Describe the various ways business intelligence, big data and analytics improves the utilization of information within an organization. Suggest a company (Netflix.com) th
Describe the various ways business intelligence, big data and analytics improves the utilization of information within an organization. Suggest a company (Netflix.com) that can use these as a key element to improve its competitive position in the marketplace.
- Paper should relate to some of the related concepts as specified in chapter 9, and chapter extensions 12, 13, 14. Besides, appropriate concepts outlined in other chapters can also be included to substantiate the paper.
- Make sure your paper focuses on the issues raised by the assignment topic. Section your paper as suggested in the template below so that it is easy to understand and follow. Make each template requirement as section title in the paper. The template structure is as follows:
- Describe business intelligence (BI), big data, and data analytics in general.
- Describe how (BI), big data, and data analytics makes a company competitive in general.
- Describe Netflix and its working/competitive environment. Anytime a company is described, make sure the company description provides details like its products, its history, etc. even if the company is widely known.
- Describe how (BI), big data, and data analytics features are enabling Netflix to enhance its competitiveness in the marketplace. Provide instances of such features that are properly referenced.
4 pages max, include reference
1
Business Intelligence Systems
Chapter 9
9-2
Study Questions
C o p y r i g h t © 2 0 1 7 P e a r s o n E d u c a t i o n , I n c .
Q1: How do organizations use business intelligence (BI) systems?
Q2: What are the three primary activities in the BI process?
Q3: How do organizations use data warehouses and data marts to acquire data?
Q4: What are three techniques for processing BI Data?
Q5: What are the alternatives for publishing BI?
How does the knowledge in this chapter help you?
2
9-3
Q1: How Do Organizations Use Business Intelligence (BI) Systems?
C o p y r i g h t © 2 0 1 7 P e a r s o n E d u c a t i o n , I n c .
Structure of Business Intelligence System
9-4
Sample of Uses of Business Intelligence
C o p y r i g h t © 2 0 1 7 P e a r s o n E d u c a t i o n , I n c .
3
9-5
What Are Typical Uses for BI?
C o p y r i g h t © 2 0 1 7 P e a r s o n E d u c a t i o n , I n c .
• Identifying changes in purchasing patterns – Important life events change what customers buy
• BI for Entertainment – Netflix has data on watching, listening, and rental habits – Classify customers by viewing patterns
• Just-in-Time Medical Reporting – Real-time injection notification services to doctors during
exams
9-6
Q2: What Are the Three Primary Activities in the BI Process?
C o p y r i g h t © 2 0 1 7 P e a r s o n E d u c a t i o n , I n c .
4
9-7
Using Business Intelligence to Find Candidate Parts at Falcon Security
C o p y r i g h t © 2 0 1 7 P e a r s o n E d u c a t i o n , I n c .
• Candidate parts – Provided by vendors who agree to make part design files
available for sale – Purchased by larger customers – Frequently ordered parts – Ordered in small quantities – Simple in design (easier to 3D print)
• Part weight and price surrogates for simplicity
9-8
Acquire Data: Extracted Order Data
C o p y r i g h t © 2 0 1 7 P e a r s o n E d u c a t i o n , I n c .
• Query Sales (CustomerName, Contact, Title, Bill Year, Number Orders, Units, Revenue, Source, PartNumber) Part (PartNumber, Shipping Weight, Vendor)
5
9-9
Sample Extracted Data: Part Table
C o p y r i g h t © 2 0 1 7 P e a r s o n E d u c a t i o n , I n c .
9-10
Joining Order Extract and Filtered Parts Tables
C o p y r i g h t © 2 0 1 7 P e a r s o n E d u c a t i o n , I n c .
6
9-11
Sample Orders and Parts View Data
C o p y r i g h t © 2 0 1 7 P e a r s o n E d u c a t i o n , I n c .
9-12
Creating Customer Summary Query
C o p y r i g h t © 2 0 1 7 P e a r s o n E d u c a t i o n , I n c .
7
9-13
Customer Summary
C o p y r i g h t © 2 0 1 7 P e a r s o n E d u c a t i o n , I n c .
