The goal of the reflection paper is for you to summarize your own take-aways from the course. Here is a suggested outline for the reflection paper: Please choose three news
The goal of the reflection paper is for you to summarize your own take-aways from the course. Here is a suggested outline for the reflection paper:
- Please choose three news items that are related to supply chains and that appeared in the media (US or otherwise) during this term. Summarize each news item briefly.
- For each news item, describe why you found it relevant to our course—for example, of which topic(s) or case(s) does the item remind you?
- What is it about this news item that compelled you to include it in your reflection paper—for example, what is it about this item that strikes you as most interesting, surprising, or informative?
Format
The reflection paper should not exceed two pages when typed in 12-point Times New Roman font with 1.5-line spacing and 1-inch margins on all sides. (You do not have to follow this format, but please make sure that the paper is no longer than two pages when formatted so.)
JHU Carey Business School | BU_610.760_SUPPLY CHAIN ANALYTICS_G.AYDIN_MODULE 4_VIDEO 3
INSTRUCTOR: Let's now put these ideas together around inventory pooling. I'm switching to inventory pooling at this point because this is going to be one of the central ideas about distribution networks, the idea of inventory pooling. What do we mean by inventory pooling?
Going with my smaller example, and we're going to generalize from here eventually, but going with my smaller
example, if ABC decides to pool all of its inventory in one location, I'm missing the word one there on that slide, so in one location. They are essentially saying I'll take the East and West warehouses, I'll combine them in one
location. Let's call it the central warehouse. That's– at this very basic level, is inventory pooling. I'll take two
locations at which are used to keep inventory, I'll put them in one and the same location.
So, when we do this, we can assume that the week demand at the central warehouse will be the sum of the
weekly demands at the East and West warehouses. Whatever demand was coming to the East and the West will now be channeled to the central warehouse. And let's also assume that the lead time from the plants to the
central warehouse will still be three weeks. Remember, when we had two separate warehouses, it was taking
three weeks for the plant to send the shipment to the East or to the West. Let's say to the central warehouse also, it takes three weeks to send the shipment from the plant.
Let's also assume that ABC decided to maintain the same total safety stock as before because in the last part of the example, we calculated if I want 95% cycle service level, here is the safety stock I need in the East. Here is
the safety stock I needed in the West, right? So now I'm saying when they replace the East and the West with
one warehouse called the central warehouse, they decided to keep the same safety stock. So they will add the
two safety stocks together, 65 from the East, 98 from the West, a total of 164 units of safety stock in this new
central warehouse.
The question I'm asking at the end is, what is the cycle service level ABC will achieve at this central warehouse, and how does it compare to the 95% cycle service level ABC was achieving before pooling when it had the East and the West separately? So let's answer this question. What is the cycle service level ABC will achieve at the
central warehouse? To be able to answer it, I'm going to have to remind you again a couple of things from your
statistics classes, probability classes, and so on.
But the key thing we need to figure out before we can answer that question is, what is the weekly demand at the
central warehouse? What do we know about that? Well, what we know about the weekly demand at the central warehouse, let me back up by one slide. It's the sum of the demands in the East and the West. I know the
demand in the East, it's some normal distribution. I know the demand in the West. It's some normal distribution. The demand in the central warehouse is the sum of the two. So it's adding two normal distributions together.
How do we get that resulting demand distribution for the central? So, recall from your statistics courses that if I have x and y and both of them are normally distributed variables and let's say they are statistically independent, meaning there is no correlation between them. Let's say the mean of x is given by mu sub x as notation, and
sigma sub x is the standard deviation of x. So the variance of x would be sigma x squared. Let's say the mean
and standard deviation of y are mu sub y and sigma y. So the variance of y is sigma y squared.
Then here's what I know about when I add x and y together. Here's what I know about x plus y. x plus y would
also be normally distributed. It's mean would be the mean of x plus the mean of y. Makes sense, right? Take the
mean of x, take the mean of y, add the two together, you get the mean of x plus y. And its variance would be the
variance of x plus the variance of y. Again, hopefully that makes sense. Take the variance of x, take the variance
of y, add the two together, you get the variance of x plus y.
By the way, if this is the variance of x plus y, then you know that standard deviation is a square root of variance. So the standard deviation of x plus y would be the square root of that. So we can now apply this to ABC. The
weekly demand at the central warehouse, it's the sum of the East and West demands, right? East demand is
normal, West demand is normal. Therefore, the weekly demand at the central warehouse also has a normal distribution. Its mean would be the mean of East plus the mean of West. Mean of East is 100, mean of West is
100, that's 200. So I know the mean of weekly demand in central warehouse.
