This week you will search the literature in the school databases for article within 5 years of todays date, that are appropriate
This week you will search the literature in the school databases for article within 5 years of today’s date, that are appropriate for your PICOT question below.
1. In the adult acute care, does hourly rounding compared to infrequent rounding impact patient falls during recovery at the hospital?
The article is a SYSTEMATIC REVIEW research study.
- Read the first few sentences of methods section of your articles to assess what type of article you have
- Critique each article using the appropriate Appraisal Forms. The form takes you through a reflection on WHY was research done-HOW was research done and WHAT was found.
- Review rubric carefully to ensure all questions have been answered. Points are deducted for articles not loaded or if incorrect type of article submitted.
- All answers to questions are brief and only 1- 2 sentences. Example: What group produced the guideline? Answer: US Preventive Services Task Force develops recommendations about preventive services based on a review of high-quality scientific evidence and publishes its recommendations on its website and or in a peer reviewed journal
- Avoid any copying and pasting 7 or more words of content from the article or another source. Use your own words to create your answers. APA is not required for content of answers on template
- APA is only required for your citation on the template.
APPENDIX C
Appraisal Guide:
Conclusions of a Systematic Review with Narrative Synthesis
Citation:
____________________________________________________________________________
____________________________________________________________________________
____________________________________________________________________________
Synopsis
What organization or persons produced the systematic review (SR)?
How many persons were involved in conducting the review?
What topic or question did the SR address?
How were potential research reports identified?
What determined if a study was included in the analysis?
How many studies were included in the review?
What research designs were used in the studies?
What were the consistent and important across-studies conclusions?
Credibility
Was the topic clearly defined? Yes No Not clear
Was the search for studies and other evidence comprehensive and unbiased? Yes No Not clear
Was the screening of citations for inclusion based on explicit criteria? Yes No Not clear
*Were the included studies assessed for quality? Yes No Not clear
Were the design characteristics and findings of the included studies displayed or discussed in sufficient detail? Yes No Not clear
*Was there a true integration (i.e., synthesis) of the findings—not merely reporting of findings from each study individually? Yes No Not clear
*Did the reviewers explore why differences in findings might have occurred? Yes No Not clear
Did the reviewers distinguish between conclusions based on consistent findings from several good studies and those based on inferior evidence (number or quality)? Yes No Not clear
Which conclusions were supported by consistent findings from two or more good or high-quality studies? List
____________________________________________________________________________
____________________________________________________________________________
____________________________________________________________________________
Are the conclusions credible? Yes All Yes Some No
Clinical Significance
*Across studies, is the size of the treatment or the strength of the association found or the meaningfulness of qualitative findings strong enough to make a difference in patient outcomes or experiences of care? Yes No Not clear
Are the conclusions relevant to the care the nurse gives? Yes No Not clear
Are the conclusions clinically significant? Yes All Yes Some No
Applicability
Does the SR address a problem, situation, or decision we are addressing in our setting? Yes No Not clear
Are the patients in the studies or a subgroup of patients in the studies similar to those we see? Yes No Not clear
What changes, additions, training, or purchases would be needed to implement and sustain a clinical protocol based on these conclusions? Specify and list
____________________________________________________________________________
____________________________________________________________________________
Is what we will have to do to implement the new protocol realistically achievable by us (resources, capability, commitment)? Yes No Not clear
How will we know if our patients are benefiting from our new protocol? Specify
____________________________________________________________________________
____________________________________________________________________________
Are these conclusions applicable to our setting? Yes All Yes Some No
Should we proceed to design a protocol incorporating these conclusions? Yes All Yes Some No
* = Important criteria
Comments
____________________________________________________________________________
____________________________________________________________________________
APP C-2 Brown
Brown APP C-1
,
���������� �������
Citation: Kristoffersson, A.; Lindén,
M. A Systematic Review of Wearable
Sensors for Monitoring Physical
Activity. Sensors 2022, 22, 573.
https://doi.org/10.3390/s22020573
Academic Editor: Emiliano Schena
Received: 29 November 2021
Accepted: 5 January 2022
Published: 12 January 2022
Publisher’s Note: MDPI stays neutral
with regard to jurisdictional claims in
published maps and institutional affil-
iations.