9-14
Qualifying Parts Query Design
C o p y r i g h t © 2 0 1 7 P e a r s o n E d u c a t i o n , I n c .
8
9-15
Publish Results: Qualifying Parts Query Results
C o p y r i g h t © 2 0 1 7 P e a r s o n E d u c a t i o n , I n c .
9-16
Publish Results: Sales History for Selected Parts
C o p y r i g h t © 2 0 1 7 P e a r s o n E d u c a t i o n , I n c .
9
9-17
Q3: How Do Organizations Use Data Warehouses and Data Marts to Acquire Data?
C o p y r i g h t © 2 0 1 7 P e a r s o n E d u c a t i o n , I n c .
• Data Warehouse Functions – Obtain data from operational, internal and external databases – Cleanse data – Organize and relate data – Catalog data using metadata
9-18
Components of a Data Warehouse
C o p y r i g h t © 2 0 1 7 P e a r s o n E d u c a t i o n , I n c .
10
9-19
Examples of Consumer Data That Can Be Purchased
C o p y r i g h t © 2 0 1 7 P e a r s o n E d u c a t i o n , I n c .
9-20
Possible Problems with Source Data
C o p y r i g h t © 2 0 1 7 P e a r s o n E d u c a t i o n , I n c .
Curse of dimensionality
11
9-21
Data Warehouses Versus Data Marts
C o p y r i g h t © 2 0 1 7 P e a r s o n E d u c a t i o n , I n c .
9-22
Q4: What are Three Techniques for Processing BI Data?
C o p y r i g h t © 2 0 1 7 P e a r s o n E d u c a t i o n , I n c .
12
9-23
Reporting Analysis
C o p y r i g h t © 2 0 1 7 P e a r s o n E d u c a t i o n , I n c .
• Process of sorting, grouping, summing, filtering, and formatting structured data
• Structured data – Data in rows and columns like tables
• Exception reports – Produced when something out of predefined bounds occurs
• Printed and dynamic reports
9-24
Data Mining
C o p y r i g h t © 2 0 1 7 P e a r s o n E d u c a t i o n , I n c .
• Application of statistical techniques to find patterns and relationships among data for classification and prediction
• Combined discipline of statistics, mathematics, artificial intelligence, and machine-learning
13
9-25
Two Broad Categories of Data Mining
C o p y r i g h t © 2 0 1 7 P e a r s o n E d u c a t i o n , I n c .
1. Unsupervised procedures – Does not start with a priori hypothesis or model – Hypothesized model created afterward based on analytical
results to explain patterns found Example: Cluster analysis
9-26
Supervised Data Mining
C o p y r i g h t © 2 0 1 7 P e a r s o n E d u c a t i o n , I n c .
• Uses a priori model to compute outcome of model
• Prediction, such as multiple linear regression • Ex: CellPhoneWeekendMinutes
= (12 + (17.5*CustomerAge)+(23.7*NumberMonthsOfAccount) = 12 + 17.5*21 + 23.7*6 = 521.7
14
9-27
BigData
C o p y r i g h t © 2 0 1 7 P e a r s o n E d u c a t i o n , I n c .
• Huge volume – Petabyte and larger
• Rapid velocity – Generated rapidly
• Great variety – Structured data, free-form text, log files, graphics, audio, and
video
9-28
MapReduce Processing Summary
C o p y r i g h t © 2 0 1 7 P e a r s o n E d u c a t i o n , I n c .
Google search log broken into pieces
15
9-29
Google Trends on the Term Web 2.0
C o p y r i g h t © 2 0 1 7 P e a r s o n E d u c a t i o n , I n c .
9-30
Hadoop
C o p y r i g h t © 2 0 1 7 P e a r s o n E d u c a t i o n , I n c .
• Open-source program supported by Apache Foundation • Manages thousands of computers • Implements MapReduce
– Written in Java • Amazon.com supports Hadoop as part of EC3 cloud offering • Pig query language platform for large dataset analysis
– Easy to master – Extensible – Automatically optimizes queries on map-reduce level
16
9-31
Q5: What Are the Alternatives for Publishing BI?
