Applying all the code on your selected dataset, complete all codes from Chapter 4 Bivariate Graphs. Make sure you submit to this link two things 1. Your report file showing screensho
Applying all the code on your selected dataset, complete all codes from Chapter 4 Bivariate Graphs. Make sure you submit to this link two things
1. Your report file showing screenshots of all commands from Rstudio GUI
Make sure you show all Rstudio GUIs
2. Submit your R script code
3. Applying all the code on your selected dataset, complete all codes from
Chapter 5 Multivariate Graphs. Make sure you submit to this link two things
4. Your report file showing screenshots of all commands from Rstudio GUI Make sure you show all Rstudio GUIs
5.Submit your R script code
6. Applying all the code on your selected dataset, complete all codes from Chapter 8 Statistical Models. Make sure you submit to this link two things
7. Your report file showing screenshots of all commands from Rstudio GUI Make sure you show all Rstudio GUIs
8.Submit your R script code
9. Applying all the code on your selected dataset, complete all codes from Chapter 9. Make sure you submit to this link two things
10. Your report file showing screenshots of all commands from Rstudio GUI Make sure you show all Rstudio GUIs
11.Submit your R script code
Data Visualization with R Rob Kabacoff 2018-09-03
2
Contents
Welcome 7
Preface 9
How to use this book . . . . . . . . . . . . . . . . . . . . . . . . . . . . . . . . . . . . . . . . . . . . 9
Prequisites . . . . . . . . . . . . . . . . . . . . . . . . . . . . . . . . . . . . . . . . . . . . . . . . . 10
Setup . . . . . . . . . . . . . . . . . . . . . . . . . . . . . . . . . . . . . . . . . . . . . . . . . . . . 10
1 Data Preparation 11
1.1 Importing data . . . . . . . . . . . . . . . . . . . . . . . . . . . . . . . . . . . . . . . . . . . . 11
1.2 Cleaning data . . . . . . . . . . . . . . . . . . . . . . . . . . . . . . . . . . . . . . . . . . . . . 12
2 Introduction to ggplot2 19
2.1 A worked example . . . . . . . . . . . . . . . . . . . . . . . . . . . . . . . . . . . . . . . . . . 19
2.2 Placing the data and mapping options . . . . . . . . . . . . . . . . . . . . . . . . . . . . . . . 30
2.3 Graphs as objects . . . . . . . . . . . . . . . . . . . . . . . . . . . . . . . . . . . . . . . . . . . 32
3 Univariate Graphs 35
3.1 Categorical . . . . . . . . . . . . . . . . . . . . . . . . . . . . . . . . . . . . . . . . . . . . . . 35
3.2 Quantitative . . . . . . . . . . . . . . . . . . . . . . . . . . . . . . . . . . . . . . . . . . . . . . 51
4 Bivariate Graphs 63
4.1 Categorical vs. Categorical . . . . . . . . . . . . . . . . . . . . . . . . . . . . . . . . . . . . . 63
4.2 Quantitative vs. Quantitative . . . . . . . . . . . . . . . . . . . . . . . . . . . . . . . . . . . . 71
4.3 Categorical vs. Quantitative . . . . . . . . . . . . . . . . . . . . . . . . . . . . . . . . . . . . . 79
5 Multivariate Graphs 103
5.1 Grouping . . . . . . . . . . . . . . . . . . . . . . . . . . . . . . . . . . . . . . . . . . . . . . . 103
6 Maps 115
6.1 Dot density maps . . . . . . . . . . . . . . . . . . . . . . . . . . . . . . . . . . . . . . . . . . . 115
6.2 Choropleth maps . . . . . . . . . . . . . . . . . . . . . . . . . . . . . . . . . . . . . . . . . . . 119
3
4 CONTENTS
7 Time-dependent graphs 127
7.1 Time series . . . . . . . . . . . . . . . . . . . . . . . . . . . . . . . . . . . . . . . . . . . . . . 127
7.2 Dummbbell charts . . . . . . . . . . . . . . . . . . . . . . . . . . . . . . . . . . . . . . . . . . 130
