One page per article 1. What were the main findings in the article? 2. To which of the above four factors (factors that are
Based on the two articles provided summarize and answer the following questions for each article for factors related to pay differences. Use APA format and cite the articles. One page per article
1. What were the main findings in the article?
2. To which of the above four factors (factors that are bolded) are the findings of the article providing support? Why?
3. How can the information from the article be helpful to management or job-seekers?
207 © Blackwell PublishingLtd and theDepartment ofEconomics,University ofOxford, 2010.PublishedbyBlackwell PublishingLtd, 9600 Garsington Road, Oxford OX4 2DQ, UK and 350 Main Street, Malden, MA02148, USA.
OXFORD BULLETIN OF ECONOMICSAND STATISTICS, 73, 2 (2011) 0305-9049 doi:10.1111/j.1468-0084.2010.00613.x
Preferences,ComparativeAdvantage, and CompensatingWageDifferentials forJob Routinization*
Climent Quintana-Domeque
Departament de Fonaments de l’Anàlisi Econòmica, Universitat d’Alacant, Campus de Sant Vicent del Raspeig, 03690, Alacant, Spain (e-mail:[email protected])
Abstract Iattempt toexplainwhythere isnotmuchevidenceoncompensatingwagedifferentials for job disamenities. I focus on the match between workers’preferences for routine jobs and thevariability in tasksassociatedwith the job.UsingdatafromtheWisconsinLongitudinal Study, I find that mismatched workers earn lower wages and that both male and female workers in routinized jobs earn, on average, 5.5% and 7% less than their counterparts in non-routinized jobs. However, once preferences and mismatch are accounted for, this differencedecreases to2%formen,and4%forwomen,not statistically significant inboth cases.
I. Introduction Formore than30years, labour economists havebeen trying tofindevidenceofwagepre- miumsfor jobs that involvesuchdisamenitiesasphysicaleffort, routinenatureof thework or job insecurity.According to the theory of compensating wage differentials, which goes back to Adam Smith and involves the framework of analysis outlined by Rosen (1974),
ÅThis article is a revised version of Chapter 1 of my Ph.D. dissertation at Princeton University. I would like to thank my advisor,Alan Krueger, who has always been exceptionally generous with his advice. I am also extremely grateful to Jesse Rothstein for his insights and suggestions. I am particularly indebted to Carlos Bozzoli and Marco Gonzalez-Navarro for their many thoughtful remarks. Thanks also go to the Editor Beata Javorcik and the seminar participants at Princeton University, Universitat d’Alacant, Universitat de les Illes Balears, SAE 2007 Meetings in Granada and Universidad Pablo de Olavide. Special thanks go to an anonymous referee of the Oxford Bulletin of EconomicsandStatisticswhosecomments, insightsandsuggestionsmade theconceptual frameworkshorter, clearer and neater. I also want to thank Erik Plug for providing me with the codes used in his previous work. Financial support from theRafael delPinoFoundation, theBankofSpain and theSpanishMinistryofScience and Innovation (ECO2008-05721/ECON) is gratefully acknowledged. The usual disclaimers apply. Previous versions of this paper are circulated as Industrial Relations Section Working Paper 525, Princeton University, and IVIE Working Paper WP-AD 2010-06. This research uses data from the Wisconsin Longitudinal Study (WLS) of the University of Wis- consin-Madison. Since 1991, theWLS has been supported principally by the National Institute onAging (AG-9775 andAG-21079), with additional support from the Vilas Estate Trust, the National Science Foundation, the Spencer Foundationand theGraduateSchoolof theUniversityofWisconsin-Madison.Apublicusefileofdata fromtheWLS is available from the University ofWisconsin-Madison, 1180 Observatory Drive, Madison,Wisconsin 53706 and at http://www.ssc.wisc.edu/∼wls/data/. The opinions expressed herein are those of the authors. JELClassification numbers: J3, J31.