Copyright: © 2022 by the authors.
Licensee MDPI, Basel, Switzerland.
This article is an open access article
distributed under the terms and
conditions of the Creative Commons
Attribution (CC BY) license (https://
creativecommons.org/licenses/by/
4.0/).
sensors
Review
A Systematic Review of Wearable Sensors for Monitoring Physical Activity Annica Kristoffersson * and Maria Lindén
School of Innovation, Design and Engineering, Mälardalen University, Box 883, 721 23 Västerås, Sweden; [email protected] * Correspondence: [email protected]
Abstract: This article reviews the use of wearable sensors for the monitoring of physical activity (PA) for different purposes, including assessment of gait and balance, prevention and/or detection of falls, recognition of various PAs, conduction and assessment of rehabilitation exercises and monitoring of neurological disease progression. The article provides in-depth information on the retrieved articles and discusses study shortcomings related to demographic factors, i.e., age, gender, healthy participants vs patients, and study conditions. It is well known that motion patterns change with age and the onset of illnesses, and that the risk of falling increases with age. Yet, studies including older persons are rare. Gender distribution was not even provided in several studies, and others included only, or a majority of, men. Another shortcoming is that none of the studies were conducted in real-life conditions. Hence, there is still important work to be done in order to increase the usefulness of wearable sensors in these areas. The article highlights flaws in how studies based on previously collected datasets report on study samples and the data collected, which makes the validity and generalizability of those studies low. Exceptions exist, such as the promising recently reported open dataset FallAllD, wherein a longitudinal study with older adults is ongoing.
Keywords: wearable sensors; sensor systems; gait and balance; fall detection; physical activity recognition; rehabilitation; neurological diseases; data sets; research shortcomings; user demography
1. Introduction
In this article, we review the use of wearable sensors for monitoring physical activity (PA). The monitoring of PA has a number of different purposes. These include: the assess- ment of gait and balance, prevention and/or detection of falls, the recognition of various PAs. PA monitoring is also useful for the conduction and assessment of rehabilitation exercises and for the monitoring of neurological disease progression. The monitoring of PAs may seem distant from the monitoring of patients with neurological diseases, but neurological diseases are often characterized by mobility disturbances and the same types of wearable sensors can, and are, being used in both areas. The WHO [1] pinpoints neuro- logical diseases as being one of the greatest threats to public health. Common neurological diseases include dementia, epilepsy, headache disorders, multiple sclerosis, neuroinfections, Parkinson’s disease, stroke and traumatic injuries but also neurological diseases associated with malnutrition and pain.
Undoubtedly, the development and evaluations of advanced wearable sensor sys- tems have the potential to generate algorithms allowing for personalized diagnoses and treatment, i.e., precision health. However, for this to be realized, algorithms need to be developed based on data that is representative to the expected users of the wearable sensor systems. In a previously published systematic review, Kristoffersson and Lindén (2020a) [2] discussed a number of issues that are important to consider when developing and eval- uating wearable sensor systems to be used in real-life conditions. These issues include studies conducted with small samples but also those not taking demographic factors,
Sensors 2022, 22, 573. https://doi.org/10.3390/s22020573 https://www.mdpi.com/journal/sensors
Sensors 2022, 22, 573 2 of 39
namely age, gender and healthy participants vs patients sufficiently into account. Here, we highlight those factors that are within the scope of this review. Starting with gait, 35% of the non-institutional adults were reported to have an abnormal gait in [3]. Further, age, fall history, mobility impairments, sleep disturbance and neurological disorders are all factors affecting fall risk [4]. Chronic conditions, which become more common in aging persons, affect PA levels, but also activities such as raising from a chair can become demanding [3]. Kristoffersson and Lindén (2020a) [2] write that “the whole motion pattern changes with age and the onset of illnesses related to the human locomotor system” (p. 15). This issue is also discussed in [4] which points out that existing systems have mainly been tested under controlled conditions in laboratory environments and that future work must focus on the conduction of longitudinal studies in real-life conditions on samples including frequent fallers, aging adults and those with neurological disorders [4].