7.3 Slope graphs . . . . . . . . . . . . . . . . . . . . . . . . . . . . . . . . . . . . . . . . . . . . . 133
7.4 Area Charts . . . . . . . . . . . . . . . . . . . . . . . . . . . . . . . . . . . . . . . . . . . . . . 135
8 Statistical Models 139
8.1 Correlation plots . . . . . . . . . . . . . . . . . . . . . . . . . . . . . . . . . . . . . . . . . . . 139
8.2 Linear Regression . . . . . . . . . . . . . . . . . . . . . . . . . . . . . . . . . . . . . . . . . . . 141
8.3 Logistic regression . . . . . . . . . . . . . . . . . . . . . . . . . . . . . . . . . . . . . . . . . . 145
8.4 Survival plots . . . . . . . . . . . . . . . . . . . . . . . . . . . . . . . . . . . . . . . . . . . . . 147
8.5 Mosaic plots . . . . . . . . . . . . . . . . . . . . . . . . . . . . . . . . . . . . . . . . . . . . . . 150
9 Other Graphs 153
9.1 3-D Scatterplot . . . . . . . . . . . . . . . . . . . . . . . . . . . . . . . . . . . . . . . . . . . . 153
9.2 Biplots . . . . . . . . . . . . . . . . . . . . . . . . . . . . . . . . . . . . . . . . . . . . . . . . . 159
9.3 Bubble charts . . . . . . . . . . . . . . . . . . . . . . . . . . . . . . . . . . . . . . . . . . . . . 161
9.4 Flow diagrams . . . . . . . . . . . . . . . . . . . . . . . . . . . . . . . . . . . . . . . . . . . . 163
9.5 Heatmaps . . . . . . . . . . . . . . . . . . . . . . . . . . . . . . . . . . . . . . . . . . . . . . . 168
9.6 Radar charts . . . . . . . . . . . . . . . . . . . . . . . . . . . . . . . . . . . . . . . . . . . . . 174
9.7 Scatterplot matrix . . . . . . . . . . . . . . . . . . . . . . . . . . . . . . . . . . . . . . . . . . 176
9.8 Waterfall charts . . . . . . . . . . . . . . . . . . . . . . . . . . . . . . . . . . . . . . . . . . . . 178
9.9 Word clouds . . . . . . . . . . . . . . . . . . . . . . . . . . . . . . . . . . . . . . . . . . . . . . 180
10 Customizing Graphs 183
10.1 Axes . . . . . . . . . . . . . . . . . . . . . . . . . . . . . . . . . . . . . . . . . . . . . . . . . . 183
10.2 Colors . . . . . . . . . . . . . . . . . . . . . . . . . . . . . . . . . . . . . . . . . . . . . . . . . 187
10.3 Points & Lines . . . . . . . . . . . . . . . . . . . . . . . . . . . . . . . . . . . . . . . . . . . . 193
10.4 Legends . . . . . . . . . . . . . . . . . . . . . . . . . . . . . . . . . . . . . . . . . . . . . . . . 195
10.5 Labels . . . . . . . . . . . . . . . . . . . . . . . . . . . . . . . . . . . . . . . . . . . . . . . . . 197
10.6 Annotations . . . . . . . . . . . . . . . . . . . . . . . . . . . . . . . . . . . . . . . . . . . . . . 199
10.7 Themes . . . . . . . . . . . . . . . . . . . . . . . . . . . . . . . . . . . . . . . . . . . . . . . . 206
11 Saving Graphs 219
11.1 Via menus . . . . . . . . . . . . . . . . . . . . . . . . . . . . . . . . . . . . . . . . . . . . . . . 219
11.2 Via code . . . . . . . . . . . . . . . . . . . . . . . . . . . . . . . . . . . . . . . . . . . . . . . . 219
11.3 File formats . . . . . . . . . . . . . . . . . . . . . . . . . . . . . . . . . . . . . . . . . . . . . . 219
11.4 External editing . . . . . . . . . . . . . . . . . . . . . . . . . . . . . . . . . . . . . . . . . . . 221
CONTENTS 5
12 Interactive Graphs 223
12.1 leaflet . . . . . . . . . . . . . . . . . . . . . . . . . . . . . . . . . . . . . . . . . . . . . . . . . 223
12.2 plotly . . . . . . . . . . . . . . . . . . . . . . . . . . . . . . . . . . . . . . . . . . . . . . . . . 223
12.3 rbokeh . . . . . . . . . . . . . . . . . . . . . . . . . . . . . . . . . . . . . . . . . . . . . . . . . 226
12.4 rCharts . . . . . . . . . . . . . . . . . . . . . . . . . . . . . . . . . . . . . . . . . . . . . . . . 226
