programming case study This is the data analysis/ collecting work. Go through the attached documentation for the data collection. Once the final analysis document is fill
programming case study
This is the data analysis/ collecting work. Go through the attached documentation for the data collection.
Once the final analysis document is filled with all the values then feed the document to any visualization tool such as power BI or Tabule to obtain the demarkation of renters Vs Owner.
Also provide the 2nd excel sheet from the Consolidated Planning/CHAS Data | HUD USER(You have to create a new excel sheet for this with col names )
Requirements:
Research Experience I
Abstract
Interest in the global unaffordable housing dilemma is manifest in its growing publications. However, there is limited systematic review of the literature concerning data visualization and mapping of the structure of knowledge and trend of publications on housing worldwide. As part of the research experience, first, we will conduct a literature review analysis on profiling affordable housing residents, second perform multifaceted linkage between ACS and AHS datasets, and third, we will perform exploratory clustering analysis to create profiles based on factors stated above and identified from the literature.
Introduction
Housing is one of the most crucial factors in a country’s socioeconomic development. As a result, an effective housing supply is one of the government’s policies. These regulations are frequently implemented to ensure housing affordability for people of all income levels, particularly those in the middle- and lower-income brackets [1]. Housing affordability refers to getting a particular standard of housing at a price or rent that does not impose an undue strain on household incomes in the eyes of a third party (typically the government). Several words have been coined to characterize housing that is affordable to middle- and low-income earners, as well as the impoverished. Depending on the country, several of these phrases could refer to various housing tenures. Affordable housing (which is commonly used in the United States and can refer to both rental and ownership housing); public and social housing (which are more strongly linked to rental housing in a European context); cooperative housing (which issued in some European countries and refers to housing where the entire building is owned in common by a homeowner; association), and so on [3]. Despite the benefits of maintaining housing affordability and accessibility for socioeconomic growth, the global housing affordability dilemma continues to be unsolvable. In both rich and developing countries, the housing crisis is a big issue. This is demonstrated by a lack of housing facilities, which has the consequence of promoting the construction of slums (overcrowded and dilapidated urban residential facilities with inadequate infrastructure) [4]. Apart from the housing shortages, several of the existing facilities are insufficient. If left unchecked, the housing scarcity and its inevitable corollary could get worse, given that the world& population is expected to expand from 3.6 billion to 6.3 billion by 2050[4]. In response to the global housing problem, international organizations such as the World Bank and the United Nations (UN) have begun to develop policies to ensure enough housing [5]. As a result, in addition to assuring housing affordability, achieving social and environmental sustainability goals for comprehensive sustainable development continues to be a hot topic in both developed and developing countries. Much empirical research on the various aspects of sustainable housing has been undertaken to achieve these goals. The abundance of studies broadens the knowledge foundation on which policymakers can base their decisions. More crucially, a systematic evaluation of the expanding articles on housing could enhance the effect of existing knowledge for policy formation.
Research Methodology:
Exploratory research has been conducted based on the data available for the owners Vs renters in the public databases. The post covid year 2019 is considered for the research because of the data accuracy. The census () has the data for the house owners Vs renters based on the number of housing units. There are certain data sets are collected based on this and Florida housing website ( ) also provide the information on the data regarding the homeowners Vs renters but there was no county level data available at the Florida housing data.
Below is the search conducted. And based on the source of the information provided for these data set. Further research is conducted to explore the source of the Florida housing
Figure 1: Screen shot of the Florida housing website search
Based on the data set some of the fields in the data set has values which determine the renter’s vs owners
And collectively I have selected these because of the clear demarcation of the data. The next data set selected was from the CHAS (Consolidated planning data set) . The search for the dataset
CHAS Data base
The CHAS data’s main purpose is to show the number of households needing housing assistance. This is estimated by the number of households that have certain housing problems and have income low enough to qualify for HUDs programs (primarily 30, 50, and 80 percent of median income). It is also important to consider the prevalence of housing problems among diverse types of households, such as the elderly, disabled, minorities, and different household types. The CHAS data provides counts of the numbers of households that fit these HUD-specified characteristics in HUD-specified geographic areas.
In addition to estimating low-income housing needs, the CHAS data contribute to a more comprehensive market analysis by documenting issues like lead paint risks, “affordability mismatch,” and the interaction of affordability with variables like age of homes, number of bedrooms, and type of building.