There are also gender differences related to the scope of this article. For example, osteoporosis is more common among women but under-diagnosed among men. The characteristics of osteoporosis are decreased bone mass density and disrupted normal trabecular architecture reducing bone strength [5]. While increasing the risk of fractures after a fall, symptoms may remain unnoticed until a fracture has already occurred [6]. Not only that, but, changes in bone mass density and bone strength are factors increasing the fall risk among patients with osteoporosis. The fear of falling, particularly among women, is associated with more falls [7]. Confidence in balance is also reported as being related to balance and mobility among older women [8]. Another illness associated with falls is thoracic kyphosis, an abnormal convex curvature of the spine at chest height. The illness is more common among older women than men due to losses in estrogen levels [9]. Women with thoracic kyphosis were found to be more likely to have had a recent fall in [7].
Evidently, healthy participants and patients differ in many aspects. Therefore, the inclusion of patients in evaluations of wearable systems is a necessity for increasing the validity of these systems. In addition, studies must be conducted with sufficiently large samples for achieving generalizable results.
The remainder of this article is organized as follows. Section 2 provides a summarized description of the systematic method used for selecting articles from the two literature searches. Section 3 reports on terminology aimed to facilitate the reading of this article. Thereafter, Section 4 reports on all articles relating to fall prevention. This is a complex issue, therefore the section includes articles on gait and balance (Section 4.1), fall detection (Section 4.2) and physical activity recognition (Section 4.3). Section 5 reports on rehabil- itation articles and Section 6 on neurological diseases articles. Section 7 reports on the included articles not fitting directly into any of the aforementioned sections. A considerable number of notes are deliberately used within Sections 4–7. There are multiple reasons for this; to facilitate the reading of this article and the identification of suitable methods that are already being used in different contexts. Section 8 provides a discussion and concluding remarks on the material analyzed in this article.
2. Methodology
Previously, Kristoffersson and Lindén (2020a) [2] performed and published a sys- tematic review on the use of wearable body sensors for health monitoring. The original article, which covered a large field of health monitoring, provided a qualitative synthesis of sociodemographic and research-methodological aspects of 73 articles. These articles were retrieved during a literature search conducted in April 2019. The search was repeated on 6 August 2020 and resulted in the retrieval of 31 additional articles fulfilling the original inclusion criteria. Broadly speaking, these articles can be divided into two areas: one being the monitoring and prevention of noncommunicable diseases (45 articles), and one being the monitoring of physical activity and neurological diseases (54 articles). The present systematic review focuses solely on the articles related to the monitoring of physical activity and neurological diseases and provides a deep analysis of these articles.
Sensors 2022, 22, 573 3 of 39
Following the requirements of MDPI Sensors, the literature searches were systematic and followed the PRISMA guidelines [10]. Search phrases were selected based on the main research question “How are wearable sensors used for health monitoring?” Seven databases were used: Web of Science Core Collection, MEDLINE, Scopus, ScienceDirect, Academic Search Elite, ACM Digital Library and IEEE Xplore. Web of Science Core Collection itself included six indices: SCI-EXPANDED, SSCI, A&HCI, CPCI-S, SPCI-SSH and ESCI. The search phrases varied slightly between databases but the same search phrases were used, both in April 2019 and August 2020. No time limit for the publications were set in the April 2019 search, the August 2020 search was limited to publications from January 2019 to August 2020. An overview of search phrases, hits, the article selection process and distribution of articles is provided in Kristoffersson and Lindén (2020b) [11], which focuses on the use of wearable sensors for monitoring and preventing noncommunicable diseases.
2.1. Selection of Articles for This Review
This article presents more in-depth information on a subset of the original 104 articles. Narrowing down the scope of the review to articles on the use of wearable sensors for monitoring physical activity and neurological diseases, articles falling into categories not relating to physical activity monitoring were out of scope. A few articles included in the article category “Additional” in [2] were excluded in this article as it does not report on studies already conducted but only studies to come.
2.2. Articles Included in This Review
In this article, we have divided the articles into six article categories: gait and balance (6 articles), fall detection (10), physical activity recognition (12), rehabilitation (10), neuro- logical diseases (9), and additional (7). The category “gait and fall” used in [2] was divided into two separate categories: “gait and balance” and “fall detection”. Further, a few articles in [2] were re-categorized in this article. In total, this review includes 54 articles.