12.5 highcharter . . . . . . . . . . . . . . . . . . . . . . . . . . . . . . . . . . . . . . . . . . . . . . 226
13 Advice / Best Practices 231
13.1 Labeling . . . . . . . . . . . . . . . . . . . . . . . . . . . . . . . . . . . . . . . . . . . . . . . . 231
13.2 Signal to noise ratio . . . . . . . . . . . . . . . . . . . . . . . . . . . . . . . . . . . . . . . . . 232
13.3 Color choice . . . . . . . . . . . . . . . . . . . . . . . . . . . . . . . . . . . . . . . . . . . . . . 234
13.4 y-Axis scaling . . . . . . . . . . . . . . . . . . . . . . . . . . . . . . . . . . . . . . . . . . . . . 234
13.5 Attribution . . . . . . . . . . . . . . . . . . . . . . . . . . . . . . . . . . . . . . . . . . . . . . 238
13.6 Going further . . . . . . . . . . . . . . . . . . . . . . . . . . . . . . . . . . . . . . . . . . . . . 238
13.7 Final Note . . . . . . . . . . . . . . . . . . . . . . . . . . . . . . . . . . . . . . . . . . . . . . . 239
A Datasets 241
A.1 Academic salaries . . . . . . . . . . . . . . . . . . . . . . . . . . . . . . . . . . . . . . . . . . . 241
A.2 Starwars . . . . . . . . . . . . . . . . . . . . . . . . . . . . . . . . . . . . . . . . . . . . . . . . 241
A.3 Mammal sleep . . . . . . . . . . . . . . . . . . . . . . . . . . . . . . . . . . . . . . . . . . . . . 241
A.4 Marriage records . . . . . . . . . . . . . . . . . . . . . . . . . . . . . . . . . . . . . . . . . . . 242
A.5 Fuel economy data . . . . . . . . . . . . . . . . . . . . . . . . . . . . . . . . . . . . . . . . . . 242
A.6 Gapminder data . . . . . . . . . . . . . . . . . . . . . . . . . . . . . . . . . . . . . . . . . . . 242
A.7 Current Population Survey (1985) . . . . . . . . . . . . . . . . . . . . . . . . . . . . . . . . . 242
A.8 Houston crime data . . . . . . . . . . . . . . . . . . . . . . . . . . . . . . . . . . . . . . . . . . 242
A.9 US economic timeseries . . . . . . . . . . . . . . . . . . . . . . . . . . . . . . . . . . . . . . . 243
A.10 Saratoga housing data . . . . . . . . . . . . . . . . . . . . . . . . . . . . . . . . . . . . . . . . 243
A.11 US population by age and year . . . . . . . . . . . . . . . . . . . . . . . . . . . . . . . . . . . 243
A.12 NCCTG lung cancer data . . . . . . . . . . . . . . . . . . . . . . . . . . . . . . . . . . . . . . 243
A.13 Titanic data . . . . . . . . . . . . . . . . . . . . . . . . . . . . . . . . . . . . . . . . . . . . . . 243
A.14 JFK Cuban Missle speech . . . . . . . . . . . . . . . . . . . . . . . . . . . . . . . . . . . . . . 244
A.15 UK Energy forecast data . . . . . . . . . . . . . . . . . . . . . . . . . . . . . . . . . . . . . . . 244
A.16 US Mexican American Population . . . . . . . . . . . . . . . . . . . . . . . . . . . . . . . . . 244
B About the Author 245
C About the QAC 247
6 CONTENTS
Welcome
R is an amazing platform for data analysis, capable of creating almost any type of graph. This book helps you create the most popular visualizations – from quick and dirty plots to publication-ready graphs. The text relies heavily on the ggplot2 package for graphics, but other approaches are covered as well.
This work is licensed under a Creative Commons Attribution-NonCommercial-NoDerivatives 4.0 Interna- tional License.
My goal is make this book as helpful and user-friendly as possible. Any feedback is both welcome and appreciated.
7
8 CONTENTS
Preface
How to use this book
You don’t need to read this book from start to finish in order to start building effective graphs. Feel free to jump to the section that you need and then explore others that you find interesting.
