distribution of the data
Estimates the center or ‘typical’ value
Measures the spread or variation in the data
Inferential statistics
Using sample data to make conclusions or decisions regarding a population
Not practical to observe the entire population
Often accompanied with a probability of making an incorrect decision based on the sample
© 2019 AHIMA
ahima.org
Structured vs Unstructured Data
Structured data
AKA Discrete data
Data stored in fields that may be delineated
Values can be listed and validated
Examples
Patient age
CPT code
Laboratory test values
Unstructured data
Free form text captured in narrative form
May be stored in a database field, but the content in not limited to values of a variable
Examples
Progress notes in an EHR
Comments in a patient satisfaction survey
Radiologist’s report of an x-ray result
© 2019 AHIMA
ahima.org
Qualitative Data
Qualitative data
Describes observations about a subject
Typically free text or comments
May be recoded or placed into categories for analysis
Example:
A nurse describes a patient as having pale skin tone.
Survey question: What do you like most about this course?
Data scales typically used for recoding qualitative data:
Nominal – categories without a natural order
Diagnosis codes
Clinical units
Colors
Ordinal – categories with a natural order
Patient satisfaction surveys
Patient severity scores
Evaluation and management code levels
© 2019 AHIMA
ahima.org
Quantitative Data
Quantitative data
Naturally numeric
May be categorical (ordinal or nominal)
Data scales found in quantitative analysis
Interval – numeric values where the distance between two values has meaning, but there is no true zero and the interpretation is not preserved when multiplying/dividing
Temperature
Dates
Ratio – numeric values where zero has meaning and multiplying/dividing values has meaning
Length of stay
Age
Weight
© 2019 AHIMA
ahima.org
Variable Scales/Data Type
© 2019 AHIMA
ahima.org
Overview of Data Type and Statistics
© 2019 AHIMA
ahima.org
Inferential Statistics – CMS
© 2019 AHIMA
ahima.org
Exploratory Data Analysis and Data Mining
Exploratory Data Analysis (EDA)
Used to uncover patterns in data
Typically a secondary use of data
Primarily graphical analysis (plots, trends, etc.)
Data Mining
Also looking for patterns in data
Adds in descriptive statistics and more formal statistical techniques
May be used for benchmarking and determining high/lower performers
© 2019 AHIMA
ahima.org
Predictive Modeling
Historical data is used to build models to determine most likely outcome in future
Data mining is used to identify the potentially best predictors
Maybe a simple function (linear regression) or more involved models (neural networks)
Examples
Used by CMS for pre-payment reviews to fight fraud
Used by credit card companies to prevent fraud
Used by providers to identify missed charges
© 2019 AHIMA
ahima.org
Data Analyst Skills
Must be able to combine:
Content knowledge (coded data, healthcare business process, etc.)
Understanding of the strengths and weaknesses of various data elements
Data acquisition skills through querying databases or effectively writing specifications for queries
Ability to identify the appropriate statistical technique to apply
Familiarity with analytic software to produce the required output
Present the analysis to the end user so that it may be the basis for business decisions
© 2019 AHIMA
ahima.org
Opportunities for HIM Professionals
HIM Professionals are uniquely positioned to:
Understand data structures and coding systems
Understand available data and methods for integration
Can communicate with both finance and IT staff
Act as a business analyst—far more valuable than a pure data analyst
© 2019 AHIMA
ahima.org
Entry Level Health Data Analyst Responsibilities
Working with data
Identify, analyze, and interpret trends or patterns in complex data sets
In collaboration with others, interpret data and develop recommendations on the basis of findings
Perform basic statistical analyses for projects and reports
Reporting Results
Develop graphs, reports, and presentations of project results, trends, data mining
Create and present quality dashboards
Generate routine and ad hoc reports
© 2019 AHIMA
ahima.org
Mid-level Health Data Analyst Responsibilities
Work collaboratively
with data and reporting
the database administrator to help produce effective production management