Its variance is, OK, that's the variance of East plus the variance of West. What is the variance of East? Well, back
up a couple of slides here. We know the standard deviation in the East. The standard deviation in the East is 20. Therefore, its variance is 20 squared. So the variance of East is 20 squared. Plus, the variance of y. What is the
variance of y? Its standard deviation is 30, if you remember from the example, the variance of the West warehouse. Multiply– take the square of that. So the variance of the central warehouse demand is going to be if you do the algebra there, that algebra comes out to 1,300. And therefore, the standard deviation at the central warehouse is the square root of 1,300, which gives me 36.1.
So there is my answer to the question about what is the weekly demand at the central warehouse? It's normally
distributed. It's mean is 200, its standard deviation is 36.1. And now that I have that, I can go back and calculate
the cycle service level we are achieving at the central warehouse, and this gives me one more opportunity to
repeat once more the mechanics of going from an order-up-to level to going from an order-up-to level or safety
stock to a cycle service level. So at the central warehouse, here's what I know is my input data. I calculate the
safety stock that I must have at the central warehouse, right? It was the safety stock of the East plus the safety
stock of the West. So we calculated that earlier to be 164 units.
We know that the lead time there is three weeks, and we know that we just calculated the standard deviation of weekly demand at the central warehouse is 36.1 units. So what is sigma sub L? Again, remember what sigma
sub L means. If sigma is standard deviation of per-period demand, the standard deviation of weekly demand, let's say, sigma sub L is standard deviation of total demand during L plus 1 periods. And that is square root of L
plus 1 times the sigma itself.
L is 3 plus 1 times sigma, we calculated earlier 36.1. If you do the algebra there, that comes out to be 72.2. Now I can calculate z, what we're calling the safety factor. The formula for safety factor is here. Take the safety stock
divided by sigma sub L. Safety stock is here, 164.5 divided by sigma sub L is here, 72.2. That comes out to be
2.27. And finally, the cycle service level, I can calculate that by plugging into this Excel function NORMSDIST, short for normal standard distribution. Plug the z value in there. It will give you 98.8%.
Now notice the result. At the central warehouse, the cycle service there will be 98.8%. When we were keeping
the inventory separately in the East and the West, the cycle service level in each was 95%. So by putting the two
together, we improved our cycle service level even though we did not increase our overall safety stock. We just combined it in one place and the formulas tell us that the cycle service level will improve. Why is this happening?
Well, because in general, inventory pooling, we expect will improve customer service level by combining safety
stocks in one location. The intuition behind this is similar to diversifying your portfolio of investments. You don't put all your money in GameStop, because if GameStop goes down, then you lose all your money. You don't put all of your money in crypto, because if crypto goes down, then you lose all your money. Instead, you diversify
your portfolio of investments so you're not too vulnerable to changes in one stock only.
The idea is similar here. When we pool our inventory to meet the demands from multiple sources– in this case, East and the West– there will be times when the East demand is high and the West demand is low, and there will be times when the West demand is high and East demand is low. Now you add the two together, those are– the
sum is sort of curves, the peaks and valleys that you see in each. It leads to a more stable demand pattern
overall, and when you have a more stable pattern overall, it becomes easier to offer better service to your
customers.
So that's the intuition behind why the cycle service level went from 95% when we had two separate locations, to
98% when we combine those two in the same location. But, of course, inventory pooling can have lots of disadvantages that our small example did not really account for. So, for example, you might have some
customers in the far side of the East and the far side of the West, and they might say, you know what? You took
away my warehouse and you put it in the center. It's too far away from me now, so I'll stop buying from you. So
that would be one possible disadvantage of consolidating two warehouses from– in the center.
It's also true that you're now going to be transporting over larger distances by shipping to customers. When you
had East and the West, East was serving only East customers, West was serving only West customers. Now
central will have to serve customers both in the East and the West. So your customers, your shipments from your
warehouse to your customers, will travel longer distances which might mean that your transportation costs will go up. So those are some possible disadvantages of inventory pooling. And we'll see some of those in our next two cases.
But at this point, the fundamental trade-off in designing distribution networks, generally, we expect that when we
pool the inventory at different locations, it helps us serve our customers better with even less safety stock. So
that's a good thing. Let's say stocks, better service, win-win, by pooling inventory in fewer locations. But also, it puts us further away from our customers if we have fewer locations. And that could have disadvantages. So how
do we manage this trade-off? This is some of what we will see as we move on to cases to look at more elaborate
examples of this inventory pooling idea and distribution networks.