Data Format
HUD has identified many characteristics of interest to housing planners and policymakers, and as a result, the CHAS data can be unwieldy. To streamline the data and make it easier to use, HUD has created a series of “tables,” which are grouped by theme.
Each of these tables contains certain “dimensions” (also referred to as variables). These dimensions can be combined in many ways, and the data files for each table present every combination. As an example, consider Table 11. Table 11 contains 3 dimensions: tenure, housing problems, and household income. Tenure has 2 options: owner-occupied or renter occupied. Housing problems has 3 options: household has at least one housing problem, household has no housing problems, or household has no income (so cost burden could not be computed) but no other housing problems. Household income, in this table, has 13 options. Thus Table 11 has 78 buckets (2*3*13=78), and every household belongs in one (and only one) of those buckets. In the CHAS data, we have counted the number of households in each of those buckets, for thousands of states, counties, cities, and neighborhoods.
Because CHAS provides count estimates, users interested in percentage estimates (e.g., percentage of low-income renters with severe housing cost burden) will need to make the calculation themselves. To make these calculations, users may need to sum count estimates (buckets) in both the numerator and denominator before dividing to obtain the percentage estimate. Users should be careful to identify the appropriate total or subtotal that serves as the denominator. In some cases, totals and subtotals are provided in the CHAS data.
Below are the data sets selected from the CHAS dataset. These have the renters and owners’ demarcation.
Housing Cost Burden Overview 3
Income by Housing Problems (Renters)
Income by Housing Problems (Owners)
Income by Cost Burden (Renters only)
Income by Cost Burden (Owners only)
In the census data base, some of the data sets chosen for this study are as below:
The data set below from the census have the percentage of homeowners Vs renters’ data which is available and based on the level of income from the CHAS data set the data is planned to be combined. Based on the level of income between the 2 data sets.
The project plan is to include the
Data Set S2502 ? Demographic characteristics for occupied housing unit
AGE OF HOUSEHOLDER -Percentage occupied housing unit Vs Owner Occupied housing unit
Under 35 years
35 to 44 years
45 to 54 years
55 to 64 years
65 to 74 years
75 to 84 years
85 years and over
YEAR HOUSEHOLDER MOVED INTO UNIT- Percentage occupied housing unit Renter Vs Owner Occupied housing unit
Moved in 2019 or later
Moved in 2015 to 2018
Moved in 2010 to 2014
Moved in 2000 to 2009
Moved in 1990 to 1999
Moved in 1989 or earlier
S2501- Occupancy Characteristics data set – Percentage occupied housing unit Renters Vs Owner
HOUSEHOLD SIZE
1-person household
2-person household
3-person household
4-or-more-person household
OCCUPANTS PER ROOM- Percentage occupied housing unit Renters Vs Owner
1.00 or less occupants per room
1.01 to 1.50 occupants per room
1.51 or more occupants per room
S2403- Financial characteristics
Occupied housing units:
HOUSEHOLD INCOME IN THE PAST 12 MONTHS (IN 2021 INFLATION-ADJUSTED DOLLARS)- Percentage occupied housing unit Renters Vs Owner
Less than $5,000
$5,000 to $9,999
$10,000 to $14,999
$15,000 to $19,999
$20,000 to $24,999
$25,000 to $34,999
$35,000 to $49,999
$50,000 to $74,999
$75,000 to $99,999
$100,000 to $149,999
$150,000 or more
Median household income (dollars)
S2504PHYSICAL HOUSING CHARACTERISTICS FOR OCCUPIED HOUSING UNITS
Occupied housing unit- Percentage occupied housing unit Vs Owner
YEAR STRUCTURE BUILT
2014 or later
2010 to 2013
2000 to 2009
1980 to 1999
1960 to 1979
1940 to 1959
1939 or earlier
ROOMS
1 room
2 or 3 rooms
4 or 5 rooms
6 or 7 rooms
8 or more rooms
BEDROOMS
No bedroom
1 bedroom
2 or 3 bedrooms
4 or more bedrooms
S2506FINANCIAL CHARACTERISTICS FOR HOUSING UNITS WITH A MORTGAGE
Owner-occupied housing units with a mortgage– Percentage occupied housing unit Vs Owner
VALUE
Less than $50,000
$50,000 to $99,999
$100,000 to $299,999
$300,000 to $499,999
$500,000 to $749,999
$750,000 to $999,999
$1,000,000 or more
HOUSEHOLD INCOME IN THE PAST 12 MONTHS (IN 2019 INFLATION-ADJUSTED DOLLARS)
Less than $10,000
$10,000 to $24,999
$25,000 to $34,999
$35,000 to $49,999