utilization management reports in support of performance management related to utilization, cost, and risk with the various health plan data
monitor data integrity and quality of reports on a monthly basis
in monitoring financial performance in each health plan
Develop and maintain
claims audit reporting and processes
contract models in support of contract negotiations with health plans
Develop, implement, and enhance evaluation and measurement models for the quality, data and reporting, and data warehouse department programs, projects, and initiatives for maximum effectiveness
Act as a business analyst
Recommend improvements to processes, programs, and initiatives by using analytical skills and a variety of reporting tools
Determine the most appropriate approach for internal and external report design, production, and distribution, specific to the relevant audience
© 2019 AHIMA
ahima.org
Senior-level Health Data Analyst Responsibilities
Understand and address the information needs of governance, leadership, and staff to support continuous improvement of patient care processes and outcomes
Lead and manage efforts to enhance the strategic use of data and analytic tools to improve clinical care processes and outcomes continuously
Work to ensure the dissemination of accurate, reliable, timely, accessible, actionable information (data analysis) to help leaders and staff actively identify and address opportunities to improve patient care and related processes
Work actively with information technology to select and develop tools to enable facility governance and leadership to monitor the progress of quality, patient safety, service, and related metrics continuously throughout the system
© 2019 AHIMA
ahima.org
Senior-level Health Data Analyst Responsibilities
Engage and collaborate with information technology and senior leadership to create and maintain:
a succinct report (e.g., dashboard),
a balanced set of system assessment measures, that conveys status and direction of key system-wide quality and patient safety initiatives for the trustee quality and safety committee and senior management;
present this information regularly to the quality and safety committee of the board to ensure understanding of information contained therein
Actively support the efforts of divisions, departments, programs, and clinical units to identify, obtain, and actively use quantitative information needed to support clinical quality monitoring and improvement activities
Function as an advisor and technical resource regarding the use of data in clinical quality improvement activities
Lead analysis of outcomes and resource utilization for specific patient populations as necessary
Lead efforts to implement state-of-the-art quality improvement analytical tools (i.e., statistical process control)
Play an active role, including leadership, where appropriate, on teams addressing system-wide clinical quality improvement opportunities
© 2019 AHIMA
ahima.org
image3.jpg
image4.png
image5.png
image6.png
image7.png
image1.jpg
image2.jpg
,
A Practical Approach to Analyzing Healthcare Data, Fourth Edition Chapter 2, Data in Healthcare
Susan White, PhD, RHIA, CHDA
ahima.org
© 2019 AHIMA
ahima.org
Learning Objectives
Compare and contrast reliability and validity
Categorize types of healthcare data
Connect the health care data flow to the data types and uses
Illustrate commonly used sources of external data
© 2019 AHIMA
ahima.org
Data Quality
Validity
Accuracy of the data
Ability of the data to measure the attribute it is intended to measure
Reliability
Repeatability or reproducibility of the results
© 2019 AHIMA
ahima.org
Types of Validity
Face validity
Does the metric appear to measure the quantity it was intended to measure?
Often assessed via expert opinion
Weakest form of validity measure, but should be the first step is assessing validity of a new test or metric
Content validity
Are the components of the metric necessary and sufficient to measure the quantity?
In survey design, this content validity ensures that there are not irrelevant questions
Construct validity
Is the measurement tool capturing the construct to be measured?
In survey design, this may be measured by asking similar questions about a topic (or construct) to ensure consistency in the responses
Criterion validity
Does the metric agree with an accepted gold standard for measuring the same quantity?