208 Bulletin
workersmust receiveawagepremiumforsuffering fromjobdisamenities, ceterisparibus. However, a surveyof theevidencehasconcluded that ‘testsof the theoryofcompensating wage differentials are inconclusive with respect to every job characteristic except risk of death’(Borjas, 2005, Ch. 6, p. 224). It is obvious that on-the-job risk of death is an undesirable job characteristic, and the
availableempirical evidence indeedsuggests thatwagesarepositivelyassociatedwithon- the-jobriskofdeath(ViscusiandAldy,2003).However,manyother jobcharacteristicsare not regarded as intrinsically undesirable by all workers. Instead, the desirability of a large number of job attributes depends crucially on individual workers’ tastes or personalities. Smith(1979)notes that theheterogeneityofworkers’tastesmaketestingforcompensating wage differentials difficult. At first glance, preference heterogeneity may seem consistent with mixed results for
repetitivework.Forexample,Lucas (1977)findsevidenceof significant compensation for repetitivework,whileBrown(1980) reportsanegativeestimate.Almost20years later, the mixed results are even more striking. Daniel and Sofer (1998) present some such results in their paper. One straightforward way to account for preference heterogeneity when looking for
compensating wage differentials is to run separate wage regressions for workers with different preferences. Still, as I show in section II, non-routine-preferring workers earn lower wages in routinized jobs, which is contrary to what the theory of compensating wage differentials would predict. Therefore, preference heterogeneity by itself does not explain the puzzle of compensating wage differentials. Why,evenafteraccountingforpreferenceheterogeneity,arecompensatingwagediffer-
entialsnotobservedor incorrectlysigned?What ifworkers’preferences forone typeof job (or job attribute) are related to their productivity in performing that type of job?Workers’ tastes for a certain job attribute may correlate with their comparative advantage in such jobs. This is not the same as saying that preferences can have a direct effect on wages, independent of the type of job; i.e. workers with different preferences may have differ- ent absolute advantages in performing any job. Rather, the key insight here is that when workers’ preferences do not match job attributes, they are less productive. For example, non-routine-preferring workers are likely to be more productive in non-routinized jobs than routine-preferring workers. By the same token, routine-preferring workers are likely to be more productive in routinized jobs than non-routine-preferring workers. If matching were perfect and each worker was assigned to a job according to com-
parative advantage, then the marginal routine-preferring worker would be willing to pay for working in a routinized job. Similarly, the marginal non-routine-preferring worker would need to be compensated for working in a routinized job. This would be consistent with the compensating wage differentials theory. However, as Lang and Majumdar (2004) pointed out, both casual empiricism and
researchshowthatmatchingis imperfect.Morerecently,Shimer(2007)acknowledgesthat skills and geographical location of workers are poorly matched with the skill requirement and locationof jobs: unemployedworkers are attached to anoccupation and ageographic location where jobs with their skills are currently scarce. Here, a similar point can be made. As I will show, a mismatch between workers’ preferences and job attributes does exist, and must be taken into account when looking for compensating wage differentials.
© Blackwell Publishing Ltd and the Department of Economics, University of Oxford 2010
Compensating wage differentials for job routinization 209
Indeed, my findings indicate that not accounting for mismatch in wage equations could bias compensating wage differentials estimates. I propose a simple assignment model with Nash bargaining over wages for analysing
the role of mismatch when looking for compensating wage differentials. Assuming that observed workers are not in long-run market equilibrium, all workers, no matter what their preferences are, need to be compensated if working in the sector with a shortage of workers in the absence of pay differentials. However, only mismatched workers, who are less productive because their sectors do not match their preferences, are penalized. This simple frameworkoffers a rationale for theexistenceofmixedestimates for com-
pensatingwagedifferentials. Indeed, in the literature, thestandardestimatesmayconfound theeffectonwagesofthejobattributebeinganalysedwiththeoneattributabletomismatch. Thispaper focusesonjobroutinization(i.e. jobs involvingrepetitiveandroutine tasks).