$50,000 to $74,999
$75,000 to $99,999
$100,000 to $149,999
$150,000 or more
B08137- Means of Transportation to work by tenure
Total:
Householder lived in owner-occupied housing units
Householder lived in renter-occupied housing units
Car, truck, or van – drove alone:
Householder lived in owner-occupied housing units
Householder lived in renter-occupied housing units
Car, truck, or van – carpooled:
Householder lived in owner-occupied housing units
Householder lived in renter-occupied housing units
Public transportation (excluding taxicab):
Householder lived in owner-occupied housing units
Householder lived in renter-occupied housing units
Walked:
Householder lived in owner-occupied housing units
Householder lived in renter-occupied housing units
Taxicab, motorcycle, bicycle, or other means:
Householder lived in owner-occupied housing units
Householder lived in renter-occupied housing units
Worked from home:
Householder lived in owner-occupied housing units
Householder lived in renter-occupied housing units
Plan for the Research II
The next step for the research is to extract the county level data from the census database from the below files for the year 2019. This research is considering only the data from 2019 as the data obtained for 2019 will be more accurate as there will not be any impact of the covid 19 pandemic.
S2502 ? Demographic characteristics for occupied housing unit
S2501- Occupancy Characteristics data set
S2403- Financial characteristics
S2504-PHYSICAL HOUSING CHARACTERISTICS FOR OCCUPIED HOUSING UNITS
S2506-FINANCIAL CHARACTERISTICS FOR HOUSING UNITS WITH A MORTGAGE
The data for the S2403- Financial characteristics
Occupied housing units:
HOUSEHOLD INCOME IN THE PAST 12 MONTHS (IN 2021 INFLATION-ADJUSTED DOLLARS)- Percentage occupied housing unit Vs Owner
Less than $5,000
$5,000 to $9,999
$10,000 to $14,999
$15,000 to $19,999
$20,000 to $24,999
The once color coded in GREEN will be Bucket I
$25,000 to $34,999
$35,000 to $49,999
The one color coded in PINK will be Bucket 2
$50,000 to $74,999
$75,000 to $99,999
The one color coded in PINK will be Bucket 3
$100,000 to $149,999
$150,000 or more
The one color coded in PINK will be Bucket 4
The data from this data set will be prepared with this bucket list manually for a single county and later the same mechanism will extend to all the counties of Florida. Based on the Percentage occupied housing unit Vs Owner which is the common unit for the all the data sets above. The data will be combined based on the comparison in unit and percentage for the renters Vs Owners.
Each county level data will be manually downloaded to the local system and all the files will be stored in the extended hard drive. The naming convention will be based on the county.
Ex: All the below files will be stored in the separate folder named Duval County.
S2502 ? Demographic characteristics for occupied housing unit
S2501- Occupancy Characteristics data set
S2403- Financial characteristics
S2504-PHYSICAL HOUSING CHARACTERISTICS FOR OCCUPIED HOUSING UNITS
S2506-FINANCIAL CHARACTERISTICS FOR HOUSING UNITS WITH A MORTGAGE
The dataset will also have a new column named County added to the final data set.
The B08137- Means of Transportation to work by tenure dataset is ignored for now as it does not have a clear comparison for the renters Vs Owners. Later part of the research we must see if this is usable.
Later the comparison will be done with the data set created from the census data and to the CHAS dataset with the selection of Income by Cost Burden (Renters only) and Income by Cost Burden (Renters only) and Percentage occupied housing unit Renters Vs Owner. Using tablue cluster modeling will be created to show the pictorial images and provide the insight based on the grouping or clustering of the data in the data. Data comparison will be done with the data analyzed from census and data obtained from the fl housing for 2019 on county bases.
References
1.
2. .
3.
References:
[1] Adabre, M. A., & Chan, A. P. (2019). Critical success factors (CSFs) for sustainable affordable
housing. Building and Environment, 156, 203?214.
[2] MacLennan, D., & Williams, R. (1990). Affordable housing in Britain and America. York: Joseph
Rowntree Foundation.
[3] Czischke, D., & van Bortel, G. (2018). An exploration of concepts and polices on ?affordable housing
in England, Italy, Poland and The Netherlands. Journal of Housing and the Built Environment, 1?21.