A new less expensive laboratory test may be compared against another accepted test for measuring the same quantity. If the test results agree, then the new test has criterion validity
© 2019 AHIMA
ahima.org
Types of Reliability
Inter-rater reliability – measures the reproducibility or consistency of the metric between two different raters
Intra-rater reliability – measures the reproducibility or consistency of the metric between two different time points using the same rater
Statistics to measure reliability
Kappa statistic or Cohen’s Kappa
Measures inter or intra rater reliability
0.41 to 0.60 – moderate
0.61 to 0.80 – substantial
0.81 to 1.00 – almost perfect
Cronbach’s Alpha
Measures internal consistency between questions
Acceptable level >= 0.70
© 2019 AHIMA
ahima.org
Types of Healthcare Data
Internal data
Electronic health records
Claims and billing data
Patient satisfaction surveys
External data
Registries (may be both internal/external)
Statewide databases
Medicare claims data
© 2019 AHIMA
ahima.org
Diagnostic Data
Transitioned to ICD-10-CM on 10/1/2015
Even after transition, both coding systems will be utilized for data profiling and analysis
ICD was designed as a disease tracking system, but used in the US as a payment driver under prospective payment systems
© 2019 AHIMA
ahima.org
Diagnostic Data – IPPS
CMS pays for inpatient services provided to Medicare patients via an inpatient prospective payment system (IPPS)
Payment is based on diagnosis related groups (DRG) – ICD-10 diagnosis and procedure codes are combined with other demographic data to ‘group’ patients in the DRGs for determination of payment
DRGs are further grouped into MDCs
ICD-10 and DRG codes are all updated based on the federal fiscal year starting on October 1.
© 2019 AHIMA
ahima.org
Diagnostic Data
© 2019 AHIMA
ahima.org
Procedural Data – ICD-10-PCS
© 2019 AHIMA
ahima.org
Procedural Data – CPT
© 2019 AHIMA
ahima.org
Pharmacy Data
National Drug Codes (NDC)
FDA website
http://www.fda.gov/Drugs/InformationOnDrugs/ucm142438.htm
Therapeutic Classification Groups
OVID Field Guide
http://resourcecenter.ovid.com/site/products/fieldguide/ipab/List_of_AHFS_Pharmacologic-.jsp
RxNorm
National Library of Medicine
© 2019 AHIMA
ahima.org
Administrative Data
Revenue Codes
Place of Service Codes
Claims Processing Codes
Relative Value Unit Data
© 2019 AHIMA
ahima.org
Revenue Codes
Four digit code
Used to categorize charges into ‘departments’ on UB-04 or 837I billing records
NOT necessarily the same department found in provider accounting system
Standard across providers
Allows comparison of departmental charges and costs across providers
http://www.resdac.org/sites/resdac.org/files/Revenue%20Center%20Table.txt
© 2019 AHIMA
ahima.org
Place of Service Codes
Used on professional claims (HCFA-1500 or 837P) to specify the type of location that the service was performed
© 2019 AHIMA
ahima.org
Healthcare Data Flow
© 2019 AHIMA
ahima.org
16
Claims Data
UB-04 Claim form (CMS-1450)
Hospital services
Submitted via 837I transaction set
5010 format
CMS-1500 Claim Form
Physician services
Submitted via 837P transaction set
5010 format
© 2019 AHIMA
ahima.org
Departmental Databases
Laboratory Information System (LIS)
May use Logical Observational Identifiers Names and Codes (LOINC)
Radiology Information System (RIS)
Images available through Picture Archiving and Communication System (PACS)
Patient Accounts Database
Includes financial data
Charges
Payments
Accounts receivable/accounts payable
Payroll
General ledger
May be called a practice management system in a physician office
© 2019 AHIMA
ahima.org
Other Internal Data
Registries
Cancer
Trauma
Birth
Diabetes
Implants
Transplants
Immunizations
© 2019 AHIMA
ahima.org
External Data
Medicare
Inpatient
Outpatient
Part B Utilization (Physician)
State Databases
HCUP
© 2019 AHIMA
ahima.org
Medicare Claims Data
MedPAR File
All Medicare inpatient claims for a given federal fiscal year (10/1 – 9/30)
Data source for many of the labs accompany text
One record for each inpatient stay
Used as the basis for IPPS DRG relative weight changes
Standard Analytic Outpatient File