I consider this isan important jobattribute tostudybecauseestimates for it in the literature aremixed (e.g.Lucas, 1977;Brown, 1980;Daniel andSofer, 1998). So, this analysismay shednewlighton thesourcesof thesemixed results.Furthermore,Table1shows that29% of male workers and 36% of female workers report that ‘being able to do different things rather than thesamethingsoverandover’is ‘muchmore important thanhighpay’. Indeed, the table indicates that variability of tasks is one of the most highly valued characteristics on the job for workers. This suggests that it should be easier to find compensating wage differentials for job routinization than for other job attributes. Using data from the Wisconsin Longitudinal Study (WLS), I find that mismatched
workersearn lowerwages.Myresultsalso indicate thataccountingformismatch is impor- tant in obtaining more reliable estimates of compensating wage differentials. On average, male workers in routinized jobs are paid 5.5% less than workers in non-routinized jobs, after accounting for: differences in completed years of education, IQ measured at high school, highschool rank, adult cognition, tenure,occupationandfirmsize.Thisdifference decreases to4.5%after accounting fordifferences in thepreference for routinework.Fur- thermore, controlling formismatch reduces thedifference inaveragewagesbetweenmale workers in routinizedvs.non-routinized jobs to2%,and this isnot statistically significant. For female workers, the difference decreases from 7% to 4%. This paper is laid out as follows. Section II briefly describes the puzzle. It presents a
brief review of the compensating wage differentials literature, offers a description of the WLS dataset, and takes a first look at the data. Section III presents a model that sheds
TABLE 1
Percentage of currently employed individuals reporting that job characteristic is much more important than high pay, WLS 1992–93
Job characteristic Men Women Being able to do different things rather than the same things over and over 29 36 Being able to work without frequent checking by a supervisor 22 27 Having the opportunity to get on-the-job training 18 25 Having a job that other people regard highly 7 11 Being able to avoid getting dirty on the job 2 6
Source: Table 2 inAndrew et al. (2006), page 51.
© Blackwell Publishing Ltd and the Department of Economics, University of Oxford 2010
210 Bulletin
light on the puzzle. Section IV offers the empirical model. My results are in section V. SectionVI offers some robustness checks. Finally, sectionVII concludes.
II. The puzzle A brief review
More than twocenturies ago,AdamSmithnoted thatworkerswith the same level of com- petence should be paid different wages if their working conditions are different. Rosen (1974) formalizesAdam Smith’s ideas showing that, under perfect competition, identical workers need to be compensated for job disamenities. The standard method for testing the prediction of this theory is to estimate a wage
equation with characteristics of the job (z) and personal characteristics (p). In general, the equation is of the form:
ln(w)= � + �z + �p+ �. (1) The estimation of equation (1) using cross-sectional data identifies a market relationship between ln(w) and z. If the market relationship is linear, then � measures the marginal cost of the disamenity for any worker who is in his most preferred job in long-run market equilibrium. For an undesirable job attribute, the theory predicts that �>0. This iswhat is predictedby the standard theoryof compensatingdifferentials:workers
have heterogeneous preferences, and firms are heterogeneous with respect to the costs of providing good working conditions; in long-run equilibrium workers who value good conditions most are matched with firms that have the lowest costs of providing them, and in thesematchesconditionsaregoodandwagesare low.Conversely,highwagesandpoor conditionsareobservedinmatchesofworkerswhocarelesswithfirmsthathavehighcosts. This long-run relationship is the only thing that the standard theory gives us.1 However, theempirical evidenceoncompensatingwagedifferentials ismixed for jobcharacteristics other than the risk of death [see Rosen (1986) for a classical discussion on the theory of equalizing differences]. There have been several previous attempts at solving this puzzle. First, omitted vari-
ables can lead to biased estimates because of the correlation between unobserved skills, preferences for the job attribute under study, individual productivities and the quality of workingconditions(e.g.Brown,1980;DuncanandHolmlund,1983;Garen,1988;Kostiuk, 1990; Hwang, Reed and Hubbard, 1992). Second, when working conditions are reported by the workers themselves, the estimates are likely to suffer from simultaneity bias (e.g. McNabb,1989).Further, ifanswerstosurveyquestionsaboutworkingconditionsaregiven in subjective terms, then the estimates are likely to suffer from subjectivity biases (e.g. McNabb, 1989). Finally, when worker conditions are defined using average occupation (or industry)characteristicsand thenmatched to individualworkers,misclassificationbias may arise. From an empirical perspective, in this paper I take into account most of these biases.
First, I control for preferences for the job attribute under study, and use IQ measured at
1I thank an anonymous referee of the Oxford Bulletin of Economics and Statistics for clarifying this issue.
