Qualitative Data Analysis Assignment Instructions DUE: by 10am
Qualitative Data Analysis Assignment Instructions
DUE: by 10am Saturday October 1, 2022 NO LATE WORK!!!!
Instructions
1. Choose a journal article that reports a qualitative study. Reflect upon how authority is established in the article.
· What conventions of social science writing are used?
· What do you learn about the researcher through the article?
· What do you learn about the researcher’s relationship to research participants?
2. Explore some aspect of your research topic by writing a short autoethnography in which you use dramatic recall and images from your own life to situate your research in the personal and the social. Reflect upon what you learned about your topic and your research participants.
Assignment Specifics:
· Student will write a 5-7 page paper.
· Citation of a journal article that reports a qualitative study.
· Citations from any of the Learn material from the assigned module/week.
· APA format.
· Abstract, keywords, Bible perspectives, conclusion, references
PLEASE CHECK ALL APA FORMATTING BEFORE SUBMISSION!!!!
Madden, R. (2017). Being ethnographic. SAGE Publications Ltd, https://dx.doi.org/10.4135/9781529716689 (Links to an external site.)
PLEASE CHECK ALL APA FORMATTING BEFORE SUBMISSION!!!!
Criteria Ratings Points
Identifies Main Issues/Problems
24 to >21.0 pts
Advanced
Identifies and demonstrates a sophisticated understanding of the main issues/problems in the study.
21 to >19.0 pts
Proficient
Identifies and demonstrates an accomplished understanding of most of the issues/problems.
19 to >0.0 pts
Developing
Identifies and demonstrates acceptable understanding of some of the issues/problems in the study.
0 pts
Not Present
24 pts
Analysis and Evaluation of Issues/Problems
23 to >21.0 pts
Advanced
Presents an insightful and thorough analysis of all identified issues/problem; includes all necessary calculations.
21 to >19.0 pts
Proficient
Presents a thorough analysis of most of the issues identified; missing some necessary calculations.
19 to >0.0 pts
Developing
Presents a superficial or incomplete analysis of some of the identified issues; omits necessary calculations.
0 pts
Not Present
23 pts
Recommendations 23 to >21.0 pts
Advanced
Supports diagnosis and opinions with strong arguments and well-documented evidence; presents a balanced and critical view; interpretation is both reasonable and objective.
21 to >19.0 pts
Proficient
Supports diagnosis and opinions with limited reasoning and evidence; presents a somewhat one-sided argument; demonstrated little engagement with ideas presented.
19 to >0.0 pts
Developing
Little or no action suggested and/or inappropriate solutions proposed to the issues in the study.
0 pts
Not Present
23 pts
APA, Spelling & Grammar
10 to >9.0 pts
Advanced
Limited to no APA, spelling or grammar mistakes.
9 to >7.0 pts
Proficient
Minimal APA, spelling and/or grammar mistakes.
7 to >0.0 pts
Developing
Noticeable APA, spelling and grammar mistakes.
0 pts
Not Present
10 pts
Page Length 10 to >9.0 pts
Advanced
5-7 double-spaced pages of content (not counting the title page or references).
9 to >7.0 pts
Proficient
1 page more or less than required length.
7 to >0.0 pts
Developing
More than 1 page more or less than required length.
0 pts
Not Present
10 pts
Qualitative Data Analysis Grading Rubric | CJUS750_B02_202240
Criteria Ratings Points
Sources 10 to >9.0 pts
Advanced
Citation of a journal article that reports a qualitative study. All web sites utilized are authoritative.
9 to >7.0 pts
Proficient
Citation of a journal article that reports a qualitative study. Most web sites utilized are authoritative.
7 to >0.0 pts
Developing
Citation of a journal article that reports a qualitative study. Not all web sites utilized are credible, and/or sources are not current.
0 pts
Not Present
10 pts
Total Points: 100
Qualitative Data Analysis Grading Rubric | CJUS750_B02_202240
,
O’Leary, Z. (2005). Researching real-world problems. Thousand Oaks, CA: SAGE.