C o p y r i g h t © 2 0 1 7 P e a r s o n E d u c a t i o n , I n c .
9-32
What Are the Two Functions of a BI Server?
C o p y r i g h t © 2 0 1 7 P e a r s o n E d u c a t i o n , I n c .
17
9-33
How Does the Knowledge in This Chapter Help You?
C o p y r i g h t © 2 0 1 7 P e a r s o n E d u c a t i o n , I n c .
• Business intelligence a critical skill
• Enables imagining innovative uses for data your employer generates and know some of constraints of such use
• Digital marketing #1 priority for technology investment
• At PRIDE, knowledge of this chapter helps understand possible uses for exercise data
9-34
Ethics Guide: Unseen Cyberazzi
C o p y r i g h t © 2 0 1 7 P e a r s o n E d u c a t i o n , I n c .
• Data broker or Data aggregator – Acquires and purchases consumer and other data from
public records, retailers, Internet cookie vendors, social media trackers, and other sources
– Used to create business intelligence to sell to companies and governments
18
9-35
Ethics Guide: Unseen Cyberazzi (cont'd)
C o p y r i g h t © 2 0 1 7 P e a r s o n E d u c a t i o n , I n c .
• Cheap cloud processing makes processing consumer data easier, less expensive
• Processing happens in secret
• Data brokers enable you to view data stored about you, but: – Difficult to learn how to request your data – Torturous process to file for it – Limited data usefulness
9-36
Ethics Guide: Unseen Cyberazzi (cont'd)
C o p y r i g h t © 2 0 1 7 P e a r s o n E d u c a t i o n , I n c .
• Do you know what data is gathered about you? What is done with it?
• Have you thought about conclusions data aggregators, or their clients, could make based on your use of frequent buyer cards?
• Concerned about what federal government might do with data it gets from data aggregators?
• Where does all of this end?
• What will life be like for your children or grandchildren?
19
9-37
Guide: Semantic Security
C o p y r i g h t © 2 0 1 7 P e a r s o n E d u c a t i o n , I n c .
1. Unauthorized access to protected data and information • Physical security Passwords and permissions Delivery system must be secure
2. Unintended release of protected information through reports and documents
3. What, if anything, can be done to prevent what Megan did?
,
1
Reporting Systems and OLAP
Chapter Extension 13
ce13-2
Study Questions
C o p y r i g h t © 2 0 1 7 P e a r s o n E d u c a t i o n , I n c .
Q1: How do reporting systems enable people to create information?
Q2: What are the components and characteristics of reporting systems?
Q3: How are reports authored, managed, and delivered?
Q4: How are OLAP reports dynamic?
2
ce13-3
Q1: How Do Reporting Systems Enable People to Create Information?
C o p y r i g h t © 2 0 1 7 P e a r s o n E d u c a t i o n , I n c .
• Reporting systems – Create meaningful information from disparate data sources – Deliver information to user on time
• Generate information by: – Filtering data – Sorting data – Grouping data – Making simple calculations
ce13-4
Raw Sales Data: List Contains Little or No Information
C o p y r i g h t © 2 0 1 7 P e a r s o n E d u c a t i o n , I n c .
3
ce13-5
Sales Data Sorted by Customer Name
C o p y r i g h t © 2 0 1 7 P e a r s o n E d u c a t i o n , I n c .
Create Information by Sorting
ce13-6
Sales Data Sorted by Customer Name and Grouped by Number of Orders and Showing Purchase Amounts
C o p y r i g h t © 2 0 1 7 P e a r s o n E d u c a t i o n , I n c .
Create Information by Grouping & Summing
4
ce13-7
Sales Data Filtered to Show Repeat Customers
C o p y r i g h t © 2 0 1 7 P e a r s o n E d u c a t i o n , I n c .
Create Information by Filtering & Formatting
ce13-8
Q2: What Are the Components and Characteristics of Reporting Systems?