© Blackwell Publishing Ltd and the Department of Economics, University of Oxford 2010
Compensating wage differentials for job routinization 211
high school and high school rank as proxies for unobserved skills and individual produc- tivities, and occupation and size of firm dummy variables to account for characteristics other than job routinization (the job attribute under study) that may be related to worker productivities. Second, job routinization is measured by time spent doing monotone tasks in order to circumvent the problem of subjectivity biases due to the use of answers given in subjective terms. Last but not least, I measure working conditions at the worker level, not at the occupation level, to avoid misclassification bias. From a theoretical point of view, this paper can be thought of as looking at the conse-
quenceof thepossibility thatobservedworkersarenot inalong-runequilibrium,providing anaccountof short-runwagedeterminationwhenworkers arenotperfectlymatched.This is the gap that this study aims to fill. I present a very simple model: workers are randomly assigned to jobs and wages are determined by Nash bargaining. The model highlights the effect of mismatch on wages, which must be taken into account when looking for compensating wage differentials. Istartbypresentingtheimplicationsofpreferenceheterogeneity(about theattractiveor
unattractive features of performing a job task) for estimates of compensating wage differ- entials. Suppose there are two types of workers: those who enjoy z (x =1) and those who havedistasteforz (x =0).Inthatcase, totest thetheoryofcompensatingwagedifferentials, the following regressions should be run:
ln(w)= �0 + �0z + �0p+ �0, if x =0 (2)
ln(w)= �1 + �1z + �1p+ �1, if x =1. (3)
If the theory is correct, I should find evidence on �0>0 and �1<0: workers who have distaste for z (x =0) are compensated for working in a job involving high levels of z, while workers who enjoy z (x =1) are willing to pay for working in a job involving high levels of z. With these predictions at hand, I can assess the existence of compensating wage differentials for job routinization depending on workers’preferences. Before taking a first look at the data, I provide a description of the dataset used in this paper.
Data
I use data from the WLS of the University of Wisconsin-Madison. The sample contains information on 10,317 men and women who graduated from Wisconsin high schools in 1957,approximatelyone-thirdofall seniors inWisconsinhighschools in1957. It contains a rich set of self-reported information fromsamplemembers, siblings andparents, aswell asadministrativedata, collected inaseriesof surveys:1957(graduates),1964(graduates), 1975 (graduates), 1977 (siblings), 1992–93 (graduates), 1993–94 (siblings) and 2003–05 (graduates and spouses). I focuson the1992–93waves,when respondentswere in their early50s.Thisdecision
is based on both informational requirements and sample (size and selectivity) consider- ations.Firstofall, informationonworkers’preferencesisnotavailablepriortothe1992–93 waves.Second,participationinthelabourmarket ishigherforpeople intheir50s(1992–93
© Blackwell Publishing Ltd and the Department of Economics, University of Oxford 2010
212 Bulletin
waves) than in their 60s (2003–05 waves): 92.4% of men were employed in 1992 while only47.8%ofthemwereemployedin2004.Finally, thishelpsmetominimizenon-random attrition problems. TheWLSdatasetoffers anopportunity for exploring the roleofmismatch inobserving
compensating wage differentials. It contains a set of individual characteristics obtained from the (graduate) respondents, such as IQ score measured at high school, high school rank, adult cognition, education, tenure, preference for job routinization, hourly wages, hours of work, number of hours performing different tasks on the job, etc. Moreover, the sample is quite homogeneous (high school graduates from Wisconsin high schools in 1957), which makes any concerns about omitted variables less important. Mysampleisrestrictedtoworkerswhowereemployedin1992.Unfortunately,employ-
mentstatusismissingfor1,824individuals.Thisimpliesadramaticdecreaseintheoriginal sample size from 10,317 to 8,493. There are 7,196 individuals employed in 1992. After restricting our sample size to those individuals having a positive hourly wage rate, the number of observations decreases to 6,756. Focusing only on Wisconsin residents, the sample decreases to 4,696.The sample also excludes individuals who were: working less than20hoursperweek, self-employed, employeesof their owncompanyor familywork- ers. Farm workers and members of the military also are excluded from my sample.After applying these restrictions, my working sample is left with approximately 3,800 observa- tions. The presence of extreme values in the wage distribution was detected accidentally through the comparison of average wages for men and women. To avoid the estimates being driven by extreme values in the wage distribution, I trim the tails of the log-wage distribution at both the 3% bottom and the 3% top. Finally, after dealing with missing observations for thevariablesused in theanalysis, theworkingsample size is about3,200. The next subsection presents the definition of the main variables used in the empirical analysis.
