Sun coast remediation
Instructions
For this assignment, you will define the research objectives and develop the research questions and hypotheses to set the stage for the Sun Coast Remediation research project.
You will utilize this Unit II template to complete the Unit II assignment.
Be sure to include the following sections in your submission, and remember to use APA-formatted section headings and subheadings.
Research objectives: Refer to the Introduction and Statement of Problems sections provided in the Unit II Assignment Template to make explicit statements as to the objectives of this study. For example, “One objective of this research study is to determine the direction (either positive or negative) and the strength of relationships between ABC Company’s various consumer promotions and sales revenue. This objective is intended to determine which promotions may be more effective and strategically important.” Research objectives should be discussed for each problem identified.
Research questions and hypotheses: Provide research questions and null and alternative hypotheses that align with the research objectives and problems. For example:
RQ1: What is the relationship between Promotion 1 and sales revenue?
Ho1 (null hypothesis): There is no statistically significant correlation between Promotion 1 (independent variable) and sales revenue (dependent variable).
Ha1 (alternative hypothesis): There is a statistically significant correlation between Promotion 1 and sales revenue.
RQ2: What is the relationship between Promotion 2 and sales revenue?
Ho2: There is no statistically significant correlation between Promotion 2 and sales revenue.
Ha2: There is a statistically significant correlation between Promotion 2 and sales revenue.
RQ3: Are there differences in return on investment (ROI) between the various promotions?
Ho3: There are no statistically significant differences in ROI between Promotion 1 and Promotion 2.
Ha3: There are statistically significant differences in ROI between Promotion 1 and Promotion 2.
The title and reference pages do not count toward the page requirement for this assignment. This assignment should be a minimum of two pages in length, follow APA-style formatting and guidelines, and use references and citations as necessary.
Unit 2 Study Guide
Unit Lesson
This unit covers the topic of research questions and hypotheses. Research questions and hypotheses are closely related since they both function to describe, and ultimately solve, the management problem(s). The following will explain these foundational concepts of business research.
Research Questions
To solve the business problem(s), it is necessary to answer the research question(s). For example, consider a scenario where a U.S. company needs to make a large capital expenditure for a piece of manufacturing equipment. There are five models offered by five different vendors, and all might be suitable replacements for the obsolete equipment. The business problem is that they do not have enough information to determine which model is the best investment. Given the expensive price tag, the company wants to make the right decision to replace the obsolete equipment. The wrong decision would be quite costly.
The company’s research consultant is commissioned to do a comparison of the five models of equipment: Models A, B, C, D, and E. The consultant’s job is to collect and test data on the five models so that the client
RCH 5301, Research Methods 1
can make an evidence-based decision to reduce risk. There are several variabUleNsITtoxcSoTnUsiDdeYr GinUclIuDdEing production capacity, cost of operation, and error rate. The research question is this: Which model will provide
the most productivity? When this question is answered, the business problem is solved. Before the question is answered, however, it must be restated as a hypothesis and statistically tested.
Hypotheses
A hypothesis is an explicit statement about relationships or differences between variables or groups that is speculative, yet unsubstantiated (Cooper & Schindler, 2011). The consultant’s hypothesis, which is related to both the problem and research question, would be a prediction about the differences in productivity between Models A, B, C, D, and E. This would be a causal-comparative study. In this scenario, model type is the independent variable (also known as predictor variable), while production capacity, cost of operation, and error rate are the dependent variables (also known as outcome variables).
In this causal-comparative scenario, the company happens to be hypothesizing about differences between models since finding differences would help answer the research question. As mentioned previously, hypotheses may also speculate about relationships between variables. For example, assume the research question is this: Which model has the highest unit output relative to cost per unit? Since cost per unit would decrease as production increases, the relationship between these variables, or correlation, would allow the company to compare models and make a sound business decision based on the strengths of the correlations. Using a hypothesis about relationships, the company would ideally like to determine the model with the greatest negative correlation between output and unit costs. In other words, as production increases, unit costs decrease.
Stating hypotheses in inferential statistics is done in a specific manner. It is customary (and sometimes referred to as traditional) to state both a null hypothesis and an alternative hypothesis. A null hypothesis is a statement where no statistically significant relationship or statistically significant difference occurs between the variables or groups under study (Creswell & Creswell, 2018).
Null hypothesis: There are no statistically significant differences in production capacity, cost of operation, and error rate between Models A, B, C, D, and E.
Alternative hypothesis: There are statistically significant differences in production capacity, cost of operation, and error rate between Models A, B, C, D, and E.
There are several unique things to notice about these hypotheses. The first thing is that the alternative hypothesis is the opposite of the null hypothesis. The null hypothesis should be viewed as the status quo. The status quo in the scenario is that no differences exist between models. The purpose of conducting the research is to determine if statistical differences do indeed exist. This is done by statistically analyzing the data to determine if the status quo, or the null hypothesis, can be rejected (Swanson & Holton, 2005). It is important to note that when engaging in hypothesis testing, the researcher can never make the statement that the hypothesis is proven or disproven. Research results simply allow the researcher to either accept or reject the null hypotheses. If research provided proof, there would be no reason to continue to conduct scientific inquiry. For example, the atom was once considered the smallest component of matter. Now, through science and hypothesis testing, atomic theory has moved to string theory. If researchers accepted the hypothesis that the atom was the smallest unit of matter as proof, we would know nothing about strings, vibrations, and their relationship to matter. Similarly, hypotheses about strings are not proven but accepted or rejected.
