Descriptive Statistics Analysis
Instructions
Descriptive Statistics Analysis
Describe the Sun Coast data using the descriptive statistics tools discussed in the unit lesson. Establish whether assumptions are met to use parametric statistical procedures. Repeat the tasks below for each tab in the Sun Coast research study data set . Utilize the Unit IV Scholarly Activity template .
You will utilize the Microsoft Excel ToolPak. The links to the ToolPak are in the Excel ToolPak Links document .
Here are some of the items you will cover.
Produce a frequency distribution table and histogram.
Generate descriptive statistics table, including measures of central tendency (mean, median, and mode), kurtosis, and skewness.
Describe the dependent variable measurement scale as nominal, ordinal, interval, or ratio.
Analyze, evaluate, and discuss the above descriptive statistics in relation to assumptions required for parametric testing. Confirm whether the assumptions are met or are not met.
The title and reference pages do not count toward the page requirement for this assignment. This assignment should be no less than five pages in length, follow APA-style formatting and guidelines, and use references and citations as necessary.
Unit Lesson
Data Analysis: Descriptive Statistics
The course is now entering the data analysis stage of research design. This is where the methodological fork in the road goes decisively down the quantitative path. The first topic of discussion under data analysis will be what is referred to as descriptive statistics. As the name suggests, the researcher describes the data that are collected. During this stage, the data are described both visually and statistically. Data may be visually displayed to reveal distribution of data, trends, anomalies, outliers, etc. Visual displays of data may take the form of graphs, histograms, tables, plots, and other diagrams. This stage is done before any statistical procedures are used to test the research hypotheses. This begs the question of why the researcher should not simply jump in and immediately start testing their hypotheses using statistical analysis. The following explains the importance of descriptive statistics to test data to ensure assumptions are met before using a parametric test.
RCH 5301, Research Methods 2
Assumptions: The Importance of Describing Data UNIT x STUDY GUIDE Title
There are various benefits of describing the data. One of the most important benefits is to determine if the data meet the assumptions that are required for the use of parametric statistical procedures. Parametric procedures include, but are not limited to, correlation, regression, t test, and ANOVA. Parametric tests have different assumptions that must be met depending on which test is being considered, but most parametric tests require that the assumption of normality be met. Normality refers to a normal distribution of data which, when graphed as
frequencies, resembles a
bell shape (as in the image
to the right). Other common assumptions that must be
met, depending on the
statistical procedure used, 60 include sample size, levels- of-measurement,
homogeneity of variance, independence, absence of
outliers, linearity, etc. (Field,
2005). It is critical that the researcher understands the 30 assumptions for any
parametric statistical
procedure being considered
to determine if they are met
before employing the 10 procedure in a research
study. An Internet search
for any parametric test will
quickly return results that
list required assumptions.
Bell Curve
80
70
50 40
20
10 20 30 40 50 60 70 80 90 100
Normal distribution graph with a bell curve
If the assumptions are not
met, parametric statistical procedures cannot be used. To use them would result in invalid results. Fortunately, there are corresponding non-parametric tests that can be used when the data do not meet assumptions for parametric tests. Non-parametric tests also have assumptions that must be met, but they are fewer and less rigid. An example of a parametric procedure for correlation would be Pearson’s correlation coefficient (Pearson’s r), while a corresponding non-parametric test for correlation would be Spearman’s rank correlation coefficient (Spearman’s rho). An example of a causal-comparative parametric procedure would be ANOVA, while a corresponding non-parametric causal-comparative test would be Kruskal-Wallis.
Since non-parametric tests do not require that as many assumptions are met, some students wonder why non-parametric tests are not always used. The reason is that parametric tests are superior to and more powerful than non-parametric tests and should be used if the assumptions are met. A parametric test is more likely to find a true effect when one exists, therefore rejecting the null hypothesis, than a non-parametric test (Norusis, 2008). In other words, a parametric test is less likely to commit a Type II error. Norusis (2008) recommends that researchers conduct both parametric and non-parametric tests if they are unsure as to which is most appropriate to use. If the test results are the same, there is nothing more to worry about. If the test results are statistically significant for the parametric test, and non-significant for the non-parametric test, the researcher should take a closer look at whether the assumptions were met or not.
