This is a Remote Sensing Test I uploaded its got 4 pages worth of questions. Please use the Internet and my uploads for answe
This is a Remote Sensing Test I uploaded its got 4 pages worth of questions. Please use the Internet and my uploads for answers. Thanks
GEOG 4770: Remote Sensing of the Environment
Exam 2
Due April 9
150 points
Instructions: You must complete the exam on your own. You may use any reference materials, including course text, your notes, lecture materials on Blackboard, etc. Answer the questions in your own words.
1. What is the difference between Manual Interpretation and Digital Classification of remotely sensed imagery? Give an example of each.
2. List and briefly explain the 7 general steps involved in the classification of remotely sensed images.
3. Discuss some of the main issues you will consider in developing a Classification Scheme (legend) for a remotely sensed image classification project.
4. What are the advantages and disadvantages of unsupervised classification?
5. What are the similarities and differences between Radar and Lidar?
6. Explain some of the advantages and disadvantages of:
a. Radar
b. Lidar
7. Explain some of the applications of Thermal Remote Sensing.
8. Explain (give examples where appropriate) the following types of resolution as it relates to remote sensing. Be sure to include why they are an important consideration in a remote sensing project.
a. Spatial
b. Spectral
c. Radiometric
d. Temporal
,
1
Active Remote Sensing
Radio Detection and Ranging = Radar
Light Detection and Ranging = Lidar
Passive vs. Active Remote Sensing • Passive remote sensing uses the energy from the sun
• Active remote sensing sends out its own energy and records how much bounces back
o Imaging Radar uses microwave wavelengths
o Lidar uses visible wavelength (laser) or sometimes NIR
Oil spill off NW coast of Spain
IKONOS image
Oil reaching shore
1
2
3
2
Radar image of oil spill off NW coast of Spain
(Black areas = oil)
Side-looking Radar
• Most radar systems do look off to the side (off-nadir)
o For military applications allows planes to fly over friendly territory and look into enemy territory
o More importantly, gives us more info about surface characteristics
• Radar is sensitive to surface roughness
Side-looking Radar
4
5
6
3
Side-looking Radar
Radar Terminology
• Swath width = range
• Direction of flight = azimuth
• Backscatter = reflectance
• Angle of view = depression angle
• Etc.—unique terminology
Depression Angle
Radar Geometry
7
8
9
4
Radar Geometry
Radar Advantages
• Can penetrate clouds
• Active, so can use day or night
• Less of a radiance vs. reflectance problem since you know exactly how much energy you send out and can measure what you get back—and atmosphere not a problem
• Can penetrate dry soil and get subsurface characteristics (e.g., archaeology)
Radar Disadvantages
• Developed by military, less civilian experience so far than passive remote sensing
• Difficult to interpret—complicated causes of reflectance
• Geometric distortions caused by side looking geometry
10
11
12
5
Radar bands were originally code names assigned by the military:
P-band
L-band
S-band
C-band
X-band
K-band
Radar Bands
Radar penetration increases with wavelength
Radar Bands
Interpretation of Radar Data
• Surface “smoothness” or “roughness” with respect to radar depends on wavelength and incident angle
o A smooth surface reflects in one direction (specular)
o A rough surface scatters radiation in all directions (Lambertian or diffuse)
o Rough surfaces tend to depolarize radiation
13
14
15
6
Surface Roughness
Shorter wavelength radar can resolve roughness more finely
Interpretation of Radar Data
Type of Backscatter (Reflection)
• Diffuse o Vegetation canopies
o Cross polarization (use HH and HV)
• Specular o Microwaves reflected away (not scattered) (e.g., oil slick)
• Corner reflectors o Tree trunks and buildings
o can make objects very “bright” or very “dark” depending on orientation
Surface roughness is described as a function of wavelength and the angle of incidence of the incoming radiation
Interpretation of Radar Data
16
17
18
7
Interpreting Radar Data
• Longer wavelength bands (P and L bands) o Penetrate canopy and reflects off of standing tree trunks
o Can detect amount of wood in a forest
o Estimate forest biomass
• Shorter wavelengths (C and X bands) o X band can detect leaves
o C (and L) band can detect twigs
Resolving Objects with Radar
• Radar resolution determined by…
o Size of area “illuminated” by microwaves at one time
• Depends on antenna length (longer antenna = better spatial resolution)
o But…difficult physically for airplane or satellite to carry a very large antenna.