C o p y r i g h t © 2 0 1 7 P e a r s o n E d u c a t i o n , I n c .
• Reads and combines data from disparate data sources
• Filters, sorts, groups, simple calculations to produce meaningful contexts
• Metadata to prepare and deliver reports • Report characteristics
– Type – Media – Mode
5
ce13-9
Components of a Reporting System
C o p y r i g h t © 2 0 1 7 P e a r s o n E d u c a t i o n , I n c .
ce13-10
Summary of Report Characteristics
C o p y r i g h t © 2 0 1 7 P e a r s o n E d u c a t i o n , I n c .
6
ce13-11
Report Media
C o p y r i g h t © 2 0 1 7 P e a r s o n E d u c a t i o n , I n c .
• Printed on paper • PDFs—Portable Document Format • Delivered to computer screens and mobile devices
Applications for CRM and ERP systems • Digital dashboards • Alerts • Exported from report generator to another program • Published via Web service
ce13-12
Digital Dashboard Example
C o p y r i g h t © 2 0 1 7 P e a r s o n E d u c a t i o n , I n c .
7
ce13-13
Report Mode
C o p y r i g h t © 2 0 1 7 P e a r s o n E d u c a t i o n , I n c .
Types of reports
• Push report – Sent or e-mailed to users according to a preset schedule
• Pull report – Users download report from web portal or digital dashboard
ce13-14
Q3: How Are Reports Authored, Managed and Delivered?
C o p y r i g h t © 2 0 1 7 P e a r s o n E d u c a t i o n , I n c .
Report authoring • Connecting to data sources, create report structure, format
report • Examples: MS Access, Visual Studio
Report management • Defines who receives what reports, when, what format, and
by what means • Defines user accounts and user groups
8
ce13-15
Connecting to a Report Data Source Using Visual Studio
C o p y r i g h t © 2 0 1 7 P e a r s o n E d u c a t i o n , I n c .
ce13-16
How Are Reports Delivered?
C o p y r i g h t © 2 0 1 7 P e a r s o n E d u c a t i o n , I n c .
Report delivery function • Pushes or allows pulling reports based on metadata
• Delivered via an email server, Web site, SOA services, or other program-specific means
• Security components ensure only authorized users receive reports
• For queries, serves as intermediary between users and report generator
9
ce13-17
Q4: How Are OLAP Reports Dynamic?
C o p y r i g h t © 2 0 1 7 P e a r s o n E d u c a t i o n , I n c .
• OLAP provides ability to sum, count, average, perform other simple arithmetic operations on groups of data
• OLAP reports – Dynamic
– Measure: item summed, averaged or processed in OLAP report.
– Dimension: category or class
– Cube: measure with associated dimensions
– OLAP vendors and products
ce13-18
OLAP CUBE: Typical OLAP Report
C o p y r i g h t © 2 0 1 7 P e a r s o n E d u c a t i o n , I n c .
OLAP Product Family by Store Type
10
ce13-19
OLAP Sales Report by Product Family, Store Country, State, Store Type
C o p y r i g h t © 2 0 1 7 P e a r s o n E d u c a t i o n , I n c .
ce13-20
OLAP Sales Report by Country, State, City and Store Type
C o p y r i g h t © 2 0 1 7 P e a r s o n E d u c a t i o n , I n c .
11
ce13-21
Role of OLAP Server and Dimensional Database
C o p y r i g h t © 2 0 1 7 P e a r s o n E d u c a t i o n , I n c .
,
1
Database Marketing
Chapter Extension 12
ce12-2
Study Questions
C o p y r i g h t © 2 0 1 7 P e a r s o n E d u c a t i o n , I n c .
Q1: What is a database marketing opportunity?
Q2: How does RFM analysis classify customers?
Q3: How does market-basket analysis identify cross-selling opportunities?
Q4: How do decision trees identify market segments?
2
ce12-3
Owner of Carbon Creek Gardens Needs Database Marketing
C o p y r i g h t © 2 0 1 7 P e a r s o n E d u c a t i o n , I n c .