Assumption of Normality
Assumptions are evaluated both visually and statistically. As mentioned previously, a normal distribution of data is the most commonly required assumption for parametric statistical tests. The following will explain how the assumption of normality can be described and tested.
A normal distribution of data exhibits the characteristics of a bell-shaped curve, as shown below. In a perfect normal curve, the frequency distribution is symmetrical about the center; the mean, median, and mode are all
RCH 5301, Research Methods 3
equal; and the tails of the curve approach but do not touch the x-axis (Salkind,U2N0I0T9x).STThUesDeYaGreUaIDllE preliminary indicators that a curve may represent a normal distribution, but there are additional factors to
consider.
Distribution curves can be short and wide, tall and thin, and anywhere in between. As shown below, each of the colored bell-shaped curves has a mean
(μ) of zero. Their standard deviations (σ),
however, or the measure of how widely the
data disperses around the mean, are different for each curve. The orange curve has a relatively small standard deviation because the data is closely clustered around the mean. The red curve has a relatively large standard deviation because the data is loosely clustered around the mean.
Distribution curves
Title
Kurtosis describes the tallness of the curves.
A platykurtic curve is short and squatty (think
plateau), which, as shown at the right in the
red curve, represents a relatively greater
number of scores in the tails of the curves. A
leptokurtic curve is tall and thin (think leapt
for the sky), which, as shown in the orange
curve, represents a data distribution of
relatively fewer number of scores in the tails (Field, 2005). Platykurtic and leptokurtic curves can challenge the assumption of normality, even when the curve is bell-shaped.
The data may also be asymmetrical with the data more heavily distributed to one side of the curve or the other. When the data distribution curve is asymmetrical, it is referred to as skewness. Below are examples of negative skewness and positive skewness. Like platykurtic and leptokurtic curves, those exhibiting skewness also threaten the assumption of normality.
The assumption of normality can be evaluated visually by describing the frequency of responses in a data set. The frequency table below shows the results of a 120-point safety test administered to 500 employees. For example, two employees scored in the test range of 50–54, 90 employees scored in the range of 85–89, and three employees scored in the range of 110–114.
Left-skewed and right-skewed graphs (Sundberg, 2014)
RCH 5301, Research Methods 4
When the frequency data is plotted in a histogram, the curve of the data can be observed. To create a histogram, the data values (test score ranges) from the data set are plotted on the x-axis, and the frequency of the values are plotted on the y-axis. So, using the same example from the discussion of the frequency table, it can be seen in the histogram that two employees scored in the test range of 50–54, 90 employees scored in the range of 85–89, and three employees scored in the range of 110–114.
By observing the histogram below, it appears the data are approximately normally distributed, and there are no visible outliers. While there is no skewness observed, the kurtosis favors a leptokurtic curve. Skewness and kurtosis can be confirmed by generating descriptive statistics, which is a routine function in statistical packages, including Excel Data Analysis Toolpak. There is a lot of debate regarding acceptable levels of skewness and kurtosis among researchers. George and Mallery (2010) suggest skewness and Kurtosis scores between -2 and +2 as satisfactory results to accept normal distribution. All researchers agree that the closer skewness and kurtosis are to 0, the better. The more kurtosis and skewness deviate from 0, the greater the chances that the data is not normally distributed (Field, 2005). As shown in the descriptive statistics table, both skewness and kurtosis are both relatively close to 0.
UNIT x STUDY GUIDE Title
It should also be noted that the mean, median, and mode are similar in the descriptive data table below. As noted above, the mean, median, and mode are identical in a perfect distribution. The data presented here would suggest that it is approximately a normal distribution of data.
RCH 5301, Research Methods 5
Descriptive Statistics
UNIT x STUDY GUIDE Title
Mean 80.546 Standard Error 0.446621439 Median 81 Mode 75 Standard Deviation 9.986758969 Sample Variance 99.73535471
Range 64 Minimum 53 Maximum 117 Sum 40273 Count 500 Largest(1) 117 Smallest(1) 53
The frequency distribution should also be observed for outliers. Outliers are extreme scores far away from the mean in the left or right tails of the curve. Outliers can bias the mean due to their extreme scores. There are different recommendations for how to treat outliers, such as removing the outlier from the data set, but the ramifications should be understood before taking any such action. This is an example where consulting the literature is strongly recommended.