• Depends on length of radar pulse (longer pulse = lower spatial resolution)
Pulse length in part determines radar resolution
Interpretation of Radar Data
19
20
21
8
Real Aperture vs. Synthetic
Aperture Radar (SAR) • Real aperture radar actually uses a single
antenna of a given length – resolution limited to what plane or satellite can carry.
• Synthetic Aperture Radar (SAR) can simulate a large antenna by taking advantage of the Doppler effect o Doppler shift allows sensor to identify
electromagnetic waves from ahead and behind the platform and therefore track an object for longer than it otherwise could, as if the antenna were longer.
Synthetic Aperture Radar – Doppler Effect
Interpretation of Radar Data
Radar Sensors • There are many imaging radar sensors available,
both airborne and on satellites o Most aircraft use SAR
o All satellites use SAR (to achieve reasonable spatial resolution)
22
23
24
9
Radar Sensors
Radar Applications • Forest inventory
• Oceanography
• Archaeology
• Sea ice studies
• Digital Elevation Models (DEMs)
• Urban mapping
• Wildfire studies
Radar Image – Angkor, Cambodia. Purple spots are possible buried ruins
Radar Applications
25
26
27
10
Radar image of oil slicks
Radar Applications
Radar derived elevation with TM draped over it
Radar Applications
Kamchatka Peninsula – Shuttle Radar Topography Mission (SRTM)
(Mission generated detailed topographic data for 80% of earth’s land surface)
Radar Applications
28
29
30
11
Lidar Remote Sensing • Like Radar but sends laser pulses instead of
microwave/radio pulses
• Can collect extremely accurate elevation data quickly (vs. ground survey)
• Typically flown on aircraft
High-resolution
LIDAR topography
Lidar Remote Sensing
Lidar vs. DEMs from topo sheets
31
32
33
12
landslides
Southern Bainbridge Island
34
35
,
1
Thermal Remote Sensing
Distinguishing materials on the ground using differences in emissivity and temperature
Landsat-based thermal change of Nisyros Island (volcanic)
Thermal = Emitted Infrared • IR = 0.76 um to 1000 um
– Reflective IR = 0.7 – 3.0 um – Thermal IR for remote sensing = 7 – 18 um
• Sometimes called far IR (vs. near and mid IR)
• Experiences almost no atmospheric scattering
• But…lots of absorption by atmospheric gases (e.g., CO2) – Must use atmospheric windows for rem. sens.
2
The Infrared portion of the electromagnetic spectrum
Emitted Thermal
Atmospheric transmission by λ
Thermal Properties of Objects
• All objects with temperature > 0o K emit thermal radiation – Amount depends on temperature (Stefan-
Boltzman Law) • M = εσT4
– Peak wavelength emitted also depends on temperature (Wien’s Displacement Law)
• Peak λ(µm) = 3000/T(oK)
3
Wien’s Displacement Law
Emissivity
• Emissivity is the ratio of the emittance of an object to that of a Black Body – A black body has ε = 1
– A white body has ε = 0
– Water has ε close to 1
– Most vegetation has ε close to 1
– Many minerals have ε << 1
• Can find tables of emissivities in reference books and textbooks
Kinetic Temperature vs. Radiant Temperature
• Kinetic temperature is caused by the vibration of molecules – sometimes called “true temperature”
– measured using conventional temperature scales (e.g. oF, oC, oK)
• Radiant temperature is the emitted energy of an object – sometimes called “apparent temperature”
– what we measure with thermal remote sensing
– depends on kinetic temperature and emissivity
4
Thermal Remote Sensing
• Incoming radiation from the sun is absorbed (converted to kinetic energy) and object emits EMR
• Objects vary in the amount of sun they “see” (different slopes, etc.) and in their emissivity
• Thermal remote sensing is sensitive to differences in emissivity.