All Medicare outpatient claims for a given calendar year
Multiple files that must be combined to summarize at the claim level
An extract of this file (HOPPS) is the basis for changes to OPPS APC relative weights
Part B Utilization File
Summary file by calendar year
Includes information by specialty and for top HCPCS codes:
Allowed services (volume)
Allowed charges
Payment amount
© 2019 AHIMA
ahima.org
CMS Payment Rule Impact Files
Released annually
Inpatient prospective payment (IPPS)
Outpatient prospective payment (OPPS)
Includes data elements that may be used for benchmarking
Hospital Demographics
Urban/rural setting
Region
Ownership
Teaching/non-teaching status
Number of beds
Operational Statistics
Volume
Average daily census
Payment adjustment factors
Ratio of cost to charge for cost estimation
Case mix index
Medicare percentage
Value based purchasing performance
Payment level (current and projected)
© 2019 AHIMA
ahima.org
Data.medicare.gov
Central repository for Medicare ‘compare’ databases
© 2019 AHIMA
ahima.org
23
State Databases
Utah
Office of Healthcare Statistics
Hospital utilization
Ambulatory surgery center utilization
Query tools to locate specific data
Massachusetts
Massachusetts Community Health Information Profile (MassCHIP)
Standard reports – ‘instant topics’
Downloadable query software for producing custom reports
© 2019 AHIMA
ahima.org
HCUP
http://hcupnet.ahrq.gov/
Data elements
Statistics on Hospital Stays
Readmission Rates
Emergency Department Use
AHRQ Quality Indicators
Online query system
© 2019 AHIMA
ahima.org
HCUP Sample Query
© 2019 AHIMA
ahima.org
image3.jpg
image4.png
image5.png
image6.png
image7.png
image8.png
image9.png
image10.png
image11.png
image12.png
image1.jpg
image2.jpg
,
A Practical Approach to Analyzing Healthcare Data, Fourth Edition Chapter 3, Tools for Data Organization, Analysis, and Presentation
Susan White, PhD, RHIA, CHDA
ahima.org
© 2019 AHIMA
ahima.org
Learning Objectives
Compare and contrast database structures
Categorize types of statistical software
Illustrate commonly used data visualization methods
© 2019 AHIMA
ahima.org
Data Organization Using Databases
Healthcare data is complex and often multi-dimensional
Provider
Patients
Insurance companies
Services
Providing an organizational structure for the data can facilitate more efficient analysis and reporting
Database – self-describing collection of integrated records.
Self-describing – contains a description of its own structure
Integrated – data elements are related to each other
© 2019 AHIMA
ahima.org
Database Vocabulary
Tables- two dimensional arrays of data
Rows = records
Columns = variables or attributes
RDMS – Relational Database Management System
Software that is used to hold and maintain data tables and their relationships
SQL – Structured Query Language
Programming language used to communicate with a relational database
ERD – Entity Relationship Diagram
Diagram that shows how tables in an RDMS relate
© 2019 AHIMA
ahima.org
Hierarchy of a Relational Database
Tables are rows and columns of values
Envision a tab in a spreadsheet
Fields are the columns in a spreadsheet
In a patient database, fields may be age, gender, admission date, etc.
Data elements or records are the rows in a spreadsheet
In a patient database, row may represent patients or services provided to patients
A unique row identifier in a table is called the
Collepals.com Plagiarism Free Papers
Are you looking for custom essay writing service or even dissertation writing services? Just request for our write my paper service, and we'll match you with the best essay writer in your subject! With an exceptional team of professional academic experts in a wide range of subjects, we can guarantee you an unrivaled quality of custom-written papers.
Get ZERO PLAGIARISM, HUMAN WRITTEN ESSAYS
Why Hire Collepals.com writers to do your paper?
Quality- We are experienced and have access to ample research materials.
We write plagiarism Free Content
Confidential- We never share or sell your personal information to third parties.
Support-Chat with us today! We are always waiting to answer all your questions.
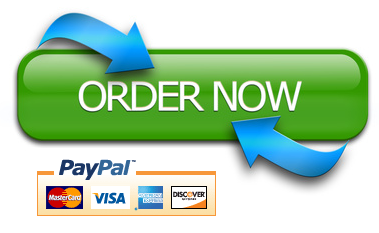