What are types of regression? For each type of regression, give an application. Does your job use any? if so how?. Please cite examples according to APA standards.? [Your post must be subst
Initial Postings: Read and reflect on the assigned readings for the week. Then post what you thought was the most important concept(s), method(s), term(s), and/or any other thing that you felt was worthy of your understanding in each assigned textbook chapter.
Your initial post should be based upon the assigned reading for the week, so the textbook should be a source listed in your reference section and cited within the body of the text. Other sources are not required but feel free to use them if they aid in your discussion.
Also, provide a graduate-level response to each of the following questions:
- What are types of regression? For each type of regression, give an application. Does your job use any? if so how?. Please cite examples according to APA standards.
[Your post must be substantive and demonstrate insight gained from the course material. Postings must be in the student's own words – do not provide quotes!] [Your initial post should be at least 250+ words and in APA format (including Times New Roman with font size 12 and double spaced).
Linear Regression as a Fundamental Descriptive Tool
Chapter 5
© 2019 McGraw-Hill Education. All rights reserved. Authorized only for instructor use in the classroom. No reproduction or distribution without the prior written consent of McGraw-Hill Education
Learning Objectives
Construct a regression line for a dichotomous treatment
Construct a regression line for a multi-level treatment
Explain both intuitively and formerly the formulas generating a regression line for a single treatment
Distinguish the use of sample moment equations from estimation via least squares
Distinguish regression equations for single and multiple treatments
Describe a dataset with multiple treatments using multiple regression
Explain the difference between linear regression and a regression line
‹#›
© 2019 McGraw-Hill Education.
Scatterplot of Price and Sales
How do we summarize the relationship between these two variables?
‹#›
© 2019 McGraw-Hill Education.
The Regression Line for a Dichotomous Treatment
Dichotomous treatment
Two treatment statuses—treated and untreated
Regression analysis
The process of using a function to describe the relationship among variables
‹#›
© 2019 McGraw-Hill Education.
The Regression Line for a Dichotomous Treatment : An Intuitive Approach
Draw a line through these data that will best describe the relationship between Price and Treatment
‹#›
© 2019 McGraw-Hill Education.
The Regression Line for a Dichotomous Treatment: An Intuitive Approach
In general, the formula for a line is: Y = f(X) = b + mX,
where b is the intercept and m is the slope of the line
‹#›
© 2019 McGraw-Hill Education.
Line Describing the Relationship Between Profits and Treatment
What is the equation for the line shown here?
Profits = 208.33 – 20 × Treatment
‹#›
© 2019 McGraw-Hill Education.
Line Describing the Relationship Between Profits and Price
Knowing the two point on the Profits/Price line, solve for slope and intercept
Profits = 248.33 – 40 × Price
‹#›
© 2019 McGraw-Hill Education.
The Regression Line for a Dichotomous Treatment
Whenever there is a dichotomous treatment, a line can be built describing the relationship between the treatment and outcome by using the means for each treatment status called the regression line for a dichotomous treatment
Set f(0) = and f(1)
The equation for the line is:
Outcome = +
(- ) × Treatment
‹#›
© 2019 McGraw-Hill Education.
The Regression Line for a Dichotomous Treatment: A Formal Approach
Observed outcomes in terms of two points on a line
Profiti = f(1.00) + ei if Pricei = 1.00
Profiti = f(1.50) + ei if Pricei = 1.50
i delineates between different observations, (i
ei is the residual for the observation i.
The residual is the difference between the observed outcome and the corresponding point on the regression line for a given observation
ei = Yi – f(xi)
‹#›
© 2019 McGraw-Hill Education.
Scatterplot of Residuals for Price of $1.00 when f(1.00) = $220
FIRST RESIDUAL IS 20. THIS MEANS THE ACTUAL PROFIT WE OBSERVE (240) IS 20 HIGHER THAN WHAT WE OBSERVE (220).
SECOND RESIDUAL IS -20. THIS MEANS THE ACTUAL PROFIT WE OBSERVE (200) IS 20 HIGHER THAN WHAT WE OBSERVE (220).
THIRD RESIDUAL IS -35. THIS MEANS THE ACTUAL PROFIT WE OBSERVE (240) IS 20 HIGHER THAN WHAT WE OBSERVE (185).
‹#›
© 2019 McGraw-Hill Education.
The Regression Line for a Dichotomous Treatment: A Formal Approach
Residuals for price of $1.00 when f(1.00) = $220
The average residual is [20 + (-20) + (-35)]/3 = -11.67
A choice for f(1.00) is best if it tends to neither overshoot nor undershoot the observed outcomes. That means, a choice for f(1.00) is best if the corresponding residuals are on average, zero.
‹#›
© 2019 McGraw-Hill Education.