Interpreting Thermal Images • Thermal images are often single-band and
look like black and white photographs – Bright areas = relatively warmer places – Dark areas = relatively cooler places – Can be the opposite for thermal weather
images!
• Must know if the image is a negative or a positive!
• Should know the time of day the image was acquired – day vs. night alters the interpretation
5
Atlanta — Daytime Atlanta — Nighttime
Daily change in radiant temperature of common objects
North
Thermal Infrared Multispectral Scanner (TIMS) image of Death Valley
Daytime Positive – Bright = warm, Dark = cool
6
Multi-band thermal
• Thermal imagery can also be multi-band (different parts of the thermal IR spectrum)
• When displayed in color, colors primarily represent differences in emissivity.
North
TIMS image of Death Valley made by combining thermal bands from different wavelengths after “decorrelation stretching”
Interpretation (cont.) • It is difficult to accurately calculate the
kinetic temperature of objects from their radiant temperature – Must know the emissivity of the target(s)
– Often have to estimate or assume emissivity values
7
Complicating Factors
• Topography (effects amount of incoming radiation from sun)
• Fine scale differences in emissivities of materials in scene
• Cloud cover history
• Precipitation history – differences in soil moisture
• Vegetation canopy geometry
• Geothermal areas
• Many others
Thermal Sensors
• Thermal Infrared Multispectral Scanner (TIMS) (Airborne – 18 m spatial res.)
• Landsat 3 MSS (237 m spatial resolution)
• Landsat TM (Band 6) (120 m spatial)
• Landsat ETM+ (Band 6) (60 m spatial)
• Landsat 8 (Band 10 and 11) (100 m spatial)
• ASTER (5 thermal bands at 90 m spatial)
• MODIS (many thermal bands at 1 km spatial resolution)
• Many others…
Applications
• Agricultural water stress (energy balance)
• Heat loss from urban areas
• Identifying and mapping materials based on their emissivities (e.g. minerals)
• Earthquake and volcanic activity prediction
• Mapping moisture amounts
• Ocean current mapping
• Plumes of warm water from power plants, etc.
• Atmospheric studies, weather forecasting, etc.
8
Evapotranspiration (ET) estimation using thermal RS
• If you know how much energy is being used to evaporate water, you can estimate how much water is evaporating!
E = H + L + r + G
Where E = irradiance, H = sensible heat, L = latent heat, r = reflected energy, and G = ground storage of energy.
– R
R
Thermal Image of Lava Flows
ASTER
9
Airborne thermal image of warm creek flowing into ocean near Anchorage, AK
ASTER images of San Francisco.
Bottom right is thermal image used for water temperature
Summary – Thermal Remote Sensing
• Typically used to map surface materials that differ in thermal properties (like emissivity)
• Usually NOT used to map absolute kinetic temperature
• Many applications but not especially good for distinguishing among vegetation types because all veg has about the same emissivity
• Gives us another tool to help distinguish materials that may be spectrally similar in the reflected wavelengths!
,
3/23/2020
1
Classification of Remotely Sensed Data
General Classification Concepts Unsupervised Classifications
What is Image Classification?
• Process of converting image pixels or regions to classes that represent self-similar features or “themes”
• Using images to create “thematic maps”
How?
• Two general approaches: – Manual interpretation (e.g., photointerpretation,
“heads-up digitizing”) – Digital classification (per pixel)
• Many digital techniques developed – Unsupervised classification – Supervised classification – Classification and Regression Trees (CART) – Neural Networks – Etc., etc., etc.
3/23/2020
2
General Classification Steps
Field reconnaissance Develop a classification scheme (legend) Enhance imagery (as needed) Use classification algorithms Incorporate ancillary data (as needed) Check accuracy of product Refine iteratively
Field Reconnaissance
• Critical for understanding the distribution of your theme in the real world
• Helps you choose useful ancillary data • Useful for understanding satellite imagery
back at the office • Nice to get out once in a while
3/23/2020
3
What characteristics of this landscape might be important for making a map using satellite data?
Developing Classification Schemes (Legends)
• How many types do you want to map? • How should you divide up the feature you are
interested in? • Can be very controversial!