Graphs are organized by
• the number of variables to be plotted
• the type of variables to be plotted • the purpose of the visualization
Chapter Description Ch 1 provides a quick overview of how to get your data into R and how to prepare it
for analysis. Ch 2 provides an overview of the ggplot2 package. Ch 3 describes graphs for visualizing the distribution of a single categorical (e.g. race)
or quantitative (e.g. income) variable. Ch 4 describes graphs that display the relationship between two variables. Ch 5 describes graphs that display the relationships among 3 or more variables. It is
helpful to read chapters 3 and 4 before this chapter. Ch 6 provides a brief introduction to displaying data geographically. Ch 7 describes graphs that display change over time. Ch 8 describes graphs that can help you interpret the results of statistical models. Ch 9 covers graphs that do not fit neatly elsewhere (every book needs a miscellaneous
chapter). Ch 10 describes how to customize the look and feel of your graphs. If you are going to
share your graphs with others, be sure to skim this chapter. Ch 11 covers how to save your graphs. Different formats are optimized for different
purposes. Ch 12 provides an introduction to interactive graphics. Ch 13 gives advice on creating effective graphs and where to go to learn more. It’s
worth a look. The Appendices describe each of the datasets used in this book, and provides a short blurb about
the author and the Wesleyan Quantitative Analysis Center.
There is no one right graph for displaying data. Check out the examples, and see which type best fits your needs.
9
10 CONTENTS
Prequisites
It’s assumed that you have some experience with the R language and that you have already installed R and RStudio. If not, here are some resources for getting started:
• A (very) short introduction to R • DataCamp – Introduction to R with Jonathon Cornelissen • Quick-R • Getting up to speed with R
Setup
In order to create the graphs in this guide, you’ll need to install some optional R packages. To install all of the necessary packages, run the following code in the RStudio console window.
pkgs <- c("ggplot2", "dplyr", "tidyr", "mosaicData", "carData", "VIM", "scales", "treemapify", "gapminder", "ggmap", "choroplethr", "choroplethrMaps", "CGPfunctions", "ggcorrplot", "visreg", "gcookbook", "forcats", "survival", "survminer", "ggalluvial", "ggridges", "GGally", "superheat", "waterfalls", "factoextra", "networkD3", "ggthemes", "hrbrthemes", "ggpol", "ggbeeswarm")
install.packages(pkgs)
Alternatively, you can install a given package the first time it is needed.
For example, if you execute
library(gapminder)
and get the message
Error in library(gapminder) : there is no package called ‘gapminder’
you know that the package has never been installed. Simply execute
install.packages("gapminder")
once and
library(gapminder)
will work from that point on.
Chapter 1
Data Preparation
Before you can visualize your data, you have to get it into R. This involves importing the data from an external source and massaging it into a useful format.
1.1 Importing data
R can import data from almost any source, including text files, excel spreadsheets, statistical packages, and database management systems. We’ll illustrate these techniques using the Salaries dataset, containing the 9 month academic salaries of college professors at a single institution in 2008-2009.
1.1.1 Text files
The readr package provides functions for importing delimited text files into R data frames.
library(readr)
# import data from a comma delimited file Salaries <- read_csv("salaries.csv")
# import data from a tab delimited file Salaries <- read_tsv("salaries.txt")
These function assume that the first line of data contains the variable names, values are separated by commas or tabs respectively, and that missing data are represented by blanks. For example, the first few lines of the comma delimited file looks like this.
"rank","discipline","yrs.since.phd","yrs.service","sex","salary" "Prof","B",19,18,"Male",139750 "Prof","B",20,16,"Male",173200 "AsstProf","B",4,3,"Male",79750 "Prof","B",45,39,"Male",115000 "Prof","B",40,41,"Male",141500 "AssocProf","B",6,6,"Male",97000
Options allow you to alter these assumptions. See the documentation for more details.
11
12 CHAPTER 1. DATA PREPARATION
1.1.2 Excel spreadsheets
The readxl package can import data from Excel workbooks. Both xls and xlsx formats are supported.
library(readxl)
# import data from an Excel workbook Salaries <- read_excel("salaries.xlsx", sheet=1)
Since workbooks can have more than one worksheet, you can specify the one you want with the sheet option. The default is sheet=1.
1.1.3 Statistical packages
The haven package provides functions for importing data from a variety of statistical packages.
library(haven)
# import data from Stata Salaries <- read_dta("salaries.dta")
# import data from SPSS Salaries <- read_sav("salaries.sav")
# import data from SAS Salaries <- read_sas("salaries.sas7bdat")
1.1.4 Databases
Importing data from a database requires additional steps and is beyond the scope of this book. Depending on the database containing the data, the following packages can help: RODBC, RMySQL, ROracle, RPostgreSQL, RSQLite, and RMongo. In the newest versions of RStudio, you can use the Connections pane to quickly access the data stored in database management systems.