Ch.11
Analysing and Interpreting Data
FROM RAW DATA TO MEANINGFUL UNDERSTANDING
It’s easy to fall into the trap of thinking the major hurdle in conducting real-world research is data collection. And yes, gathering credible data is certainly a challenge – but so is making sense of it. As George Eliot states, the key to meaning is ‘interpretation’.
Now attempting to interpret a mound of data can be intimidating. Just looking at it can bring on a nasty headache or a mild anxiety attack. So the question is, what is the best way to make a start? How can you begin to work through your data?
Well, if I were only allowed to give one piece of advice, it would be to engage in creative and inspired analysis using a methodical and organized approach. As described in Box 11.1, the best way to move from messy, complex and chaotic raw data … towards rich, meaningful and eloquent understandings is by working through your data in ways that are creative, yet managed within a logical and systematic framework.
Box 11.1 Balancing Creativity and Focus
Think outside the square … yet stay squarely on target
Be original, innovative, and imaginative … yet know where you want to go
Use your intuition … but be able to share the logic of that intuition
Be fluid and flexible … yet deliberate and methodical
Be inspired, imaginative and ingenious … yet realistic and practical
Easier said than done, I know. But if you break the process of analysis down into a number of defined tasks, it’s a challenge that can be conquered. For me, there are five tasks that need to be managed when conducting analysis:
Keeping your eye on the main game. This means not getting lost in a swarm of numbers and words in a way that causes you to lose a sense of what you’re trying to accomplish.
Managing, organizing, preparing and coding your data so that it’s ready for your intended mode(s) of analysis.
Engaging in the actual process of analysis. For quantified data, this will involve some level of statistical analysis, while working with words and images will require you to call on qualitative data analysis strategies.
Presenting data in ways that capture understandings, and being able to offer those understandings to others in the clearest possible fashion.
Drawing meaningful and logical conclusions that flow from your data and address key issues.
This chapter tackles each of these challenges in turn.
Keeping your eye on the main game
While the thought of getting into your data can be daunting, once you take the plunge it’s actually quite easy to get lost in the process. Now this is great if ‘getting lost’ means you are engaged and immersed and really getting a handle on what’s going on. But getting lost can also mean getting lost in the tasks, that is, handing control to analysis programs, and losing touch with the main game. You need to remember that while computer programs might be able to do the ‘tasks’, it is the researcher who needs to work strategically, creatively and intuitively to get a ‘feel’ for the data; to cycle between data and existing theory; and to follow the hunches that can lead to sometimes unexpected, yet significant findings.
FIGURE 11.1 THE PROCESS OF ANALYSIS
Have a look at Figure 11.1. It’s based on a model I developed a while ago that attempts to capture the full ‘process’ of analysis; a process that is certainly more complex and comprehensive than simply plugging numbers or words into a computer. In fact, real-world analysis involves staying as close to your data as possible – from initial collection right through to drawing final conclusions. And as you move towards these conclusions, it’s essential that you keep your eye on the game in a way that sees you consistently moving between your data and … your research questions, aims and objectives, theoretical underpinnings and methodological constraints. Remember, even the most sophisticated analysis is worthless if you’re struggling to grasp the implications of your findings to your overall project.
Rather than relinquish control of your data to ‘methods’ and ‘tools’, thoughtful analysis should see you persistently interrogating your data, as well as the findings that emerge from that data. In fact, as highlighted in Box 11.2, keeping your eye on the game means asking a number of questions throughout the process of analysis.
Box 11.2 Questions for Keeping the Bigger Picture in Mind
Questions related to your own expectations
What do I expect to find i.e. will my hypothesis bear out?
What don’t I expect to find, and how can I look for it?
Can my findings be interpreted in alternative ways? What are the implications?
Questions related to research question, aims and objectives
How should I treat my data in order to best address my research questions?