The 54 articles were published between 2010 and 2020. The distribution per year was the following: 2010 (1 article), 2011 (1), 2012 (5), 2013 (2), 2014 (2), 2015 (6), 2016 (4), 2017 (6), 2018 (8), 2019 (14) and 5 articles were published and indexed prior to 6 August 2020. Five articles [12–16] were based on data from ≥1 previously published datasets [17–26]. These five articles [12–16] were published in 2017 or later and were found to report on the datasets’ demographics and sensors/activities to varying degrees. We therefore report on these articles within Sections 4–7. Appendix A provides information provided in articles based on datasets [12–16] in Table A1 and the original datasets [17–26] in Table A2.
In addition, we have conducted follow up searches if the included articles reported on were ongoing or were planned studies. These searches resulted in an additional four included articles. Three of them, which are discussed in Section 4.2, were published during 2019–2021. Interestingly, one of them [27] reports on a new dataset that is also included in Table A2 in Appendix A. Another article, from 2014, is discussed in Section 5.
3. Abbreviations and Terminology
To facilitate the reading, this section introduces abbreviations and terminology com- monly used within this article. The majority of works use sensors measuring PA, i.e., inertial measurement units (IMUs). For readability, we use the notations accelerometer, 6D IMU and 9D IMU in the remainder of this paper. The notation 6D IMU is used for sensors including a 3D accelerometer and a 3D gyroscope. The notation 9D IMU is used for sensors that also include a 3D magnetometer. A few works use sensors measuring vital signs, such as heart rate (HR), peripheral oxygen saturation (SpO2) and respiration rate (RR). Other sensors included are electrocardiography (ECG), electrodermal activity (EDA), electromyography (EMG), energy expenditure (EE), finger tapping, inter-beat interval, photoplethosmography (PPG), pressure, skin temperature (ST) and surface EMG (sEMG). A few works report on the use of motion capture systems such as Vicon1 and Microsoft Kinect [28] for validation.
Sensors 2022, 22, 573 4 of 39
Several common tests for assessing gait and balance are used within the included articles. These include the: sit-to-stand (S2S) test, six-minute walk test (6MWT), 30-s chair stand (30SCS) test, and the Timed Up and Go (TUG) test. A number of works adopt machine learning algorithms, these include: artificial neural network (ANN), bagged tree, convolutional neural network (CNN), decision tree (DT), dynamic time warping (DTW), hidden Markov model (HMM), k-nearest neighbors (kNN), linear discriminate analysis (LDA), naïve Bayes (NB), nearest neighbor with DTW (NN-DTW), random forest (RF), recurrent convolutional neural network (RCNN), support vector machine (SVM), support vector machine recursive feature extraction (SVM-RFE), support vector regression (SVR), time kNN, weighted-SVM (W-SVM) and wireless kNN.
4. Fall Prevention
Falls have been pointed out as the second leading cause of accidental or unintentional injury deaths worldwide by the WHO [29] who reports an estimated 646,000 fatal falls per year. Only traffic incidents result in more deaths by unintentional injury. The death rate after a fall is highest among adults aged ≥60. Studying the number of falls leading to death, adults aged ≥65 suffer the greatest number of falls. The majority of the non-fatal 37.3 million yearly falls require medical attention. Together, they are responsible for a loss of more than 17 million disability-adjusted life years (DALYs). Needless to say, the financial costs resulting from fall-related injuries are high. It is reported that the average hospital cost for a fall injury exceeds $30,000 [4]. Preventing falls is important not only from an economical perspective but also for the well-being of individuals. Being afraid of falling is associated with the avoidance of activities in daily living (ADLs), a reduction in performed PAs, depression, the perception of a lower quality of life and falls [30]. Rajagopalan et al. [4] reviewed the state-of-the-art (SoA) in fall detection and prediction systems and found that existing systems focus mainly on detecting falls. There is a need for monitoring systems that can reduce the number of falls and improve quality of life and safety for those who have fallen or are afraid of falling. Fall prediction and prevention systems are important [4]. We argue that reliable fall prediction methods are necessary if we are to prevent falls.
The WHO [29] outlines different measures for identifying and reducing the risk of falling, these include: screening for fall risk within living environments, clinical interven- tions (e.g., medication reviews, low blood pressure treatment, recommendations on vitamin intake, and correction of visual impairment), home assessment and modification to reduce risk, the prescription of assistive devices addressing physical and/or sensory impairments, muscle and balance training, etc.