• As business grew, lost track of customers
• Lost valuable customer and didn't know it
• Has lot of sales data, but needs system to: – Store and track customers – Store and track services provided to customers – Store and report future scheduled services
ce12-4
Database Marketing
C o p y r i g h t © 2 0 1 7 P e a r s o n E d u c a t i o n , I n c .
• Application of business intelligence systems to: – Planning marketing programs – Executing marketing programs – Assessing marketing programs
• Databases and data mining techniques key components
3
ce12-5
Q2: How Does RFM Analysis Classify Customers?
C o p y r i g h t © 2 0 1 7 P e a r s o n E d u c a t i o n , I n c .
• Recently
• Frequently
• Money
ce12-6
RFM Analysis Classifies Customers
C o p y r i g h t © 2 0 1 7 P e a r s o n E d u c a t i o n , I n c .
4
ce12-7
Q3: How Does Market-Basket Analysis Identify Cross-Selling Opportunities?
C o p y r i g h t © 2 0 1 7 P e a r s o n E d u c a t i o n , I n c .
• Unsupervised data mining method – Statistical methods to identify sales patterns in large volumes
of data – Products customers tend to buy together – Probabilities of customer purchases – Identify cross-selling opportunities
How many customers bought both fins and a mask?
ce12-8
Market-Basket Example: Transactions = 400
C o p y r i g h t © 2 0 1 7 P e a r s o n E d u c a t i o n , I n c .
5
ce12-9
Support: Probability that Two Items Will Be Bought Together
C o p y r i g h t © 2 0 1 7 P e a r s o n E d u c a t i o n , I n c .
• P(Fins and Masks) = 250/400, or 62% • P(Fins and Weights) = 20/400, or 5%
ce12-10
Confidence = Conditional Probability Estimate
C o p y r i g h t © 2 0 1 7 P e a r s o n E d u c a t i o n , I n c .
• Probability of buying Fins = 250 — Probability of buying Mask = 270 • P(After buying Mask, then will buy Fins) — Confidence = 250/270 or 92.6%
6
ce12-11
Lift = Confidence ÷ Base Probability
C o p y r i g h t © 2 0 1 7 P e a r s o n E d u c a t i o n , I n c .
• Lift = Confidence of Mask/Base P(Fins)
• Lift = .926/.625 = 1.32
ce12-12
Warning!
C o p y r i g h t © 2 0 1 7 P e a r s o n E d u c a t i o n , I n c .
• Analysis only shows shopping carts with two items
• Must analyze large number of shopping carts with three or more items
• Know what problem you are solving before mining the data – Know what question you want to answer
7
ce12-13
Q4: How Do Decision Trees Identify Market Segments?
C o p y r i g h t © 2 0 1 7 P e a r s o n E d u c a t i o n , I n c .
• Hierarchical arrangement of criteria to predict a classification or value
• If/Then hierarchy
• Unsupervised data mining technique
• Basic idea of a decision tree – Select attributes most useful for classifying something on
some criterion to create “pure groups”
ce12-14
Decision Tree for Student
Performance
C o p y r i g h t © 2 0 1 7 P e a r s o n E d u c a t i o n , I n c .
If Junior = Yes
Lower-level groups more similar than higher-level groups
8
ce12-15
Transforming a Set of Decision Rules
C o p y r i g h t © 2 0 1 7 P e a r s o n E d u c a t i o n , I n c .
• If student is a junior and works in a restaurant, – Then predict grade =>3.0
• If student is a senior and is a nonbusiness major, – Then predict grade <3.0
• If student is a junior and does not work in a restaurant, – Then predict grade <3.0
• If student is a senior and is a business major, – Then make no prediction
ce12-16
Decision Tree for Loan Evaluation
C o p y r i g h t © 2 0 1 7 P e a r s o n E d u c a t i o n , I n c .
• Classify loan applications by likelihood of default • Rules identify loans for bank approval • Identify market segment • Structure marketing campaign • Predict problems
9
ce12-17
Credit Score Decision Tree
C o p y r i g h t © 2 0 1 7 P e a r s o n E d u c a t i o n , I n c .
ce12-18
Ethics Guide: Data Mining in the Real World
C o p y r i g h t © 2 0 1 7 P e a r s o n E d u c a t i o n , I n c .
