Probability
Exercise 2
Email Phishing is the fraudulent practice of sending emails purporting to be from reputable companies in order to induce individuals to reveal person information, such as passwords, credit card numbers, etc.
WT just recently sent the following email to students, faculty, and staff, warning them of possible email phishing scams:
For campus wide distribution: Employment SCAM Targeting College Students
Dear Students, Faculty, and Staff,
Recently we have had reports of scammers targeting students or using the University to promote their scams. Employment scams are very common and typically involve an online job advertisement or something similar. A detailed PSA provided by the FBI back in January is included in this email and it provides information as to how the employment scams work. I would also like to caution students when buying or selling items online or on Craigslist as scammers frequently use those avenues to conduct scams similar to the employment scams. Buy/Sell scams typically involve the scammer offering to purchase an item or items with a cashiers check, personal check, or money order. The method of payment (which is fraudulent) will typically be more than what the buyer or seller agreement is for the item and a request made by the scammer for the person to deposit the payment and wire the extra funds somewhere else. Or the buyer/seller will just provide a fraudulent method of payment and take the item.
Tips on how to protect yourself from this scam:
Never accept a job that requires depositing checks into your account or wiring portions to other individuals or accounts.
Many of the scammers who send these messages are not native English speakers. Look for poor use of the English language in e-mails such as incorrect grammar, capitalization, and tenses.
Use caution if the employer refuses to speak to you on the phone as most jobs require an interview.
Forward suspicious e-mails to the colleges IT personnel and report to the FBI and or UPD. Tell your friends to be on the lookout for the scam.
If you become the victim of a scam, make a police report. This may help you repair any potential credit damage as a result of the scam and can allow you to flag your credit to prevent further problems.
Sergeant Barbara Ferrara
Criminal Investigations Division
West Texas A&M University Police Department
WTAMU Box 60295
Canyon, TX 79016-0001
806-651-2318 Office
806-651-2310 Fax
Here is an example of a phishing email that Dr. Crisostomo received from Security [[email protected]].
Assignment:
Read article: Think About This Divine Providence and Spam (See below or can also be found in the textbook on pg. 163-164)
Explain how probability is used to filter email spam.
What is the difference between spam and a phishing email?
It would be beneficial to develop a way to filter out specifically phishing emails, as opposed to just spam, because of the severe consequences that can arise due to falling for a phishing email. Describe how you can use probability to create a program that would detect email phishing (i.e. what phrases would your program look for? Hint: View the sample phishing emails for key phrases).
Most people dont realize that probability is at play for your spam filter. Provide another example of something from your own life that also uses probability.
Submit your assignment to WTClass as a PDF or DOC, Times New Roman Font size 12, double spaced
See grading rubric below
Think about This Divine Providence and Spam
Would you ever guess that the essays Divine Benevolence: Or, An Attempt to Prove That the Principal End of the Divine Providence and Government Is the Happiness of His Creatures and An Essay Towards Solving a Problem in the Doctrine of Chances were written by the same person? Probably not, and in doing so, you illustrate a modern-day application of Bayesian statistics: spam, or junk mail filters.
In not guessing correctly, you probably looked at the words in the titles of the essays and concluded that they were talking about two different things. An implicit rule you used was that word frequencies vary by subject matter. A statistics essay would very likely contain the word statistics as well as words such as chance, problem, and solving. An eighteenth-century essay about theology and religion would be more likely to contain the uppercase forms of Divine and Providence.
Likewise, there are words you would guess to be very unlikely to appear in either book, such as technical terms from finance, and words that are most likely to appear in bothcommon words such as a, and, and the. That words would be either likely or unlikely suggests an application of probability theory. Of course, likely and unlikely are fuzzy concepts, and we might occasionally misclassify an essay if we kept things too simple, such as relying solely on the occurrence of the words Divine and Providence.
For example, a profile of the late Harris Milstead, better known as Divine, the star of Hairspray and other films, visiting Providence (Rhode Island), would most certainly not be an essay about theology. But if we widened the number of words we examined and found such words as movie or the name John Waters (Divines director in many films), we probably would quickly realize the essay had something to do with twentieth-century cinema and little to do with theology and religion.