Predicting falls is complex. The motion patterns, i.e., gait and balance, changes with age and the onset of illnesses affecting human locomotion. Distinguishing near-fall events and fall events require the ability to discriminate between ADLs and fall-related events, i.e., a wearable sensor system should assist in preventing falls from happening. At the same time, the system should not issue alarms when the user is carrying out normal ADLs. In the remainder of this section, we first present articles related to gait and balance in Section 4.1. Thereafter, we present articles related to fall detection and near-fall detection in Section 4.2 and physical activity recognition in Section 4.3.
4.1. Gait and Balance
Six works use wearable sensors for assessing gait and balance. Gait is at focus in [31–33] while [34,35] focus on balance. TUG is known to predict older adult’s ability to walk independently and [36] presents work on a mobile real-time TUG test for home use. Table 1 shows that the number of older participants, i.e., adults being ≥65 years, in the included studies is low. Most studies have been conducted with both male and female participants. All studies were conducted with healthy participants, i.e., not with patients. One work [35] provides little information on demographics. Both young and old adults participated in two of the gait studies [32,33], however only [33] compares the two groups. Young participants are used in [31,34,36]. Three studies [34–36] have been conducted with less than
Sensors 2022, 22, 573 5 of 39
10 participants and all studies are observational. As shown in Table 2, there is no consistency in the sensor types used, number of sensors, sensor locations or parameters assessed.
Table 1. Participant demographics for studies on gait and balance. – = no information.
Ref. Research Design No. of Participants Age Group Age Statistics Male/Female Patient/Healthy
[31] observational 34 – 28.22 ± 12.77 21 /13 0/34 [32] observational 24 (12/12) 20–40 32.5 ± 4.8 7/5 0/12
65.0 ± 8.8 5/7 0/12 [33] observational 56 (28/28) – 24.6 ± 2.7 14/14 0/28
>55 66.1 ± 5.0 18/10 0/28 [34] observational 2 28 and 24 – 1/1 0 /2 [35] observational 3 40–70 – – – [36] observational 5–6 (1/5) 27 – 1/0 –
21–36 27 4/1 –
Table 2. Study characteristics for studies on gait and balance.
Ref. Sensor and Amount Sensor Location Aim
[31] 1 accelerometer, 1 force-place instrumented treadmill for validation
on the ear validating the use of an ear-worn accelerometer for monitoring gait
[32] 1 accelerometer, 1 GaitRite instrumented walkway for validation
on the lumbar vertebrae (L5) validate the use of an accelerometer worn on the lower back for monitoring gait
[33] 4 6D IMUs on the ankles and wrists assess and compare younger and older adults’ gait
[34] 1 6D IMU, 1 Qualisys motion capture system for validation
on the back at waist estimate state of balance
[35] 1 accelerometer between the lower shoulder blades improving balance through feedback when center of mass is shifted
[36] 5 9D IMUs on the foot, thigh, two shins, torso side
mobile TUG test
Starting with the works focusing on gait, references [31–33] present three different approaches. Aiming at validating the use of an ear-worn accelerometer for gait monitoring, Atallah et al. (2012) [31] asked 34 participants to walk on a force-plate instrumented treadmill at seven different speeds while wearing an accelerometer behind the ear. The features extracted per speed and participant were the zero-crossing, which indicates new gait cycles per axis, the maximal amplitude per stride for each acceleration axis and all possible combinations of axes. Then, multiple linear regression was conducted for assessing the relationship between the maximal amplitude features for all speeds and participants. For comparison, a number of normalized gait parameters derived from the treadmill were used: maximal force, weight-acceptance peak force and impulse. Regarding maximal force, the highest R2 values and lowest estimate of error variance values were obtained for a combination of the VT/AP axes, and for a combination of the VT/AP/ML axes where VT is the vertical axis, AP is the anterior–posterior axis and ML is the medio-lateral axis (the axes are depicted in Figure 1). Regarding weight-acceptance peak force, the highest R2 values were obtained for the same combinations of axes, i.e., the VT/AP and VT/AP/ML axes, whereas the derived R2 values for impulse were lower. Comparing gait cycle parameters predicted by the treadmill and zero-crossings, the errors were higher for the AP and VT axes at lower speeds. Atallah et al. [31] discuss that these speeds may allow for more sway and head movement. The gait cycle parameters derived from accelerometer data were close to the values derived from the treadmill, particularly for the ML axis, with a mean difference of 0.02 s for data within the first standard deviation. It is concluded that
Sensors 2022, 22, 573 6 of 39
the development of regression models using the methodology could provide real-time force-loading features at different speeds.