Definition of the main variables
The main variables in this paper are job routinization; worker’s preference for routine; and mismatch, i.e. the discrepancy between job routinization and worker’s preference for routine. In this subsection, I discuss how these variables are measured. The job routinization indicator (z) – whether a job is classified as routinized or non-
routinized – is constructed using the fraction of working time doing the same things over and over: job routinization is measured as 1 (routinized job) if the fraction of working time doing the same things over and over is equal to or higher than 0.5. Sensitivity anal- yses with alternative definitions of job routinization will be performed in the robustness checks section. I compute this fraction as the ratio of the number of weekly hours doing the same things over and over on the job to the total number of weekly working hours. Note that the reportednumberofhours canbecomparedacross individuals; this addresses standard subjectivity bias concernsdue toworkers’subjective assessments aboutworking conditions.Moreover, the fact that thenumberofhoursworked is reportedby theworkers themselves confronts the misclassification bias that is attributable to imprecise matching of average job (occupationor industry) characteristics to individualswhose jobcharacter- isticsmaydepart (byand large) fromtheaveragecharacteristicswithin theiroccupationor
© Blackwell Publishing Ltd and the Department of Economics, University of Oxford 2010
Compensating wage differentials for job routinization 213
industry.Ofcourse, as inprevious studies, simultaneitybiasesmayexist:workerswhoare unhappy with earnings that they receive may also respond negatively when asked about job attributes (McNabb, 1989). The worker’s preference for routine indicator (x) – whether a worker is classified as a
routine-preferringworkeroranon-routine-preferringworker–ismeasuredbytheresponse to this question: ‘To what extent do you see yourself as someone who prefers work that is routine and simple?’ The possible answers to this question are: agree strongly, agree moderately, agree slightly, neither agree nor disagree, disagree slightly, disagree moder- ately, disagree strongly.This is oneof thequestions asked in scoring thefive-factormodel of personality structure, and it is included in the personality section of the 1992–93 ques- tionnaire, separate from jobhistoryor current/last jobcharacteristics.Hence, thepotential concerns about framing effects are minimized. For workers who agree strongly, moder- ately or slightly, preferring work that is routine and simple, x =1. Sensitivity analyses with alternative definitions of worker’s preference for routine will be performed in the robustness checks section. Finally, mismatch between job routinization and worker’s preference for routine and
simplework ismeasuredas theabsolutevalueof thedifferencebetween z and x,m(z,x)= |z −x|. I adopt this approach because absolute value seems to be the most intuitive way of thinking about the discrepancy between two variables. Note that for binary indicators, the absolute-value deviation is equivalent to the quadratic deviation.
Descriptive statistics
Table2presents themaindescriptive statistics of theWLSsample for currently employed individuals (1992–93). A first glance at the table shows that, on average, male workers in non-routinized jobs earn $18.09 per hour, while male workers in routinized jobs earn $15.21: a difference of approximately $3 in the hourly wage. Women in non- routinized jobs earn $11.41 per hour, while women in routinized jobs earn $9.33. Although these are unadjusted averages, workers do not seem to be compensated for job routinization. The tablealsoshowsthat themajorityofmen(52%)work innon-routinized jobs,while
the majority of women work in routinized jobs (64%). At the same time, the fraction of workers who prefer routine and simple work is higher for women than for men: 0.24 vs. 0.18.Thefact thatworkers innon-routinizedjobsarenotcompensatedfor jobroutinization is evenmore strikinggiven that the supplyof routine-preferringworkers seems tobevery low(24%ofmaleworkers,18%of femaleworkers) incomparison to thedemandfor them (48% of male workers, 64% of female workers). Can mismatch explain the apparent lower wages in routinized jobs? The percentages
of well-matched workers (according to job routinization and preference for routine and simple work) are 62% and 53% for men and women, respectively. Hence, mismatch is higher forwomen (47%) than formen (38%).Forbothmenandwomen,mismatch isvery high.Moreover,mismatchmayberesponsible for (partof) thedifference inaveragewages between routinized and non-routinized jobs: mismatched men are paid $15.51 per hour while those who are well-matched are paid $17.44 per hour. For women the difference is smaller: $9.61 vs. $10.53.