Problems with data: • Dirty data • Missing values • Lack of knowledge at start of project • Overfitting – too many variables • Probabilistic—good model may have unlucky first uses • Seasonality influences • High risk – uncovering something self-defeating to reveal
10
ce12-19
Ethics Guide: Data Mining in the Real World (cont’d)
C o p y r i g h t © 2 0 1 7 P e a r s o n E d u c a t i o n , I n c .
• When you start a data mining project, you never know how it will turn out
• Decision trees can be used to select variables for other types of data mining analysis
,
1
Data Breaches
Chapter Extension 14
ce14-2
Study Questions
C o p y r i g h t © 2 0 1 7 P e a r s o n E d u c a t i o n , I n c .
Q1: What is a data breach?
Q2: How do data breaches happen?
Q3: How should organizations respond to data breaches?
Q4: What are the legal consequences of a data breach?
Q5: How can data breaches be prevented?
2
ce14-3
Q1: What is A Data Breach?
C o p y r i g h t © 2 0 1 7 P e a r s o n E d u c a t i o n , I n c .
• Data breach – Unauthorized person views, alters, or steals secured data
• 1+ billion people affected in past 5 years, 75% of breaches happened in US
• Average cost of a single data breach $3.5 million • Average costs per stolen record
Healthcare ($359), Pharmaceutical ($227 Communications industries ($177)
Education ($294) Financial ($206)
ce14-4
Costs of Handling a Data Breach
Direct Costs
• Notification
• Detection
• Escalation
• Remediation
• Legal fees and consultation
Indirect Costs • Loss of reputation • Abnormal customer
turnover • Increased customer
acquisition activities • Additional $3.3 million
per incident in US
C o p y r i g h t © 2 0 1 7 P e a r s o n E d u c a t i o n , I n c .
3
ce14-5
What Are the Odds?
• More likely to lose smaller amounts of data than larger amounts of data 22% chance of losing 10,000 records over any 24-month
period <1% chance of losing 100,000 records over same period
C o p y r i g h t © 2 0 1 7 P e a r s o n E d u c a t i o n , I n c .
ce14-6
Well-known Data Breaches
C o p y r i g h t © 2 0 1 7 P e a r s o n E d u c a t i o n , I n c .
4
ce14-7
Why Do Data Breaches Happen?
• 67% are hackers trying to make money from: – Personally identifiable information (PII) Names, addresses, dates of birth, Social Security
numbers, credit card numbers, health records, bank account numbers, PINs, email addresses
• Rogue internal employees
• Credit card fraud, identity theft, extortion, industrial espionage
C o p y r i g h t © 2 0 1 7 P e a r s o n E d u c a t i o n , I n c .
ce14-8
Q2: How Do Data Breaches Happen?
• Attack vectors – Phishing scam – Trick users into donating funds for a natural disaster – Exploit new software vulnerability
C o p y r i g h t © 2 0 1 7 P e a r s o n E d u c a t i o n , I n c .
5
ce14-9
Hitting Target
• Lost 40 million credit and debit card numbers to attackers (Dec. 18, 2013)
• Less than a month later, announced additional 70 million customer names, emails, addresses, phone numbers stolen – Total 98 million customers affected
• Stolen from point-of-sale (POS) systems at Target retail stores
C o p y r i g h t © 2 0 1 7 P e a r s o n E d u c a t i o n , I n c .
ce14-10
How Did They Do It?
C o p y r i g h t © 2 0 1 7 P e a r s o n E d u c a t i o n , I n c .