,
JHU Carey Business School | BU.610.760_SUPPLY CHAIN ANALYTICS_G.AYDIN_MODULE 5_VIDEO 1
INSTRUCTOR: Hi, everyone. Welcome to module 5. In this module, we're going to go back to distribution networks. We're going
to talk about our case, Imagen Healthcare. And most of what we will do in this module will be about the case
itself. And we're going to actually evaluate three things– one, what's happening in the status quo in the case, two, evaluate option one, which is what we will call pairwise inventory sharing, and evaluate option two, the
collective inventory sharing.
So by now, you read the case. And you're aware of the decisions at hand. So let's dive straight into it. And let's
talk about Imagen Healthcare's status quo. What is the safety stock needed for 90% fill rate at each dealer?
We are told in the case description that each dealer uses order-up-to policy to manage the inventory of the RF
amplifier, that spare part that we are interested in. And the lead time for each dealer, when ordering from the
plant, is four weeks. We have enough data in the case to estimate the weekly demand at each dealer. In other
words, we can fit a distribution to weekly demand data at each dealer. And we are told that each dealer keeps
sufficient safety stock to achieve 90% fill rate.
This is actually similar to examples we covered before. We can set up a model to determine the safety stock
needed at each dealer. And I'll show you an example of that using Buffalo– the dealer in Buffalo– as an example. So for our work on this, you can see the spreadsheet Status Quo Buffalo and its video. Let me now go to the
spreadsheet and cover that.
So here's my spreadsheet, Status Quo Buffalo. As usual, on Canvas, I have two versions of the spreadsheet. One
is a template. The other one is a complete version. You can either follow along from the template and fill in with
me, or follow from the complete version, see what we are building up to.
I have two tabs in this spreadsheet. One is called Simulation, the other is called Demand Data. So let me start with the Demand Data tab here. What you're going to notice in the Demand Data tab is that it reproduces the
demand data that we were given in the case. And for each of, I think, 50 weeks of data, or rather 52 weeks of data, for each dealer– Atlanta, Buffalo, Denver, Houston– we are told what the demand was in that week. We are
focusing on Buffalo for this example.
The first thing that I want to do with this data is, I have 52 weeks of demand observations in Buffalo. I want to fit a demand distribution to this data so that I have a handle on what kind of demand we are expecting in Buffalo. So let me start @RISK here.
I'll zoom in a little bit. So I'm going to go to Fit. From the dropdown menu, choose Fit. And the next thing that I want to do is I want to make sure that I have the right range of data that I chose to do a fit. I'm looking at Buffalo's demand, all of these. And I'm fitting a distribution to those demand observations. Those are the weekly
demand observations in Buffalo.
It's suggesting a name already for it, based on the title of the column, Buffalo, NY. That name is fine with me. The
next decision we need to make is whether we're going to fit a continuous distribution or a discrete distribution to
this demand data. I will choose discrete here. Continuous would mean that we would allow fractional demand
values, like 10 1/2, 2.5, and so on.
That wouldn't be really much of a problem if I went that way. But I'm going to choose discrete, mainly because
the demand numbers are on the lower side. And these are important, expensive spare parts that we're talking
about. So I don't want to allow fractional inventory. I want to make sure that I treat these units as the individual, distinct units they are.
So I'm going to choose discrete sample data. Click OK. I'll wait for the next dialog box to appear. And we have
seen examples of this earlier. Let me, again, talk about how to interpret what we have in front of us.
First of all, you're going to see under the input column, the blue column, some of the statistical properties of the
input data. This is the input data, as in the 52 weeks of demand data in Buffalo. What was the minimum demand
across to 52 weeks, what was the maximum demand, what was the average demand across 52 weeks in Buffalo, and so on?
And here on the upper left, we are given some choices about what could be a good fit for this demand distri– for
this demand data. The top choice is a uniform distribution. And the visual graphic here is showing us how the
input data compares to the proposed distribution by @RISK. So for example, the blue bars are showing you that there was– about 9 1/2 percent of the time, the demand was just below 20. About 9% of the time, the demand
was just about 25. Here, there was about 4% of cases where the demand in Buffalo was 35 or so.
Looking at this data in Buffalo, the @RISK is suggesting that the best fit it could find was a uniform distribution at the top choice. And it's showing you, as red bars, what uniform distribution looks like. As the name implies, uniform distribution assumes uniform, equal chance for every outcome between the minimum, about 2 units of demand, and the maximum, about 35 units of demand.
So we could choose this. We could go with uniform as our best fit. Although, I want to uncheck that one and show
you the next one, too. This is Negbin, short for negative binomial, which is a distribution, really. And it looks like
this one here. It has higher chance of occurrence in the middle. And as you go to the tails, either to the left or to
the right, the chance of occurrence goes down.
So the question is, which one of these do you like better? I could choose either one of the two, because @RISK is
suggesting these as the best possible fit. I'm going to actually go with negative binomial, because I feel like the
red bars shown here for negative binomial are a better representation of what's happening with the actual blue
bars that show my demand in Buffalo.