Finally, normality can be tested statistically. Several tests can be used to objectively test for normality including Kolmogorov-Smirnov, Shapiro-Wilk, chi-square, Jarque-Bera, Anderson-Darling, and others. Each test has advantages and disadvantages. Once again, this is where the researcher is well-served to consult the literature to determine the most appropriate test for his or her project.
The Kolmogorov-Smirnov (KS) test is often used to test for normality. KS compares the frequency distribution of the sample data set to a model of normally distributed data with the same mean and distribution as the sample data. The KS test is performed to test a null and alternative hypothesis, like any other statistical test. The following are the hypotheses.
Ho1: There is no statistically significant difference in normality between the sample data and model data. Ha1: There is a statistically significant difference in normality between the sample data and model data.
If the results are statistically significant at a p level < .05, the null hypothesis is rejected, and the alternative hypothesis is accepted that there is a statistically significant difference in normality between the sample data and model data. Therefore, we would conclude that the assumption of normality is not met, and a non- parametric test would be required to test our data.
If the results are not statistically significant at a p level > .05, the null hypothesis is accepted (and the alternative rejected) that there is no statistically significant difference in normality between the sample data and model data. Therefore, we would conclude that the assumption of normality is met, and a parametric test would be acceptable to test our data.
It is important to note that the above steps for evaluating the assumption of normality require a holistic view. No single description of the data is sufficient to make a decision about normality. For example, the KS test is sensitive to small changes in normality for large sample sizes. The result is that it can be prone to Type I errors. Therefore, the researcher should consider all the available information, both visual inspection and statistical analysis, before making a decision about normality (Field, 2005). If, after following the steps above,
0.095314585
0.065078019
Kurtosis
Skewness
RCH 5301, Research Methods 6
the assumption of normality does not appear to be met, non-parametric statistUicNalITprxocSeTdUuDreYs GshUoIuDldE be
considered in lieu of parametric tests.
Assumptions Other Than Normality
Title
There are two additional assumptions that should be met for any statistical test. They are measurement scales and measures of central tendency.
Measurement scales: Statistical procedures used to test hypotheses have unique assumptions about the scales on which the data are measured. Data are measured on nominal, ordinal, interval, or ratio scales. It is important to determine the assumption of measurement scales for any statistical procedure being considered to test the data. For example, an assumption of Pearson’s r is that data be measured at the interval or ratio level. Pearson’s r could not be used to analyze ordinal data. The non-parametric test, Spearman’s rho, would be required to analyze ordinal data for correlation.
Rules for Measurement Scales
Nominal: Nominal data can be classified but not ordered and have no meaningful distance between variables or unique origin (true zero). This is also referred to as categorical data. Examples include names or categories, like gender and marital status. Examples of statistical procedures that use nominal data include chi-square (Cooper & Schindler, 2014).
Ordinal: Ordinal data can be classified and ordered but have no meaningful distance between data values or unique origin (true zero). Examples include surveys with responses ranked on a five-point Likert scale, such as strongly agree to strongly disagree. Examples of statistical procedures that use ordinal data include Spearman’s rho, Mann-Whitney test, Wilcoxon test, Kruskal-Wallis test, and Friedman test (Cooper & Schindler, 2014).
Interval: Interval data can be classified and ordered and have meaningful distance between data values but no unique origin (true zero). A classic example of an interval level of measurement is temperature measured in degrees. The data is ordered, there are differences between measures, but there is no true zero. Since there is no true zero, it would be improper to say 40 degrees is twice as cold as 20 degrees. Examples of statistical procedures that use interval data include Pearson’s r, regression analysis, t test, and ANOVA (Cooper & Schindler, 2014).