Classification Schemes (List of types to map)
• What thematic classes are you going to assign pixels to?
1) Must be useful 2) Must be detectable using the data you have 3) Should be hierarchical 4) Categories must be mutually exclusive 5) Require explicit definitions of each class
3/23/2020
4
Classification Scheme — Example
I. Vegetated A. Forest
1. Evergreen a. Spruce-fir forest
i. Spruce-fir with winterberry understory
b. Lodgepole pine forest c. etc.
2. Deciduous
B. Shrubland
II. Non-Vegetated
Basic Classification Steps
1) Field reconnaissance 2) Development of classification scheme 3) Image enhancements (Veg indices, etc.) 4) Run classification algorithm 5) Incorporate ancillary data 6) Check accuracy of product 7) Refine iteratively
Classification Algorithms
• Procedures for grouping pixels and/or areas into the classes from your classification scheme
3/23/2020
5
Manual processing
• Aerial photos, print-outs of images, photos
– Transparent overlays – Delineate features (interpretation) – Compile maps – Generate reports
Digital processing
• Satellite images, digital or scanned photo • Digital on-screen interpretation (“Heads-up
digitizing”)
– Display geo-referenced image/photo on-screen – Digital line-drawing (with mouse or digital pen) to
delineate features • Analogous to drawing on an overlay
– Processed lines are converted to features in a Geographic Information System (GIS)
– Generate maps and reports
Detailed view of Wyoming GAP Land Cover Map
3/23/2020
6
Digital classification
• Conversion of pixel values into thematic classes – Statistical clustering of the data (lumping
spectrally similar pixels into the same class) – Spectral vs. informational classes – Sometimes combine spectral classes together
to make informational classes – Converting digital satellite data into meaningful
maps—the heart of remote sensing!
Use many bands at once to create a map of classes
Classification
General Types of Classifications
• Unsupervised – computer clusters pixels together based only on the similarity of their DNs.
• Supervised – computer uses training data— examples of target classes—and assigns pixels to the training class that they are most similar to.
• Others (neural networks, fuzzy logic etc).
3/23/2020
7
Classification Analogy
– Truck-load of fruits (pixels): Apples, oranges, kiwis, nectarines, bananas, pineapples, tangerines, plums, peaches, lemons (hundreds of each)
– Goal: separate them by type and put them in separate baskets (classes)
– Using a person (= computer) who has never seen these fruits before (!) or doesn’t know the difference between them
Unsupervised Classification
• Software Identifies natural groups (spectral classes) within multi-spectral data based on limited input from the analyst
• Pixel values are grouped based similarity of their DNs, radiance or reflectance – Pixels => Clusters
• Analyst has to match each cluster to a thematic class
Unsupervised Classification 2-bands
. .
.
.
.
.
. .
.. .
.. .
.
.
. . ..
.
.
. ..
. ..
… .
… .
.
.
.
.. . .
. .
.
.
.
.
.
. .
.. .
.. .
.
.
. . ..
.
.
. ..
. ..
… .
… .
.
.
.
.. . .
.
.
.
.
.
.
. .
.. .
.. .
.
.
. . ..
.
.
. ..
. ..
… .
.. .
.
.
.
Band X0 Max
M a x
Class
B an
d Y
3/23/2020
8
Unsupervised Classification 3-bands
1 pixel
1 Class
Unsupervised Classification
Unsupervised Classification
• Choose bands, indices, enhancements, etc. that highlight differences in your classes
• Decide how many classes to separate • Choose a grouping algorithm
– Simple clustering, K-means, etc.
• Classify the image • Group and evaluate the results
3/23/2020
9
Advantages of Unsupervised Classifications
• No extensive prior knowledge of map area required (but you have to label the classes!)