How do my findings relate to my research questions, aims and objectives?
Questions related to theory
Are my findings confirming my theories? How? Why? Why not?
Does my theory inform/help to explain my findings? In what ways?
Can my unexpected findings link with alternative theories?
Questions related to methods
Have my methods of data collection and/or analysis coloured my results. If so, in what ways?
How might my methodological shortcomings be affecting my findings?
Managing the data
Data can build pretty quickly, and you might be surprised by the amount of data you have managed to collect. For some, this will mean coded notebooks, labelled folders, sorted questionnaires, transcribed interviews, etc. But for the less pedantic, it might mean scraps of paper, jotted notes, an assortment of cuttings and bulging files. No matter what the case, the task is to build or create a ‘data set’ that can be managed and utilized throughout the process of analysis.
Now this is true whether you are working with: (a) data you’ve decided to quantify; (b) data you’ve captured and preserved in a qualitative form; (c) a combination of the above (there can be real appeal in combining the power of words with the authority of numbers). Regardless of approach, the goal is the same – a rigorous and systematic approach to data management that can lead to credible findings. Box 11.3 runs through six steps I believe are essential for effectively managing your data.
Box 11.3 Data Management
Step 1 Familiarize yourself with appropriate software
This involves accessing programs and arranging necessary training. Most universities (and some workplaces) have licences that allow students certain software access, and many universities provide relevant short courses. Programs themselves generally contain comprehensive tutorials complete with mock data sets.
Quantitative analysis will demand the use of a data management/statistics program, but there is some debate as to the necessity of specialist programs for qualitative data analysis. This debate is taken up later in the chapter, but the advice here is that it’s certainly worth becoming familiar with the tools available.
Quantitative programs Qualitative programs
SPSS – sophisticated and user-friendly (www.spss.com)
SAS – often an institutional standard, but many feel it is not as user-friendly as SPSS (www.sas.com)
Minitab – more introductory, good for learners/small data sets (www.minitab.com)
Excel – while not a dedicated stats program it can handle the basics and is readily available on most PCs (Microsoft Office product)
Absolutely essential: here is an up-to-date word processing package
Specialist packages include:
NU*DIST, NVIVO, MAXqda, The Ethnograph – used for indexing, searching and theorizing
ATLAS.ti – can be used for images as well as words
CONCORDANCE, HAMLET, DICTION – popular for content analysis (all above available: www.textanalysis.info)
CLAN-CA popular for conversation analysis (http://childes.psy.cmu.edu)
Step 2 Log in your data
Data can come from a number of sources at various stages throughout the research process, so it’s well worth keeping a record of your data as it’s collected. Keep in mind that original data should be kept for a reasonable period of time; researchers need to be able to trace results back to original sources.
Step 3 Organize your data
This involves grouping like sources, making any necessary copies and conducting an initial cull of any notes, observations, etc. not relevant to the analysis.
Step 4 Screen your data for any potential problems
This includes a preliminary check to see if your data is legible and complete. If done early, you can uncover potential problems not picked up in your pilot/trial, and make improvements to your data collection protocols.
Step 5 Enter the data
This involves systematically entering your data into a database or analysis program, as well as creating codebooks, which can be electronically based, that describe your data and keep track of how it can be accessed.
Quantitative data Qualitative data
Codebooks often include: the respondent or group; the variable name and description; unit of measurement; date collected; any relevant notes Codebooks often include: respondents; themes; data collection procedures; collection dates; commonly used shorthand; and any other notes relevant to the study
Data entry: data can be entered as it is collected or after it has all come in. Analysis does not take place until after data entry is complete. Figure 11.2 depicts an SPSS data entry screen Data entry: whether using a general word processing program or specialist software, data is generally transcribed in an electronic form and is worked through as it is received. Analysis tends to be ongoing and often begins before all the data has been collected/entered
FIGURE 11.2 DATA ENTRY SCREEN FOR SPSS
Step 6 Clean the data
This involves combing through the data to make sure any entry errors are found, and that the data set looks in order.