Ratio: Ratio data can be classified and ordered, have meaningful distance between data values, and have unique origin (true zero). Examples include age in years and income in dollars. Examples of statistical procedures that use ratio data include Pearson’s r, regression analysis, t test, and ANOVA (Cooper & Schindler, 2014). It should be noted that parametric tests are used to analyze data measure at the interval and ratio levels but cannot be used to analyze data measured at the nominal and ordinal levels.
Measures of central tendency: It may have become evident by now, from the use of the histogram and the discussion of normality, that there is interest in how the data points are dispersed around the mid-point of the curve. This is called central tendency and is the foundation for statistical analysis using linear models. In short, our statistical procedures evaluate how much our data vary from that midpoint when a straight line is fit to the data (Field, 2005). The important takeaway is that the central tendency of that midpoint can be measured in three different ways: a) mean, b) median, and c) mode. As was seen in the descriptive statistics output above, mean, median, and mode are usually included in descriptive statistics generated by software. As was the case with normality and levels of measurement, it is important to determine the assumption of central tendency for any statistical procedure being considered to test the data.
Mean: The arithmetic mean is the most commonly used measure of central tendency. It is calculated by adding the data scores and dividing by the number of cases. The mean is the measure of central tendency used with interval and ratio data and is used for statistical procedures like correlation, regression analysis, t test, and ANOVA (Salkind, 2009).
Median: The median is the score among the distribution of data, when ordered from highest to lowest, where half of the data points occur above the median and half of the data points occur below the median. In the data
RCH 5301, Research Methods 7
set 1, 3, 5, 7, and 9, the median would be 5 since half of the values occur aboUveNaITndx hSaTlfUbDeYloGw.UTIDheE median
is the measure of central tendency used with ordinal data (Salkind, 2009).
Mode: The mode is the data value that occurs most frequently in the data set, regardless of order. In a data set of 5, 5, 5, 3, 3, 9, 9, 9, 9, 1, 1, 1, 7, 7, 7, 7, 7, the mode would be 7 because it is the value that occurs most frequently in the data set. The mode is the measure of central tendency used with nominal levels of measurement (Salkind, 2009).
In Closing—A Word About Validity and Reliability
Although some of the most important and common assumptions of statistical testing have been discussed in this lesson, there are still more. This may seem like a very taxing and laborious process to partake in before even getting to the point of testing the research hypotheses. It is absolutely critical that researchers ensure assumptions are met to have certainty that their results reflect the integrity of validity and reliability.
To be able to make confident decisions using research, the statistical results must be both valid and reliable. Validity means that the statistical procedure measures what was intended to be measured. As was discussed about normality, if a parametric statistical procedure is used for a data set that lacks a normal distribution of data, the results will be invalid.
Reliability refers to repeatability. If a second research study was conducted by replicating the conditions of the original research study (e.g., sampling, data collection, levels of measurement, statistical test, etc.), the results should be similar if the original research results were reliable.
It should also be noted that research results can be reliable but not valid. It is conceivable that a research study could be replicated multiple times and reliably generate the same invalid results each time. A classic example is the broken bathroom scale. Assume a person’s actual weight is 150 pounds. Each morning, for a week they step on the bathroom scale and the reading is 145 pounds. The measurement is invalid because, due to calibration problems, the measurement is incorrect. The test, however, is reliable because the same result was replicated each day. For research results to have integrity, they must be both valid and reliable.
Collepals.com Plagiarism Free Papers
Are you looking for custom essay writing service or even dissertation writing services? Just request for our write my paper service, and we'll match you with the best essay writer in your subject! With an exceptional team of professional academic experts in a wide range of subjects, we can guarantee you an unrivaled quality of custom-written papers.
Get ZERO PLAGIARISM, HUMAN WRITTEN ESSAYS
Why Hire Collepals.com writers to do your paper?
Quality- We are experienced and have access to ample research materials.
We write plagiarism Free Content
Confidential- We never share or sell your personal information to third parties.
Support-Chat with us today! We are always waiting to answer all your questions.
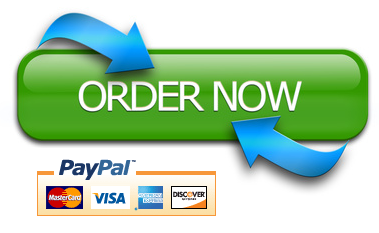