© Blackwell Publishing Ltd and the Department of Economics, University of Oxford 2010
214 Bulletin
TABLE 2
Descriptive statistics, WLS 1992–93
Men Women
Obs. Mean SD Obs. Mean SD Hourly wage routinized jobs 800 15.21 4.98 1,111 9.33 3.46 Hourly wage non-routinized jobs 865 18.09 6.10 637 11.41 4.19 Job routinization (z =1 if fraction of weekly worked 1,665 0.48 0.50 1,748 0.64 0.48 hours doing the same things over and over is equal or higher than 0.5, z =0 otherwise)
Routine-preferring worker (Preference for routine 1,656 0.18 0.38 1,743 0.24 0.42 and simple work: x =1 if strongly/moderately/slightly agree, x =0 if strongly/moderately/slightly/disagree or neither agree nor disagree)
Mismatch, |z −x| 1,656 0.38 0.49 1,743 0.47 0.50 Fraction of weekly worked hours doing the 1,665 0.48 0.38 1,748 0.61 0.37 same things over and over
Preferences for routine and simple work Strongly agree 94 0.06 – 108 0.06 – Moderately agree 162 0.10 – 238 0.14 – Slightly agree 35 0.02 – 48 0.03 – Neither agree nor disagree 6 0.00 – 18 0.01 – Slightly disagree 59 0.04 – 68 0.04 – Moderately disagree 447 0.27 – 503 0.29 – Strongly disagree 853 0.52 – 760 0.44 –
Hourly wage mismatched workers 636 15.51 5.08 821 9.61 3.52 Hourly wage well-matched workers 1,020 17.44 6.04 922 10.53 4.12 Hourly wage 1,665 16.71 5.77 1,748 10.09 3.87 IQ (measured at high school) 1,665 98.95 14.35 1,748 100.10 13.89 High school rank 1,543 41.59 27.03 1,636 57.04 27.21 Education (years of completed education) 1,665 13.44 2.19 1,748 12.93 1.71 Adult cognition score (WAIS) 1,653 7.47 2.78 1,739 7.62 2.63 Tenure 1,659 19.34 11.00 1,744 12.09 9.00
Notes:Author’s calculations.
As expected, men are paid higher hourly wages than women: $16.71 vs. $10.09. Not surprisingly, given the cohort under study, born around 1940, women on average are less educated than men. Table 3 shows the distribution of workers (by their preferences for routine and simple
work) across jobs (by routinization) and the average hourly wages by worker-job type. Among men, 42% of non-routine-preferring workers are mismatched into routinized jobs (567/1359×100),while thispercentage is57 forwomen(758/1331×100).Forbothmen and women, the percentage of mismatched workers is lower in non-routinized jobs. This is consistent with the fact that the majority of men and women are non-routine-preferring workers (76%ofmen, and82%ofwomen).Regarding the averagehourlywage, the table describes an interesting feature of my data: there are no differences in average wages betweenmismatchedandwell-matched routineworkers. Indeed, thedifferencesare found only for non-routine-preferring workers.
© Blackwell Publishing Ltd and the Department of Economics, University of Oxford 2010
Compensating wage differentials for job routinization 215
TABLE 3
Distribution of workers across jobs and average hourly wages by worker-job type, WLS 1992–93
(Number of observations) z =0 z =1 Male x =0 48% 34%
18.4 15.7 (792) (567)
x =1 4% 14% 14.3 14.0 (69) (228)
Female x =0 33% 43%
11.8 9.7 (573) (758)
x =1 4% 20% 8.4 8.5 (63) (349)
Notes:Author’s calculations.
TABLE 4
Fraction of weekly worked hours doing the same things over and over by occupational category, WLS 1992–93
Occupational category Men Women Professional and technical specialty occupations 0.27 0.46 Executive, administrative and managerial occupations 0.27 0.41 Sales occupations 0.54 0.70 Administrative support occupations (including clerical) 0.63 0.65 Precision production, craft, and repair occupations 0.44 0.83 Operators and fabricators 0.79 0.86 Service occupations 0.64 0.79 Handlers,equipmentcleaners,helpers, labourers, farmoperators 0.73 0.90 farm workers and related occupations
Source:Author’s calculations.