[4] Golubchikov, O., & Badyina, A. (2012). Sustainable housing for sustainable cities: a policy
framework for developing countries. Nairobi, Kenya: UNHABITAT
Definitions:
HAMFI ? HUD Area Median Family Income. This is the median family income calculated by HUD for each authority, to determine Fair Market Rents (FMRs) and income limits for HUD programs. HAMFI will not necessarily be the same as other calculations of median incomes (such as a simple Census number), due to a series of adjustments that are made (For full documentation of these adjustments, consult the ). If you see the terms “area median income” (AMI) or “median family income” (MFI) used in the CHAS, assume it refers to HAMFI.
Household ? All people living in a housing unit. Members of a household can be related (see family) or unrelated.
Household Income ? Adjusted household income, which includes the income of all members of the household at the time of the survey, adjusted for inflation to reflect the most recent year of the data release (e.g. 2013 dollars in the 2009-2013 CHAS data).
Family ? Related individuals living in the same household. The Census Bureau also tracks subfamilies.
Housing Problems ? There are four housing problems in the CHAS data: 1) housing unit lacks complete kitchen facilities; 2) housing unit lacks complete plumbing facilities; 3) household is overcrowded; and 4) household is cost burdened. A household is said to have a housing problem if they have any 1 or more of these 4 problems.
Overcrowding ? More than 1 person per room.
Severe overcrowding ? More than 1.5 persons per room.
Cost burden ? Monthly housing costs (including utilities) exceeding 30% of monthly income.
Severe cost burden ? Monthly housing costs (including utilities) exceeding 50% of monthly income.
Elderly ? People aged 62 and up. Individuals aged 75 and up are recognized as a population with unique needs than those 62-74, so the CHAS data separates these groups. “Elderly” refers to individuals 62-74, while those 75 and up may be referred to as “extra elderly” or “frail elderly”.
Disabled ? In 2008, Census modified the ACS questions related to disability. Beginning with the 2008-2010 and 2008-2012 CHAS data, HUD has separately identified four different physical or cognitive limitations: hearing or vision impairment, ambulatory limitation, cognitive limitation, and independent living limitation
Steps :
Go to ()
Go to Data and Maps then click on GO TO DATA CENSUS.GOV
4. Use the below data set and get the values for these are add it to the Final Analysis excel document both for the renters and owners.
Data Set S2502 ? Demographic characteristics for occupied housing unit
AGE OF HOUSEHOLDER -Percentage occupied housing unit Vs Owner Occupied housing unit
Under 35 years
35 to 44 years
45 to 54 years
55 to 64 years
65 to 74 years
75 to 84 years
85 years and over
YEAR HOUSEHOLDER MOVED INTO UNIT- Percentage occupied housing unit Renter Vs Owner Occupied housing unit
Moved in 2019 or later
Moved in 2015 to 2018
Moved in 2010 to 2014
Moved in 2000 to 2009
Moved in 1990 to 1999
Moved in 1989 or earlier
S2501- Occupancy Characteristics data set – Percentage occupied housing unit Renters Vs Owner
HOUSEHOLD SIZE
1-person household
2-person household
3-person household
4-or-more-person household
OCCUPANTS PER ROOM- Percentage occupied housing unit Renters Vs Owner
1.00 or less occupants per room
1.01 to 1.50 occupants per room
1.51 or more occupants per room
S2403- Financial characteristics
Occupied housing units:
HOUSEHOLD INCOME IN THE PAST 12 MONTHS (IN 2021 INFLATION-ADJUSTED DOLLARS)- Percentage occupied housing unit Renters Vs Owner
Less than $5,000
$5,000 to $9,999
$10,000 to $14,999
$15,000 to $19,999
$20,000 to $24,999
$25,000 to $34,999
$35,000 to $49,999
$50,000 to $74,999
$75,000 to $99,999
$100,000 to $149,999
$150,000 or more
Median household income (dollars)
S2504PHYSICAL HOUSING CHARACTERISTICS FOR OCCUPIED HOUSING UNITS
Occupied housing unit- Percentage occupied housing unit Vs Owner
YEAR STRUCTURE BUILT
2014 or later
2010 to 2013
2000 to 2009
1980 to 1999