Quantitative data
When entering quantified data it’s easy to make mistakes – particularly if you’re moving fast, i.e. typos. It’s essential that you go through your data to make sure it’s as accurate as possible
Qualitative data
Because qualitative data is generally handled as it’s collected, there is often a chance to refine processes as you go. In this way your data can be as ‘ready’ as possible for analysis
STATISTICS – THE KISS (KEEP IT SIMPLE AND SENSIBLE) APPROACH
‘Doctors say that Nordberg has a 50/50 chance of living, though there’s only a 10 percent chance of that.’
– Naked Gun
It wasn’t long ago that ‘doing’ statistics meant working with formulae, but personally, I don’t believe in the need for all real-world researchers to master formulae. Doing statistics in the twenty-first century is more about your ability to use statistical software than your ability to calculate means, modes, medians and standard deviations – and look up p-values in the back of a book. To say otherwise is to suggest that you can’t ride a bike unless you know how to build one. What you really need to do is to learn how to ride, or in this case learn how to run a stats program.
Okay, I admit these programs do demand a basic understanding of the language and logic of statistics. And this means you will need to get your head around (1) the nature of variables; (2) the role and function of both descriptive and inferential statistics; (3) appropriate use of statistical tests; and (4) effective data presentation. But if you can do this, effective statistical analysis is well within your grasp.
Now before I jump in and talk about the above a bit more, I think it’s important to stress that …
Very few students can get their heads around statistics without getting into some data.
While this chapter will familiarize you with the basic language and logic of statistics, it really is best if your reading is done in conjunction with some hands-on practice (even if this is simply playing with the mock data sets provided in stats programs). For this type of knowledge ‘to stick’, it needs to be applied.
Variables
Understanding the nature of variables is essential to statistical analysis. Different data types demand discrete treatment. Using the appropriate statistical measures to both describe your data and to infer meaning from your data requires that you clearly understand your variables in relation to both cause and effect and measurement scales.
Cause and effect
The first thing you need to understand about variables relates to cause and effect. In research-methods-speak, this means being able to clearly identify and distinguish your dependent and independent variables. Now while understanding the theoretical difference is not too tough, being able to readily identify each type comes with practice.
DEPENDENT VARIABLES These are the things you are trying to study or what you are trying to measure. For example, you might be interested in knowing what factors are related to high levels of stress, a strong income stream, or levels of achievement in secondary school – stress, income and achievement would all be dependent variables.
INDEPENDENT VARIABLES These are the things that might be causing an effect on the things you are trying to understand. For example, conditions of employment might be affecting stress levels; gender may have a role in determining income; while parental influence may impact on levels of achievement. The independent variables here are employment conditions, gender and parental influence.
One way of identifying dependent and independent variables is simply to ask what depends on what. Stress depends on work conditions or income depends on gender. As I like to tell my students, it doesn’t make sense to say gender depends on income unless you happen to be saving for a sex-change operation!
Measurement scales
Measurement scales refer to the nature of the differences you are trying to capture in relation to a particular variable (examples below). As summed up in Table 11.1, there are four basic measurement scales that become respectively more precise: nominal, ordinal, interval and ratio. The precision of each type is directly related to the statistical tests that can be performed on them. The more precise the measurement scale, the more sophisticated the statistical analysis you can do.
NOMINAL Numbers are arbitrarily assigned to represent categories. These numbers are simply a coding scheme and have no numerical significance (and therefore cannot be used to perform mathematical calculations). For example, in the case of gender you would use one number for female, say 1, and another for male, 2. In an example used later in this chapter, the variable ‘plans after graduation’ is also nominal with numerical values arbitrarily assigned as 1 = vocational/technical training, 2 = university, 3 = workforce, 4 = travel abroad, 5 = undecided and 6 = other. In nominal measurement, codes should not overlap (they should be mutually exclusive) and together should cover all possibilities (be collectively exhaustive). The main function of nominal data is to allow researchers to tally respondents in order to understand population distributions.