1.2 Cleaning data
The processes of cleaning your data can be the most time-consuming part of any data analysis. The most important steps are considered below. While there are many approaches, those using the dplyr and tidyr packages are some of the quickest and easiest to learn.
Package Function Use dplyr select select variables/columns dplyr filter select observations/rows dplyr mutate transform or recode variables dplyr summarize summarize data dplyr group_by identify subgroups for further processing tidyr gather convert wide format dataset to long format tidyr spread convert long format dataset to wide format
1.2. CLEANING DATA 13
Examples in this section will use the starwars dataset from the dplyr package. The dataset provides descriptions of 87 characters from the Starwars universe on 13 variables. (I actually prefer StarTrek, but we work with what we have.)
1.2.1 Selecting variables
The select function allows you to limit your dataset to specified variables (columns).
library(dplyr)
# keep the variables name, height, and gender newdata <- select(starwars, name, height, gender)
# keep the variables name and all variables # between mass and species inclusive newdata <- select(starwars, name, mass:species)
# keep all variables except birth_year and gender newdata <- select(starwars, -birth_year, -gender)
1.2.2 Selecting observations
The filter function allows you to limit your dataset to observations (rows) meeting a specific criteria. Multiple criteria can be combined with the & (AND) and | (OR) symbols.
library(dplyr)
# select females newdata <- filter(starwars,
gender == "female")
# select females that are from Alderaan newdata <- select(starwars,
gender == "female" & homeworld == "Alderaan")
# select individuals that are from # Alderaan, Coruscant, or Endor newdata <- select(starwars,
homeworld == "Alderaan" | homeworld == "Coruscant" | homeworld == "Endor")
# this can be written more succinctly as newdata <- select(starwars,
homeworld %in% c("Alderaan", "Coruscant", "Endor"))
1.2.3 Creating/Recoding variables
The mutate function allows you to create new variables or transform existing ones.
14 CHAPTER 1. DATA PREPARATION
library(dplyr)
# convert height in centimeters to inches, # and mass in kilograms to pounds newdata <- mutate(starwars,
height = height * 0.394, mass = mass * 2.205)
The ifelse function (part of base R) can be used for recoding data. The format is ifelse(test, return if TRUE, return if FALSE).
library(dplyr)
# if height is greater than 180 # then heightcat = "tall", # otherwise heightcat = "short"
newdata <- mutate(starwars, heightcat = ifelse(height > 180,
"tall", "short")
# convert any eye color that is not # black, blue or brown, to other newdata <- mutate(starwars,
eye_color = ifelse(eye_color %in% c("black", "blue", "brown"), eye_color, "other")
# set heights greater than 200 or # less than 75 to missing newdata <- mutate(starwars,
height = ifelse(height < 75 | height > 200, NA, height)
1.2.4 Summarizing data
The summarize function can be used to reduce multiple values down to a single value (such as a mean). It is often used in conjunction with the by_group function, to calculate statistics by group. In the code below, the na.rm=TRUE option is used to drop missing values before calculating the means.
library(dplyr)
# calculate mean height and mass newdata <- summarize(starwars,
mean_ht = mean(height, na.rm=TRUE), mean_mass = mean(mass, na.rm=TRUE))
newdata
## # A tibble: 1 x 2 ## mean_ht mean_mass
1.2. CLEANING DATA 15
## <dbl> <dbl> ## 1 174. 97.3
# calculate mean height and weight by gender newdata <- group_by(starwars, gender) newdata <- summarize(newdata,
mean_ht = mean(height, na.rm=TRUE), mean_wt = mean(mass, na.rm=TRUE))
newdata
## # A tibble: 5 x 3 ## gender mean_ht mean_wt ## <chr> <dbl> <dbl> ## 1 female 165. 54.0 ## 2 hermaphrodite 175. 1358. ## 3 male 179. 81.0 ## 4 none 200. 140. ## 5 <NA> 120. 46.3
1.2.5 Using pipes
Packages like dplyr and tidyr allow you to write your code in a compact format using the pipe %>% operator. Here is an example.
library(dplyr)
# calculate the mean height for women by species newdata <- filter(starwars,
gender == "female") newdata <- group_by(species) newdata <- summarize(newdata,
mean_ht = mean(height, na.rm = TRUE))
# this can be written as newdata <- starwars %>% filter(gender == "female") %>% group_by(species) %>% summarize(mean_ht = mean(height, na.rm = TRUE))
The %>% operator passes the result on the left to the first parameter of the function on the right.