1960 to 1979
1940 to 1959
1939 or earlier
ROOMS
1 room
2 or 3 rooms
4 or 5 rooms
6 or 7 rooms
8 or more rooms
BEDROOMS
No bedroom
1 bedroom
2 or 3 bedrooms
4 or more bedrooms
S2506FINANCIAL CHARACTERISTICS FOR HOUSING UNITS WITH A MORTGAGE
Owner-occupied housing units with a mortgage– Percentage occupied housing unit Vs Owner
VALUE
Less than $50,000
$50,000 to $99,999
$100,000 to $299,999
$300,000 to $499,999
$500,000 to $749,999
$750,000 to $999,999
$1,000,000 or more
HOUSEHOLD INCOME IN THE PAST 12 MONTHS (IN 2019 INFLATION-ADJUSTED DOLLARS)
Less than $10,000
$10,000 to $24,999
$25,000 to $34,999
$35,000 to $49,999
$50,000 to $74,999
$75,000 to $99,999
$100,000 to $149,999
$150,000 or more
B08137- Means of Transportation to work by tenure
Total:
Householder lived in owner-occupied housing units
Householder lived in renter-occupied housing units
Car, truck, or van – drove alone:
Householder lived in owner-occupied housing units
Householder lived in renter-occupied housing units
Car, truck, or van – carpooled:
Householder lived in owner-occupied housing units
Householder lived in renter-occupied housing units
Public transportation (excluding taxicab):
Householder lived in owner-occupied housing units
Householder lived in renter-occupied housing units
Walked:
Householder lived in owner-occupied housing units
Householder lived in renter-occupied housing units
Taxicab, motorcycle, bicycle, or other means:
Householder lived in owner-occupied housing units
Householder lived in renter-occupied housing units
Worked from home:
Householder lived in owner-occupied housing units
Householder lived in renter-occupied housing units
The next step for the research is to extract the county level data from the census database from the below files for the year 2019. This research is considering only the data from 2019 as the data obtained for 2019 will be more accurate as there will not be any impact of the covid 19 pandemic.
S2502 ? Demographic characteristics for occupied housing unit
S2501- Occupancy Characteristics data set
S2403- Financial characteristics
S2504-PHYSICAL HOUSING CHARACTERISTICS FOR OCCUPIED HOUSING UNITS
S2506-FINANCIAL CHARACTERISTICS FOR HOUSING UNITS WITH A MORTGAGE
The data for the S2403- Financial characteristics
Occupied housing units:
HOUSEHOLD INCOME IN THE PAST 12 MONTHS (IN 2021 INFLATION-ADJUSTED DOLLARS)- Percentage occupied housing unit Vs Owner
Less than $5,000
$5,000 to $9,999
$10,000 to $14,999
$15,000 to $19,999
$20,000 to $24,999
The once color coded in GREEN will be Bucket I
$25,000 to $34,999
$35,000 to $49,999
The one color coded in PINK will be Bucket 2
$50,000 to $74,999
$75,000 to $99,999
The one color coded in PINK will be Bucket 3
$100,000 to $149,999
$150,000 or more
The one color coded in PINK will be Bucket 4
5. Then go to
6. Check for only these header level columns in the downloaded data set
Below are the data sets selected from the CHAS dataset. These have the renters and owners’ demarcation.
Housing Cost Burden Overview 3
Income by Housing Problems (Renters)
Income by Housing Problems (Owners)
Income by Cost Burden (Renters only)
Income by Cost Burden (Owners only)
7.Based on the county name download the excel sheet and prepare comparison between the 2 data sets and show the comparison in excel pie chart or pie chart.
Collepals.com Plagiarism Free Papers
Are you looking for custom essay writing service or even dissertation writing services? Just request for our write my paper service, and we'll match you with the best essay writer in your subject! With an exceptional team of professional academic experts in a wide range of subjects, we can guarantee you an unrivaled quality of custom-written papers.
Get ZERO PLAGIARISM, HUMAN WRITTEN ESSAYS
Why Hire Collepals.com writers to do your paper?
Quality- We are experienced and have access to ample research materials.
We write plagiarism Free Content
Confidential- We never share or sell your personal information to third parties.
Support-Chat with us today! We are always waiting to answer all your questions.
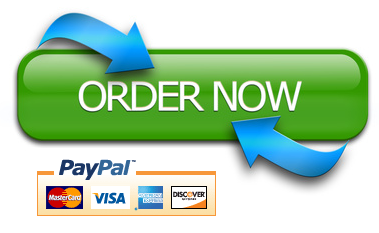