• Repeatable – objective classes are based only on spectral information
• Unique spectral classes recognized as units
Disadvantages of Unsupervised Classifications
• Spectral classes do not always correspond to informational classes
• Limited control over the output classes you end up with
• Spectral properties of informational classes change over time so you can’t always use same class statistics when moving from one image to another
Grouping Algorithms
• Statistical routines for grouping similar pixels together
• Differ in how they: – Determine what is similar (distance measures) – Determine the statistical center (centroid) of a
class – Test the distinctivness of classes
3/23/2020
10
Common Algorithms
• ISODATA • K-means Clustering
ISODATA
• ISODATA = Iterative Self-Organizing Data Analysis – Choose how many classes you want – The algorithm chooses class centers (centroid) by
spreading them evenly through the “data cloud” – Groups each pixel with nearest centroid – Calculates the centroid of the new cluster – Regroups each pixel with nearest new centroid – Keeps doing this until centroids don’t move much
K-means Clustering
• Like ISODATA but starts by picking centroids as far apart as possible from one another in the data cloud
• Iteratively groups pixels with the centroid and then re-calculates centroid
• Iterates until centroid stops moving
3/23/2020
11
K-means Clustering
Seed the Clusters
Assign Pixels
Move Centroids
Recalculate Cluster
Members
Unsupervised Classification – Summary
• Classification is the statistical clustering of pixels into groups
• Clusters => Thematic classes • Results should be checked and the
classification revised if necessary
3/23/2020
12
Task 1 – Unsupervised Classification
3/23/2020
13
37
Image Classification
Thematic information can be mapped and further analyzed.
• Satellite images “clustered” based on spectral similarities.
• These “clusters” are then assigned into a “theme” or class
38
Image Classification
Derives thematic information from spectral information • Reduces data volume
• Permits analysis of features
Class 1 Vegetation
Class 3 Water
Class 2 Urban
Water
Vegetation
Urban
39
Image Classification: K Means
This process organizes groups (clusters) of pixels with similar spectral responses
Spectral clusters (like land covers) are identified
K Means requires minimal input • # of desired classes
• Iterations
• Convergence threshold
3/23/2020
14
40
Image Classification: K Means
2. Minimum Distance calculations: Each pixel is associated with closest mean
Band 1
….. . . .
..
.. .
.
.
.
.
…
. …. .
. .
. .
.. . ..
.
. . ..
. . . . .
.. .. . . .
.
. . . . …
..
.
. . . .
.
.
.
.. . .
. . …
.
. . . .
. .
.
. .
.
Cluster Means 1. Means are initialized along diagonal
3. New mean calculated for each cluster and means migrate to new locations
2
4. Iterations continue until convergence or maximum iterations is reached
5. Each cluster associated with a value. Each pixel given this value
Task 2 – Renaming Classes
42
Labelling Classes
The process of identifying land cover classes and naming them
Label Water Forest Grass Agriculture Urban
ISODATA Class 1 Class 2 Class 3 Class 4 Class 5
Class Names
3/23/2020
15
43
Labelling Classes
Raster attribute editor Color assignments
Naming Classes
Making educated guesses
Forest
Water
Grass
Wheat
Forest1 (Decid)
Forest2 (Conif)
Forest3 (Mix)
Water1 (Deep)
Water2 (Shallow)
Task 3 – Recoding Classes
45
Recode or Merging Classes
This allows for the combining similar classes. For example:
Water 1(Silted) and
water 2 (clear)
may be RECODED to a new class of WATER
3/23/2020
16
46
Recode (Merge) Classes
Greater class delineation by selecting more classes than ultimately desired
The likelihood of mixed classes is reduced
Water
Land
Water Vegetation1
Vegetation2
Vegetation3
Vegetation4
Vegetation5
,
1
Resolution
Landsat ETM+ image
2
Types of Resolution
Spatial
Spectral
Radiometric
Temporal
Spatial Resolution
The dimension of a single pixel
The extent of the smallest object on the ground that can be distinguished in the imagery
Determined by the Instantaneous Field of View of satellite instruments (IFOV)
Determined by altitude and film characteristics for air photos.
Spatial Resolution
3
IFOV
1 pixel
Raster grid size
finer
Coarser
4
Available Resolution
Satellites: ~ .61 m to > 1 km
Air photos ~ <0.6 m to large.