We can use a similar process to try to classify a new email message in your in-box as either spam or a legitimate message (called ham, in this context). We would first need to add to your email program a spam filter that has the ability to track word frequencies associated with spam and ham messages as you identify them on a day-to-day basis. This would allow the filter to constantly update the prior probabilities necessary to use Bayes theorem. With these probabilities, the filter can ask, What is the probability that an email is spam, given the presence of a certain word?
Applying the terms of on page , such a Bayesian spam filter would multiply the probability of finding the word in a spam email, P(A|B), by the probability that the email is spam, P(B), and then divide by the probability of finding the word in an email, the denominator in . Bayesian spam filters also use shortcuts by focusing on a small set of words that have a high probability of being found in a spam message as well as on a small set of other words that have a low probability of being found in a spam message.
As spammers (people who send junk email) learned of such new filters, they tried to outfox them. Having learned that Bayesian filters might be assigning a high P(A|B) value to words commonly found in spam, such as Viagra, spammers thought they could fool the filter by misspelling the word as Vi@gr@ or V1agra. What they overlooked was that the misspelled variants were even more likely to be found in a spam message than the original word. Thus, the misspelled variants made the job of spotting spam easier for the Bayesian filters.
Other spammers tried to fool the filters by adding good words, words that would have a low probability of being found in a spam message, or rare words, words not frequently encountered in any message. But these spammers overlooked the fact that the conditional probabilities are constantly updated and that words once considered good would be soon discarded from the good list by the filter as their P(A|B), value increased. Likewise, as rare words grew more common in spam and yet stayed rare in ham, such words acted like the misspelled variants that others had tried earlier.
Even then, and perhaps after reading about Bayesian statistics, spammers thought that they could break Bayesian filters by inserting random words in their messages. Those random words would affect the filter by causing it to see many words whose P(A|B), value would be low. The Bayesian filter would begin to label many spam messages as ham and end up being of no practical use. Spammers again overlooked that conditional probabilities are constantly updated.
Other spammers decided to eliminate all or most of the words in their messages and replace them with graphics so that Bayesian filters would have very few words with which to form conditional probabilities. But this approach failed, too, as Bayesian filters were rewritten to consider things other than words in a message. After all, Bayes theorem concerns events, and graphics present with no text is as valid an event as some word, X, present in a message. Other future tricks will ultimately fail for the same reason. (By the way, spam filters use non-Bayesian techniques as well, which makes spammers lives even more difficult.)
Bayesian spam filters are an example of the unexpected way that applications of statistics can show up in your daily life. You will discover more examples as you read the rest of this book. By the way, the author of the two essays mentioned earlier was Thomas Bayes, who is a lot more famous for the second essay than the first essay, a failed attempt to use mathematics and logic to prove the existence of God.
Rubric
There is no minimum page requirement for this assignment, though students typically write 1.5 2 pages double spaced. Papers that under 1 page typically do not meet all assignment components, and do not score well. The grading rubric is as follows:
Student describes how probability is used to filter spam: 15 points
Student describes the difference between spam and phishing: 10 points
Student includes specific words and phrases that could be used in a phishing filter: 15 points
Student provides another example where probability is used: 5 points
Overall Organization (See rubric below): 5 points
Collepals.com Plagiarism Free Papers
Are you looking for custom essay writing service or even dissertation writing services? Just request for our write my paper service, and we'll match you with the best essay writer in your subject! With an exceptional team of professional academic experts in a wide range of subjects, we can guarantee you an unrivaled quality of custom-written papers.
Get ZERO PLAGIARISM, HUMAN WRITTEN ESSAYS
Why Hire Collepals.com writers to do your paper?
Quality- We are experienced and have access to ample research materials.
We write plagiarism Free Content
Confidential- We never share or sell your personal information to third parties.
Support-Chat with us today! We are always waiting to answer all your questions.
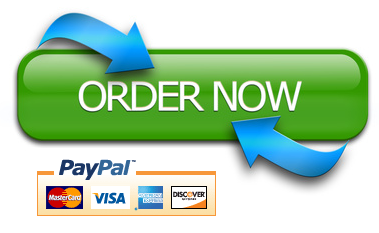