ORDINAL This scale rank orders categories in some meaningful way – there is an order to the coding. Magnitudes of difference, however, are not indicated. Take for example, socio-economic status (lower, middle, or upper class). Lower class may denote less status than the other two classes but the amount of the difference is not defined. Other examples include air travel (economy, business, first class), or items where respondents are asked to rank order selected choices (biggest environmental challenges facing developed countries). Likert-type scales, in which respondents are asked to select a response on a point scale (for example, ‘I enjoy going to work’: 1 = strongly disagree, 2 = disagree, 3 = neutral, 4 = agree, 5 = strongly agree), are ordinal since a precise difference in magnitude cannot be determined. Many researchers, however, treat Likert scales as interval because it allows them to perform more precise statistical tests. In most small-scale studies this is not generally viewed as problematic.
INTERVAL In addition to ordering the data, this scale uses equidistant units to measure difference. This scale does not, however, have an absolute zero. An example here is date – the year 2006 occurs 41 years after the year 1965, but time did not begin in AD1. IQ is also considered an interval scale even though there is some debate over the equidistant nature between points.
RATIO Not only is each point on a ratio scale equidistant, there is also an absolute zero. Examples of ratio data include age, height, distance and income. Because ratio data are ‘real’ numbers all basic mathematical operations can be performed.
Descriptive statistics
Descriptive statistics are used to describe the basic features of a data set and are key to summarizing variables. The goal is to present quantitative descriptions in a manageable and intelligible form. Descriptive statistics provide measures of central tendency, dispersion and distribution shape. Such measures vary by data type (nominal, ordinal, interval, ratio) and are standard calculations in statistical programs. In fact, when generating the example tables for this section, I used the statistics program SPSS. After entering my data, I generated my figures by going to ‘Analyze’ on the menu bar, clicking on ‘Descriptive Statistics’, clicking on ‘Frequencies’, and then defining the statistics and charts I required.
Measuring central tendency
One of the most basic questions you can ask of your data centres on central tendency. For example, what was the average score on a test? Do most people lean left or right on the issue of abortion? Or what do most people think is the main problem with our health care system? In statistics, there are three ways to measure central tendency (see Table 11.2): mean, median and mode – and the example questions above respectively relate to these three measures. Now while measures of central tendency can be calculated manually, all stats programs can automatically calculate these figures.
MEAN The mathematical average. To calculate the mean, you add the values for each case and then divide by the number of cases. Because the mean is a mathematical calculation, it is used to measure central tendency for interval and ratio data, and cannot be used for nominal or ordinal data where numbers are used as ‘codes’. For example, it makes no sense to average the 1s, 2s and 3s that might be assigned to Christians, Buddhists and Muslims.
MEDIAN The mid-point of a range. To find the median you simply arrange values in ascending (or descending) order and find the middle value. This measure is generally used in ordinal data, and has the advantage of negating the impact of extreme values. Of course, this can also be a limitation given that extreme values can be significant to a study.
MODE The most common value or values noted for a variable. Since nominal data is categorical and cannot be manipulated mathematically, it relies on mode as its measure of central tendency.
Measuring dispersion
While measures of central tendency are a standard and highly useful form of data description and simplification, they need to be complemented with information on response variability. For example, say you had a group of students with IQs of 100, 100, 95 and 105, and another group of students with IQs of 60, 140, 65 and 135, the central tendency, in this case the mean, of both groups would be 100. Dispersion around the mean, however, will require you to design curriculum and engage learning with each group quite differently. There are several ways to understand dispersion, which are appropriate for different variable types (see Table 11.3). As with central tendency, statistics programs will automatically generate these figures on request.
RANGE This is the simplest way to calculate dispersion, and is simply the highest minus the lowest value. For example, if your respondents ranged in age from 8 to 17, the range would be 9 years. While this measure is easy to calculate, it is dependent on extreme values alone, and ignores intermediate values.