Figure 1. Axes of rotation and anatomical planes. The frontal plane (coronal plane) is dividing the body into anterior and posterior sections, the sagittal plane is dividing the body into left and right sections and the transverse (horizontal) plane is dividing the body into upper and lower sections.
Godfrey et al. (2014) [32] assess the validity and reliability of using an accelerometer on the lumbar vertebrae (L5) to assess gait. An equal portion of 12 younger and 12 older adults performed two tasks during which they walked five laps along a 25-m oval route at a normal or fast speed. The participants rested for 1 min between the two walking tasks. Additional data was collected using a 7-m-long GaitRite instrumented walkway located along one of the oval’s long-sides (gait characteristics) and a video camera (step counting). Information on step and stride times was estimated based on the extracted initial and final contacts from the accelerometer data collected during laps 2–4 for each speed, according to the method in [37], which is a modification of the method in [38]. Step length was estimated according to the method in [39], after which step velocity was calculated. To compare the accelerometer data with the GaitRite data, only the data segments collected when walking along the GaitRite were extracted. All gait parameters were validated with the younger group. For the older group, similar validity values were achieved for step time and stride times. The estimated step velocity was less accurate for the older group but acceptable according to [40]. Future work on replicating the full gait model [41] and conducting studies with healthy older adults is reported but we have not found such work.
Zhong et al. (2019) [33] explored differences in gait patterns between 28 healthy younger and 28 older adults. The 56 participants wore four bracelets equipped with 9D IMUs on their ankles and wrists while walking ten times along a 14-m-long corridor at three self-selected paces: slow, normal and fast. Tape indicated the start and end points and only the middle 10 m for the third and fifth walking trial at each walking pace were used for assessing gait patterns. Gait speed, stride frequency, average stride length, stride regularity, stride time variability and magnitude of acceleration were extracted. In total, the analysis included 336 trials (56 people performing two walking trials at three different speeds). The magnitude of the acceleration, i.e., the root-mean-square (RMS) acceleration was found to increase significantly when the walking pace increased. The RMS accelerations along the AP and ML directions (see Figure 1) were significantly lower for the older group. There was no significant difference between the groups when comparing stride regularity and variability. Technology acceptance was assessed using a 15-item questionnaire. There were significant differences (ρ < 0.05) between the older and younger adults’ responses to seven items. The older adults found the “ability to learn how to use the bracelet” and “family support” more important. They also found items relating to the risk of harming the body,
Sensors 2022, 22, 573 7 of 39
measurement accuracy, costs and protecting the privacy of personal data less important than the younger adults. In addition, the older adults were less concerned with the bracelet being inconspicuous, i.e., not clearly visible or attracting attention. The evaluation resulted in a number of possible enhancemen
Collepals.com Plagiarism Free Papers
Are you looking for custom essay writing service or even dissertation writing services? Just request for our write my paper service, and we'll match you with the best essay writer in your subject! With an exceptional team of professional academic experts in a wide range of subjects, we can guarantee you an unrivaled quality of custom-written papers.
Get ZERO PLAGIARISM, HUMAN WRITTEN ESSAYS
Why Hire Collepals.com writers to do your paper?
Quality- We are experienced and have access to ample research materials.
We write plagiarism Free Content
Confidential- We never share or sell your personal information to third parties.
Support-Chat with us today! We are always waiting to answer all your questions.
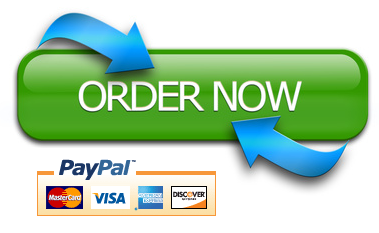