6
ce14-11
The Damage
• Attackers sold about 2 million credit card numbers and PINs for about $26.85 each (total $53.7 million)
• Sold in batches of 100,000 card numbers
• Cost Target $450 million – Upgraded POS terminals to support chip-and-PIN enabled cards – Increased insurance premiums, legal fees, credit card processors
settlement, pay for consumer credit monitoring, regulatory fines – Lost sales, 46% drop in next quarter revenues
C o p y r i g h t © 2 0 1 7 P e a r s o n E d u c a t i o n , I n c .
ce14-12
Collateral Damage
• Credit unions and banks – Spent more than $200 million issuing new cards
• Consumers – Enrolled in credit monitoring, continually watch their credit,
and fill out paperwork if fraudulent charges appear on statements
• Increased insurance premiums, stricter controls, and more system auditing for organizations similar to Target
C o p y r i g h t © 2 0 1 7 P e a r s o n E d u c a t i o n , I n c .
7
ce14-13
Q3: How Should Organizations Respond To Data Breaches?
• Respond Quickly – Stop hackers from doing more damage Exfiltration or illegally transferring data out
– Immediately notify affected users
• Plan for a Data Breach – Walkthroughs, business continuity planning, computer
security incident response team (CSIRT)
C o p y r i g h t © 2 0 1 7 P e a r s o n E d u c a t i o n , I n c .
ce14-14
Q3: How Should Organizations Respond To Data Breaches? (cont'd)
• Get experts to perform an effective forensic investigation
• Identify additional technical and law enforcement professionals needed
• Be honest about the breach
C o p y r i g h t © 2 0 1 7 P e a r s o n E d u c a t i o n , I n c .
8
ce14-15
Best Practices for Notifying Users of a Data Breach
C o p y r i g h t © 2 0 1 7 P e a r s o n E d u c a t i o n , I n c .
ce14-16
Q4: What Are The Legal Consequences of a Data Breach?
C o p y r i g h t © 2 0 1 7 P e a r s o n E d u c a t i o n , I n c .
9
ce14-17
Regulatory Laws Govern the Secure Storage of Data in Certain Industries • Federal Information Security Management Act (FISMA)
– Requires security precautions for government agencies
• Gramm-Leach-Bliley Act (GLBA), a.k.a., Financial Services Modernization Act – Requires data protection for financial institutions
• Health Information Portability and Accountability Act (HIPAA) – Requires data protection for healthcare institutions
• Payment Card Industry Data Security Standard (PCI DSS) – Governs secure storage of cardholder data
• Family Educational Rights and Privacy Act (FERPA) – Provides protection for student education records
C o p y r i g h t © 2 0 1 7 P e a r s o n E d u c a t i o n , I n c .
ce14-18
Q5: How Can Data Breaches Be Prevented?
• Use countermeasures software or procedures to prevent an attack
• Better phishing detection software
• Better authentication (i.e., multifactor authentication
• Network intrusion detection system (NIDS) to examine traffic passing through internal network
• Data loss prevention systems (DLP) to prevent sensitive data from being released to unauthorized persons
C o p y r i g h t © 2 0 1 7 P e a r s o n E d u c a t i o n , I n c .
10
ce14-19
Q5: How Can Data Breaches Be Prevented? (cont'd) • Appoint a chief information security officer (CISO) to ensure
sufficient executive support and resources
C o p y r i g h t © 2 0 1 7 P e a r s o n E d u c a t i o n , I n c .
Collepals.com Plagiarism Free Papers
Are you looking for custom essay writing service or even dissertation writing services? Just request for our write my paper service, and we'll match you with the best essay writer in your subject! With an exceptional team of professional academic experts in a wide range of subjects, we can guarantee you an unrivaled quality of custom-written papers.
Get ZERO PLAGIARISM, HUMAN WRITTEN ESSAYS
Why Hire Collepals.com writers to do your paper?
Quality- We are experienced and have access to ample research materials.
We write plagiarism Free Content
Confidential- We never share or sell your personal information to third parties.
Support-Chat with us today! We are always waiting to answer all your questions.
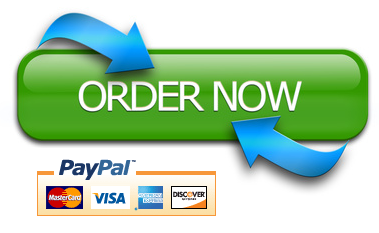