Satellite data resolution
MODIS: 250 – 1000 m
Landsat MSS: 80 m
Landsat TM5, 7: 28.5 m
IRS MS: 22.5 m
SPOT: 20 m
ASTER: 15m
IRS Pan: 5 m
Quickbird Pan: 0.6 m pan
Quickbird (Digital Globe, Inc.)
~ 2.4 m spatial resolution in multispectral bands.
5
MODIS
500 m spatial resolution
Spatial Resolution Trade-offs
Data volume
Signal to Noise Ratio Dwell Time
“Salt and Pepper”
Money
Spectral Resolution
How finely an instrument “divides up” the range of wavelengths in the electromagnetic spectrum
How many spectral “bands” an instrument records
6
Spectral resolution
Related to the measured range of EMR
Wide range – coarse resolution
Narrow range – finer resolution
Case 1
Measure the EMR across a wide range
E.g., the visible portion of EMR
Assign a single DN for sum of all visible light energy hitting the sensor
Analogous to black and white (panchromatic) film
b lu e
g re e n
re d
0.4 0.70.60.5 UV Near-infrared
Case 1
7
Case 2
Measure EMR across narrower ranges
E.g., Blue, green and red bands
Assign a DN for each of these wavelength ranges to create 3 bands
Case 2
b lu e
g re e n
re d
0.4 0.70.60.5 UV Near-infrared
Coarser (lower) Spectral Resolution
Finer (higher) Spectral Resolution
RGB
Red Green Blue
8
400 500 600 700 800 900 1000 1100 1200 1300 1400 1500 1600 1700 1800 1900 2000 2100 2200 2300 2400 2500 0.0
0.1
0.2
0.3
0.4
0.5
0.6
0.7
0.8
400 500 600 700 800 900 1000 1100 1200 1300 1400 1500 1600 1700 1800 1900 2000 2100 2200 2300 2400 2500
0.0
0.2
0.4
0.6
0.8
High Spectral Resolution
Low Spectral Resolution
Wavelength (nm)
Wavelength (nm)
R e
fl e
c ta
n c e
R e
fl e
c ta
n c e
Spectral Resolution
Spectral Resolution Trade-Offs
Data Volume!
Signal to Noise Ratio
Processing complexity (time)
Money
9
Radiometric Resolution
How finely does the satellite divide up the radiance it receives in each band?
Usually expressed as number of bits used to store the maximum radiance 8 bits = 28 = 256 levels (usually 0 to 255)
64 levels (6 bit)
4 levels (2 bit)
Radiometric resolution
1 bit ( 0 – 1)
8 bit ( 0 – 255 )
16 bit ( 0 – 65,535 )
32 bit ( 0 – 4,294,967,295 ) & more
0: No EMR or below some minimum
value (threshold)
255: Max EMR or above some threshhold
for 8 bit data type
10
Radiometric resolution
8 bit data (256 values) Everything will be scaled from 0 – 255
Subtle details may not be represented
16 bit data (65,536 values) Wide range of choices
Required storage space will be twice that of 8 bit
Radiometric resolution
1 bit 2 ( coarse )
8 bit 256
16 bit 65536
32 bit 4 Billion
64 bit ( detailed )
Radiometric Radiation Trade Offs
Data volume
Signal to Noise Ratio
11
Calculating Image Size
Computer hard drives store data in “
Collepals.com Plagiarism Free Papers
Are you looking for custom essay writing service or even dissertation writing services? Just request for our write my paper service, and we'll match you with the best essay writer in your subject! With an exceptional team of professional academic experts in a wide range of subjects, we can guarantee you an unrivaled quality of custom-written papers.
Get ZERO PLAGIARISM, HUMAN WRITTEN ESSAYS
Why Hire Collepals.com writers to do your paper?
Quality- We are experienced and have access to ample research materials.
We write plagiarism Free Content
Confidential- We never share or sell your personal information to third parties.
Support-Chat with us today! We are always waiting to answer all your questions.
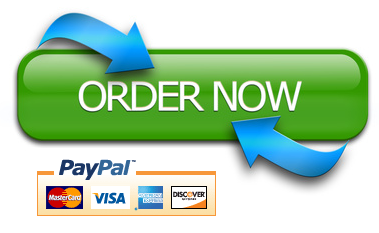