A first look at the data
I start bymeasuring job routinizationas the fractionof timeatworkdoing the same things over and over. Routine-preferring workers (x =1) are defined as those individuals who strongly agree, moderately agree, slightly agree or neither agree nor disagree, with the statement ‘I see myself as someone who prefers work that is routine and simple’. Table 4 reports the degree of job routinization by occupational category for men and
women, respectively. As the table makes clear, ‘Professional and Technical Specialty Operations’, and ‘Executive,Administrative, and Managerial’occupational categories on average involve less routinization, while occupations such as ‘Operators and Fabricators’ involve more routinization of tasks. Another interesting feature that emerges from this table is that female workers tend to spend a higher fraction of time than male workers
© Blackwell Publishing Ltd and the Department of Economics, University of Oxford 2010
216 Bulletin
TABLE 5
Job routinization and wages by workers’ preferences. OLS estimates for men and women Men Women
Workers’ preferences Workers’ preferences Routine Non routine Routine Non routine (1) (2) (3) (4)
Job routinization 0.035 −0.082 0.004 −0.096 (0.052) (0.024) (0.046) (0.016)
Completed years of education 0.044 0.032 0.042 0.016 (0.016) (0.005) (0.015) (0.006)
IQ measured at high school 0.002 0.002 0.002 0.004 (0.002) (0.001) (0.002) (0.001)
High school rank 0.000 0.000 −0.000 0.000 (0.001) (0.005) (0.001) (0.000)
Adult cognition score −0.005 0.004 −0.002 0.001 (0.007) (0.003) (0.006) (0.004)
Tenure 0.009 0.008 0.016 0.013 (0.002) (0.001) …
,
WORKER LEARNING AND COMPENSATING DIFFERENTIALS
W. KIP VISCUSI and MICHAEL J. MOORE*
The authors hypothesize that in industries with relatively high levels of job-related injury risk, workers with longer job tenure will more clearly appreciate the degree of job risk than will newly hired workers, and will thus be more willing to accept lower wages in return for higher workers' compensation benefits. This hypothesis is confirmed by an analysis of quit behavior using 1981-83 data from the Michigan Panel Study of Income Dynamics and 1981-85 data from the National Institute of Occupational Safety and Health.
IN the standard compensating wagedifferential model, workers value their wage and workers' compensation compo- nents hased on full job risk information. Market forces generate positive wage differentials as ex ante compensation for exposure to relatively high risk. Similarly, market forces generate wage offsets for the increases in ex post risk compensation embodied in workers' compensation bene- fits.
These predictions can be modified to take into account potential imperfections in worker information, as in Viscusi (1979a,b, 1980a,b,d), where the role of learning is incorporated into the worker's decision model. The potential for learning about risks introduces a new market
* W. Kip Viscusi is George G. Allen Professor of Economics, Department of Economics, and Michael J. Moore is Associate Professor of Economics, Fuqua School of Business, Duke University. The first author's research was supported by the endowment of the G
Collepals.com Plagiarism Free Papers
Are you looking for custom essay writing service or even dissertation writing services? Just request for our write my paper service, and we'll match you with the best essay writer in your subject! With an exceptional team of professional academic experts in a wide range of subjects, we can guarantee you an unrivaled quality of custom-written papers.
Get ZERO PLAGIARISM, HUMAN WRITTEN ESSAYS
Why Hire Collepals.com writers to do your paper?
Quality- We are experienced and have access to ample research materials.
We write plagiarism Free Content
Confidential- We never share or sell your personal information to third parties.
Support-Chat with us today! We are always waiting to answer all your questions.
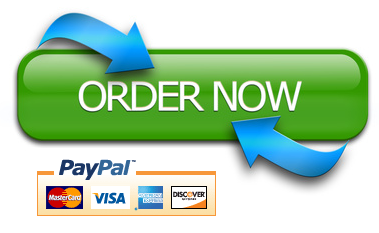