The researcher should always speak in terms of either rejecting or accepting the null and alternative hypotheses. More specifically, it should be explicitly stated that, based on the statistical results, either the null hypothesis is rejected and the alternative hypothesis accepted, or the null hypothesis is accepted and the alternative hypothesis is rejected. It is an impossibility to either reject both the null and alternative hypotheses or accept both the null and alternative hypotheses since they are mutually exclusive events.
Statistical Significance
Notice in the null and alternative hypotheses stated above the use of the phrase statistically significant. Statistical procedures test for significant differences or significant relationships between variables. Before making decisions about Models A, B, C, D, and E, the researcher must have a certain level of confidence in
Title
RCH 5301, Research Methods 2
the results from the statistical procedure. The information needed to interpret sUtaNtIiTstixcaSlTreUsDuYltsGinUcIlDudEes the significance level (also known as alpha), a calculated statistical value, and the calculated statistic’s
corresponding p value (probability value). The alpha is a benchmark that is set by the researcher. In business and social science research, the alpha is customarily set at .05 (5%). An alpha of .05 is the normally accepted cut-off for rejecting the null hypothesis and accepting the alternative hypothesis (Field, 2005; Norusis, 2008).
After the statistical procedure has been completed, the calculated statistic’s p value is then compared to the alpha. If the p value is less than .05, the null hypothesis is rejected, and the alternative hypothesis is accepted. If the p value is greater than .05, the null hypothesis is accepted, and the alternative hypothesis is rejected. Having a p value <.05 is the same as saying that there is less than a 5% probability that the results were due purely to chance (Field, 2005). Conversely, the researcher can be at least 95% confident that the test results represent the true effect being measured.
Having 95% confidence still leaves a small chance of error. Even if the test results are statistically significant at a p value <.05, suggesting a greater than 95% confidence that the calculated value represents the true effect, there is still a small possibility that our results could have been due to random chance. If these small possibilities occur, they are known as Type I and Type II errors. A Type I error is when the null hypothesis is rejected when, in fact, it should have been accepted; this is a false positive. A Type II error is when the null hypothesis is accepted when, in fact, it should have been rejected; this is a false negative.
After testing the above hypotheses for statistically significant differences in production capacity, cost of operation, and error rate between equipment Models A, B, C, D, and E, the results can be interpreted and recommendations made. For example, if the statistical results indicate that Model A has statistically higher production capacity, lower cost of operation, and lower error rates versus Models B, C, D, and E, an evidence-based decision could be made to purchase Model A.
Hypothesis Testing Steps
The basic steps for completing a test of statistical significance are listed below (Cooper & Schindler, 2011).
Title
1. State the null and alternative hypotheses.
2. Choose a statistical test (e.g., correlation, regression, t test, ANOVA, etc.)
3. Select the desired alpha (significance level). Before running the statistical test, it must be decided how much risk is tolerated. In business research, a .05 significance level, or level of risk, is customary.
4. Calculate the statistic and associated p value. This is commonly done via statistical software.
5. Interpret the test. If the p value for the calculated statistic is less than .05, the null hypothesis is rejected, and the alternative hypothesis is accepted. If the p value is greater than .05, the null hypothesis is accepted, and the alternative hypothesis is rejected.
RCH 5301, Research Methods 3
Characteristics of Good Hypotheses UNIT x STUDY GUIDE Title
Good hypotheses are declarative, speculative, brief, and testable (Cooper & Schindler, 2011). Hypotheses examples: Both the null and alternative hypotheses should be stated.
Example one: Statistical test looking for a difference (causal-comparative) between the same x variable in different groups.
Ho1: There is no statistically significant difference in x between Group A and Group B.
Ha1: There is a statistically significant difference in x between Group A and Group B. Example two: Statistical test looking for a relationship (correlation) between x and y variables. Ho2: There is no statistically significant relationship between x and y.
Ha2: There is a statistically significant relationship between x and y.
As shown above, the null and alternative hypotheses should both be stated when formulating hypotheses. Similarly, both the null and alternative hypotheses should be stated when interpreting test results. It should be clear which is accepted and which is rejected when evaluating the statistical value and its corresponding p value.
References
Cooper, D. R., & Schindler, P. S. (2011). Business research methods (11th ed.). McGraw-Hill/Irwin. Creswell, J. W., & Creswell, J. D. (2018). Research design: Qualitative, quantitative, and mixed methods
approaches (5th ed.). SAGE. https://online.vitalsource.com/#/books/9781506386690
Field, A. (2005). Discovering stats using SPSS (2nd ed.). SAGE.
Norusis, M. J. (2008). SPSS 16.0 guide to data analysis (2nd ed.). Prentice Hall.
Swanson, R. A., & Holton, E. F., III (Eds.). (2005). Research in organizations: Foundations and methods of inquiry. Berrett-Koehler.
Collepals.com Plagiarism Free Papers
Are you looking for custom essay writing service or even dissertation writing services? Just request for our write my paper service, and we'll match you with the best essay writer in your subject! With an exceptional team of professional academic experts in a wide range of subjects, we can guarantee you an unrivaled quality of custom-written papers.
Get ZERO PLAGIARISM, HUMAN WRITTEN ESSAYS
Why Hire Collepals.com writers to do your paper?
Quality- We are experienced and have access to ample research materials.
We write plagiarism Free Content
Confidential- We never share or sell your personal information to third parties.
Support-Chat with us today! We are always waiting to answer all your questions.
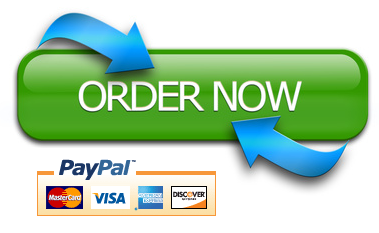