Integer uniform wasn't quite as good a fit, in my opinion. It wasn't capturing the more likely demands occurring
around this middle point. When I choose negative binomial, at least the peak of the red bars is capturing a little
bit better these high likelihood scenarios shown by the high blue bars here.
So I'm going to choose negative binomial as my distribution. Click right to Excel. I'll accept everything that it's
suggesting here, the names and so on. Click Next.
The next thing you need to choose is where do you want to place this on your spreadsheet. I'm going to place
this in this cell, as the name states, fitted demand distribution for Buffalo. So click OK. And now you'll see a
number there.
Even though you're seeing a number here, remember it's a placeholder. If you double click on that, behind it, you'll see a formula, which is telling you Excel and @RISK know that the demand in Buffalo is a random variable. It's given by this negative binomial distribution with particular parameters. But of course, when you click Enter
there, it's going to have to show you something. So it's showing a number as a placeholder.
Similarly, we'd want to calculate the average demand we have observed in Buffalo. So I'm going to take the
average of all weeks of the demands I observed in Buffalo so that I have a record of what was the demand in
Buffalo on average. That averages about 23.36. So what we have done so far is we got a handle on the demand
in Buffalo. It's this distribution with this average value per week.
So let's now go back to the Simulation tab where we are going to evaluate, remember, our goal. Instead [? of ?] school, we are told Buffalo needs to be achieving 90% fuel rate. So to achieve 90% rate, what kind of safety
stock should they be carrying?
If you look at this spreadsheet, it's going to look similar to some of what we have done before. I have inputs here, which I'm going to answer in just a moment. We have decisions, in particular what safety stock to carry. We have
outputs that we're tracking here. And in particular, we'll have a simulation table here.
Just as some of the examples we have done in previous modules, it's going to be a weekly simulation where
we're keeping track of on-hand inventory, pipeline inventory, inventory position, order-up-to level, orders placed
in each week for the– for 52 weeks or so as the year progresses.
So let me zoom in a little bit and start entering information in the spreadsheet. The first thing is weekly demand
distribution. What is the weekly demand distribution in Buffalo? Well, I'll get that formula. I'll literally copy and
paste this formula that I got for weekly demand in Buffalo. So I'll put that here. Again, the idea here is that I don't know what the weekly demand in Buffalo is. But I know that it's a random input given by this formula.
Average weekly demand, I will set that equal to what I calculated for average weekly demand in Buffalo in the
next step. Lead time in weeks, the lead time, according to the case, was, I believe, three weeks. But let me make
sure to go back to my notes. It was actually four weeks. OK, so when ordering from the plant, each dealer's lead
time is 4 weeks. So let's put four weeks down for lead time.
Safety stock is our choice. We need to choose it so that Buffalo is achieving 90% fill rate. That's what's
happening in status quo, we are told. I don't know what safety stock will give me 90% fill rate just yet. For now, I'm just going to enter a [? measure of ?] 10 there, and then we'll see how to set it so that we make the fill rate
equal to 90%.
Now safety stock and order-up-to level, there is a 1 to 1 relationship between the two of them, if you remember
from our earlier discussions. In particular, if you tell me what safety stock you choose, I can tell you what order- up-to level it corresponds to. And the way we do that is you look at the lead time plus 1 period, times, you look at the average weekly demand, and to that, we add the safety stock to get the order-up-to level. This is the 1 to 1
relationship between safety stock and order-up-to level that we have seen before, for example, in module 3.
So I have my safety stock here. I have my resulting order-up-to level here. The outputs that we're going to track
are going to be mostly fill rate, what fill rate do we achieve. But before I can calculate the outputs, let me take
care of the simulation of what happens in Buffalo here.
Collepals.com Plagiarism Free Papers
Are you looking for custom essay writing service or even dissertation writing services? Just request for our write my paper service, and we'll match you with the best essay writer in your subject! With an exceptional team of professional academic experts in a wide range of subjects, we can guarantee you an unrivaled quality of custom-written papers.
Get ZERO PLAGIARISM, HUMAN WRITTEN ESSAYS
Why Hire Collepals.com writers to do your paper?
Quality- We are experienced and have access to ample research materials.
We write plagiarism Free Content
Confidential- We never share or sell your personal information to third parties.
Support-Chat with us today! We are always waiting to answer all your questions.
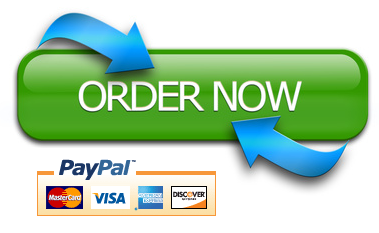