1.2.6 Reshaping data
Some graphs require the data to be in wide format, while some graphs require the data to be in long format.
You can convert a wide dataset to a long dataset using
library(tidyr) long_data <- gather(wide_data,
key="variable", value="value", sex:income)
16 CHAPTER 1. DATA PREPARATION
Table 1.2: Wide data
id name sex age income 01 Bill Male 22 55000 02 Bob Male 25 75000 03 Mary Female 18 90000
Table 1.3: Long data
id name variable value 01 Bill sex Male 02 Bob sex Male 03 Mary sex Female 01 Bill age 22 02 Bob age 25 03 Mary age 18 01 Bill income 55000 02 Bob income 75000 03 Mary income 90000
Conversely, you can convert a long dataset to a wide dataset using
library(tidyr) wide_data <- spread(long_data, variable, value)
1.2.7 Missing data
Real data are likely to contain missing values. There are three basic approaches to dealing with missing data: feature selection, listwise deletion, and imputation. Let’s see how each applies to the msleep dataset from the ggplot2 package. The msleep dataset describes the sleep habits of mammals and contains missing values on several variables.
1.2.7.1 Feature selection
In feature selection, you delete variables (columns) that contain too many missing values.
data(msleep, package="ggplot2")
# what is the proportion of missing data for each variable? pctmiss <- colSums(is.na(msleep))/nrow(msleep) round(pctmiss, 2)
## name genus vore order conservation ## 0.00 0.00 0.08 0.00 0.35 ## sleep_total sleep_rem sleep_cycle awake brainwt ## 0.00 0.27 0.61 0.00 0.33 ## bodywt ## 0.00
Sixty-one percent of the sleep_cycle values are missing. You may decide to drop it.
1.2. CLEANING DATA 17
1.2.7.2 Listwise deletion
Listwise deletion involves deleting observations (rows) that contain missing values on any of the variables of interest.
# Create a dataset containing genus, vore, and conservation. # Delete any rows containing missing data. newdata <- select(msleep, genus, vore, conservation) newdata <- na.omit(newdata)
1.2.7.3 Imputation
Imputation involves replacing missing values with “reasonable” guesses about what the values would have been if they had not been missing. There are several approaches, as detailed in such packages as VIM, mice, Amelia and missForest. Here we will use the kNN function from the VIM package to replace missing values with imputed values.
# Impute missing values using the 5 nearest neighbors library(VIM) newdata <- kNN(msleep, k=5)
Basically, for each case with a missing value, the k most similar cases not having a missing value are selected. If the missing value is numeric, the mean of those k cases is used as the imputed value. If the missing value is categorical, the most frequent value from the k cases is used. The process iterates over cases and variables until the results converge (become stable). This is a bit of an oversimplification – see Imputation with R Package VIM for the actual details.
Important caveate: Missing values can bias the results of studies (sometimes severely). If you have a significant amount of missing data, it is probably a good idea to consult a statistician or data scientist before deleting cases or imputing missing values.
18 CHAPTER 1. DATA PREPARATION
Chapter 2
Introduction to ggplot2
This section provides an brief overview of how the ggplot2 package works. If you are simply seeking code to make a specific type of graph, feel free to skip this section. However, the material can help you understand how the pieces fit together.
2.1 A worked example
The functions in the ggplot2 package build up a graph in layers. We’ll build a a complex graph by starting with a simple graph and adding additional elements, one at a time.
The example u
Collepals.com Plagiarism Free Papers
Are you looking for custom essay writing service or even dissertation writing services? Just request for our write my paper service, and we'll match you with the best essay writer in your subject! With an exceptional team of professional academic experts in a wide range of subjects, we can guarantee you an unrivaled quality of custom-written papers.
Get ZERO PLAGIARISM, HUMAN WRITTEN ESSAYS
Why Hire Collepals.com writers to do your paper?
Quality- We are experienced and have access to ample research materials.
We write plagiarism Free Content
Confidential- We never share or sell your personal information to third parties.
Support-Chat with us today! We are always waiting to answer all your questions.
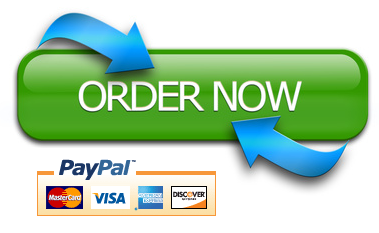