The Regression Line for a Dichotomous Treatment: A Formal Approach
For the residuals to average zero means:
THE RESIDUALS TO AVERAGE ZERO, BEST CHOICE FOR f(1.00):
Similarly, when price is $1.50, the best choice is the average of profits when the price is $1.50 = (205 + 170 + 190)/3 = 188.33
‹#›
© 2019 McGraw-Hill Education.
The Regression Line for a Multi-Level Treatment: An Intuitive Approach
Multi-level treatment is a treatment that can be administered in more than one quantity
HERE, PRICES ARE, $1.00, $1.50, $2.00. PRICE OF $1.00 IS UNTREATED AND A $0.50 PRICE INCREASE IS THE TREATMENT.
‹#›
© 2019 McGraw-Hill Education.
The Regression Line for a Multi-Level Treatment: An Intuitive Approach
The approach we used for the dichotomous treatment generally does not work for a multi-level treatment
The problem is that when three or more points are plotted on a graph, it is generally the case that they might not fall on the same line
‹#›
© 2019 McGraw-Hill Education.
The Regression Line for a Multi-Level Treatment: An Intuitive Approach
Line attempting to connect average profits to the following price levels:
f(1.00) = 208.33
f(1.50) = 188.33
f(2.00) = 160
‹#›
© 2019 McGraw-Hill Education.
The Regression Line for a Multi-Level Treatment: An Intuitive Approach
Using the average outcome to plot the points for each treatment level generally will result in not being able to connect three points on a single line when there more than two treatment levels
f(1.00) = b + m × 1.00 208.33 = b + m × 1.00
f(1.50) = b + m × 1.50 188.33 = b + m × 1.50
f(2.00) = b + m × 2.00 160 = b + m × 2.00
We cannot solve for m and b as there are three equations to solve but only two unknowns
‹#›
© 2019 McGraw-Hill Education.
The Regression Line for a Multi-Level Treatment: An Intuitive Approach
Rather than plot an “ideal” point for each treatment level and then solve for the corresponding slope and intercept, try to directly solve for the slope and intercept of the line believed to best describe the describes the data
It should not generally overshoot or undershoot the data
Its tendency to over or undershoot the data across specific price levels should not depend on the price level
‹#›
© 2019 McGraw-Hill Education.
Two Candidate Lines for Describing Profits and Price Data
‹#›
© 2019 McGraw-Hill Education.
The Regression Line for a Multi-Level Treatment: A Formal Approach
For our example, we have three levels and nine points. Expressing them in terms of intercept and slope:
Profiti = b + m × 1.00 + ei, if Pricei = 1.00
Profiti = b + m × 1.50 + ei, if Pricei = 1.50
Profiti = b + m × 2.00 + ei, if Pricei = 2.00
Here i takes on the values one through nine, since there are nine points. Residuals, ei, are the difference between the observed profit and the corresponding point on the line for a given observation.
Ei = Profiti – b – m × Pricei
‹#›
© 2019 McGraw-Hill Education.
The Regression Line for a Multi-Level Treatment: A Formal Approach
Applying the same approach used for a dichotomous treatment, solve for the “best” line by finding a slope and intercept that makes the residuals average zero for each price point.
THIS AGAIN GIVES US THREE EQUATIONS AND TWO UNKNOWNS.
‹#›
© 2019 McGraw-Hill Education.
The Regression Line for a Multi-Level Treatment: A Formal Approach
Alternative way of defining what makes a line the best to describe the data. Criteria includes:
It should not generally overshoot or undershoot the data
Its tendency to over or undershoot the data across specific price levels should not depend on the price level
‹#›
© 2019 McGraw-Hill Education.
The Regression Line for a Multi-Level Treatment: A Formal Approach
Translating these criteria in terms of residuals:
The residuals for all data points average to zero
The size of the residuals is not correlated with the treatment level
Expressing these two criteria in equation form:
‹#›
© 2019 McGraw-Hill Education.
The Regression Line for a Multi-Level Treatment: A Formal Approach
The first equation ensures that the residual average zero across all observations, and the second equation ensures that the size of the residuals is not related to Price level
Solving these two equations yields:
m = -48.33
b = 258.06
The line that best fits the data, where “best” implies residuals that average zero and are not correlated with the treatment:
Profit = 258.06 – 48.33 × Price
‹#›
© 2019 McGraw-Hill Education.
The Regression Line for a Multi-Level Treatment: A Formal Approach
Simple regression line
The slope is the sample covariance of the treatment and outcome divided by the sample variance of the treatment
The intercept is the mean value of the outcome minus the slope times the mean value of the treatment
Y = b + mX
Solving for m and b yields the following formulas for the slope and intercept of a simple regression line:
m =
b = – m
‹#›
© 2019 McGraw-Hill Education.