QUARTILES This involves subdividing your range into four equal parts or ‘quartiles’ and is a commonly used measure of dispersion for ordinal data, or data whose central tendency is measured by a median. It allows researchers to compare the various quarters or present the inner 50% as a dispersion measure. This is known as the inner-quartile range.
VARIANCE This measure uses all values to calculate the spread around the mean, and is actually the ‘average squared deviation from the mean’. It needs to be calculated from interval and ratio data and gives a good indication of dispersion. It’s much more common, however, for researchers to use and present the square root of the variance which is known as the standard deviation.
STANDARD DEVIATION This is the square root of the variance, and is the basis of many commonly used statistical tests for interval and ratio data. As explained below, its power comes to the fore with data that sits under a normal curve.
Measuring the shape of the data
To fully understand a data set, central tendency and dispersion need to be considered in light of the shape of the data, or how the data is distributed. As shown in Figure 11.3, a normal curve is ‘bell-shaped’; the distribution of the data is symmetrical, with the mean, median and mode all converged at the highest point in the curve. If the distribution of the data is not symmetrical, it is considered skewed. In skewed data the mean, median and mode fall at different points.
Kurtosis characterizes how peaked or flat a distribution is compared to ‘normal’. Positive kurtosis indicates a relatively peaked distribution, while negative kurtosis indicates a flatter distribution.
The significance in understanding the shape of a distribution is in the statistical inferences that can be drawn. As shown in Figure 11.4, a normal distribution is subject to a particular set of rules regarding the significance of a standard deviation. Namely that:
68.2% of cases will fall within one standard deviation of the mean
95.4% of cases will fall within two standard deviations of the mean
99.6% of cases will fall within three standard deviations of the mean
So if we had a normal curve for the sample data relating to ‘age of participants’ (mean = 12.11, s.d. = 2.22 – see Boxes 11.2, 11.3), 68.2% of participants would fall between the ages of 9.89 and 14.33 (12.11–2.22 and 12.11+2.22).
These rules of the normal curve allow for the use of quite powerful statistical tests and are generally used with interval and ratio data (sometimes called parametric tests). For data that does not follow the assumptions of a normal curve (nominal and ordinal data), the researcher needs to call on non-parametric statistical tests in making inferences.
Table 11.4 shows the curve, skewness and kurtosis of our sample data set.
Inferential statistics
While the goal of descriptive statistics is to describe and summarize, the goal of inferential statistics is to draw conclusions that extend beyond immediate data. For example, inferential statistics can be used to estimate characteristics of a population from sample data, or to test various hypotheses about the relationship between different variables. Inferential statistics allow you to assess the probability that an observed difference is not just a fluke or chance finding. In other words, inferential statistics is about drawing conclusions that are statistically significant.
Statistical significance
Statistical significance refers to a measure, or ‘p-value’, which assesses the actual &#x
Collepals.com Plagiarism Free Papers
Are you looking for custom essay writing service or even dissertation writing services? Just request for our write my paper service, and we'll match you with the best essay writer in your subject! With an exceptional team of professional academic experts in a wide range of subjects, we can guarantee you an unrivaled quality of custom-written papers.
Get ZERO PLAGIARISM, HUMAN WRITTEN ESSAYS
Why Hire Collepals.com writers to do your paper?
Quality- We are experienced and have access to ample research materials.
We write plagiarism Free Content
Confidential- We never share or sell your personal information to third parties.
Support-Chat with us today! We are always waiting to answer all your questions.
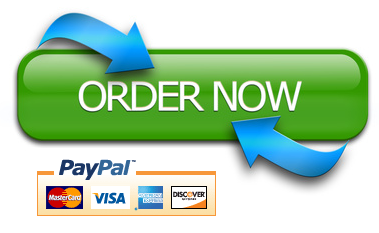