The Regression Line for a Multi-Level Treatment: A Formal Approach
Applying these generalized formulas for our dichotomous price/profit example:
USING THE FORMULAS FOR VARIANCE AND COVRIANCE:
sCov (Profit, Price) = -3,
sVar (Price) = 0.075,
= 1.25 and = 198.33
Plugging these into our formulas,
m = -3/0.075 = -40, and
b = 198.33 – (-40)1.25= 248.33.
‹#›
© 2019 McGraw-Hill Education.
Sample Moments and Least Squares
Sample moment
The mean of a function of a random variable(s) for a given sample
For example, for a sample size 20 that contains information on salaries, is a sample moment, where Salaryi is the random variable and the function is defined as f(a) = a3
Ordinary least squares
The process of solving for the slope and intercept that minimize the sum of the squared residuals
Minb,m =Yi – b – mXi)2
‹#›
© 2019 McGraw-Hill Education.
Sample Moments and Least Square
Objective function
A function ultimately wished to be maximized or minimized
For ordinary least squares, the objective function is the sum of squared residuals ()
Least absolute deviations (LAD)
Use the sum of the absolute value of the residuals as the objective function and solve for the slope and intercept that minimize it
‹#›
© 2019 McGraw-Hill Education.
Ordinary Least Square vs Least Absolute Deviation for Describing a Dataset
LINE A IS CLOSER TO THE OUTLIER, SO IT IS COMING FROM OLS AND LINE B IS COMING FROM LAD.
‹#›
© 2019 McGraw-Hill Education.
Regression for Multiple Treatments
CHOLESTEROL LEVEL AND DRUG DOSES FOR 15 INDIVIDUALS.
‹#›
© 2019 McGraw-Hill Education.
Regression for Multiple Treatments
Single vs. Multiple Treatments
Cholesterol = 235.17 – 0.997 × Drug A
Cholesterol = 205.83 – 0.107 × Drug B
Cholesterol outcome as follows:
Cholesteroli = b + m1DrugAi + m2DrugBi + ei
Expressing the OLS criteria in equation form:
‹#›
© 2019 McGraw-Hill Education.
Regression Output in Excel for Cholesterol Regressed on Drug A and Drug B
HERE WE HAVE THE VALUES FOR:
b = 256.20,
m1 = -1.259, AND
m2 = -0.514.
‹#›
© 2019 McGraw-Hill Education.
Regression Plane for Cholesterol Regressed on Drug A and Drug B
‹#›
© 2019 McGraw-Hill Education.
Multiple regression
Solving for a function that best describes the data the implies the use of OLS (or equivalently, the sample moment equations)
Single regression the process that produces the simple regression line for a single treatment
Multiple Regression
‹#›
© 2019 McGraw-Hill Education.
Multiple Regression
For a sample size of N with K treatments, the associated equations are:
‹#›
© 2019 McGraw-Hill Education.
What Makes Regression Linear?
Linear regression is the process of fitting a function that is linear in its parameters to a given dataset
Y = b + m1X1 + m2X2 + … + mKXK
Here {b, m1, …, mK} are the parameters for this function
The use of linear regression does not at all imply construction of a line to fit the data
Linear regression is linear in the parameters but not necessarily the treatment(s)
It allows for an unlimited number of possible “shapes” for the relationship between the outcome and any particular treatment
‹#›
© 2019 McGraw-Hill Education.
image1.png
image2.png
image3.JPG
image4.png
image5.png
image6.png
image7.png
image8.png
image7.JPG
image9.png
image10.JPG
image11.JPG
image12.png
image13.png
image14.png
image15.png
image16.png
image17.JPG
image18.JPG
image20.png
image19.JPG
image22.png
image21.png
image23.png
image24.JPG
image25.JPG
image26.JPG
image27.JPG
image28.JPG
image29.JPG
Collepals.com Plagiarism Free Papers
Are you looking for custom essay writing service or even dissertation writing services? Just request for our write my paper service, and we'll match you with the best essay writer in your subject! With an exceptional team of professional academic experts in a wide range of subjects, we can guarantee you an unrivaled quality of custom-written papers.
Get ZERO PLAGIARISM, HUMAN WRITTEN ESSAYS
Why Hire Collepals.com writers to do your paper?
Quality- We are experienced and have access to ample research materials.
We write plagiarism Free Content
Confidential- We never share or sell your personal information to third parties.
Support-Chat with us today! We are always waiting to answer all your questions.
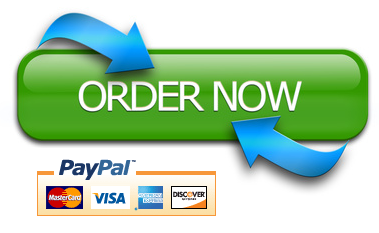